一、信号的收敛域与零极点图的关系
如果信号的拉普拉斯变换是:
X(s),假设它是一个有理的(即可以表示成两个多项式的比值):
X(s)=D(s)N(s)
令
N(s)=0,求出来的解是
X(s) 的零点。令
D(s)=0,求出的解是
X(s) 的极点。值得注意的是,解可以是实数,也可以是复数。 接下来,我们将零点在复平面上用圆圈表示;将极点在复平面上用交叉表示。如下图的一个例子所示:
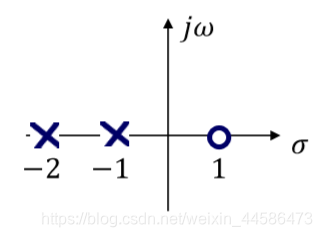
从这个图我们可以看出,
X(s) 的零点是:
s=1;极点是
s=−1,−2。所以据此,我们可以推导出
X(s) 的表达式,只不过有可能会相差一个常数系数:
X(s)=C(s+1)(s+2)s−1
下面我们给出
ROC(收敛域)与零极点图的关系性质:
- 对于右边信号而言,ROC一定位于
X(s) 最右边极点的右边。
- 对于左边信号而言,ROC一定位于
X(S) 最左边极点的左边。
- 对于双边信号而言,
ROC 可能是任意两个相邻极点之间的带状区域。
- 对于持续时间有限的信号,其
ROC 是整个平面。
二、拉普拉斯变换的特点
2.1 线性性
若:
x(t)
L X(s)R1,且ROC =
R1 ;
y(t)
L Y(s),且 ROC =
R2。
那么有:
ax(t)+by(t)
L aX(s)+bY(s),其中,新的ROC至少包含
R1∩R2
特别要注意的是:在极点抵消的时候会出现ROC变大的情况。那么下面我们给出一个判断什么时候新信号ROC变大的条件:如果
ax(t)+by(t)中,零点和极点有相互抵消的情况,那么其 ROC 就会变大。
我们看一个例子:
x(t)=δ(t)+e−tu(t);
y(t)=−e−tu(t),那么问
x(t)+y(t) 的拉普拉斯变换。
如果单独看每一个信号的拉氏变换:
x(t)
L 1+s+11;
y(t)
L −s+11
我们可以看到 ,
X(s) 的 ROC 是
Re{s}>−1,
Y(s) 的ROC是
Re{s}>−1。那么按理讲,信的信号的拉氏变换的 ROC 应该是
Re{s}>−1吧。可是,如果我们把整个表达式合起来分析,有:
x(t)+y(t)
L 1+s+11−s+11=1
所以真正的 ROC 应该是整个平面。即 ROC 扩大了。这是因为信号的极点被抵消了。
2.2 时移性
这个其实是和傅里叶变换的时移性一样的。表述如下:
若
x(t)
L X(s),且ROC =
R1 ;那么:
x(t−t0)
L X(s)e−st0,其 ROC 范围不变。
2.3 S域平移
若
x(t)
L X(s),且ROC =
R1 ;那么:
x(t)es0t
L X(s−s0)。其 ROC 将会变为 :
R+Re{s0}
2.4 尺度变换
若
x(t)
L X(s),且ROC =
R1 ,那么:
x(at)
L ∣a∣1X(as),相应地,ROC变为:
aR1.是扩展还是压缩就看
a 的值,如下图所示:
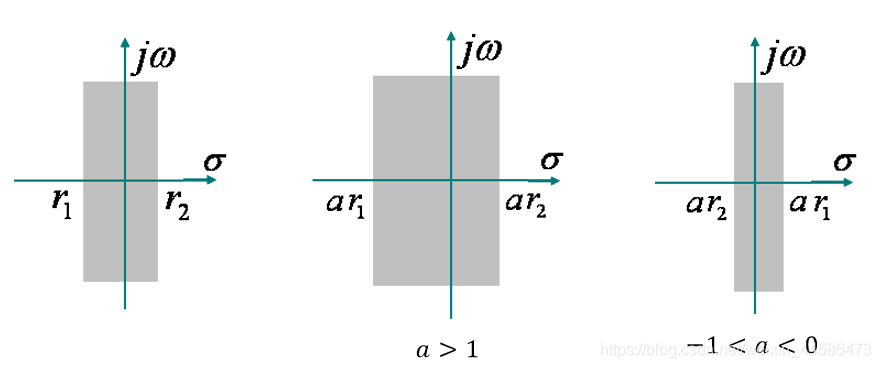
2.5 卷积性
若:
x(t)
L X(s),
y(t)
L Y(s),且其ROC分别是
R1,R2。那么,
x(t)∗y(t)
L X(s)Y(s),且其 ROC 至少包括
R1∩R2
同样的道理,这里的 ROC是至少包括
R1∩R2。因为在
X(s),Y(s) 相乘的时候,可能会发生分子分母相互约掉的情况,就会导致极点发生变化。而如果很不幸,这个被约掉的极点恰好就是决定ROC的极点,那么就会导致ROC的扩大。
2.6 时域微分性
若:
x(t)
L X(s),那么:
dtdx(t)
L sX(s),新的 ROC包括 R,但是也是可能会扩大。
2.7 时域积分
若
x(t)
L X(s),那么:
∫−∞tx(τ)dτ
L s1X(s),其 ROC 包括
R∩(Re{s}>0)
2.8 S域微分
若
x(t)
L X(s),那么
dsdX(s)
L −tx(t),S域微分的话,ROC区域是不会改变的。
OK!这一节暂时先写到这儿。这两天出
Blog 的速度会比平时慢一些,因为最近不少DDL要肝哈哈哈。大家互勉!共同加油吧!下一篇博客将会介绍拉普拉斯反变换以及利用零极点图对傅里叶变换进行几何求值的问题。See You!