k-近邻算法(kNN)---它的工作原理是:存在一个样本数据集合,也称做训练样本集,并且样本集中每个数据都存在标签,即我们知道样本集中每个数据与所属分类的对应关系。输入没有标签的新数据后,将新数据的每个特征与样本集中数据对应的特征进行比较,然后算法提取样本集中特征最相似数据(最近邻)的分类标签。一般来说,我们只选择样本数据集中前k个最相似的数据,这就是k-近邻算法中k的出处,通常k是不大于20的整数。最后,选择k个最相似数据中出现次数最多的分类,作为新数据得到分类。
1. 说明一下,文本数据格式:文本名字为:datingTestSet2.txt,该文件我放在项目里面的python文件同级
2. 使用python转化文件
1 def file2matrix(filename): 2 """ 3 Cynthia:解析文本数据,将属性数据放在returnMat,将标签放在classLabelVector
4 导入训练数据 5 :param filename: 数据文件路径 6 :return: 数据矩阵returnMat和对应的类别classLabelVector 7 """ 8 # 打开文件 9 fr = open(filename, 'r') 10 # 获得文件中的数据行的行数 11 numberOfLines = len(fr.readlines()) 12 # 生成对应的空矩阵 13 # 例如:zeros(2,3)就是生成一个 2*3 的矩阵,各个位置上全是 0 14 returnMat = zeros((numberOfLines, 3)) # prepare matrix to return 15 classLabelVector = [] # prepare labels return 16 fr = open(filename, 'r') 17 index = 0 18 for line in fr.readlines(): 19 # str.strip([chars]) --返回移除字符串头尾指定的字符生成的新字符串 20 line = line.strip() 21 # 以 '\t' 切割字符串 22 listFromLine = line.split('\t') 23 # 每列的属性数据,即 features 24 returnMat[index] = listFromLine[0: 3] 25 # 每列的类别数据,就是 label 标签数据 26 classLabelVector.append(int(listFromLine[-1])) 27 index += 1 28 # 返回数据矩阵returnMat和对应的类别classLabelVector 29 return returnMat, classLabelVector
扫描二维码关注公众号,回复:
6560469 查看本文章
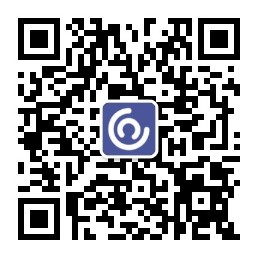
3. 数据归一化:
1 def autoNorm(dataSet): 2 """ 3 Desc: 4 归一化特征值,消除属性之间量级不同导致的影响 5 Args: 6 dataSet -- 需要进行归一化处理的数据集 7 Returns: 8 normDataSet -- 归一化处理后得到的数据集 9 ranges -- 归一化处理的范围 10 minVals -- 最小值 11 12 归一化公式: 13 Y = (X-Xmin)/(Xmax-Xmin) 14 其中的 min 和 max 分别是数据集中的最小特征值和最大特征值。该函数可以自动将数字特征值转化为0到1的区间。 15 """ 16 # 计算每种属性的最大值、最小值、范围 17 minVals = dataSet.min(0) 18 maxVals = dataSet.max(0) 19 # 极差 20 ranges = maxVals - minVals 21 normDataSet = (dataSet - minVals) / ranges 22 return normDataSet, ranges, minVals
4. KNN分类的算法核心:
1 def classify0(inX, dataSet, labels, k):
2 """ 3 Desc: 4 kNN 的分类函数 5 Args: 6 inX -- 用于分类的输入向量/测试数据 7 dataSet -- 训练数据集的 features 8 labels -- 训练数据集的 labels 9 k -- 选择最近邻的数目 10 Returns: 11 sortedClassCount[0][0] -- 输入向量的预测分类 labels 12 13 注意:labels元素数目和dataSet行数相同;程序使用欧式距离公式. 14 15 预测数据所在分类可在输入下列命令 16 kNN.classify0([0,0], group, labels, 3) 17 """ 18 19 # -----------实现 classify0() 方法的第一种方式---------------------------------------------------------------------------------------------------------------------------- 20 # 1. 距离计算 21 #shape函数是查看矩阵或者数组的维数 22 dataSetSize = dataSet.shape[0] 23 # tile生成和训练样本对应的矩阵,并与训练样本求差 24 #tile是用于对某个序列进行重复。 25 #示例:tile([1,2],(2,2,3)),输出为array([[[1, 2, 1, 2, 1, 2],[1, 2, 1, 2, 1, 2]] , [[1, 2, 1, 2, 1, 2],[1, 2, 1, 2, 1, 2]] ]) 26 #(1)可以看到是先将[1,2]重复3次得到[1, 2, 1, 2, 1, 2], 27 #(2)再将[1, 2, 1, 2, 1, 2]重复2次得到[[1, 2, 1, 2, 1, 2],[1, 2, 1, 2, 1, 2]], 28 #(3)最后再将[[1, 2, 1, 2, 1, 2],[1, 2, 1, 2, 1, 2]]重复两次[[[1, 2, 1, 2, 1, 2],[1, 2, 1, 2, 1, 2]] , [[1, 2, 1, 2, 1, 2],[1, 2, 1, 2, 1, 2]]]。 29 #总结:从上面的例子可以看出,tile(A,rep)按照rep里面从右到左的序列顺序对A进行重复,每对重复完一次,A就增加一个维度。 30 diffMat = tile(inX, (dataSetSize, 1)) - dataSet 31 #目的是计算出该测试点对训练集中的每个点的距离。 32 """ 33 欧氏距离: 点到点之间的距离 34 """ 35 # 取平方 36 sqDiffMat = diffMat ** 2 37 # 将矩阵的每一行相加 38 sqDistances = sqDiffMat.sum(axis=1) 39 # 开方 40 distances = sqDistances ** 0.5 41 # 根据距离排序从小到大的排序,返回对应的索引位置 42 # argsort() 是将x中的元素从小到大排列,提取其对应的index(索引),然后输出到y。 43 # print 'distances=', distances 44 sortedDistIndicies = distances.argsort() 45 # print 'distances.argsort()=', sortedDistIndicies 46 47 # 2. 选择距离最小的k个点 48 classCount = {} 49 for i in range(k): 50 # 找到该样本的类型 51 voteIlabel = labels[sortedDistIndicies[i]] 52 # 在字典中将该类型加一 53 # 字典的get方法 54 # 如:list.get(k,d) 其中 get相当于一条if...else...语句,参数k在字典中,字典将返回list[k];如果参数k不在字典中则返回参数d,如果K在字典中则返回k对应的value值 55 # l = {5:2,3:4} 56 # print l.get(3,0)返回的值是4; 57 # Print l.get(1,0)返回值是0; 58 # 感觉像Java中的键值对原理,键是这里的标签类型,值是标签出现的次数 59 classCount[voteIlabel] = classCount.get(voteIlabel, 0) + 1
60 # 3. 排序并返回出现最多的那个类型
61 # 字典的 items() 方法,以列表返回可遍历的(键,值)元组数组。 62 # 例如:dict = {'Name': 'Zara', 'Age': 7} print "Value : %s" % dict.items() Value : [('Age', 7), ('Name', 'Zara')] 63 # sorted 中的第2个参数 key=operator.itemgetter(1) 这个参数的意思是先比较第几个元素 64 # 例如:a=[('b',2),('a',1),('c',0)] b=sorted(a,key=operator.itemgetter(1)) >>>b=[('c',0),('a',1),('b',2)] 可以看到排序是按照后边的0,1,2进行排序的,而不是a,b,c 65 # b=sorted(a,key=operator.itemgetter(0)) >>>b=[('a',1),('b',2),('c',0)] 这次比较的是前边的a,b,c而不是0,1,2 66 # b=sorted(a,key=opertator.itemgetter(1,0)) >>>b=[('c',0),('a',1),('b',2)] 这个是先比较第2个元素,然后对第一个元素进行排序,形成多级排序。 67 sortedClassCount = sorted(classCount.items(), key=operator.itemgetter(1), reverse=True) 68 return sortedClassCount[0][0]
5. 用约会网站的数据进行训练中的数据标签测试
1 #分类器针对约会网站的进行测试代码 2 def datingClassTest(): 3 """ 4 Desc: 5 对约会网站的测试方法,并将分类错误的数量和分类错误率打印出来 6 Args: 7 None 8 Returns: 9 None 10 """ 11 # 设置测试数据的的一个比例(训练数据集比例=1-hoRatio) 12 hoRatio = 0.1 # 测试范围,一部分测试一部分作为样本 13 # 从文件中加载数据 14 datingDataMat, datingLabels = file2matrix("datingTestSet2.txt") # load data setfrom file 15 # 归一化数据 16 normMat, ranges, minVals = autoNorm(datingDataMat) 17 # m 表示数据的行数,即矩阵的第一维 18 m = normMat.shape[0] 19 # 设置测试的样本数量, numTestVecs:m表示训练样本的数量 20 numTestVecs = int(m * hoRatio) 21 print('numTestVecs=', numTestVecs) 22 errorCount = 0 23 for i in range(numTestVecs): 24 # 对数据测试 25 classifierResult = classify0(normMat[i], normMat[numTestVecs : m], datingLabels[numTestVecs : m], 3) 26 print("the classifier came back with: %d, the real answer is: %d" % (classifierResult, datingLabels[i])) 27 errorCount += classifierResult != datingLabels[i] 28 print("the total error rate is: %f" % (errorCount / numTestVecs)) 29 print(errorCount)
6. 做预测
1 def classifyPerson(): 2 resultList = ['not at all','in small doses', 'in large doses'] 3 percentTats = float(input("percentage of time spent playing video games?")) 4 ffMiles = float(input("frequent flier miles earned per years?")) 5 iceCream = float(input("liters of ice cream consumed per year?")) 6 datingDataMat, datingLabels = file2matrix("datingTestSet2.txt") 7 normMat, ranges, minVals = autoNorm(datingDataMat) 8 inArr = array([ffMiles, percentTats, iceCream]) 9 classifierResult = classify0((inArr-minVals)/ranges,normMat,datingLabels,3) 10 print("you will probably like this person:",resultList[classifierResult-1])
注:python3中的控制台交互是input
python2的是raw_input
控制台测试结果:
kNN代码测试已完毕。
本人github代码链接:https://github.com/CynthiaWendy/Machine-Learning-in-Action-KNN