(2)Self-supervised 6D Object Pose Estimation for Robot Manipulation(机器人操纵的自监督6D物体姿态估计)
(4)End-to-End 6DoF Pose Estimation From Monocular RGB Images(单目RGB图像的端到端6DoF姿态估计)
(5)Self6D: Self-Supervised Monocular 6D Object Pose Estimation(Self6D:自监督单眼6D物体姿态估计)
(6)Occlusion-Aware Self-Supervised Monocular 6D Object Pose Estimation(遮挡感知自监督单眼6D物体姿态估计)
(1)Robust 6D Object Pose Estimation by Learning RGB-D Features(基于RGB-D特征的鲁棒6D目标姿态估计)
(2)DenseFusion: 6D Object Pose Estimation by Iterative Dense Fusion (DenseFusion:基于迭代密集融合的6D目标姿态估计)
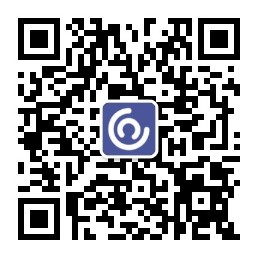
(3)Texture-less object detection and 6D pose estimation in RGB-D images (RGB-D图像中的无纹理目标检测和6D姿态估计)
(1)Semantic Segmentation for Thermal Images: A Comparative Survey(热图像语义分割的比较研究)
(3)ARTSeg: Employing Attention for Thermal images Semantic Segmentation(ARTSeg:关注热图像语义分割)
(2)PointRCNN: 3D Object Proposal Generation and Detection from Point Cloud(PointRCNN:从点云生成和检测3D对象建议)
(3)PV-RCNN: Point-Voxel Feature Set Abstraction for 3D Object Detection(PV-RCNN:用于3D对象检测的点体素特征集抽象)
(4)PointPillars: Fast Encoders for Object Detection from Point Clouds(PointPillars:用于点云目标检测的快速编码器)
1、单目:
(1)GDR-Net: Geometry-Guided Direct Regression Network for Monocular 6D Object Pose Estimation (GDR-Net:用于单目6D物体姿态估计的几何引导直接回归网络)
(2)Self-supervised 6D Object Pose Estimation for Robot Manipulation(机器人操纵的自监督6D物体姿态估计)
(3)Region Pixel Voting Network (RPVNet) for 6D Pose Estimation from Monocular Image(单目图像6D姿态估计的区域像素投票网络RPVNet)
Region Pixel Voting Network (RPVNet) for 6D Pose Estimation from Monocular Image
(4)End-to-End 6DoF Pose Estimation From Monocular RGB Images(单目RGB图像的端到端6DoF姿态估计)
End-to-End 6DoF Pose Estimation From Monocular RGB Images | IEEE Journals & Magazine | IEEE Xplore
(5)Self6D: Self-Supervised Monocular 6D Object Pose Estimation(Self6D:自监督单眼6D物体姿态估计)
(6)Occlusion-Aware Self-Supervised Monocular 6D Object Pose Estimation(遮挡感知自监督单眼6D物体姿态估计)
(7)CPS++: Improving Class-level 6D Pose and Shape Estimation From Monocular Images With Self-Supervised Learning (CPS++:利用自监督学习改进单目图像的类级6D姿态和形状估计)
2、RGBD:
(1)Robust 6D Object Pose Estimation by Learning RGB-D Features(基于RGB-D特征的鲁棒6D目标姿态估计)
(2)DenseFusion: 6D Object Pose Estimation by Iterative Dense Fusion (DenseFusion:基于迭代密集融合的6D目标姿态估计)
(3)Texture-less object detection and 6D pose estimation in RGB-D images (RGB-D图像中的无纹理目标检测和6D姿态估计)
https://www.sciencedirect.com/science/article/abs/pii/S0921889016308442
(4)Multi-view 6D Object Pose Estimation and Camera Motion Planning using RGBD Images(使用RGBD图像的多视图6D对象姿态估计和相机运动规划)
(5)Learning 6D Pose Estimation from Synthetic RGBD Images for Robotic Applications(用于机器人应用的合成RGBD图像学习6D姿态估计)
3、红外热成像:
(1)Semantic Segmentation for Thermal Images: A Comparative Survey(热图像语义分割的比较研究)
(2)A Generative Adversarial Network for Infrared and Visible Image Fusion Based on Semantic Segmentation(基于语义分割的红外可见图像融合生成对抗网络)
(3)ARTSeg: Employing Attention for Thermal images Semantic Segmentation(ARTSeg:关注热图像语义分割)
(4)MS-UDA: Multi-Spectral Unsupervised Domain Adaptation for Thermal Image Semantic Segmentation(MS-UDA:用于热图像语义分割的多光谱无监督域自适应)
4、激光雷达:
(1)ECPC-ICP: A 6D Vehicle Pose Estimation Method by Fusing the Roadside Lidar Point Cloud and Road Feature(ECPC-ICP:一种融合路边激光雷达点云和道路特征的6D车辆姿态估计方法)
(2)PointRCNN: 3D Object Proposal Generation and Detection from Point Cloud(PointRCNN:从点云生成和检测3D对象建议)
(3)PV-RCNN: Point-Voxel Feature Set Abstraction for 3D Object Detection(PV-RCNN:用于3D对象检测的点体素特征集抽象)
(4)PointPillars: Fast Encoders for Object Detection from Point Clouds(PointPillars:用于点云目标检测的快速编码器)
5、混合:
(1)CL3D: Camera-LiDAR 3D Object Detection With Point Feature Enhancement and Point-Guided Fusion(CL3D:具有点特征增强和点引导融合的摄像机LiDAR 3D目标检测)
(2)CLOCs: Camera-LiDAR Object Candidates Fusion for 3D Object Detection(CLOC:用于3D目标检测的摄像机LiDAR目标候选融合)
(3)CramNet: Camera-Radar Fusion with Ray-Constrained Cross-Attention for Robust 3D Object Detection(CramNet:基于光线约束交叉关注的相机-雷达融合用于鲁棒三维目标检测)