RGBD semantic segmentation
A paper list of RGBD semantic segmentation.
*Last updated: 2022/07/26
Update log
2020/May - update all of recent papers and make some diagram about history of RGBD semantic segmentation.
2020/July - update some recent papers (CVPR2020) of RGBD semantic segmentation.
2020/August - update some recent papers (ECCV2020) of RGBD semantic segmentation.
2020/October - update some recent papers (CVPR2020, WACV2020) of RGBD semantic segmentation.
2020/November - update some recent papers (ECCV2020, arXiv), the links of papers and codes for RGBD semantic segmentation.
2020/December - update some recent papers (PAMI, PRL, arXiv, ACCV) of RGBD semantic segmentation.
2021/February - update some recent papers (TMM, NeurIPS, arXiv) of RGBD semantic segmentation.
2021/April - update some recent papers (CVPR2021, ICRA2021, IEEE SPL, arXiv) of RGBD semantic segmentation.
2021/July - update some recent papers (CVPR2021, ICME2021, arXiv) of RGBD semantic segmentation.
2021/August - update some recent papers (IJCV, ICCV2021, IEEE SPL, arXiv) of RGBD semantic segmentation.
2022/January - update some recent papers (TITS, PR, IEEE SPL, arXiv) of RGBD semantic segmentation.
2022/March - update benchmark results on Cityscapes and ScanNet datasets.
2022/April - update some recent papers (CVPR, BMVC, IEEE TMM, arXiv) of RGBD semantic segmentation.
2022/May - update some recent papers of RGBD semantic segmentation.
2022/July - update some recent papers of RGBD semantic segmentation.
Datasets
The papers related to datasets used mainly in natural/color image segmentation are as follows.
- [NYUDv2] The NYU-Depth V2 dataset consists of 1449 RGB-D images showing interior scenes, which all labels are usually mapped to 40 classes. The standard training and test set contain 795 and 654 images, respectively.
- [SUN RGB-D] The SUN RGB-D dataset contains 10,335 RGBD images with semantic labels organized in 37 categories. The 5,285 images are used for training, and 5050 images are used for testing.
- [2D-3D-S] Stanford-2D-3D-Semantic dataset contains 70496 RGB and depth images as well as 2D annotation with 13 object categories. Areas 1, 2, 3, 4, and 6 are utilized as the training and Area 5 is used as the testing set.
- [Cityscapes] Cityscapes contains a diverse set of stereo video sequences recorded in street scenes from 50 different cities, with high quality pixel-level annotations of 5 000 frames in addition to a larger set of 20 000 weakly annotated frames.
- [ScanNet] ScanNet is an RGB-D video dataset containing 2.5 million views in more than 1500 scans, annotated with 3D camera poses, surface reconstructions, and instance-level semantic segmentations.
Metrics
The papers related to metrics used mainly in RGBD semantic segmentation are as follows.
- [PixAcc] Pixel accuracy
- [mAcc] Mean accuracy
- [mIoU] Mean intersection over union
- [f.w.IOU] Frequency weighted IOU
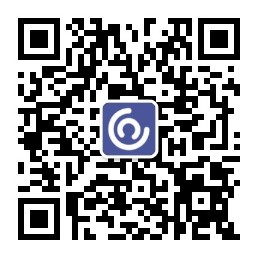
Performance tables
Speed is related to the hardware spec (e.g. CPU, GPU, RAM, etc), so it is hard to make an equal comparison. We select four indexes namely PixAcc, mAcc, mIoU, and f.w.IOU to make comparison. The closer the segmentation result is to the ground truth, the higher the above four indexes are.
NYUDv2
Method | PixAcc | mAcc | mIoU | f.w.IOU | Input | Ref. from | Published | Year |
---|---|---|---|---|---|---|---|---|
POR | 59.1 | 28.4 | 29.1 | RGBD | CVPR | 2013 | ||
RGBD R-CNN | 60.3 | 35.1 | 31.3 | 47(in LSD-GF) | RGBD | ECCV | 2014 | |
DeconvNet | 69.9 | 56.4 | 42.7 | 56 | RGB | LSD-GF | ICCV | 2015 |
DeepLab | 68.7 | 46.9 | 36.8 | 52.5 | RGBD | STD2P | ICLR | 2015 |
CRF-RNN | 66.3 | 48.9 | 35.4 | 51 | RGBD | STD2P | ICCV | 2015 |
Multi-Scale CNN | 65.6 | 45.1 | 34.1 | 51.4 | RGB | LCSF-Deconv | ICCV | 2015 |
FCN | 65.4 | 46.1 | 34 | 49.5 | RGBD | LCSF-Deconv | CVPR | 2015 |
Mutex Constraints | 63.8 | 31.5 | 48.5 (in LSD-GF) | RGBD | ICCV | 2015 | ||
E2S2 | 58.1 | 52.9 | 31 | 44.2 | RGBD | STD2P | ECCV | 2016 |
BI-3000 | 58.9 | 39.3 | 27.7 | 43 | RGBD | STD2P | ECCV | 2016 |
BI-1000 | 57.7 | 37.8 | 27.1 | 41.9 | RGBD | STD2P | ECCV | 2016 |
LCSF-Deconv | 47.3 | RGBD | ECCV | 2016 | ||||
LSTM-CF | 49.4 | RGBD | ECCV | 2016 | ||||
CRF+RF+RFS | 73.8 | RGBD | PRL | 2016 | ||||
RDFNet-152 | 76 | 62.8 | 50.1 | RGBD | ICCV | 2017 | ||
SCN-ResNet152 | 49.6 | RGBD | ICCV | 2017 | ||||
RDFNet-50 | 74.8 | 60.4 | 47.7 | RGBD | ICCV | 2017 | ||
CFN(RefineNet) | 47.7 | RGBD | ICCV | 2017 | ||||
RefineNet-152 | 73.6 | 58.9 | 46.5 | RGB | CVPR | 2017 | ||
LSD-GF | 71.9 | 60.7 | 45.9 | 59.3 | RGBD | CVPR | 2017 | |
3D-GNN | 55.7 | 43.1 | RGBD | ICCV | 2017 | |||
DML-Res50 | 40.2 | RGB | IJCAI | 2017 | ||||
STD2P | 70.1 | 53.8 | 40.1 | 55.7 | RGBD | CVPR | 2017 | |
PBR-CNN | 33.2 | RGB | ICCBS | 2017 | ||||
B-SegNet | 68 | 45.8 | 32.4 | RGB | BMVC | 2017 | ||
FC-CRF | 63.1 | 39 | 29.5 | 48.4 | RGBD | TIP | 2017 | |
LCR | 55.6 | 31.7 | 21.8 | 39.9 | RGBD | ICIP | 2017 | |
SegNet | 54.1 | 30.5 | 21 | 38.5 | RGBD | LCR | TPAMI | 2017 |
D-Refine-152 | 74.1 | 59.5 | 47 | RGB | ICPR | 2018 | ||
TRL-ResNet50 | 76.2 | 56.3 | 46.4 | RGB | ECCV | 2018 | ||
D-CNN | 56.3 | 43.9 | RGBD | ECCV | 2018 | |||
RGBD-Geo | 70.3 | 51.7 | 41.2 | 54.2 | RGBD | MTA | 2018 | |
Context | 70 | 53.6 | 40.6 | RGB | TPAMI | 2018 | ||
DeepLab-LFOV | 70.3 | 49.6 | 39.4 | 54.7 | RGBD | STD2P | TPAMI | 2018 |
D-depth-reg | 66.7 | 46.3 | 34.8 | 50.6 | RGBD | PRL | 2018 | |
PU-Loop | 72.1 | 44.5 | RGB | CVPR | 2018 | |||
C-DCNN | 69 | 50.8 | 39.8 | RGB | TNNLS | 2018 | ||
GAD | 84.8 | 68.7 | 59.6 | RGB | CVPR | 2019 | ||
CTS-IM | 76.3 | 50.6 | RGBD | ICIP | 2019 | |||
PAP | 76.2 | 62.5 | 50.4 | RGB | CVPR | 2019 | ||
KIL-ResNet101 | 75.1 | 58.4 | 50.2 | RGB | ACPR | 2019 | ||
2.5D-Conv | 75.9 | 49.1 | RGBD | ICIP | 2019 | |||
ACNet | 48.3 | RGBD | ICIP | 2019 | ||||
3M2RNet | 76 | 63 | 48 | RGBD | SIC | 2019 | ||
FDNet-16s | 73.9 | 60.3 | 47.4 | RGB | AAAI | 2019 | ||
DMFNet | 74.4 | 59.3 | 46.8 | RGBD | IEEE Access | 2019 | ||
MMAF-Net-152 | 72.2 | 59.2 | 44.8 | RGBD | arXiv | 2019 | ||
RTJ-AA | 42 | RGB | ICRA | 2019 | ||||
JTRL-ResNet50 | 81.3 | 60.0 | 50.3 | RGB | TPAMI | 2019 | ||
3DN-Conv | 52.4 | 39.3 | RGB | 3DV | 2019 | |||
SGNet | 76.8 | 63.1 | 51 | RGBD | TIP | 2020 | ||
SCN-ResNet101 | 48.3 | RGBD | TCYB | 2020 | ||||
RefineNet-Res152-Pool4 | 74.4 | 59.6 | 47.6 | RGB | TPAMI | 2020 | ||
TSNet | 73.5 | 59.6 | 46.1 | RGBD | IEEE IS | 2020 | ||
PSD-ResNet50 | 77.0 | 58.6 | 51.0 | RGB | CVPR | 2020 | ||
Malleable 2.5D | 76.9 | 50.9 | RGBD | ECCV | 2020 | |||
BCMFP+SA-Gate | 77.9 | 52.4 | RGBD | ECCV | 2020 | |||
MTI-Net | 75.3 | 62.9 | 49.0 | RGB | ECCV | 2020 | ||
VCD+RedNet | 63.5 | 50.7 | RGBD | CVPR | 2020 | |||
VCD+ACNet | 64.4 | 51.9 | RGBD | CVPR | 2020 | |||
SANet | 75.9 | 50.7 | RGB | arXiv | 2020 | |||
Zig-Zag Net (ResNet152) | 77.0 | 64.0 | 51.2 | RGBD | TPAMI | 2020 | ||
MCN-DRM | 56.1 | 43.1 | RGBD | ICNSC | 2020 | |||
CANet | 76.6 | 63.8 | 51.2 | RGBD | ACCV | 2020 | ||
CEN(ResNet152) | 77.7 | 65.0 | 52.5 | RGBD | NeurIPS | 2020 | ||
ESANet | 50.5 | RGBD | ICRA | 2021 | ||||
LWM(ResNet152) | 81.46 | 65.24 | 51.51 | RGB | TMM | 2021 | ||
GLPNet(ResNet101) | 79.1 | 66.6 | 54.6 | RGBD | arXiv | 2021 | ||
ESOSD-Net(Xception-65) | 73.3 | 64.7 | 45.0 | RGB | arXiv | 2021 | ||
NANet(ResNet101) | 77.9 | 52.3 | RGBD | IEEE SPL | 2021 | |||
InverseForm | 78.1 | 53.1 | RGB | CVPR | 2021 | |||
FSFNet | 77.9 | 52.0 | RGBD | ICME | 2021 | |||
CSNet | 77.5 | 63.6 | 51.5 | RGBD | ISPRS JPRS | 2021 | ||
ShapeConv | 75.8 | 62.8 | 50.2 | 62.6 | RGBD | ICCV | 2021 | |
CI-Net | 72.7 | 42.6 | RGB | arXiv | 2021 | |||
RGBxD | 76.7 | 63.5 | 51.1 | RGBD | Neurocomput. | 2021 | ||
TCD(ResNet101) | 77.8 | 53.1 | RGBD | IEEE SPL | 2021 | |||
RAFNet-50 | 73.8 | 60.3 | 47.5 | RGBD | Displays | 2021 | ||
RTLNet | 77.7 | 53.1 | RGBD | IEEE SPL | 2021 | |||
H3S-Fuse | 78.3 | 53.5 | RGB | BMVC | 2021 | |||
EBANet | 76.82 | 51.51 | RGBD | ICCSIP | 2021 | |||
CANet(ResNet101) | 77.1 | 64.6 | 51.5 | RGBD | PR | 2022 | ||
ADSD(ResNet50) | 77.5 | 65.3 | 52.5 | RGBD | arXiv | 2022 | ||
InvPT | 53.56 | RGB | arXiv | 2022 | ||||
PGDENet | 78.1 | 66.7 | 53.7 | RGBD | IEEE TMM | 2022 | ||
CMX | 80.1 | 56.9 | RGBD | arXiv | 2022 | |||
RFNet | 80.1 | 64.7 | 53.5 | RGBD | IEEE TETCI | 2022 | ||
MTF | 79.0 | 66.9 | 54.2 | RGBD | CVPR | 2022 | ||
FRNet | 77.6 | 66.5 | 53.6 | RGBD | IEEE JSTSP | 2022 | ||
DRD | 51.0 | 38.2 | RGB | IEEE ICASSP | 2022 | |||
SAMD | 74.4 | 67.2 | 52.3 | 61.9 | RGBD | Neurocomput. | 2022 | |
BFFNet-152 | 47.5 | RGBD | IEEE ICSP | 2022 | ||||
MQTransformer | 49.18 | RGBD | arXiv | 2022 | ||||
GED | 75.9 | 62.4 | 49.4 | RGBD | MTA | 2022 | ||
LDF | 84.8 | 68.7 | 59.6 | RGB | MTA | 2022 | ||
PCGNet | 77.6 | 52.1 | RGBD | IEEE ICMEW | 2022 |
SUN RGB-D
Method | PixAcc | mAcc | mIoU | f.w.IOU | Input | Ref. from | Published | Year |
---|---|---|---|---|---|---|---|---|
FCN | 68.2 | 38.4 | 27.4 | RGB | SegNet | CVPR | 2015 | |
DeconvNet | 66.1 | 32.3 | 22.6 | RGB | SegNet | ICCV | 2015 | |
IFCN | 77.7 | 55.5 | 42.7 | RGB | arXiv | 2016 | ||
CFN(RefineNet) | 48.1 | RGBD | ICCV | 2017 | ||||
RDFNet-152 | 81.5 | 60.1 | 47.7 | RGBD | ICCV | 2017 | ||
RefineNet-Res152 | 80.6 | 58.5 | 45.9 | RGB | CVPR | 2017 | ||
3D-GNN | 57 | 45.9 | RGBD | ICCV | 2017 | |||
DML-Res50 | 42.3 | RGB | IJCAI | 2017 | ||||
HP-SPS | 75.7 | 50.1 | 38 | RGB | BMVC | 2017 | ||
FuseNet | 76.3 | 48.3 | 37.3 | RGBD | ACCV | 2017 | ||
LRN | 72.5 | 46.8 | 33.1 | RGB | arXiv | 2017 | ||
SegNet | 72.6 | 44.8 | 31.8 | RGB | MMAF-Net-152 | TPAMI | 2017 | |
B-SegNet | 71.2 | 45.9 | 30.7 | RGB | BMVC | 2017 | ||
LSD-GF | 58 | RGBD | CVPR | 2017 | ||||
TRL-ResNet101 | 84.3 | 58.9 | 50.3 | RGB | ECCV | 2018 | ||
CCF-GMA | 81.4 | 60.3 | 47.1 | RGB | CVPR | 2018 | ||
D-Refine-152 | 80.8 | 58.9 | 46.3 | RGB | ICPR | 2018 | ||
Context | 78.4 | 53.4 | 42.3 | RGB | TPAMI | 2018 | ||
D-CNN | 53.5 | 42 | RGBD | ECCV | 2018 | |||
G-FRNet-Res101 | 75.3 | 47.5 | 36.9 | RGB | arXiv | 2018 | ||
DeepLab-LFOV | 71.9 | 42.2 | 32.1 | RGB | TPAMI | 2018 | ||
PU-Loop | 80.3 | 45.1 | RGB | CVPR | 2018 | |||
C-DCNN | 77.3 | 50 | 39.4 | RGB | TNNLS | 2018 | ||
GAD | 85.5 | 74.9 | 54.5 | RGB | CVPR | 2019 | ||
KIL-ResNet101 | 84.8 | 58 | 52 | RGB | ACPR | 2019 | ||
PAP | 83.8 | 58.4 | 50.5 | RGB | CVPR | 2019 | ||
3M2RNet | 83.1 | 63.5 | 49.8 | RGBD | SIC | 2019 | ||
CTS | 82.4 | 48.5 | RGBD | ICIP | 2019 | |||
2.5D-Conv | 82.4 | 48.2 | RGBD | ICIP | 2019 | |||
ACNet | 48.1 | RGBD | ICIP | 2019 | ||||
MMAF-Net-152 | 81 | 58.2 | 47 | RGBD | arXiv | 2019 | ||
LCR-RGBD | 42.4 | RGBD | CVPRW | 2019 | ||||
EFCN-8s | 76.9 | 53.5 | 40.7 | RGB | TIP | 2019 | ||
DSNet | 75.6 | 32.1 | RGB | ICASSP | 2019 | |||
JTRL-ResNet101 | 84.8 | 59.1 | 50.8 | RGB | TPAMI | 2019 | ||
SCN-ResNet152 | 50.7 | RGBD | TCYB | 2020 | ||||
SGNet | 81.8 | 60.9 | 48.5 | RGBD | TIP | 2020 | ||
CGBNet | 82.3 | 61.3 | 48.2 | RGB | TIP | 2020 | ||
CANet-ResNet101 | 81.9 | 47.7 | RGB | arXiv | 2020 | |||
RefineNet-Res152-Pool4 | 81.1 | 57.7 | 47 | RGB | TPAMI | 2020 | ||
PSD-ResNet50 | 84.0 | 57.3 | 50.6 | RGB | CVPR | 2020 | ||
BCMFP+SA-Gate | 82.5 | 49.4 | RGBD | ECCV | 2020 | |||
QGN | 82.4 | 45.4 | RGBD | WACV | 2020 | |||
VCD+RedNet | 62.9 | 50.3 | RGBD | CVPR | 2020 | |||
VCD+ACNet | 64.1 | 51.2 | RGBD | CVPR | 2020 | |||
SANet | 82.3 | 51.5 | RGB | arXiv | 2020 | |||
Zig-Zag Net (ResNet152) | 84.7 | 62.9 | 51.8 | RGBD | TPAMI | 2020 | ||
MCN-DRM | 54.6 | 42.8 | RGBD | ICNSC | 2020 | |||
CANet | 82.5 | 60.5 | 49.3 | RGBD | ACCV | 2020 | ||
CEN(ResNet152) | 83.5 | 63.2 | 51.1 | RGBD | NeurIPS | 2020 | ||
AdapNet++ | 38.4 | RGBD | IJCV | 2020 | ||||
ESANet | 48.3 | RGBD | ICRA | 2021 | ||||
LWM(ResNet152) | 82.65 | 70.21 | 53.12 | RGB | TMM | 2021 | ||
GLPNet(ResNet101) | 82.8 | 63.3 | 51.2 | RGBD | arXiv | 2021 | ||
NANet(ResNet101) | 82.3 | 48.8 | RGBD | IEEE SPL | 2021 | |||
FSFNet | 81.8 | 50.6 | RGBD | ICME | 2021 | |||
CSNet | 82.0 | 63.1 | 52.8 | RGBD | ISPRS JPRS | 2021 | ||
ShapeConv(ResNet101) | 82.0 | 58.5 | 47.6 | 71.2 | RGBD | ICCV | 2021 | |
CI-Net | 80.7 | 44.3 | RGB | arXiv | 2021 | |||
RGBxD | 81.7 | 58.8 | 47.7 | RGBD | Neurocomput. | 2021 | ||
TCD(ResNet101) | 83.1 | 49.5 | RGBD | IEEE SPL | 2021 | |||
RAFNet-50 | 81.3 | 59.4 | 47.2 | RGBD | Displays | 2021 | ||
GRBNet | 81.3 | 45.7 | RGBD | TITS | 2021 | |||
RTLNet | 81.3 | 45.7 | RGBD | IEEE SPL | 2021 | |||
CANet(ResNet101) | 85.2 | 50.6 | RGBD | PR | 2022 | |||
ADSD(ResNet50) | 81.8 | 62.1 | 49.6 | RGBD | arXiv | 2022 | ||
PGDENet | 87.7 | 61.7 | 51.0 | RGBD | IEEE TMM | 2022 | ||
CMX | 83.3 | 51.1 | RGBD | IEEE TMM | 2022 | |||
RFNet | 87.3 | 59.0 | 50.7 | RGBD | IEEE TETCI | 2022 | ||
MTF | 84.7 | 64.1 | 53.0 | RGBD | CVPR | 2022 | ||
FRNet | 87.4 | 62.2 | 51.8 | RGBD | IEEE JSTSP | 2022 | ||
DRD | 48.9 | 39.5 | RGB | IEEE ICASSP | 2022 | |||
SAMD | 63.4 | RGBD | Neurocomput. | 2022 | ||||
BFFNet-152 | 86.7 | 44.6 | RGBD | IEEE ICSP | 2022 | |||
LDF | 85.5 | 68.3 | 47.5 | RGB | MTA | 2022 | ||
PCGNet | 82.1 | 49.0 | RGBD | IEEE ICMEW | 2022 |
2D-3D-S
Method | PixAcc | mAcc | mIoU | f.w.IOU | Input | Ref. from | Published | Year |
---|---|---|---|---|---|---|---|---|
Deeplab | 64.3 | 46.7 | 35.5 | 48.5 | RGBD | MMAF-Net-152 | ICLR | 2015 |
D-CNN | 65.4 | 35.9 | RGBD | CMX | ECCV | 2018 | ||
DeepLab-LFOV | 88.0 | 42.2 | 69.8 | RGB | PU-Loop | TPAMI | 2018 | |
D-CNN | 65.4 | 55.5 | 39.5 | 49.9 | RGBD | ECCV | 2018 | |
PU-Loop | 91.0 | 76.5 | RGB | CVPR | 2018 | |||
MMAF-Net-152 | 76.5 | 62.3 | 52.9 | RGBD | arXiv | 2019 | ||
3M2RNet | 79.8 | 75.2 | 63 | RGBD | SIC | 2019 | ||
ShapeConv | 82.7 | 60.6 | RGBD | CMX | ICCV | 2021 | ||
CMX | 82.6 | 62.1 | RGBD | arXiv | 2022 |
Cityscapes
Benchmark Suite – Cityscapes Dataset
ScanNet
Benchmark Results - ScanNet Benchmark (2D Semantic label benchmark)
Paper list
- [POR] Gupta, S., et al. (2013). Perceptual Organization and Recognition of Indoor Scenes from RGB-D Images. IEEE Conference on Computer Vision and Pattern Recognition: 564-571. [Paper] [Code]
- [RGBD R-CNN] Gupta, S., et al. (2014). Learning Rich Features from RGB-D Images for Object Detection and Segmentation. European Conference on Computer Vision: 345-360. [Paper] [Code]
- [FCN] Long, J., et al. (2015). Fully convolutional networks for semantic segmentation. IEEE Conference on Computer Vision and Pattern Recognition: 3431-3440. [Paper] [Code]
- [CRF-RNN] Zheng, S., et al. (2015). Conditional Random Fields as Recurrent Neural Networks. IEEE International Conference on Computer Vision: 1529-1537. [Paper] [Code]
- [Mutex Constraints] Deng, Z., et al. (2015). Semantic Segmentation of RGBD Images with Mutex Constraints. IEEE International Conference on Computer Vision: 1733-1741. [Paper] [Code]
- [DeepLab] Chen, L., et al. (2015). Semantic Image Segmentation with Deep Convolutional Nets and Fully Connected CRFs. International Conference on Learning Representations. [Paper] [Code]
- [Multi-Scale CNN] Eigen, D. and R. Fergus (2015). Predicting Depth, Surface Normals and Semantic Labels with a Common Multi-scale Convolutional Architecture. IEEE International Conference on Computer Vision: 2650-2658. [Paper] [Code]
- [DeconvNet] Noh, H., et al. (2015). Learning Deconvolution Network for Semantic Segmentation. International Conference on Computer Vision: 1520-1528. [Paper] [Code]
- [LSTM-CF] Li, Z., et al. (2016). LSTM-CF: Unifying Context Modeling and Fusion with LSTMs for RGB-D Scene Labeling. European Conference on Computer Vision: 541-557. [Paper] [Code]
- [LCSF-Deconv] Wang, J., et al. (2016). Learning Common and Specific Features for RGB-D Semantic Segmentation with Deconvolutional Networks. European Conference on Computer Vision: 664-679. [Paper] [Code]
- [BI] Gadde, R., et al. (2016). Superpixel Convolutional Networks using Bilateral Inceptions. European Conference on Computer Vision: 597-613. [Paper] [Code]
- [E2S2] Caesar, H., et al. (2016). Region-Based Semantic Segmentation with End-to-End Training. European Conference on Computer Vision: 381-397. [Paper] [Code]
- [IFCN] Shuai, B., et al. (2016). Improving Fully Convolution Network for Semantic Segmentation. arXiv:1611.08986. [Paper] [Code]
- [CRF+RF+RFS] Thøgersen, M., et al. (2016). Segmentation of RGB-D Indoor Scenes by Stacking Random Forests and Conditional Random Fields. Pattern Recognition Letters 80, 208-215. [Paper] [Code]
- [SegNet] Badrinarayanan, V., et al. (2017). SegNet: A Deep Convolutional EnCoder-Decoder Architecture for Image Segmentation. IEEE Transactions on Pattern Analysis and Machine Intelligence 39(12): 2481-2495. [Paper] [Code]
- [LSD-GF] Cheng, Y., et al. (2017). Locality-Sensitive Deconvolution Networks with Gated Fusion for RGB-D Indoor Semantic Segmentation. IEEE Conference on Computer Vision and Pattern Recognition: 1475-1483. [Paper] [Code]
- [LCR] Chu, J., et al. (2017). Learnable contextual regularization for semantic segmentation of indoor scene images. IEEE International Conference on Image Processing: 1267-1271. [Paper] [Code]
- [RefineNet] Lin, G., et al. (2017). RefineNet: Multi-path Refinement Networks for High-Resolution Semantic Segmentation. IEEE Conference on Computer Vision and Pattern Recognition : 5168-5177, [Paper] [Code1] [Code2]
- [FuseNet] Hazirbas, C., et al. (2017). FuseNet: Incorporating Depth into Semantic Segmentation via Fusion-Based CNN Architecture. Asian Conference on Computer Vision: 213-228. [Paper] [Code]
- [STD2P] He, Y., et al. (2017). STD2P: RGBD Semantic Segmentation Using Spatio-Temporal Data-Driven Pooling. IEEE Conference on Computer Vision and Pattern Recognition: 7158-7167. [Paper] [Code]
- [RDFNet] Lee, S., et al. (2017). RDFNet: RGB-D Multi-level Residual Feature Fusion for Indoor Semantic Segmentation. IEEE International Conference on Computer Vision: 4990-4999. [Paper] [Code]
- [CFN(RefineNet)] Lin, D., et al. (2017). Cascaded Feature Network for Semantic Segmentation of RGB-D Images. IEEE International Conference on Computer Vision: 1320-1328. [Paper] [Code]
- [3D-GNN] Qi, X., et al. (2017). 3D Graph Neural Networks for RGBD Semantic Segmentation. IEEE International Conference on Computer Vision: 5209-5218. [Paper] [Code1] [Code2]
- [DML-Res50] Shen, T., et al. (2017). Learning Multi-level Region Consistency with Dense Multi-label Networks for Semantic Segmentation. Proceedings of the Twenty-Sixth International Joint Conference on Artificial Intelligence: 2708-2714. [Paper] [Code]
- [PBR-CNN] Zhang, Y., et al. (2017). Physically-Based Rendering for Indoor Scene Understanding Using Convolutional Neural Networks. IEEE Conference on Computer Vision and Pattern Recognition: 5057-5065. [Paper] [Code]
- [FC-CRF] Liu, F., et al. (2017). Discriminative Training of Deep Fully Connected Continuous CRFs With Task-Specific Loss. IEEE Transactions on Image Processing 26(5), 2127-2136. [Paper] [Code]
- [HP-SPS] Park, H., et al. (2017). Superpixel-based semantic segmentation trained by statistical process control. British Machine Vision Conference. [Paper] [Code]
- [LRN] Islam, M. A., et al. (2017). Label Refinement Network for Coarse-to-Fine Semantic Segmentation. arXiv1703.00551. [Paper] [Code]
- [G-FRNet-Res101] Islam, M. A., et al. (2018). Gated Feedback Refinement Network for Coarse-to-Fine Dense Semantic Image Labeling. arXiv:1806.11266 [Paper] [Code]
- [CCF-GMA] Ding, H., et al. (2018). Context Contrasted Feature and Gated Multi-scale Aggregation for Scene Segmentation. IEEE Conference on Computer Vision and Pattern Recognition: 2393-2402. [Paper] [Code]
- [Context] Lin, G., et al. (2018). Exploring Context with Deep Structured Models for Semantic Segmentation. IEEE Transactions on Pattern Analysis and Machine Intelligence, 40(6), 1352-1366. [Paper] [Code]
- [D-Refine-152] Chang, M., et al. (2018). Depth-assisted RefineNet for Indoor Semantic Segmentation. International Conference on Pattern Recognition: 1845-1850. [Paper] [Code]
- [D-depth-reg] Guo, Y. and T. Chen (2018). Semantic segmentation of RGBD images based on deep depth regression. Pattern Recognition Letters 109: 55-64. [Paper] [Code]
- [RGBD-Geo] Liu, H., et al. (2018). RGB-D joint modeling with scene geometric information for indoor semantic segmentation. Multimedia Tools and Applications 77(17): 22475-22488. [Paper] [Code]
- [D-CNN] Wang, W. and U. Neumann (2018). Depth-aware CNN for RGB-D Segmentation. European Conference on Computer Vision: 144-161. [Paper] Code
- [TRL-ResNet50/101] Zhang, Z., et al. (2018). Joint Task-Recursive Learning for Semantic Segmentation and Depth Estimation. European Conference on Computer Vision. [Paper] [Code]
- [DeepLab-LFOV] Chen, L., et al. (2018). DeepLab: Semantic Image Segmentation with Deep Convolutional Nets, Atrous Convolution, and Fully Connected CRFs. IEEE Transactions on Pattern Analysis and Machine Intelligence, 40(4), 834-848. [Paper] [Code]
- [PU-Loop] Kong, S. and C. Fowlkes (2018). Recurrent Scene Parsing with Perspective Understanding in the Loop. IEEE Conference on Computer Vision and Pattern Recognition: 956-965. [Paper] [Code]
- [PAD-Net] Xu, D., et al. (2018). PAD-Net: Multi-Tasks Guided Prediction-and-Distillation Network for Simultaneous Depth Estimation and Scene Parsing. IEEE Conference on Computer Vision and Pattern Recognition: 675-684. [Paper] [Code]
- [C-DCNN] Liu, J., et al. (2018) Collaborative Deconvolutional Neural Networks for Joint Depth Estimation and Semantic Segmentation. IEEE Transactions on Neural Networks and Learning Systems 29(11): 5655-5666. [Paper] [Code]
- [EFCN-8s] Shuai, B., et al. (2019). Toward Achieving Robust Low-Level and High-Level Scene Parsing. IEEE Transactions on Image Processing, 28(3), 1378-1390. [Paper] [Code]
- [3M2RNet] Fooladgar, F., and Kasaei, S. (2019). 3M2RNet: Multi-Modal Multi-Resolution Refinement Network for Semantic Segmentation. Science and Information Conference: 544-557. [Paper] [Code]
- [RFBNet] Deng, L., et al. (2019). RFBNet: Deep Multimodal Networks with Residual Fusion Blocks for RGB-D Semantic Segmentation. arXiv:1907.00135 [Paper] [Code]
- [MMAF-Net-152] Fooladgar, F. and S. Kasaei (2019). "Multi-Modal Attention-based Fusion Model for Semantic Segmentation of RGB-Depth Images." arXiv:1912.11691. [Paper] [Code]
- [LCR-RGBD] Giannone, G. and B. Chidlovskii (2019). Learning Common Representation from RGB and Depth Images. IEEE Conference on Computer Vision and Pattern Recognition Workshops. [Paper] [Code]
- [ACNet] Hu, X., et al. (2019). ACNET: Attention Based Network to Exploit Complementary Features for RGBD Semantic Segmentation. IEEE International Conference on Image Processing: 1440-1444. [Paper] [Code]
- [DSNet] Jiang, F., et al. (2019). DSNET: Accelerate Indoor Scene Semantic Segmentation. IEEE International Conference on Acoustics, Speech and Signal Processing: 3317-3321. [Paper] [Code]
- [GAD] Jiao, J., et al. (2019). Geometry-Aware Distillation for Indoor Semantic Segmentation*. IEEE Conference on Computer Vision and Pattern Recognition: 2864-2873. [Paper] [Code]
- [RTJ-AA] Nekrasov, V., et al. (2019). Real-Time Joint Semantic Segmentation and Depth Estimation Using Asymmetric Annotations. International Conference on Robotics and Automation: 7101-7107. [Paper] [Code]
- [CTS-IM] Xing, Y., et al. (2019). Coupling Two-Stream RGB-D Semantic Segmentation Network by Idempotent Mappings. IEEE International Conference on Image Processing: 1850-1854. [Paper] [Code]
- [2.5D-Conv] Xing, Y. J., et al. (2019). 2.5d Convolution for RGB-D Semantic Segmentation. IEEE International Conference on Image Processing: 1410-1414. [Paper] [Code]
- [DMFNet] Yuan, J., et al. (2019). DMFNet: Deep Multi-Modal Fusion Network for RGB-D Indoor Scene Segmentation. IEEE Access 7: 169350-169358. [Paper] [Code]
- [PAP] Zhang, Z., et al. (2019). Pattern-Affinitive Propagation Across Depth, Surface Normal and Semantic Segmentation. IEEE Conference on Computer Vision and Pattern Recognition: 4101-4110. [Paper] [Code]
- [KIL-ResNet101] Zhou, L., et al. (2019). KIL: Knowledge Interactiveness Learning for Joint Depth Estimation and Semantic Segmentation. Asian Conference on Pattern Recognition: 835-848. [Paper] [Code]
- [FDNet-16s] Zhen, M., et al. (2019). Learning Fully Dense Neural Networks for Image Semantic Segmentation. The Thirty-Third AAAI Conference on Artificial Intelligence: 9283-9290. [Paper] [Code]
- [JTRL-ResNet50/101] Zhang, Z., et al. (2019). Joint Task-Recursive Learning for RGB-D Scene Understanding. IEEE Transactions on Pattern Analysis and Machine Intelligence. [Paper] [Code]
- [3DN-Conv] Chen, Y., et al. (2019). 3D Neighborhood Convolution: Learning Depth-Aware Features for RGB-D and RGB Semantic Segmentation. International Conference on 3D Vision. [Paper] [Code]
- [SGNet] Chen, L.-Z., et al. (2020). Spatial Information Guided Convolution for Real-Time RGBD Semantic Segmentation. IEEE Transactions on Image Processing. [Paper] [Code]
- [SCN-ResNet101] Lin, D., et al. (2020). SCN: Switchable Context Network for Semantic Segmentation of RGB-D Images. IEEE Transactions on Cybernetics 50(3): 1120-1131. [Paper] [Code]
- [RefineNet-Res152-Pool4] Lin, G., et al. (2020). RefineNet: Multi-Path Refinement Networks for Dense Prediction. IEEE Transactions on Pattern Analysis and Machine Intelligence 42(5): 1228-1242. [Paper] [Code]
- [CANet-ResNet101] Tang, Q., et al. (2020). Attention-guided Chained Context Aggregation for Semantic Segmentation. arXiv:2002.12041. [Paper] [Code]
- [CGBNet] Ding, H., et al. (2020). Semantic Segmentation with Context Encoding and Multi-Path Decoding. IEEE Transactions on Image Processing 29: 3520-3533. [Paper] [Code]
- [TSNet] Zhou, W., et al. (2020). TSNet: Three-stream Self-attention Network for RGB-D Indoor Semantic Segmentation. IEEE Intelligent Systems. [Paper] [Code]
- [PSD-ResNet50] Zhou, L., et al. (2020). Pattern-Structure Diffusion for Multi-Task Learning. IEEE Conference on Computer Vision and Pattern Recognition. [Paper] [Code]
- [Malleable 2.5D] Xing, Y., et al. (2020). Malleable 2.5D Convolution: Learning Receptive Fields along the Depth-axis for RGB-D Scene Parsing. European Conference on Computer Vision. [Paper] [Code]
- [BCMFP+SA-Gate] Chen X., et al. (2020). Bi-directional Cross-Modality Feature Propagation with Separation-and-Aggregation Gate for RGB-D Semantic Segmentation. European Conference on Computer Vision. [Paper] [Code]
- [MTI-Net] Vandenhende S., et al. (2020). MTI-Net: Multi-Scale Task Interaction Networks for Multi-Task Learning. European Conference on Computer Vision. [Paper] [Code]
- [QGN] Kashyap C., et al. (2020). Quadtree Generating Networks: Efficient Hierarchical Scene Parsing with Sparse Convolutions. IEEE Winter Conference on Applications of Computer Vision. [Paper] [Code]
- [VCD+RedNet/ACNet] Xiong, Z.-T., et al. (2020). Variational Context-Deformable ConvNets for Indoor Scene Parsing. IEEE Conference on Computer Vision and Pattern Recognition. [Paper] [Code]
- [SANet] Yu, L., et al. (2020). Multi-layer Feature Aggregation for Deep Scene Parsing Models. arXiv:2011.02572. [Paper] [Code]
- [Zig-Zag Net] Lin, D., et al. (2020). Zig-Zag Network for Semantic Segmentation of RGB-D Images. IEEE Transactions on Pattern Analysis and Machine Intelligence 42(10): 2642-2655. [Paper] [Code]
- [MCN-DRM] Zheng, Z., et al. (2020). Multi-resolution Cascaded Network with Depth-similar Residual Module for Real-time Semantic Segmentation on RGB-D Images. IEEE International Conference on Networking, Sensing and Control (ICNSC). [Paper] [Code]
- [CANet] Zhou H., et al. (2020). RGB-D Co-attention Network for Semantic Segmentation. Asian Conference on Computer Vision. [Paper] [Code]
- [CEN] Wang, Y., et al. (2020). Deep Multimodal Fusion by Channel Exchanging. 34th Conference on Neural Information Processing Systems [Paper] [Code]
- [Z-ACN] Wu, Z., et al. (2020). Depth-Adapted CNN for RGB-D cameras. Asian Conference on Computer Vision. [Paper]
- [AdapNet++] Valada, A., et al. (2020). Self-Supervised Model Adaptation for Multimodal Semantic Segmentation. International Journal of Computer Vision [Paper] [Code]
- [ESANet] Seichter, D., et al. (2021). Efficient RGB-D Semantic Segmentation for Indoor Scene Analysis. IEEE International Conference on Robotics and Automation. [Paper] [Code]
- [LWM] Gu, Z., et al. (2021). Hard Pixel Mining for Depth Privileged Semantic Segmentation. IEEE Transactions on Multimedia. [Paper] [Code]
- [GLPNet] Chen, S., et al. (2021). Global-Local Propagation Network for RGB-D Semantic Segmentation. arXiv:2101.10801. [Paper] [Code]
- [ESOSD-Net] He, L., et al. (2021). SOSD-Net: Joint Semantic Object Segmentation and Depth Estimation from Monocular images. arXiv:2101.07422. [Paper] [Code]
- [NANet] Zhang, G., et al. (2021). Non-local Aggregation for RGB-D Semantic Segmentation. IEEE Signal Processing Letters. [Paper] [Code]
- [ARLoss] Cao, L., et al. (2021). Use square root affinity to regress labels in semantic segmentation. arXiv:2103.04990. [Paper] [Code]
- [InverseForm] Borse, S., et al. (2021). InverseForm: A Loss Function for Structured Boundary-Aware Segmentation. IEEE Conference on Computer Vision and Pattern Recognition. [Paper] [Code]
- [FSFNet] Su, Y., et al. (2021). Deep feature selection-and-fusion for RGB-D semantic segmentation. IEEE International Conference on Multimedia and Expo. [Paper] [Code]
- [3D-to-2D] Liu, Z., et al. (2021). 3D-to-2D Distillation for Indoor Scene Parsing. IEEE Conference on Computer Vision and Pattern Recognition. [Paper] [Code]
- [ATRC] Bruggemann, D., et al. (2021). Exploring Relational Context for Multi-Task Dense Prediction. International Conference on Computer Vision [Paper] [Code]
- [CSNet] Huan L., et al. (2021). Learning deep cross-scale feature propagation for indoor semantic segmentation. ISPRS Journal of Photogrammetry and Remote Sensing [Paper] [Code]
- [ShapeConv] Cao J., et al. (2021). ShapeConv: Shape-aware Convolutional Layer for Indoor RGB-D Semantic Segmentation. International Conference on Computer Vision [Paper] [Code]
- [CI-Net] Gao T., et al. (2021). CI-Net: Contextual Information for Joint Semantic Segmentation and Depth Estimation. arXiv:2107.13800 [Paper]
- [UMT] Du C., et al. (2021). Improving Multi-Modal Learning with Uni-Modal Teachers. arXiv:2106.11059 [Paper]
- [RGBxD] Cao J., et al. (2021). RGBxD: Learning depth-weighted RGB patches for RGB-D indoorsemantic segmentation. Neurocomputing [Paper]
- [TCD] Yue Y., et al. (2021). Two-Stage Cascaded Decoder for Semantic Segmentation of RGB-D Images. IEEE Signal Processing Letters [Paper]
- [RAFNet] Yan X., et al. (2021). RAFNet: RGB-D attention feature fusion network for indoor semantic segmentation. Displays. [Paper] [Code]
- [MAMD] RazzaghiYan P., et al. (2021). Modality adaptation in multimodal data. Expert Systems with Applications.[Paper] [Code]
- [FuseNet-3DEF] Terreran M., et al. (2022). Light deep learning models enriched with Entangled features for RGB-D semantic segmentation. Robotics and Autonomous Systems. [Paper] [Code]
- [GRBNet] Zhou W., et al. (2021). Gated-Residual Block for Semantic Segmentation Using RGB-D Data. IEEE Transactions on Intelligent Transportation Systems. [Paper] [Code]
- [RTLNet] Zhou W., et al. (2021). RTLNet: Recursive Triple-Path Learning Network for Scene Parsing of RGB-D Images. IEEE Signal Processing Letters. [Paper] [Code]
- [EBANet] Wang R., et al. (2021). EBANet: Efficient Boundary-Aware Network for RGB-D Semantic Segmentation. International Conference on Cognitive Systems and Signal Processing. [Paper]
- [CANet] Zhou H., et al. (2022). CANet: Co-attention network for RGB-D semantic segmentation. Pattern Recognition. [Paper] [Code]
- [ADSD] Zhang Y., et al. (2022). Attention-based Dual Supervised Decoder for RGBD Semantic Segmentation. arXiv:2201.01427 [Paper] [Code]
- [HS3] Borse S., et al. (2021). HS3: Learning with Proper Task Complexity in Hierarchically Supervised Semantic Segmentation. British Machine Vision Conference [Paper] [Code]
- [InvPT] Ye H., et al. (2022). Inverted Pyramid Multi-task Transformer for Dense Scene Understanding. arXiv:2203.07997 [Paper] [Code]
- [PGDENet] Zhou W., et al. (2022). PGDENet: Progressive Guided Fusion and Depth Enhancement Network for RGB-D Indoor Scene Parsing. IEEE Transactions on Multimedia [Paper] [Code]
- [CMX] Liu X., et al. (2022). CMX: Cross-Modal Fusion for RGB-X Semantic Segmentation with Transformers. arXiv:2203.04838 [Paper] [Code]
- [RFNet] Zhou W., et al. (2022). RFNet: Reverse Fusion Network With Attention Mechanism for RGB-D Indoor Scene Understanding. IEEE Transactions on Emerging Topics in Computational Intelligence [Paper] [Code]
- [MTF] Wang Y., et al. (2022). Multimodal Token Fusion for Vision Transformers. IEEE Conference on Computer Vision and Pattern Recognition. [Paper] [Code]
- [FRNet] Zhou W., et al. (2022). FRNet: Feature Reconstruction NetworkforRGB-DIndoor Scene Parsing. IEEE Journal of Selected Topics in Signal Processing. [Paper] [Code]
- [DRD] Fang T., et al. (2022). Depth Removal Distillation for RGB-D Semantic Segmentation. IEEE International Conference on Acoustics, Speech and Signal Processing (ICASSP). [Paper]
- [SAMD] Zhou F., et al. (2022). Scale-aware network with modality-awareness for RGB-D indoor semantic segmentation. Neurocomputing. [Paper]
- [BFFNet-152] He Y., et al. (2022). Bimodal Feature Propagation and Fusion for Realtime Semantic Segmentation on RGB-D Images. International Conference on Intelligent Computing and Signal Processing. [Paper]
- [MQTransformer] Xu Y., et al. (2022). Multi-Task Learning with Multi-Query Transformer for Dense Prediction. arXiv:2203.04838 [Paper]
- [GED] Zou W., et al. (2022). RGB‑D Gate‑guided edge distillation for indoor semantic segmentation. Multimedia Tools and Applications. [Paper]
- [LDF] Chen S., et al. (2022). Learning depth‑aware features for indoor scene understanding. Multimedia Tools and Applications. [Paper]
- [PCGNet] Liu H., et al. (2022). Pyramid-Context Guided Feature Fusion for RGB-D Semantic Segmentation. IEEE International Conference on Multimedia and Expo Workshops (ICMEW). [Paper]