1.简介
理论知识回顾
逻辑回归的主要公式罗列如下:
sigmoid(x)=1+e(−x)1
y^(i)=a(i)=sigmoid(z(i))=sigmoid(wTx(i)+b)\hat{y}^{(i)} = a^{(i)} = sigmoid(z^{(i)}) = sigmoid(w^Tx^{(i)} + b)y^(i)=a(i)=sigmoid(z(i))=sigmoid(wTx(i)+b)
损失函数(cost function):
J=m1∑i=1mL(a(i),y(i))
其中
L(a(i),y(i))=−y(i)log(a(i))−(1−y(i))log(1−a(i))
损失函数求偏导(derivative cost function):
∂w∂J=m1X(A−Y)T
∂b∂J=m1∑i=1m(a(i)−y(i))
2.训练模型
数据准备
扫描二维码关注公众号,回复:
718794 查看本文章
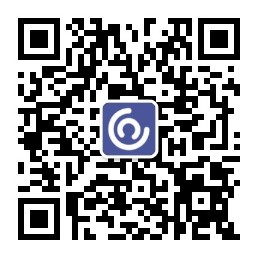
首先我们需要先下载MNIST的数据集。使用以下的命令进行下载:
wget http://tensorflow-1253902462.cosgz.myqcloud.com/mnist_cnn/t10k-images-idx3-ubyte.gz wget http://tensorflow-1253902462.cosgz.myqcloud.com/mnist_cnn/t10k-labels-idx1-ubyte.gz wget http://tensorflow-1253902462.cosgz.myqcloud.com/mnist_cnn/train-images-idx3-ubyte.gz wget http://tensorflow-1253902462.cosgz.myqcloud.com/mnist_cnn/train-labels-idx1-ubyte.gz
创建代码
现在您可以在 /home/ubuntu 目录下创建源文件 logistic_regression.py,内容可参考:
#-*- coding:utf-8 -*- import time import numpy as np import tensorflow as tf from tensorflow.examples.tutorials.mnist import input_data MNIST = input_data.read_data_sets("./", one_hot=True) learning_rate = 0.01 batch_size = 128 n_epochs = 25 X = tf.placeholder(tf.float32, [batch_size, 784]) Y = tf.placeholder(tf.float32, [batch_size, 10]) w = tf.Variable(tf.random_normal(shape=[784,10], stddev=0.01), name="weights") b = tf.Variable(tf.zeros([1, 10]), name="bias") logits = tf.matmul(X, w) + b entropy = tf.nn.softmax_cross_entropy_with_logits(labels=Y, logits=logits) loss = tf.reduce_mean(entropy) optimizer = tf.train.GradientDescentOptimizer(learning_rate=learning_rate).minimize(loss) init = tf.global_variables_initializer() with tf.Session() as sess: sess.run(init) n_batches = int(MNIST.train.num_examples/batch_size) for i in range(n_epochs): for j in range(n_batches): X_batch, Y_batch = MNIST.train.next_batch(batch_size) _, loss_ = sess.run([optimizer, loss], feed_dict={ X: X_batch, Y: Y_batch}) print "Loss of epochs[{0}] batch[{1}]: {2}".format(i, j, loss_)
执行代码
python logistic_regression.py
运行过程中,如果出现网络错误,请重试。
运行输出:
Loss of epochs[0] batch[0]: 2.28968191147 Loss of epochs[0] batch[1]: 2.30224704742 Loss of epochs[0] batch[2]: 2.26435565948 Loss of epochs[0] batch[3]: 2.26956915855 Loss of epochs[0] batch[4]: 2.25983452797 Loss of epochs[0] batch[5]: 2.2572259903 ...... Loss of epochs[24] batch[420]: 0.393310219049 Loss of epochs[24] batch[421]: 0.309725940228 Loss of epochs[24] batch[422]: 0.378903746605 Loss of epochs[24] batch[423]: 0.472946226597 Loss of epochs[24] batch[424]: 0.259472459555 Loss of epochs[24] batch[425]: 0.290799200535 Loss of epochs[24] batch[426]: 0.256865829229 Loss of epochs[24] batch[427]: 0.250789999962 Loss of epochs[24] batch[428]: 0.328135550022
3.测试模型
示例代码编辑源文件 logistic_regression.py,内容可参考:
#-*- coding:utf-8 -*- import time import numpy as np import tensorflow as tf from tensorflow.examples.tutorials.mnist import input_data MNIST = input_data.read_data_sets("./", one_hot=True) learning_rate = 0.01 batch_size = 128 n_epochs = 25 X = tf.placeholder(tf.float32, [batch_size, 784]) Y = tf.placeholder(tf.float32, [batch_size, 10]) w = tf.Variable(tf.random_normal(shape=[784,10], stddev=0.01), name="weights") b = tf.Variable(tf.zeros([1, 10]), name="bias") logits = tf.matmul(X, w) + b entropy = tf.nn.softmax_cross_entropy_with_logits(labels=Y, logits=logits) loss = tf.reduce_mean(entropy) optimizer = tf.train.GradientDescentOptimizer(learning_rate=learning_rate).minimize(loss) init = tf.global_variables_initializer() with tf.Session() as sess: sess.run(init) n_batches = int(MNIST.train.num_examples/batch_size) for i in range(n_epochs): for j in range(n_batches): X_batch, Y_batch = MNIST.train.next_batch(batch_size) _, loss_ = sess.run([optimizer, loss], feed_dict={ X: X_batch, Y: Y_batch}) print "Loss of epochs[{0}] batch[{1}]: {2}".format(i, j, loss_) n_batches = int(MNIST.test.num_examples/batch_size) total_correct_preds = 0 for i in range(n_batches): X_batch, Y_batch = MNIST.test.next_batch(batch_size) preds = tf.nn.softmax(tf.matmul(X_batch, w) + b) correct_preds = tf.equal(tf.argmax(preds, 1), tf.argmax(Y_batch, 1)) accuracy = tf.reduce_sum(tf.cast(correct_preds, tf.float32)) total_correct_preds += sess.run(accuracy) print "Accuracy {0}".format(total_correct_preds/MNIST.test.num_examples)
执行代码
python logistic_regression.py
运行输出:
Accuracy 0.9108
4.完成实验
实验内容已完成