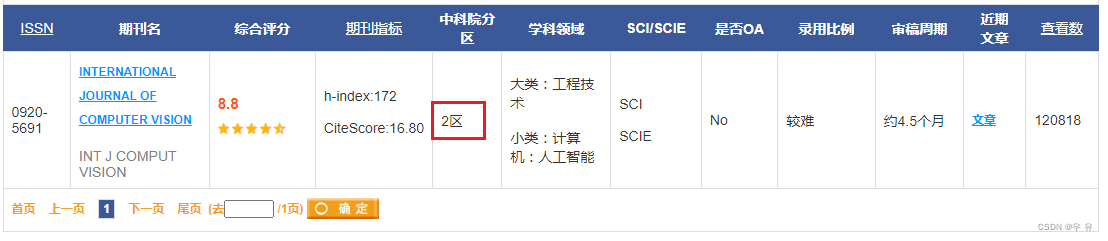
Abstract
Related Work
For deep learning based facial landmark detection, there are two widely used detection heads, namely heatmap regression and coordinate regression. Heatmap regression can achieve good results, but it has two drawbacks: (1) it is computationally expensive; (2) it is sensitive to outliers (see Figure 5(b)). In contrast, coordinate regression is fast and robust, but not accurate enough (see Figure 5(a)). Although coordinate regression can be used in a multi-stage manner to yield better performance, its inference speed becomes slow as a result.
基于DL脸部点检测方法×2:热图回归、坐标回归
Heatmap:√结果好 ×计算量大,对异常值敏感
coordinate:√快速且鲁棒 ×准确度不够(虽然多阶段来提高性能但会降低速度)
⇒目的是结合二者优点(the first study in this area that discusses the connection between heatmap and coordinate regression.)
Coordinate Regression Models
Heatmap Regression Models
Cross-Domain Generalization
Semi-Supervised Facial Landmark Detection
Method
PIP regression、
neighbor regression module、
self-training with curriculum framework、
implicit prior we observe from CNN-based facial landmark detectors
知识点+词汇
Generalization capability across domains 跨域的泛化能力
domain gaps
Stacked hourglass networks堆叠沙漏网络
hybrids of classification and regression分类和回归的混合体
leverage利用