3,Experimental Evaluation
3.1 实验设置
Datasets.
To evaluate the performance of our proposed
PFLD, we conduct experiments on two widely-adopted
challenging datasets, say 300W [
25
] and AFLW [
18
].
300W.
This dataset annotates fifive face datasets including
LFPW, AFW, HELEN, XM2VTS and IBUG, with 68 land
marks. We follow [
9
,
34
,
19
] to utilize 3,148 images for
training and 689 images for testing. The testing images are
divided into two subsets, say the common subset formed by
554 images from LFPW and HELEN, and the challenging
subset by 135 images from IBUG. The common and the
challenging subsets form the full testing set.
AFLW
. This dataset consists of 24,386 in-the-wild human
faces, which are obtained from Flicker with extreme poses,
expressions and occlusions. The faces are with head pose
ranging from
0
◦
to
120
◦
for yaw, and upto
90
◦
for pitch
and roll, respectively. AFLW offers at most 21 landmarks
for each face. We use 20,000 images and 4,386 images for
training and testing, respectively
数据集:
为了评价我们提出的PFLD的表现效果,我们在两个被广泛接受的具有挑战性的数据集即 300W【25】和 AFLW【18】。这个数据集标注出了五个人脸数据集,包括 LFPW ,AFW, HELEN, XM2VTS 和 IBUG 有68个标记。我们的实验像【9,34,19】一样使用3148张图用来训练,689张图用来测试。测试用图被分成两组,有554张来自LFPW和HELEN的554张图,剩下135张图具有挑战性的,来自IBUG。
AFLW 这个数据集有24386张自然条件下的人脸图片,是从闪拍器中获得,包括很多极端的姿势,表情和遮挡情况。这些人连的头部倾斜角度从0°到120° for yaw ,到90° for pitch and roll ,AFLW提供最多每张脸21个关键点位置。使用2w和4386张图分别用来训练和测试。
竞争者:
The compared approaches include classic
and recently proposed deep learning based schemes,
which are RCPR (ICCV’13) [
4
], SDM (CVPR’13)
[
38
], CFAN (ECCV’14) [
42
], CCNF (ECCV’14) [
1
], ESR
(IJCV’14) [
5
], ERT (CVPR’14) [
16
], LBF (CVPR’14) [
24
],
TCDCN (ECCV’14) [
45
], CFSS (CVPR’15) [
46
], 3DDFA
(CVPR’16) [
48
], MDM (CVPR’16) [
29
], RAR (ECCV’16)
[
37
], CPM (CVPR’16) [
33
], DVLN (CVPRW’17) [
35
],
TSR (CVPR’17) [
22
], Binary-CNN (ICCV’17) [
3
],
PIFA-CNN (ICCV’17) [
15
], RDR (CVPR’17) [
36
],
DCFE (ECCV’18) [
30
], SeqMT (CVPR’18) [
12
], PCD
CNN (CVPR’18) [
19
], SAN (CVPR’18) [
9
] and LAB
(CVPR’18) [
34
].
评价优点:
和之前的工作类似【5,34,9,19】,使用归一化的平均误差来作为测量精度的标准,从所有的关键点标记中计算平均值。对于300W,我们用两个标准化的因素来报告结果,一个采用 eye-center-distance 作为瞳间距离的标准化因素。对于ALFW来说,因为侧脸变化多端,我们像【19,9,34】一样归一化 由真实边界框大小得到的误差。 积累误差分布 (CED)曲线也被用来比较这些方法。除了精度之外,处理速度和模型的体量也是被比较的重要因素。
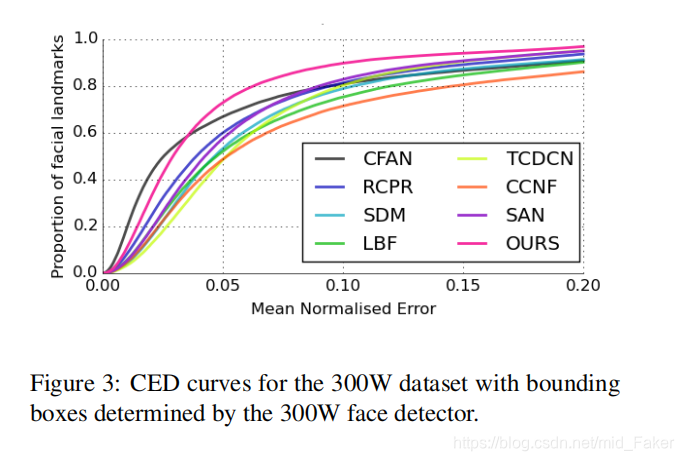
3.2 实验结果
检测精度。
模型体量。
处理速度比较。
消融实验。
(
Ablation study 消融实验,在计算机视觉领域中,往往提及消融实验来验证本文的创新点。
比如你弄了个目标检测的pipeline用了A, B, C,然后效果还不错,但你并不知道A, B, C各自到底起了多大的作用,可能B效率很低同时精度很好,也可能A和B彼此相互促进。
Ablation study/experiment就是用来告诉你或者读者整个流程里面的关键部分到底起了多大作用,就像Ross将RPN换成SS进行对比实验,以及与不共享主干网络进行对比,就是为了给读者更直观的数据来说明算法的有效性。
在原理上有些像控制变量法
原文链接:https://blog.csdn.net/qq_38742161/article/details/86477776
)
额外结果。
4.结论
面部地标探测器的三个方面需要考虑到能够胜任大规模和/或实时任务,即准确性、效率和紧凑性。本文提出了一种实用的人脸地标探测器,称为pfld,它由主干网和辅助网两个子网组成。主干网由mobilenet块构成,可以在很大程度上释放卷积层的计算压力,并通过调整宽度参数使模型的尺寸根据用户的要求灵活。引入了一个多尺度的全连接层,扩大了接收场,从而增强了捕捉人脸结构的能力。为了进一步规范地标定位,我们定制了另一个分支,即辅助网络,通过它可以有效地估计旋转信息。考虑到几何正则化和数据不平衡问题,设计了一种新的损耗。广泛的实验结果表明,我们的设计在精度、模型尺寸和加工速度方面优于最先进的方法,因此验证了我们的PFLD 0.25x是一个很好的折衷实际使用。
在当前版本中,pfld仅采用旋转信息(偏航、侧倾和俯仰角)作为几何约束。预计将采用其他几何/结构信息来帮助进一步提高精度。例如,像实验室[34]一样,我们可以调整地标,使其不偏离边界线太远。从另一个角度来看,提高性能的一个可能尝试是用一些特定的任务来代替基本损失,即“2损失”。在损失中设计更复杂的加权策略也会带来好处,尤其是当培训数据不平衡和有限时。我们将上述思想作为我们今后的工作。
reference
References
[1] T. Baltrusaitis, P. Robinson, and L.-P. Morency. Con-
ˇ
tinuous conditional neural fifields for structured regres
sion. In
ECCV
, 2014.
6
[2] P. Belhumeur, D. Jacobs, D. Kriegman, and N. Kumar.
Localizing parts of faces using a consensus of exem
plars. In
CVPR
, 2011.
2
[3] A. Bulat and G. Tzimiropoulos. Binarized convolu
tional landmark localizers for human pose estimation
and face alignment with limited resources. In
ICCV
,
2017.
4
,
6
,
8
[4] X. Burgos-Artizzu, P. Perona, and P. Dollar. Robust
face landmark estimation under occlusion. In
ICCV
,
2013.
6
,
7
,
8
[5] X. Cao, Y. Wei, F. Wen, and J. Sun. Face alignment
by explicit shape regression.
IJCV
, 107(2):177–190,
2014.
2
,
6
,
7
[6] T. Cootes, G. Edwards, and C. Taylor. Active appear
ance models.
IEEE TPAMI
, 23(6):681–685, 2001.
2
[7] D. Cristinacce and T. Cootes. Automatic feature local
isation with constrained local models.
Pattern Recog
nition
, 41(10):3054–3067, 2008.
2
[8] D. DeTone, T. Malisiewicz, and A. Rabinovich.
Deep image homography estimation.
CoRR,
abs/1606.03798
, 2016.
5
[9] X. Dong, Y. Yan, W. Ouyang, and Y. Yang. Style
aggregated network for facial landmark detection. In
CVPR
, 2018.
2
,
6
,
7
,
8
[10] Z. Feng, J. Kittler, M. Awais, P. Huber, and X. Wu.
Wing loss for robust facial landmark localisation with
convolutional neural networks. In
CVPR
, 2018.
2
[11] T. Hassner, S. Harel, E. Paz, and R. Enbar. Effective
face frontalization in unconstrained images. In
CVPR
,
2015.
1
[12] S. Honari, P. Molchanov, S. Tyree, P. Vincent, C. Pal,
and J. Kautz. Improving landmark localization with
semi-supervised learning. In
CVPR
, 2018.
2
,
6
,
7
[13] A. Howard, M. Zhu, B. Chen, D. Kalenichenko,
W. Wang, T. Weyand, M. Andreetto, and H. Adam.
Mobilenets: Effificient convolutional neural net
works for mobile vision applications.
CoRR,
abs/1704.04861
, 2017.
3
,
4
[14] A. Jourabloo and X. Liu. Pose-invariant 3d face align
ment. In
ICCV
, 2015.
2
,
3
,
4
,
5
[15] A. Jourabloo, X. Liu, M. Ye, and L. Ren. Pose
invariant face alignment with a single cnn. In
ICCV
,
2017.
2
,
4
,
6
,
7
[16] V. Kazemi and J. Sullivan. One millisecond face align
ment with an ensemble of regression trees. In
CVPR
,
2014.
2
,
6
,
8
[17] F. Khraman, M. Gokmen, S. Darkner, and R. Larsen.
¨
An active illumination and appearance (AIA) model
for face alignment. In
CVPR
, 2007.
2
[18] M. Kostinger, P. Wohlhard, P. Roth, and H. Bischof.
¨
Annotated facial landmarks in the wild: A large-scale,
real-world database for facial landmark localization.
In
ICCVW
, 2011.
3
,
6
[19] A. Kumar and R. Chellappa. Disentangling 3d pose in
a dendritic cnn for unconstrained 2d face alignment.
In
CVPR
, 2018.
2
,
4
,
5
,
6
,
7
,
8
[20] L. Liang, R. Xiao, F. Wen, and J. Sun. Face alignment
via component-based discriminative search. In
ECCV
,
2008.
2
[21] Y. Liu, F. Wei, J. Shao, L. Sheng, J. Yan, and X. Wang.
Exploring disentangled feature representation beyond
face identifification. In
CVPR
, 2018.
1
[22] J. Lv, X. Shao, J. Xing, C. Cheng, and X. Zhou.
A deep regression architecture with two-stage re
initialization for high performance facial landmark de
tection. In
CVPR
, 2017.
2
,
6
,
7
,
8
[23] I. Matthews and S. Baker. Active appearance models
revisited.
IJCV
, 60(2):135–164, 2004.
2
[24] S. Ren, X. Cao, Y. Wei, and J. Sun. Face alignment
at 3000 fps via regressing local binary features. In
CVPR
, 2014.
6
,
7
,
8
[25] C. Sagonas, G. Tzimiropoulos, S. Zafeiriou, and
M. Pantic. 300 faces in-the-wild challenge: The fifirst
facial landmark localization challenge. In
ICCVW
,
2013.
3
,
6
[26] M. Sandle, A. Howard, M. Zhu, A. Zhmoginov, and
L.-C. Chen. Mobilenetv2: Inverted residuals and lin
ear bottlenecks.
CoRR, abs/1801.04381
, 2018.
3
,
4
[27] Y. Sun, X. Wang, and X. Tang. Hybrid deep learn
ing for face verifification.
IEEE TPAMI
, 38(10):1997–
2009, 2016.
1
10
[28] J. Thies, M. Zollhofer, M. Stamminger, C. Theobalt,
¨
and M. Nießner. Face2face: Real-time face capture
and reenactment of rgb videos. In
CVPR
, 2016.
1
[29] G. Trigeogis, P. Snape, M. Nicolaou, E. Antonakos,
and S. Zafeiriou. Mnemonic descent method: A re
current process applied for end-to-end face alignment.
In
CVPR
, 2016.
2
,
6
,
7
[30] R. Valle, J. Buenaposada, A. Valdes, and L. Baumela.
´
A deeply-initialized coarse-to-fifine ensemble of regres
sion trees for face alignment. In
ECCV
, 2018.
2
,
6
,
7
[31] M. Valstar, B. Martinez, X. Binefa, and M. Pantic.
Facial point detection using boosted regression and
graph models. In
CVPR
, 2010.
2
[32] N. Wang, X. Gao, D. Tao, H. Yang, and X. Li. Fa
cial feature point detection: A comprehensive survey.
Neurocomputing
, 275:50–65, 2018.
2
[33] S. Wei, V. Ramakrishna, T. Kanade, and Y. Sheikh.
Convolutional pose machines. In
CVPR
, 2016.
2
,
6
,
7
,
8
[34] W. Wu, C. Qian, S. Yang, Q. Wang, Y. Cai, and
Q. Zhou. Look at boundary: A boundary-aware face
alignment algorithm. In
CVPR
, 2018.
5
,
6
,
7
,
10
[35] W. Wu and S. Yang. Leveraging intra and inter-dataset
variations for robust face alignment. In
CVPRW
, 2017.
6
,
7
[36] S. Xiao, J. Feng, L. Liu, X. Nie, W. Wang, S. Yang,
and A. Kassim. Recurrent 3d-2d dual learning for
large-pose facial landmark detection. In
CVPR
, 2017.
2
,
6
,
7
[37] S. Xiao, J. Feng, J. Xing, H. Lai, S. Yan, and A. Kas
sim. Robust facial landmark detection via recurrent
attentive-refifinement networks. In
ECCV
, 2016.
2
,
6
,
7
[38] X. Xiong and F. De la Torre. Supervised decent
method and its applications to face alignment. In
CVPR
, 2013.
2
,
6
,
7
,
8
[39] H. Yang, W. Mou, Y. Zhang, I. Patras, H. Gunes, and
P. Robinson. Face alignment assisted by head pose
estimation. In
BMVC
, 2015.
2
,
4
[40] S. Yang, P. . Luo, C. Loy, and X. Tang. Wider face: A
face detection benchmark. In
CVPR
, 2016.
6
[41] X. Yu, J. Huang, S. Zhang, W. Yan, and D. Metaxas.
Pose-free facial landmark fifitting via optimized part
mixtures and cascaded deformable shape model. In
ICCV
, 2013.
8
[42] J. Zhang, S. Shan, M. Kan, and X. Chen. Coarse-to-
fifine auto-encoder networks (cfan) for real-time face
alignment. In
ECCV
, 2014.
2
,
6
,
7
[43] K. Zhang, Z. Zhang, Z. Li, and Y. Qiao. Joint face de
tection and alignment using multitask cascaded con
volutional networks.
IEEE Signal Processing Letters
,
23(10):1499–1503, 2016.
3
,
8
[44] X. Zhang, X. Zhou, M. Lin, and J. Sun. Shufflflenet:
An extremely effificient convolutional neural network
for mobile devices. In
CVPR
, 2018.
4
[45] Z. Zhang, P. Luo, C. Loy, and X. Tang. Facial land
mark detection via deep multi-task learning. In
ECCV
,
2014.
2
,
6
,
7
[46] S. Zhu, C. Li, C. Loy, and X. Tang. Face alignment by
coarse-to-fifine shape searching. In
CVPR
, 2015.
6
,
7
,
8
[47] S. Zhu, C. Li, C. Loy, and X. Tang. Unconstrained
face alignment via cascaded compositional learning.
In
CVPR
, 2016.
2
,
8
[48] X. Zhu, Z. Lei, X. Liu, H. Shi, and S. Z. Li. Face
alignment across large poses: A 3d solution. In
CVPR
,
2016.
2
,
5
,
6
,
7
[49] X. Zhu, Z. Lei, J. Yan, D. Yi, and S. Z. Li. High-
fifidelity pose and expression normalization for face
recognition in the wild. In
CVPR
, 2015.
1
[50] X. Zhu and D. Ramanan. Face detection, pose estima
tion, and landmark localization in the wild. In
CVPR
,
2012.
2