一、准备待识别的图片(格式 .jpg、.png)
1、通过画图工具可手写数字,在改变成28*28像素大小。
2、可以通过解析MNIST数字集,得到数字图片。
3、可以通过手机拍照获得数字照片,一般需要做二值化处理。
二、利用Tensorflow进行模型训练
详细代码如下:
# -*- coding: utf-8 -*-
"""
Created on Tue Jun 5 08:34:09 2018
"""
Created on Tue Jun 5 08:34:09 2018
@author: jaminliu
"""
"""
from tensorflow.examples.tutorials.mnist import input_data
mnist = input_data.read_data_sets('MNIST_data', one_hot=True)
mnist = input_data.read_data_sets('MNIST_data', one_hot=True)
import tensorflow as tf
sess = tf.InteractiveSession()
#权重使用的truncated_normal进行初始化,stddev标准差定义为0.1 偏置初始化为常量0.1
def weight_variable(shape):
initial = tf.truncated_normal(shape, stddev=0.1)
return tf.Variable(initial)
initial = tf.truncated_normal(shape, stddev=0.1)
return tf.Variable(initial)
def bias_variable(shape):
initial = tf.constant(0.1, shape=shape)
return tf.Variable(initial)
initial = tf.constant(0.1, shape=shape)
return tf.Variable(initial)
'''
卷积函数:
strides[0]和strides[3]的两个1是默认值,中间两个1代表padding时在x方向运动1步,y方向运动1步
padding='SAME'代表经过卷积之后的输出图像和原图像大小一样
'''
def conv2d(x, W): #x是图片的所有参数,W是此卷积 层的权重
return tf.nn.conv2d(x, W, strides=[1, 1, 1, 1], padding='SAME')
'''
池化函数
ksize指定池化核函数的大小
根据池化核函数的大小定义strides的大小
'''
def max_pool_2x2(x):
return tf.nn.max_pool(x, ksize=[1, 2, 2, 1],
strides=[1, 2, 2, 1], padding='SAME') #池化的核函数大小为2x2,因此ksize=[1,2,2,1],步长为2,因此strides=[1,2,2,1]
x = tf.placeholder("float", shape=[None, 784]) #输入值
y_ = tf.placeholder("float", shape=[None, 10]) #输出值
x_image = tf.reshape(x, [-1,28,28,1]) #-1代表先不考虑输入的图片例子多少这个维度,后面的1是channel的数量,
#因为我们输入的图片是黑白的,因此channel是1,例如如果是RGB图像,那么channel就是3
y_ = tf.placeholder("float", shape=[None, 10]) #输出值
x_image = tf.reshape(x, [-1,28,28,1]) #-1代表先不考虑输入的图片例子多少这个维度,后面的1是channel的数量,
#因为我们输入的图片是黑白的,因此channel是1,例如如果是RGB图像,那么channel就是3
#第一层卷积层
W_conv1 = weight_variable([5, 5, 1, 32]) #卷积核定义为5x5,1是输入的通道数目,32是输出的通道数目
b_conv1 = bias_variable([32]) # 每个输出通道对应一个偏置
W_conv1 = weight_variable([5, 5, 1, 32]) #卷积核定义为5x5,1是输入的通道数目,32是输出的通道数目
b_conv1 = bias_variable([32]) # 每个输出通道对应一个偏置
h_conv1 = tf.nn.relu(conv2d(x_image, W_conv1) + b_conv1) # 卷积运算,并使用ReLu激活函数激活
h_pool1 = max_pool_2x2(h_conv1) # pooling操作
h_pool1 = max_pool_2x2(h_conv1) # pooling操作
#第二层卷积层
W_conv2 = weight_variable([5, 5, 32, 64])
b_conv2 = bias_variable([64])
W_conv2 = weight_variable([5, 5, 32, 64])
b_conv2 = bias_variable([64])
h_conv2 = tf.nn.relu(conv2d(h_pool1, W_conv2) + b_conv2)
h_pool2 = max_pool_2x2(h_conv2)
h_pool2 = max_pool_2x2(h_conv2)
#第一层全连接层
W_fc1 = weight_variable([7 * 7 * 64, 1024]) # 将最后操作的数据展开
b_fc1 = bias_variable([1024]) # 下面就是定义一般神经网络的操作了,继续扩大为1024
W_fc1 = weight_variable([7 * 7 * 64, 1024]) # 将最后操作的数据展开
b_fc1 = bias_variable([1024]) # 下面就是定义一般神经网络的操作了,继续扩大为1024
h_pool2_flat = tf.reshape(h_pool2, [-1, 7*7*64]) # 对应的偏置
h_fc1 = tf.nn.relu(tf.matmul(h_pool2_flat, W_fc1) + b_fc1) # 运算、激活(这里不是卷积运算了,就是对应相乘)
h_fc1 = tf.nn.relu(tf.matmul(h_pool2_flat, W_fc1) + b_fc1) # 运算、激活(这里不是卷积运算了,就是对应相乘)
#输出层
keep_prob = tf.placeholder("float")
h_fc1_drop = tf.nn.dropout(h_fc1, keep_prob) # dropout防止过拟合
keep_prob = tf.placeholder("float")
h_fc1_drop = tf.nn.dropout(h_fc1, keep_prob) # dropout防止过拟合
W_fc2 = weight_variable([1024, 10]) # 最后一层权重初始化
b_fc2 = bias_variable([10]) # 对应偏置
b_fc2 = bias_variable([10]) # 对应偏置
y_conv=tf.nn.softmax(tf.matmul(h_fc1_drop, W_fc2) + b_fc2) # 使用softmax分类器
#训练
cross_entropy = -tf.reduce_sum(y_*tf.log(y_conv)) # 交叉熵损失函数来定义cost function
train_step=tf.train.AdamOptimizer(1e-4).minimize(cross_entropy) #调用梯度下降
correct_prediction = tf.equal(tf.argmax(y_conv,1), tf.argmax(y_,1))
accuracy = tf.reduce_mean(tf.cast(correct_prediction, "float"))
sess.run(tf.initialize_all_variables())
saver = tf.train.Saver()
for i in range(3000):
batch = mnist.train.next_batch(50) # 使用SGD,每次选50个数据训练
if i%100 == 0:
train_accuracy = accuracy.eval(feed_dict={
x:batch[0], y_: batch[1], keep_prob: 1.0}) # dropout值定义为0.5
print("step %d, training accuracy %g"%(i, train_accuracy))
train_step.run(feed_dict={x: batch[0], y_: batch[1], keep_prob: 0.5}) # 每50次输出一下准确度
batch = mnist.train.next_batch(50) # 使用SGD,每次选50个数据训练
if i%100 == 0:
train_accuracy = accuracy.eval(feed_dict={
x:batch[0], y_: batch[1], keep_prob: 1.0}) # dropout值定义为0.5
print("step %d, training accuracy %g"%(i, train_accuracy))
train_step.run(feed_dict={x: batch[0], y_: batch[1], keep_prob: 0.5}) # 每50次输出一下准确度
#红色部分要保存为自己的路径,和测试加载路径相对应
save_path = saver.save(sess,'my_net/model.ckpt')
print("test accuracy %g"%accuracy.eval(feed_dict={
x: mnist.test.images, y_: mnist.test.labels, keep_prob: 1.0}))
x: mnist.test.images, y_: mnist.test.labels, keep_prob: 1.0}))
三、使用训练好的模型进行识别
代码如下:
扫描二维码关注公众号,回复:
1533884 查看本文章
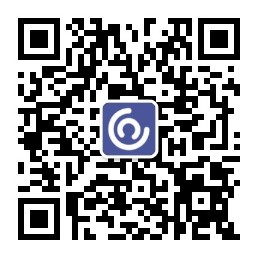
# -*- coding: utf-8 -*-
"""
Created on Wed Jun 6 15:56:16 2018
"""
Created on Wed Jun 6 15:56:16 2018
@author: jaminliu
"""
from PIL import Image, ImageFilter
import tensorflow as tf
import matplotlib.pyplot as plt
#import cv2
"""
from PIL import Image, ImageFilter
import tensorflow as tf
import matplotlib.pyplot as plt
#import cv2
def imageprepare(i):
"""
This function returns the pixel values.
The imput is a png file location.
"""
file_name='test_image/' + str(i+1) + ".png" #导入自己的图片地址#导入自己的图片地址
#in terminal 'mogrify -format png *.jpg' convert jpg to png
im = Image.open(file_name).convert('L')
"""
This function returns the pixel values.
The imput is a png file location.
"""
file_name='test_image/' + str(i+1) + ".png" #导入自己的图片地址#导入自己的图片地址
#in terminal 'mogrify -format png *.jpg' convert jpg to png
im = Image.open(file_name).convert('L')
# im.save("C:/software-projects/tensorflow_projects/shou_xie_shibie3/test_image/sample.png")
plt.imshow(im)
plt.show()
#tf.reset_default_graph()
tv = list(im.getdata()) #get pixel values
#normalize pixels to 0 and 1. 0 is pure white, 1 is pure black.
tva = [ (255-x)*1.0/255.0 for x in tv]
#print(tva)
return tva
tva = [ (255-x)*1.0/255.0 for x in tv]
#print(tva)
return tva
"""
This function returns the predicted integer.
The imput is the pixel values from the imageprepare() function.
"""
# Define the model (same as when creating the model file)
result=imageprepare(1)
This function returns the predicted integer.
The imput is the pixel values from the imageprepare() function.
"""
# Define the model (same as when creating the model file)
result=imageprepare(1)
#此行代码很重要,为其他图片的测试设置重置,清除此前的初值
tf.reset_default_graph()
x = tf.placeholder(tf.float32, [None, 784])
W = tf.Variable(tf.zeros([784, 10]))
b = tf.Variable(tf.zeros([10]))
x = tf.placeholder(tf.float32, [None, 784])
W = tf.Variable(tf.zeros([784, 10]))
b = tf.Variable(tf.zeros([10]))
def weight_variable(shape):
initial = tf.truncated_normal(shape, stddev=0.1)
return tf.Variable(initial)
initial = tf.truncated_normal(shape, stddev=0.1)
return tf.Variable(initial)
def bias_variable(shape):
initial = tf.constant(0.1, shape=shape)
return tf.Variable(initial)
initial = tf.constant(0.1, shape=shape)
return tf.Variable(initial)
def conv2d(x, W):
return tf.nn.conv2d(x, W, strides=[1, 1, 1, 1], padding='SAME')
return tf.nn.conv2d(x, W, strides=[1, 1, 1, 1], padding='SAME')
def max_pool_2x2(x):
return tf.nn.max_pool(x, ksize=[1, 2, 2, 1], strides=[1, 2, 2, 1], padding='SAME')
return tf.nn.max_pool(x, ksize=[1, 2, 2, 1], strides=[1, 2, 2, 1], padding='SAME')
W_conv1 = weight_variable([5, 5, 1, 32])
b_conv1 = bias_variable([32])
b_conv1 = bias_variable([32])
x_image = tf.reshape(x, [-1,28,28,1])
h_conv1 = tf.nn.relu(conv2d(x_image, W_conv1) + b_conv1)
h_pool1 = max_pool_2x2(h_conv1)
h_conv1 = tf.nn.relu(conv2d(x_image, W_conv1) + b_conv1)
h_pool1 = max_pool_2x2(h_conv1)
W_conv2 = weight_variable([5, 5, 32, 64])
b_conv2 = bias_variable([64])
b_conv2 = bias_variable([64])
h_conv2 = tf.nn.relu(conv2d(h_pool1, W_conv2) + b_conv2)
h_pool2 = max_pool_2x2(h_conv2)
h_pool2 = max_pool_2x2(h_conv2)
W_fc1 = weight_variable([7 * 7 * 64, 1024])
b_fc1 = bias_variable([1024])
b_fc1 = bias_variable([1024])
h_pool2_flat = tf.reshape(h_pool2, [-1, 7*7*64])
h_fc1 = tf.nn.relu(tf.matmul(h_pool2_flat, W_fc1) + b_fc1)
h_fc1 = tf.nn.relu(tf.matmul(h_pool2_flat, W_fc1) + b_fc1)
keep_prob = tf.placeholder(tf.float32)
h_fc1_drop = tf.nn.dropout(h_fc1, keep_prob)
h_fc1_drop = tf.nn.dropout(h_fc1, keep_prob)
W_fc2 = weight_variable([1024, 10])
b_fc2 = bias_variable([10])
b_fc2 = bias_variable([10])
y_conv=tf.nn.softmax(tf.matmul(h_fc1_drop, W_fc2) + b_fc2)
init_op = tf.initialize_all_variables()
saver = tf.train.Saver()
with tf.Session() as sess:
sess.run(init_op)
sess.run(init_op)
saver.restore(sess,'C:/software-projects/tensorflow_projects/shou_xie_shibie3/models/model.ckpt')
prediction=tf.argmax(y_conv,1)
for i in range(9):
result = imageprepare(i)
predint=prediction.eval(feed_dict={x: [result],keep_prob: 1.0}, session=sess)
for i in range(9):
result = imageprepare(i)
predint=prediction.eval(feed_dict={x: [result],keep_prob: 1.0}, session=sess)
print('recognize result:')
print(predint[0])
print(predint[0])
四、测试结果如下:
等
训练模型中,我是训练了3000次,其中图片9的识别不准确,大家可以尝试训练更多次,增加准确性。