深度学习里的Attention model其实模拟的是人脑的注意力模型,举个例子来说,当我们观赏一幅画时,虽然我们可以看到整幅画的全貌,但是在我们深入仔细地观察时,其实眼睛聚焦的就只有很小的一块,这个时候人的大脑主要关注在这一小块图案上,也就是说这个时候人脑对整幅图的关注并不是均衡的,是有一定的权重区分的。这就是深度学习里的Attention Model的核心思想。
AM刚开始也确实是应用在图像领域里的,AM在图像处理领域取得了非常好的效果!于是,就有人开始研究怎么将AM模型引入到NLP领域。最有名的当属“Neural machine translation by jointly learning to align and translate”这篇论文了,这篇论文最早提出了Soft Attention Model,并将其应用到了机器翻译领域。后续NLP领域使用AM模型的文章一般都会引用这篇文章(目前引用量已经上千了!!!)
如下图所示,机器翻译主要使用的是Encoder-Decoder模型,在Encoder-Decoder模型的基础上引入了AM,取得了不错的效果:
Soft Attention Model:
这里其实是上面图的拆解,我们前面说过,“Neural machine translation by jointly learning to align and translate”这篇论文提出了soft Attention Model,并将其应用到了机器翻译上面。其实,所谓Soft,意思是在求注意力分配概率分布的时候,对于输入句子X中任意一个单词都给出个概率,是个概率分布。
即上图中的ci是对Encoder中每一个单词都要计算一个注意力概率分布,然后加权得到的。如下图所示:
其实有Soft AM,对应也有一个Hard AM。既然Soft是给每个单词都赋予一个单词对齐概率,那么如果不这样做,直接从输入句子里面找到某个特定的单词,然后把目标句子单词和这个单词对齐,而其它输入句子中的单词硬性地认为对齐概率为0,这就是Hard Attention Model的思想。Hard AM在图像里证明有用,但是在文本里面用处不大,因为这种单词一一对齐明显要求太高,如果对不齐对后续处理负面影响很大。
但是,斯坦福大学的一篇paper“Effective Approaches to Attention-based Neural Machine Translation”提出了一个混合Soft AM 和Hard AM的模型,论文中,他们提出了两种模型:Global Attention Model和Local Attention Model,Global Attention Model其实就是Soft Attention Model,Local Attention Model本质上是Soft AM和 Hard AM的一个混合。一般首先预估一个对齐位置Pt,然后在Pt左右大小为D的窗口范围来取类似于Soft AM的概率分布。
Global Attention Model和Local Attention Model
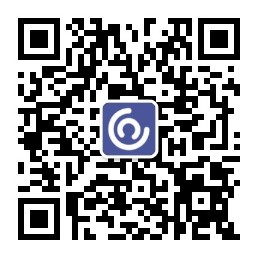
Global AM其实就是soft AM,Decoder的过程中,每一个时间步的Context vector需要计算Encoder中每一个单词的注意力权重,然后加权得到。
Local AM则是首先找到一个对其位置,然后在对其位置左右一个窗口内来计算注意力权重,最终加权得到Context vector。这其实是Soft AM和Hard AM的一个混合折中。
静态AM
其实还有一种AM叫做静态AM。所谓静态AM,其实是指对于一个文档或者句子,计算每个词的注意力概率分布,然后加权得到一个向量来代表这个文档或者句子的向量表示。跟soft AM的区别是,soft AM在Decoder的过程中每一次都需要重新对所有词计算一遍注意力概率分布,然后加权得到context vector,但是静态AM只计算一次得到句子的向量表示即可。(这其实是针对于不同的任务而做出的改变)
强制前向AM
Soft AM在逐步生成目标句子单词的时候,是由前向后逐步生成的,但是每个单词在求输入句子单词对齐模型时,并没有什么特殊要求。强制前向AM则增加了约束条件:要求在生成目标句子单词时,如果某个输入句子单词已经和输出单词对齐了,那么后面基本不太考虑再用它了,因为输入和输出都是逐步往前走的,所以看上去类似于强制对齐规则在往前走。
看了这么多AM模型以及变种,那么我们来看一看AM模型具体怎么实现,涉及的公式都是怎样的。
我们知道,注意力机制是在序列到序列模型中用于注意编码器状态的最常用方法,它同时还可用于回顾序列模型的过去状态。使用注意力机制,系统能基于隐藏状态 s_1,...,s_m 而获得环境向量(context vector)c_i,这些环境向量可以和当前的隐藏状态 h_i 一起实现预测。环境向量 c_i 可以由前面状态的加权平均数得出,其中状态所加的权就是注意力权重 a_i:
注意力函数 f_att(h_i,s_j) 计算的是目前的隐藏状态 h_i 和前面的隐藏状态 s_j 之间的非归一化分配值。
而实际上,注意力函数也有很多种变体。接下来我们将讨论四种注意力变体:加性注意力(additive attention)、乘法(点积)注意力(multiplicative attention)、自注意力(self-attention)和关键值注意力(key-value attention)。
加性注意力(additive attention)
加性注意力是最经典的注意力机制 (Bahdanau et al., 2015) [15],它使用了有一个隐藏层的前馈网络(全连接)来计算注意力的分配:
也就是:
乘法(点积)注意力(multiplicative attention)
乘法注意力(Multiplicative attention)(Luong et al., 2015) [16] 通过计算以下函数而简化了注意力操作:
加性注意力和乘法注意力在复杂度上是相似的,但是乘法注意力在实践中往往要更快速、具有更高效的存储,因为它可以使用矩阵操作更高效地实现。两个变体在低维度 d_h 解码器状态中性能相似,但加性注意力机制在更高的维度上性能更优。
自注意力(self-attention)
注意力机制不仅能用来处理编码器或前面的隐藏层,它同样还能用来获得其他特征的分布,例如阅读理解任务中作为文本的词嵌入 (Kadlec et al., 2017) [37]。然而,注意力机制并不直接适用于分类任务,因为这些任务并不需要情感分析(sentiment analysis)等额外的信息。在这些模型中,通常我们使用 LSTM 的最终隐藏状态或像最大池化和平均池化那样的聚合函数来表征句子。
自注意力机制(Self-attention)通常也不会使用其他额外的信息,但是它能使用自注意力关注本身进而从句子中抽取相关信息 (Lin et al., 2017) [18]。自注意力又称作内部注意力,它在很多任务上都有十分出色的表现,比如阅读理解 (Cheng et al., 2016) [38]、文本继承 (textual entailment/Parikh et al., 2016) [39]、自动文本摘要 (Paulus et al., 2017) [40]。
关键值注意力(key-value attention)
关键值注意力 (Daniluk et al., 2017) [19] 是最近出现的注意力变体机制,它将形式和函数分开,从而为注意力计算保持分离的向量。它同样在多种文本建模任务 (Liu & Lapata, 2017) [41] 中发挥了很大的作用。具体来说,关键值注意力将每一个隐藏向量 h_i 分离为一个键值 k_i 和一个向量 v_i:[k_i;v_i]=h_i。键值使用加性注意力来计算注意力分布 a_i:
其中 L 为注意力窗体的长度,I 为所有单元为 1 的向量。然后使用注意力分布值可以求得环境表征 c_i:
其中环境向量 c_i 将联合现阶段的状态值 v_i 进行预测。
最后的最后,再加一个自己用keras实现的简单的静态AM(自注意力)层的代码吧:
from keras import backend as K
from keras.engine.topology import Layer
from keras import initializers, regularizers, constraints
class Attention_layer(Layer):
"""
Attention operation, with a context/query vector, for temporal data.
Supports Masking.
Follows the work of Yang et al. [https://www.cs.cmu.edu/~diyiy/docs/naacl16.pdf]
"Hierarchical Attention Networks for Document Classification"
by using a context vector to assist the attention
# Input shape
3D tensor with shape: `(samples, steps, features)`.
# Output shape
2D tensor with shape: `(samples, features)`.
:param kwargs:
Just put it on top of an RNN Layer (GRU/LSTM/SimpleRNN) with return_sequences=True.
The dimensions are inferred based on the output shape of the RNN.
Example:
model.add(LSTM(64, return_sequences=True))
model.add(AttentionWithContext())
"""
def __init__(self,
W_regularizer=None, b_regularizer=None,
W_constraint=None, b_constraint=None,
bias=True, **kwargs):
self.supports_masking = True
self.init = initializers.get('glorot_uniform')
self.W_regularizer = regularizers.get(W_regularizer)
self.b_regularizer = regularizers.get(b_regularizer)
self.W_constraint = constraints.get(W_constraint)
self.b_constraint = constraints.get(b_constraint)
self.bias = bias
super(Attention_layer, self).__init__(**kwargs)
def build(self, input_shape):
assert len(input_shape) == 3
self.W = self.add_weight((input_shape[-1], input_shape[-1],),
initializer=self.init,
name='{}_W'.format(self.name),
regularizer=self.W_regularizer,
constraint=self.W_constraint)
if self.bias:
self.b = self.add_weight((input_shape[-1],),
initializer='zero',
name='{}_b'.format(self.name),
regularizer=self.b_regularizer,
constraint=self.b_constraint)
super(Attention_layer, self).build(input_shape)
def compute_mask(self, input, input_mask=None):
# do not pass the mask to the next layers
return None
def call(self, x, mask=None):
uit = K.dot(x, self.W)
if self.bias:
uit += self.b
uit = K.tanh(uit)
a = K.exp(uit)
# apply mask after the exp. will be re-normalized next
if mask is not None:
# Cast the mask to floatX to avoid float64 upcasting in theano
a *= K.cast(mask, K.floatx())
# in some cases especially in the early stages of training the sum may be almost zero
# and this results in NaN's. A workaround is to add a very small positive number to the sum.
# a /= K.cast(K.sum(a, axis=1, keepdims=True), K.floatx())
a /= K.cast(K.sum(a, axis=1, keepdims=True) + K.epsilon(), K.floatx())
print a
# a = K.expand_dims(a)
print x
weighted_input = x * a
print weighted_input
return K.sum(weighted_input, axis=1)
def compute_output_shape(self, input_shape):
return (input_shape[0], input_shape[-1])