文章目录
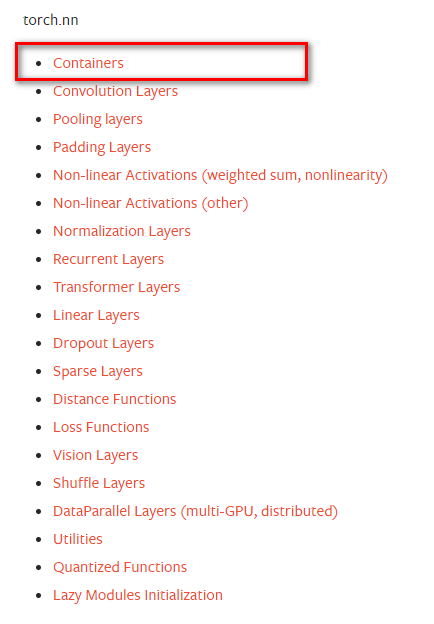
一、模型创建步骤
二、构建模型两要素
三、torch.nn模块
nn.Module
• parameters : 存储管理nn.Parameter类
• modules : 存储管理nn.Module类
• buffers:存储管理缓冲属性,如BN层中的running_mean
• ***_hooks:存储管理钩子函数
self._parameters = OrderedDict()
self._buffers = OrderedDict()
self._backward_hooks = OrderedDict()
self._forward_hooks = OrderedDict()
self._forward_pre_hooks = OrderedDict()
self._state_dict_hooks = OrderedDict()
self._load_state_dict_pre_hooks = OrderedDict()
self._modules = OrderedDict()
例:
class Model(nn.Module):
def __init__(self):
super().__init__()
self.conv1 = nn.Conv2d(1, 20, 5)
self.conv2 = nn.Conv2d(20, 20, 5)
def forward(self, x):
x = F.relu(self.conv1(x))
return F.relu(self.conv2(x))
四、模型容器——Containers
4.1 nn.Sequential
nn.Sequential 是 nn.module的容器,用于按顺序包装一组网络层
• 顺序性:各网络层之间严格按照顺序构建
• 自带forward():自带的forward里,通过for循环依次执行前向传播运算
# Using Sequential to create a small model. When `model` is run,
# input will first be passed to `Conv2d(1,20,5)`. The output of
# `Conv2d(1,20,5)` will be used as the input to the first
# `ReLU`; the output of the first `ReLU` will become the input
# for `Conv2d(20,64,5)`. Finally, the output of
# `Conv2d(20,64,5)` will be used as input to the second `ReLU`
model = nn.Sequential(
nn.Conv2d(1,20,5),
nn.ReLU(),
nn.Conv2d(20,64,5),
nn.ReLU()
)
# Using Sequential with OrderedDict. This is functionally the
# same as the above code
model = nn.Sequential(OrderedDict([
('conv1', nn.Conv2d(1,20,5)),
('relu1', nn.ReLU()),
('conv2', nn.Conv2d(20,64,5)),
('relu2', nn.ReLU())
]))
4.2 nn.ModuleList
nn.ModuleList是 nn.module的容器,用于包装一组网络层,以迭代方式调用网络层主要方法:
• append():在ModuleList后面添加网络层
• extend():拼接两个ModuleList
• insert():指定在ModuleList中位置插入网络层
class MyModule(nn.Module):
def __init__(self):
super(MyModule, self).__init__()
self.linears = nn.ModuleList([nn.Linear(10, 10) for i in range(10)])
def forward(self, x):
# ModuleList can act as an iterable, or be indexed using ints
for i, l in enumerate(self.linears):
x = self.linears[i // 2](x) + l(x)
return x
4.3 nn.ModuleDict
nn.ModuleDict是 nn.module的容器,用于包装一组网络层,以索引方式调用网络层主要方法:
• clear():清空ModuleDict
• items():返回可迭代的键值对(key-value pairs)
• keys():返回字典的键(key)
• values():返回字典的值(value)
• pop():返回一对键值,并从字典中删除
class MyModule(nn.Module):
def __init__(self):
super(MyModule, self).__init__()
self.choices = nn.ModuleDict({
'conv': nn.Conv2d(10, 10, 3),
'pool': nn.MaxPool2d(3)
})
self.activations = nn.ModuleDict([
['lrelu', nn.LeakyReLU()],
['prelu', nn.PReLU()]
])
def forward(self, x, choice, act):
x = self.choices[choice](x)
x = self.activations[act](x)
return x
4.4 容器总结
- nn.Sequential:顺序性,各网络层之间严格按顺序执行,常用于block构建
- nn.ModuleList:迭代性,常用于大量重复网构建,通过for循环实现重复构建
- nn.ModuleDict:索引性,常用于可选择的网络层