文章目录
Introduction
BERT: Bidirectional Encoder Representations from Transformers.
通过预训练的语言模型可有效地提升多数下游任务,处理下游任务(迁移学习)的方法大致分为两种:
-
feature-based (feature-extraction),such as ELMo,uses task-specific architectures that include the pre-trained representations as additional features;
-
fine-tuning,such as the Generative Pre-trained Transformer (OpenAI GPT),is trained on the downstream tasks by simply fine-tuning all pretrained parameters;
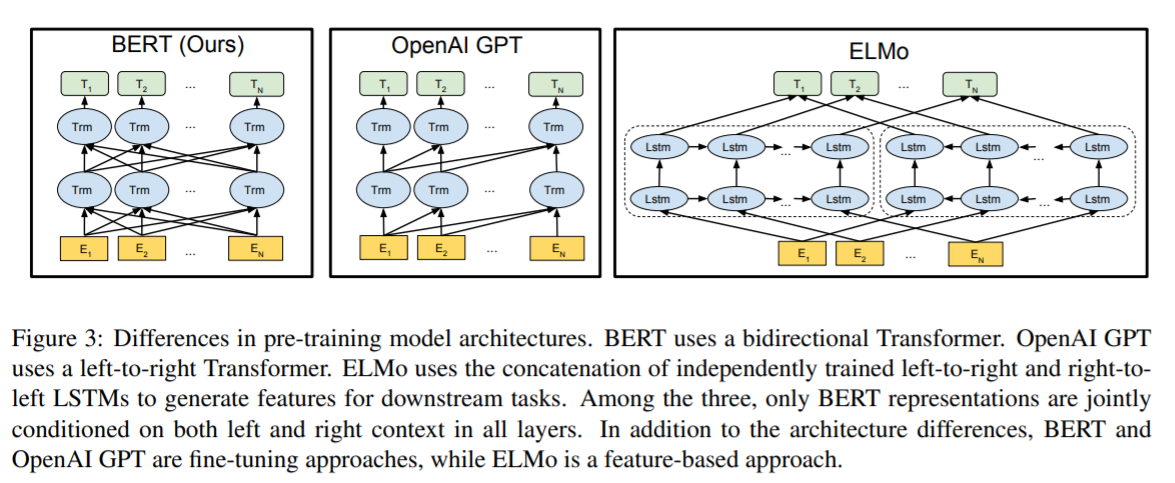
单向的标准语言模型,很大程度限制了预训练模型表示能力的学习。
OpenAI GPT使用从左到右的架构,transformer的self-attention层的每一个token仅依赖于之前的tokens(训练任务约束),这种架构预训练的语言模型很难处理句子层级的下游任务,如Qestion-Answer,因为Answer往往和Qestion的上下文有关。
为什么语言生成模型是单向的?
语言生成模型能够基于部分单词序列,生成下一个单词的概率分布,这种性质决定语言生成模型是单向模型。语言模型适合做生成任务,如单向语言模型GPT(生成模型)的文本生成能力很强。
BERT使用双向编码器中,单词可以看到其自身,通过上下文单词信息“填空”,BERT不是语言生成模型,可看作为语言理解模型,但这种模型不适合做生成任务,适合做利用上下文信息的标注和分类任务!
BERT预训练语言模型的特点:
- 基于掩盖的方式预训练双向语言模型,可以使用更深的网络架构;
- 基于fine-tuning处理下游任务,同时在sentence-level和token-level的多数任务中均达到出色的表现;
Pre-trained Model
Unsupervised Feature-based Approaches
嵌入表达是NLP领域的重要组成部分,如词嵌入、句嵌入和篇章嵌入等。对于句嵌入,常用的训练方法包括:
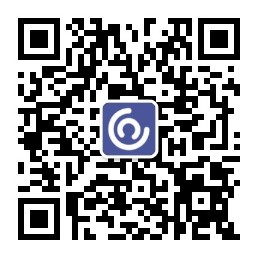
- 给定前一句表示,从左到右依次生成下一句;
- auto-encoder方法;
ELMo通过双向网络提取具有上下文信息的词嵌入(拼接前后向词嵌入),在多数NLP任务取得非常好的表现,如QA、情感分析、NER等。
Unsupervised Fine-tuning Approaches
最近利用无标签数据预训练的句子和篇章编码器,可生成具有上下文信息的词嵌入用于下游监督任务,这种方法的好处是下游任务从头开始学的参数较少。
OpenAI GPT在句子级别的任务中达到很好的效果,这种模型一般利用单向RNN网络或auto-encoder预训练。
Transfer Learning from Supervised Data
利用大规模标注数据预训练,在小规模标注集的类似任务微调,也能得到较好的效果,比如自然语言推理、机器翻译等。翻译模型可在不同语言间迁移,模型能够学习到语言的语法等特征表示。
CV领域也有类似处理,如在ImageNet数据集预训练,具体下游任务fine-tuning。
BERT
BERT架构两部曲:pre-training and fine-tuning。
- pre-training:用不同任务在无标签数据集上预训练;
- fine-tuning:用相同的预训练参数初始化不同的下游任务,再对所有参数微调;
BERT使用统一的预训练模型架构,能够处理不同的下游任务,不同下游任务的差异较小。
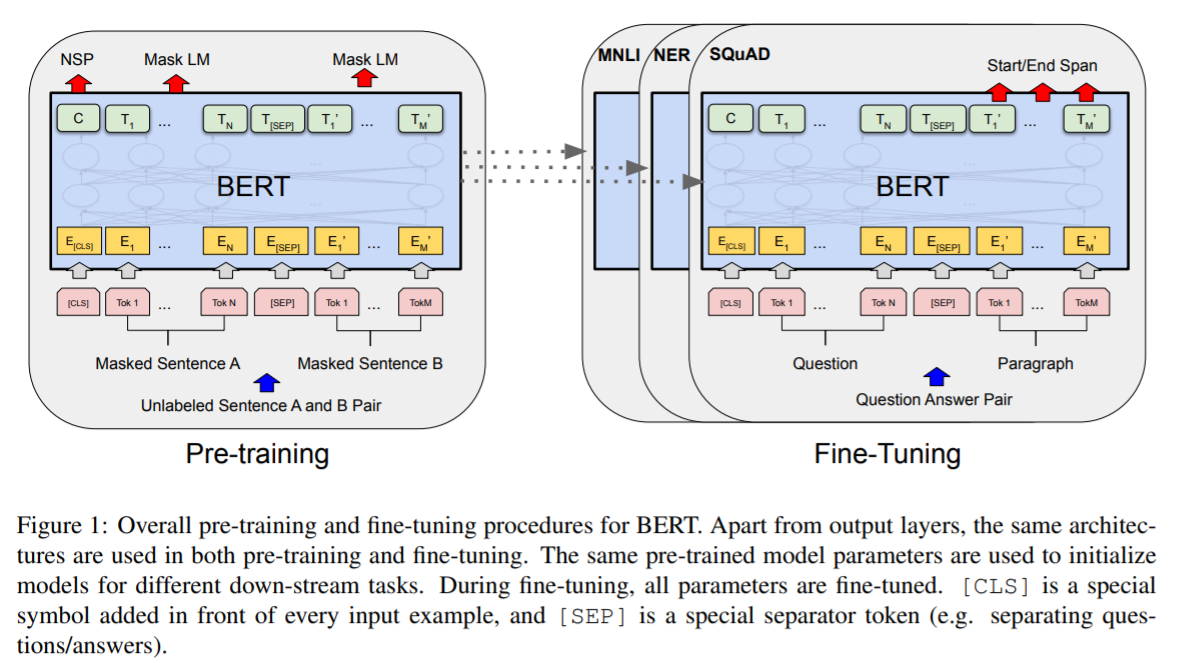
BERT考虑不同嵌入信息,并对不同嵌入求和得到输入序列嵌入:
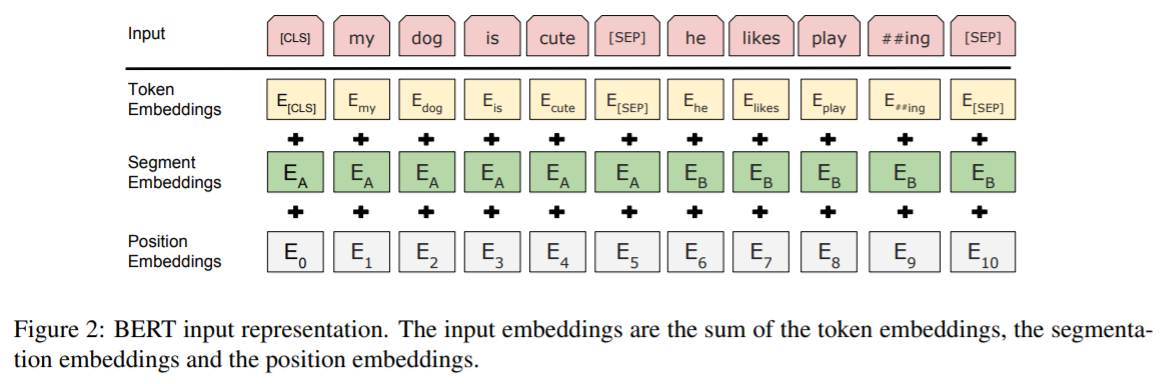
Model Architecture
使用multi-layer bidirectional Transformer encoder,参考Attenion is All You Need这篇paper,BERT模型参数:
- : the number of layers.
- : the hidden size.
- : the number of self-attention heads.
- BERTbase(L=12, h=768, A=12, Total Parameters=110M), the same model size as OpenAI GPT.
- BERTlarge(L=24, h=1024, A=16, Total Parameters=340M).
Input/Output Representations
BERT模型将不同的输入统一为单一输入序列,以处理多样化输入的下游任务,如单句输入的NER任务、多句输入的QA任务等。输入序列的处理方式:
- 使用[CLS]作为每个输入序列的第一个token,其最终一个隐藏状态的输出可用于下游分类任务
- 使用[SEP]连接不同句子的输入序列
- 每个token加上位置嵌入(mask),区别token属于哪个句子
如图1所示,输入序列是一对句子A和B,图中各符号的意义:
- 句子A和B添加位置嵌入,使用[SEP]连接为输入嵌入序列
- 是特殊标记[CLS]的最后一个隐状态
- 是输入序列第 位置的最后一个隐状态
如图2所示,输入序列嵌入是对不同嵌入方式的求和。
Pre-training BERT
预训练BERT模型使用两种无监督任务:Masked LM和Next Sentence Prediction (NSP)。
Task #1: Masked LM
标准条件语言模型只能按照left-to-right或right-to-left的方式预训练模型,而Bidirectional RNN网络能够捕获上下文信息,每个单词能够间接的“see itself”,使得模型可在多层上下文中预测目标词。
为训练深度表征语言模型,BERT随机mask一定百分比的tokens,然后基于上下文预测这些被mask的tokens,这种过程称为“masked LM” (MLM),类似于完形填空。
训练过程中,将mask tokens在最后一层的隐状态接入softmax层,与标准LM处理方式一样,通过最小化mask tokens的预测分布与实际分布的交叉熵,优化语言模型。BERT的所有试验中,随机mask 15%的tokens。
Masked LM如何用于下游任务的Fine-tuing?
Mask tokens方法可有效预训练双向深层语言模型,然而实际fine-tuning过程中不存在[MASK] token,造成预训练模型和fine-tuning不匹配。
BERT为匹配pre-trained model和fine-tuning,对于已确定需要mask的tokens,BERT不总将这些“被选中”的tokens替换为[MASK],而是将其中80%会被替换为[MASK]、10%随机替换为其它token (1.5%)、10%不做改变,使得预训练阶段中也具有没有mask的输入,匹配预训练和微调。
![]() |
![]() |
Task #2: Next Sentence Predition (NSP)
为处理依赖不同句子关联关系的下游任务,如Question Answering (QA)和Natural Language Inference (NLI),BERT预训练sentence A是否是sentence B的下一句这种简单的二分类任务。
具体地说:选中sentence A和sentence B作为训练数据,50%的B是A的下一句(IsNEXT),50%的B从语料库中随机选择(NotNext),图1中 用于next sentence predict (NSP)。尽管这种模型非常简单,但可十分有效地应用于具有句子级别关联关系的下游任务。
NSP任务与一些研究人员提出的表征学习(Representation Learning)很相似,一些表征学习模型(feature-based)仅将句嵌入用于下游任务,BERT是将所有预训练的参数用于下游任务(fine-tuning)。
理解特殊Token的意义(BERT的两个任务)
BERT在输入序列头部添加[CLS] token,不同句子间添加[SEP] token,掩盖词替换为[MASK] token。
在预训练**“下句预测”**任务时,使用[CLS] token在最后一层的隐状态用于预测类别,通过训练使得从上(输出侧)至下(输入侧)的网络结构可用于分类任务,分类任务中输出侧仅使用[CLS] token对应的隐状态。对应的[SEP] token,BERT也能学习到其代表连接不同句子。
在预训练**“完形填空”**任务时,使用[MASK] token在最后一层的隐状态用于预测真实词,通过训练使得不同输出位置具有语义表征能力。
看到没有,这两个任务好像可以同时训练,即在NSP任务中随机mask一些词,牛哄哄!!!
Fine-tuning BERT
BERT基于Transformer的self-attention机制(不同位置词的距离都是1,语义表征能力强)预训练模型,可基于fine-tuning所有参数处理多数下游任务。
Sentence A and B from pre-training are analogous (类似) to:
- sentence pairs in paraphrasing.
- hypothesis-premise pairs in entailment.
- question-passage pairs in question answering.
- a degenerate text-∅ pair in text classification or sequence tagging.
At the output, the token representations are fed into an output layer for tokenlevel tasks, such as sequence tagging or question answering, and the [CLS] representation is fed into an output layer for classification, such as entailment or sentiment analysis.
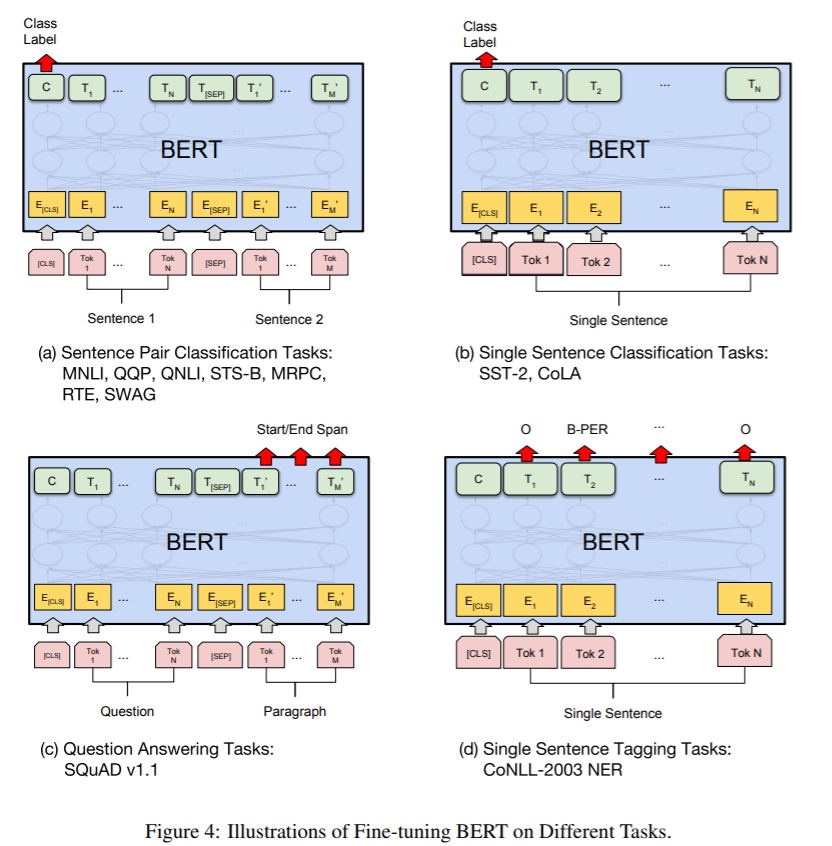
[CLS]输入对应输出层的隐状态用于分类任务,其它输入对应输出层的隐状态序列用于序列标注或问答等任务。
Experiments
BERT在11项NLP任务的微调结果。
GLUE
General Language Understanding Evaluation (GLUE) benchmark is collection of diverse natural language understanding tasks.
Fine-tuning:
- Final hidden vector corresponding to the first inout token [CLS] as the aggregate representation.
- Classification layer weights are only new parameters during fine-tuning, is the number of labels.
- Loss function is:
- Hyper parameters: batch_size=32, epochs=2, learning rate among 5e-5 ~ 2e-5
- Samll datasets was sometimes unstable for BERTlarge, choose best model on Dec set by running several random restarts???
SQuAD v1.1
Stanford Question Answering Dataset. Given a question and a passage from Wikipedia containing the answer, the task is to predict the answer text span in the passage.
Fine-tuning:
-
Represent the input sequence (embedding A) and passage (embedding B) as a single packed sequence.
-
Indroduce a start vector amd a end vector
-
Probability of -th word being the start of the answer span is (end word like this):
-
Candidate span from position to position is:
-
Training objective is the sum of log-likehood of the correct start and end positions .
微调思想:真实范围值尽可能大,非真实范围值尽可能小。
SQuAD v2.0
SQuAD v2.0 extends the SQuAD 1.1 problem definition by allowing for the possibility that no short answer exists in the provided paragraph, making the problem more realistic.
Fine-tuning:
- Based on SQuAD v1.1 BERT model.
- Treat questions that don’t have an answer span with start and end at the [CLS] token, compare the score of the no-answer span to the best non-null span:
- Predict a non-null answer where , where is selected on the dev set to maximize F1.
不理解怎么训练!!!20200507
SWAG
Situations With Adversarial Generations. Given a sentence, the task is to choose the most plausible continuation among four choices.
Fine-tuning:
- Construct four input sequences, each containing the concatenation of the given sentence A and a possible continuation sentence B.
- Only task-specific parameters intruduced is a vector whose dot product with the [CLS] token representation denotes a score for each chioce which is normalized with a softmax layer.
- Hyper patameters: batch_size=16, leraning_rate=2e-5.
大佬关于BERT SWAG实操解释
Let’s assume your batch size is 8 and your sequence length is 128. Each SWAG example has 4 entries, the correct one and 3 incorrect ones.
- Instead of your
input_fn
returning aninput_ids
of size[128]
, it should return one of size[4, 128]
. Same for mask and sequence ids. So for each example, you will generate the sequencespredicate ending0
,predicate ending1
,predicate ending2
,predicate ending3
. Also return a label scalar which is in an integer in the range[0, 3]
to indicate what the gold ending is.- After batching, your
model_fn
will get an input of shape[8, 4, 128]
. Reshape these to[32, 128]
before passing them intoBertModel
. I.e., BERT will consider all of these independently.- Compute the logits as in
run_classifier.py
, but your “classifier layer” will just be a vector of size[768]
(or whatever your hidden size is).- Now you have a set of logits of size
[32]
. Re-shape these back into[8, 4]
and then computetf.nn.log_softmax()
over the 4 endings for each example. Now you have log probabilities of shape[8, 4]
over the 4 endings and a label tensor of shape[8]
, so compute the loss exactly as you would for a classification problem.
-若不考虑原句选择4个选项的概率分布,即认为每句各自的4个选项间相互独立,则可以把SWAG任务看成是4N个独立的二分类任务,N为训练集大小。
若考虑原句选择4个选项的概率分布,则可将原句和4个选项生成的4个输入序列在一个batch里面作为BERT的输入(BERT认为每个batch的样本无关),在输出层可同时获得这4个输入序列对应的[CLS]的隐状态,选择4个选项的概率分布表示为:
Fine-tuning模型仅包含一个与输出层隐状态同维的向量 。
为什么输出层不用4*hidden_size维矩阵,而仅使用hidden_size维向量?
个人理解:K分类任务输出层参数为K*hidden_size的矩阵,即输出层含K个神经元(参数维度hidden_size*K, 其实只有K-1个自由神经元),而SWAG本质是二分类任务,从任务输出(是否是正确选项)也能看出,因此输出层用一个神经元即可。
Ablation Studies
消融实验,理解模型参数。
![]() |
![]() |
![]() |
Effect of Pre-training Tasks
使用相同的预训练数据、模型参数、微调参数等,评估深层双向网络的重要性。
Standard Left-to-Right LM (LTR). The addition of NSP task significantly improved the LTR model.
各模型区别如图5所示。
Effect of Model Size
We trained a number of BERT models with a differing number of layers, hidden units, and attention heads, while otherwise using the same hyperparameters and training procedure as described previously.
各模型区别如图6所示。
Feature-based Approach with BERT
某些下游任务可能无法表示成Transformer encoder架构,所以有必要考虑能够处理特定任务的预训练模型架构。此外,预先训练模型学习特征表示,之后直接用这些特征表示处理下游任务,计算代价更小。如拼接BERT的后四层作为token特征表示,NER任务的F1值仅比使用BERT fine-tuning低0.3。
各模型区别如图7所示。
Reference:
1. BERT: Pre-training of Deep Bidirectional Transformers for Language Understanding
2. plan to release SWAG code?