论文:Rethinking the Faster R-CNN Architecture for Temporal Action Localization
CVPR 2018
link: http://cn.arxiv.org/pdf/1804.07667.pdf
摘要:
主要贡献有下面三个
1. we improve receptive field alignment using a multi-scale architecture that can accommodate extreme variation in action durations;
2. we better exploit the temporal context of actions for both proposal generation and action classification by appropriately extending receptive fields
3. we explicitly consider multi-stream feature fusion and demonstrate that fusing motion late is important
介绍:
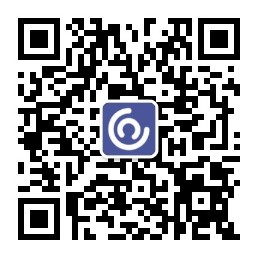
除了识别出动作,还要识别出动作的开始结束时间
where the task is to not only identify the action class, but also detect the start and end time of each action instance
相关工作:
1.Action Recognition 动作识别
Tremendous progress has recently been made due to the introduction of large datasets and the developments on deep neural networks [37, 30, 43, 49, 7, 14]深度学习的发展。
2.Temporal Action Localization 时间动作定位
Yuan et al. [54] proposed a multi-scale pooling scheme to capture features at multiple resolutions.
many recent approaches adopt a two-stage, proposal-plus-classification framework [6, 36,12, 3, 4, 35, 56]
3 Faster R-CNN
数据集:THUMOS’14
未完待续