Apache Spark提供了一个名为MLlib的机器学习API。 PySpark也在Python中使用这个机器学习API。它支持不同类型的算法,如下所述 -
mllib.classification - spark.mllib包支持二分类,多分类和回归分析的各种方法。包含分类中一些最流行的算法如随机森林,朴素贝叶斯,决策树等。
mllib.clustering - 聚类是一种无监督的学习问题,以根据某些相似概念将实体的子集彼此分组。
mllib.fpm - 频繁模式匹配是挖掘频繁项,项集,子序列或其他子结构,这些通常是分析大规模数据集的第一步。多年来,这一直是数据挖掘领域的一个活跃的研究课题。
mllib.linalg - 线性代数的MLlib实用程序。
mllib.recommendation - 协同过滤通常用于推荐系统。这些技术旨在填写用户项关联矩阵的缺失条目。
spark.mllib - 它目前支持基于模型的协同过滤,其中用户和产品由一小组可用于预测缺失条目的潜在因素描述。 spark.mllib使用交替最小二乘(ALS)算法来学习这些潜在因素。
mllib.regression - 线性回归属于回归算法族。回归的目标是找到变量之间的关系和依赖关系。使用线性回归模型和模型摘要的界面类似于逻辑回归案例。
还有其他算法,类和函数也作为mllib包的一部分。截至目前,让我们了解一下pyspark.mllib的演示。
以下示例是使用ALS算法进行协同过滤以构建推荐模型并在训练数据上进行评估。
Dataset used − test.data
1,1,5.0
1,2,1.0
1,3,5.0
1,4,1.0
2,1,5.0
2,2,1.0
2,3,5.0
2,4,1.0
3,1,1.0
3,2,5.0
3,3,1.0
3,4,5.0
4,1,1.0
4,2,5.0
4,3,1.0
4,4,5.0
-------------------------------------recommend.py----------------------------------------
from __future__ import print_function
from pyspark import SparkContext
from pyspark.mllib.recommendation import ALS, MatrixFactorizationModel, Rating
if __name__ == "__main__":
sc = SparkContext(appName="Pspark mllib Example")
data = sc.textFile("test.data")
ratings = data.map(lambda l: l.split(','))\
.map(lambda l: Rating(int(l[0]), int(l[1]), float(l[2])))
# Build the recommendation model using Alternating Least Squares
rank = 10
numIterations = 10
model = ALS.train(ratings, rank, numIterations)
# Evaluate the model on training data
testdata = ratings.map(lambda p: (p[0], p[1]))
predictions = model.predictAll(testdata).map(lambda r: ((r[0], r[1]), r[2]))
ratesAndPreds = ratings.map(lambda r: ((r[0], r[1]), r[2])).join(predictions)
MSE = ratesAndPreds.map(lambda r: (r[1][0] - r[1][1])**2).mean()
print("Mean Squared Error = " + str(MSE))
# Save and load model
model.save(sc, "target/tmp/myCollaborativeFilter")
sameModel = MatrixFactorizationModel.load(sc, "target/tmp/myCollaborativeFilter")
--------------------------------------recommend.py----------------------------------------
Command − The command will be as follows −
$SPARK_HOME/bin/spark-submit recommend.py
Output − The output of the above command will be −
Mean Squared Error = 1.20536041839e-05
Spark MLlib中的协同过滤:http://blog.javachen.com/2015/04/17/spark-mllib-collaborative-filtering.html
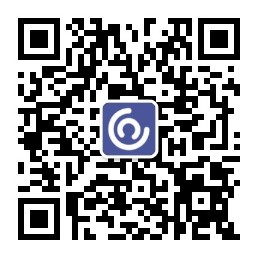