1、简介
报告嘉宾:杨萌林 (香港中文大学)
报告题目
双曲图神经网络在推荐系统与量化上的应用
报告摘要
The first part is about hyperbolic recommender system.
Considering the prevalence of the power-law distribution in user-item networks, hyperbolic space has attracted considerable attention and achieved impressive performance in the recommender system recently. The advantage of hyperbolic recommendation lies in that its exponentially increasing capacity is well-suited to describe the power-law distributed user-item network, whereas the Euclidean equivalent is deficient. Nonetheless, it remains unclear which kinds of items can be effectively recommended by the hyperbolic model and which cannot.
To address the above concerns, we take the most basic recommendation technique, collaborative filtering, as a medium, to investigate the behaviors of hyperbolic and Euclidean recommendation models.
The second part is about quantitative trading in hyperbolic space.
Trading and making investment decisions based on quantitative data are complex financial jobs in a market with a value of more than $60 trillion. Stock forecasting has come a long way, but one drawback of current neural approaches is that they ignore the rich signals between the movements of linked stocks, making them less effective. Based on financial literature, we could model inter-stock linkages as a graph in four major global stock markets and construct stock selection as graph-based learning to rank issues based on scale-free graph-based network features.
报告人简介
Menglin Yang is currently a final-year PhD student in the Department of Computer Science and Engineering, The Chinese University of Hong Kong (CUHK). Before that, he received B.S. and M.S. degrees from Southwest University and University of Chinese Academy of Sciences. His research interests include hyperbolic graph learning, machine learning, and graph-related application. His several works related to hyperbolic graph representation learning were accepted at recent top conferences, including KDD 2021, WSDM 2022, WWW 2022, and KDD2022.
2、Hyperbolic GNN:Method and application
大纲
背景
双曲空间
经典方法
1 Poincare Ball Model
2 Lorentz Model
动机
双曲图神经网络
经典工作
作者工作(分别在消息、聚合和更新这块做工作)
挑战与机遇
应用
推荐
工作1 HRCF
工作2 HGCF
工作3 LKGN
工作4 HICF
量化
工作1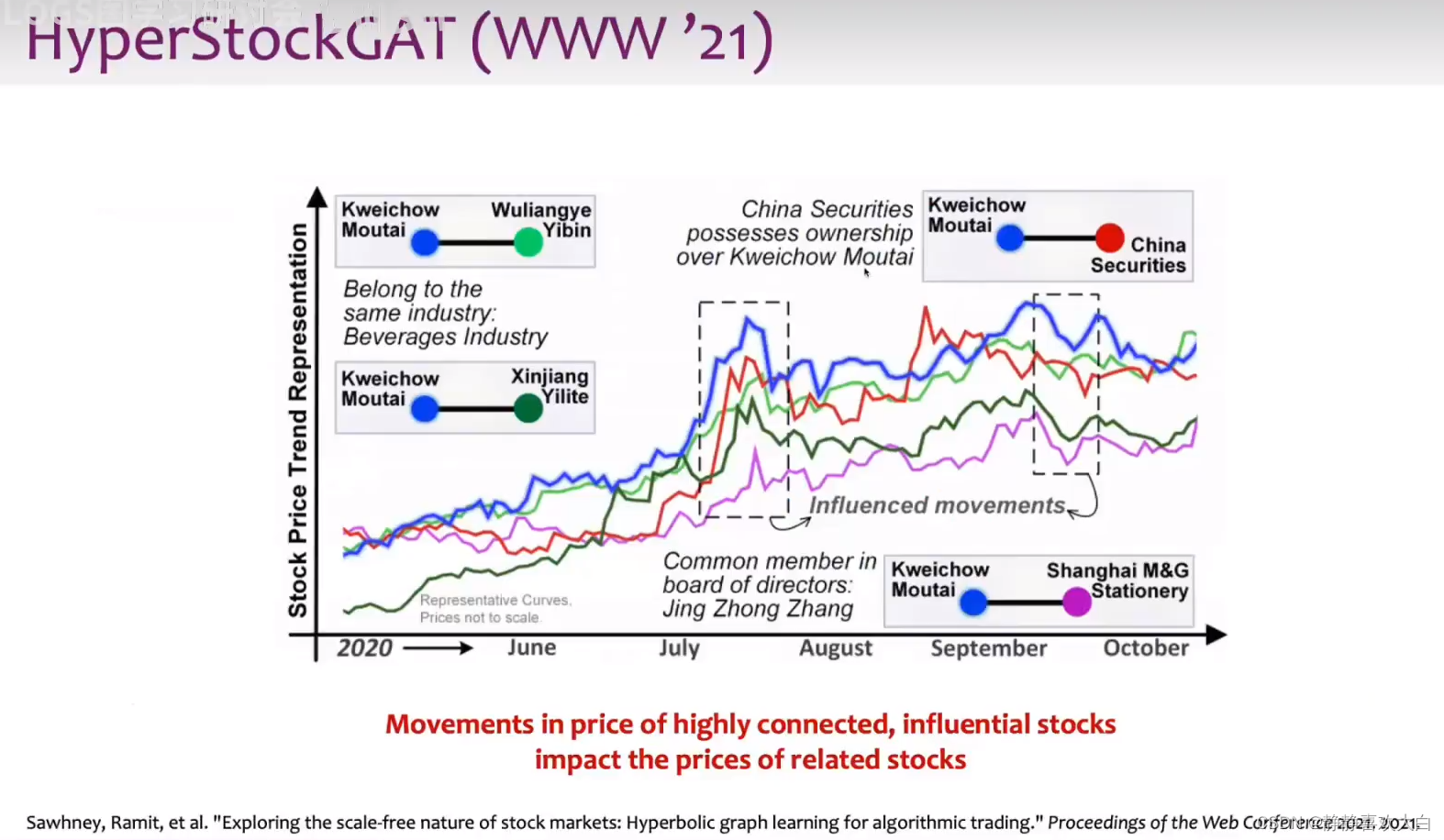
小结
3、参考
LOGS 第2022/08/06期 || 香港中文大学杨萌林: 双曲图神经网络在推荐系统与量化上的应用
LOGS 第2022/08/06期 || 香港中文大学杨萌林: 双曲图神经网络在推荐系统与量化上的应用_哔哩哔哩_bilibili
参考文献
-
HRCF: Enhancing collaborative filtering via hyperbolic geometric regularization
-
Modeling Scale-free Graphs with Hyperbolic Geometry for Knowledge-aware Recommendation
-
Hyperbolic Graph Neural Networks: A Review of Methods and Applications
-
HICF: Hyperbolic Informative Collaborative Filtering
-
Exploring the Scale-Free Nature of Stock Markets: Hyperbolic Graph Learning for Algorithmic Trading
-
Discrete-time Temporal Network Embedding via Implicit Hierarchical Learning in Hyperbolic Space