链接:https://spandan-madan.github.io/A-Collection-of-important-tasks-in-pytorch/#SECTION-2---Model-Saving/Loading
pytorch fine-tuning的一些思考
1.为什么要用transfer learning
interpretation: Suppose, I want to train a dataset to learn to differentiate between a car and a bicycle. Now, I could potentially gather images of both categories and train a network from scratch. But, given the majority of work already out there, it's easy to find a model trained to identify things like Dogs, cats, and humans. Admittedly, neither of these 3 look like cars or bicycles. However, it's still better than nothing. We could start by taking this model, and train it to learn car v/s bicycle. Gains : 1) It will be faster, 2) We need lesser images of cats and bicycles.
假设,我想要训练一个数据集来学习区分汽车和自行车。现在,我可以收集这两种类型的图像,并从头开始训练网络。但是,考虑到已经有了大部分的工作,很容易找到一个训练有素的模型来识别狗、猫和人类之类的东西。无可否认,这三样东西都不像汽车或自行车。然而,这总比没有好。我们可以从这个模型开始,训练它学习汽车/自行车。好处:1)它会更快,2)我们需要较少的猫和自行车的图像。
2.transfer learning的两种方式
- fine-tuning:简单讲,迁移的模型权重作为初始化,适当的调整最后的全连接层适应自己的数据,然后训练
- freeze:冻结模型的权重,在训练过程中不做改变。只训练改变后的最后全连接层(包括最后的softmax层)
-----------------------------------代码中学习pytoch transfer learning--------------------------------
3.使用pytorch model里面的预训练网络前,可以先打印看看这个模型长什么样子,都有些什么层,每层的参数是多少。代码链接里有。
child_counter = 0
for child in model.children():
print(" child", child_counter, "is -")
print(child)
child_counter += 1
结果:部分展示
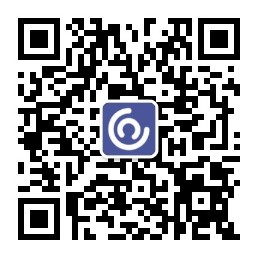
4.再来看一下每一层参数的取值
for child in model.children(): for param in child.parameters(): print("This is what a parameter looks like - \n",param) break break
因为网络巨大,参数几百万,这里只显示第一个卷积层的参数,从上面结果知道,conv1的卷积核是64*3*7*7,这里的结果就是卷积核的取值
结果:
5.freeze
显然,训练这需要大量的计算。所以,通过将这些冷冻起来,训练就会快得多。现在,让我们冻结到child6的第一个模块上
child_counter = 0 for child in model.children(): if child_counter < 6: print("child ",child_counter," was frozen") for param in child.parameters(): param.requires_grad = False elif child_counter == 6: # 因为child里面还有children children_of_child_counter = 0 for children_of_child in child.children(): if children_of_child_counter < 1: for param in children_of_child.parameters(): param.requires_grad = False print('child ', children_of_child_counter, 'of child',child_counter,' was frozen') else: print('child ', children_of_child_counter, 'of child',child_counter,' was not frozen') children_of_child_counter += 1 else: print("child ",child_counter," was not frozen") child_counter += 1
结果:
NOTE
当你冻结住这些层时,如果还使用标准的optimizer就会出错
应将optimizer = torch.optim.RMSprop(model.parameters(), lr=0.1)
改为optimizer = torch.optim.RMSprop(filter(lambda p: p.requires_grad, model.parameters()), lr=0.1)
6.模型的save/load
# Let's assume we will save/load from a path MODEL_PATH # Saving a Model torch.save(model.state_dict(), MODEL_PATH) # Loading the model. # First create a model and define it's architecture as done above in this notebook. If you want a custom architecture. # read below it's been covered below. checkpoint = torch.load(MODEL_PATH) model.load_state_dict(checkpoint)
7.change the final layer
# Load the model
model = models.resnet18(pretrained = False)
# Get number of parameters going in to the last layer. we need this to change the final layer.
num_final_in = model.fc.in_features
# The final layer of the model is model.fc so we can basically just overwrite it
#to have the output = number of classes we need. Say, 300 classes.
NUM_CLASSES = 300
model.fc = nn.Linear(num_final_in, NUM_CLASSES)
8.delete last layer
We can get the layers by using model.children() as before. Then, we can convert this into a list by using a list() command on it. Then, we can remove the last layer by indexing the list. Finally, we can use the PyTorch function nn.Sequential() to stack this modified list together into a new model. You can edit the list in any way you want. That is, you can delete the last 2 layers if you want the features of an image from the 3rd last layer!
我们可以像以前一样使用model.children()来获得图层。然后,我们可以通过在上面使用list()命令将其转换为列表。然后,我们可以通过索引列表来删除最后一层。最后,我们可以使用PyTorch函数nn.Sequential()把这个修改后的列表堆叠到一个新的模型中。您可以以任何您想要的方式编辑列表。也就是说,如果您想要从第三层的图像的特性,您可以删除最后两层!
You may even delete layers from the middle of the model. But obviously, this would lead to incorrect number of features going in to the layer after it as most layers change size of image. In this case, you can index that specific layer of the model and overwrite it just as I showed you immediately above!
您甚至可以从模型中间删除层。但很明显,这将导致不正确的特征数量,因为大多数层改变了图像的大小。在这种情况下,您可以对模型的特定层进行索引并覆盖它,就像我在上面显示的那样!
# Load the model
model = models.resnet18(pretrained = False)
new_model = nn.Sequential(*list(model.children())[:-1])
new_model_2_removed = nn.Sequential(*list(model.children())[:-2])
9.add layer
这部分和以上部分进行糅合,也叫做custom models,自定义模型。
# Some imports first
import torch.nn as nn
import math
import torch.utils.model_zoo as model_zoo
import torch
from torch.autograd.variable import Variable
from torchvision import datasets, models, transforms
# New models are defined as classes. Then, when we want to create a model we create an object instantiating this class.
class Resnet_Added_Layers_Half_Frozen(nn.Module):
def __init__(self,LOAD_VIS_URL=None):
super(ResnetCombinedFull2, self).__init__()
# Start with half the resnet model, swap out the final layer because that's the model we had defined above.
model = models.resnet18(pretrained = False)
num_final_in = model.fc.in_features
model.fc = nn.Linear(num_final_in, 300)
# Now that the architecture is defined same as above, let's load the model we would have trained above.
checkpoint = torch.load(MODEL_PATH)
model.load_state_dict(checkpoint)
# Let's freeze the same as above. Same code as above without the print statements
child_counter = 0
for child in model.children():
if child_counter < 6:
for param in child.parameters():
param.requires_grad = False
elif child_counter == 6:
children_of_child_counter = 0
for children_of_child in child.children():
if children_of_child_counter < 1:
for param in children_of_child.parameters():
param.requires_grad = False
else:
children_of_child_counter += 1
else:
print("child ",child_counter," was not frozen")
child_counter += 1
# Now, let's define new layers that we want to add on top.
# Basically, these are just objects we define here. The "adding on top" is defined by the forward()
# function which decides the flow of the input data into the model.
# NOTE - Even the above model needs to be passed to self.
self.vismodel = nn.Sequential(*list(model.children())
self.projective = nn.Linear(512,400)
self.nonlinearity = nn.ReLU(inplace=True)
self.projective2 = nn.Linear(400,300)
# The forward function defines the flow of the input data and thus decides which layer/chunk goes on top of what.
def forward(self,x):
x = self.vismodel(x)
x = torch.squeeze(x)
x = self.projective(x)
x = self.nonlinearity(x)
x = self.projective2(x)
return x
10CUSTOM LOSS FUNCTIONS
Now that we have our model all in place we can load anything and create any architecture we want. That leaves us with 2 important components in any pipeline - Loading the data, and the training part. Let's take a look at the training part. The two most important components of this step are the optimizer and the loss function. The loss function quantifies how far our existing model is from where we want to be, and the optimizer decides how to update parameters such that we can minimize the loss.
Sometimes, we need to define our own loss functions. And here are a few things to know about this -
- custom Loss functions are defined using a custom class too. They inherit from torch.nn.Module just like the custom model.
- Often, we need to change the dimenions of one of our inputs. This can be done using view() function.
- If we want to add a dimension to a tensor, use the unsqueeze() function.
- The value finally being returned by a loss function MUST BE a scalar value. Not a vector/tensor.
- The value being returned must be a Variable. This is so that it can be used to update the parameters. The best way to do so is to just make sure that both x and y being passed in are Variables. That way any function of the two will also be a Variable.
- A Pytorch Variable is just a Pytorch Tensor, but Pytorch is tracking the operations being done on it so that it can backpropagate to get the gradient.
Here I show a custom loss called Regress_Loss which takes as input 2 kinds of input x and y. Then it reshapes x to be similar to y and finally returns the loss by calculating L2 difference between reshaped x and y. This is a standard thing you'll run across very often in training networks.
Consider x to be shape (5,10) and y to be shape (5,5,10). So, we need to add a dimension to x, then repeat it along the added dimension to match the dimension of y. Then, (x-y) will be the shape (5,5,10). We will have to add over all three dimensions i.e. three torch.sum() to get a scalar.
class Regress_Loss(torch.nn.Module):
def __init__(self):
super(Regress_Loss,self).__init__()
def forward(self,x,y):
y_shape = y.size()[1]
x_added_dim = x.unsqueeze(1)
x_stacked_along_dimension1 = x_added_dim.repeat(1,NUM_WORDS,1)
diff = torch.sum((y - x_stacked_along_dimension1)**2,2)
totloss = torch.sum(torch.sum(torch.sum(diff)))
return totloss