基于长短期记忆网络的视频摘要
作者:Ke Zhang, Wei-Lun Chao, Fei Sha, and Kristen Grauman
单位:University of Southern California、University of Texas at Austin
论文来自2016 ECCV,作者来自USC、UT 论文地址:https://arxiv.org/abs/1605.08110 DOI: 10.1007/978-3-319-46478-7 47
写在前面: 中间第3节公式多的部分没咋翻译,后边也没咋弄太好,多担待,原文还是要看的,毕竟翻译的有些不准确 -_-||
Abstract
We propose a novel supervised learning technique for summarizing videos by automatically selecting keyframes or key subshots. Casting the task as a structured prediction problem, our main idea is to use Long Short-Term Memory (LSTM) to model the variable-range temporal dependency among video frames, so as to derive both representative and compact video summaries. The proposed model successfully accounts for the sequential structure crucial to generating meaningful video summaries, leading to state-of-the-art results on two benchmark datasets. In addition to advances in modeling techniques, we introduce a strategy to address the need for a large amount of annotated data for training complex learning approaches to summarization. There, our main idea is to exploit auxiliary annotated video summarization datasets, in spite of their heterogeneity in visual styles and contents. Specifically, we show that domain adaptation techniques can improve learning by reducing the discrepancies in the original datasets’ statistical properties.
Keywords : Video summarization;Long short-term memory
我们提出了一种新颖的监督学习技术,可通过自动选择关键帧或关键子镜头来汇总视频。将任务投射为结构化的预测问题,我们的主要思想是使用长短期记忆(LSTM)对视频帧之间的可变范围的时间相关性进行建模,从而得到既有代表性又紧凑的视频摘要。该模型成功地解释了序列结构对生成有意义的视频摘要的重要性,并在两个基准数据集上获得了最新的结果。除了建模技术方面的进步外,我们还介绍了一种策略,用于解决训练复杂的摘要学习方法时需要大量注释数据的问题。尽管视觉样式和内容的异质性,我们的主要思想是利用辅助带注释的视频摘要数据集。具体来说,我们证明了领域自适应技术可以通过减少原始数据集的统计属性差异来改善学习。除了在建模技术方面的进步之外,我们引入了一种策略来解决训练复杂学习方法时对大量注释数据的需求。在这里,我们的主要想法是利用辅助带有注释的视频摘要数据集,尽管它们在视觉风格和内容上是异构的。具体地说,我们表明领域适应技术可以通过减少原始数据集统计特性的差异来改善学习。
1 introduction
Video has rapidly become one of the most common sources of visual information. The amount of video data is daunting — it takes over 82 years to watch all videos uploaded to YouTube per day! Automatic tools for analyzing and understanding video contents are thus essential. In particular , automatic video summarization is a key tool to help human users browse video data. A good video summary would compactly depict the original video, distilling its important events into a short watchable synopsis. Video summarization can shorten video in several ways. In this paper, we focus on the two most common ones: keyframe selection, where the system identifies a series of defining frames [1,2,3,4,5] and key subshot selection, where the system identifies a series of defining subshots, each of which is a temporally contiguous set of frames spanning a short time interval [6,7,8,9].
视频已迅速成为视觉信息的最常见来源之一。视频数据量巨大,每天观看上传到YouTube的所有视频需要82年以上的时间! 因此,用于分析和理解视频内容的自动工具至关重要。特别地,自动视频摘要是帮助人类用户浏览视频数据的关键工具。一个好的视频摘要将紧凑地(简洁地)描述原始视频,并将其重要事件提炼成一个可供观看的简短摘要。视频摘要可以通过多种方式缩短视频。在本文中,我们重点介绍两种最常见的方法:关键帧选择(其中系统识别一系列定义帧[1,2,3,4,5])和关键子镜头的选择(其中系统识别一系列定义的子镜头),其中每个子镜头是一个时间连续的帧集,跨度很短的时间间隔[6,7,8,9]。
There has been a steadily growing interest in studying learning techniques for video summarization. Many approaches are based on unsupervised learning, and define intuitive criteria to pick frames [1,5,6,9,10,11,12,13,14] without explicitly optimizing the evaluation metrics. Recent work has begun to explore supervised learning techniques [2,15,16,17,18]. In contrast to unsupervised ones, supervised methods directly learn from human-created summaries to capture the underlying frame selection criterion as well as to output a subset of those frames that is more aligned with human semantic understanding of the video contents.
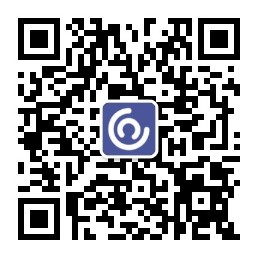
对研究视频摘要的学习技术的兴趣一直在稳定增长。许多方法都是基于无监督学习的,并定义了直观的标准来挑选框架[1、5、6、9、10、11、12、13、14],而没有明确优化评估指标。 最近的工作已开始探索监督学习技术[2,15,16,17,18]。 与不受监督的方法相反,受监督的方法直接从人工创建的摘要中学习,以捕获底层的帧选择标准,并输出与人类对视频内容的语义理解更加一致的那些帧的子集。
Supervised learning for video summarization entails two questions: what type of learning model to use? and how to acquire enough annotated data for fitting those models? Abstractly, video summarization is a structured prediction problem: the input to the summarization algorithm is a sequence of video frames, and the output is a binary vector indicating whether a frame is to be selected or not. This type of sequential prediction task is the underpinning of many popular algorithms for problems in speech recognition, language processing, etc. The most important aspect of this kind of task is that the decision to select cannot be made locally and in isolation—the inter-dependency entails making decisions after considering all data from the original sequence.
视频摘要的监督学习涉及两个问题:要使用哪种类型的学习模型?以及如何获取足够的带注释数据以拟合这些模型?抽象而言,视频摘要是结构化的预测问题:摘要算法的输入是视频帧序列,输出是表示是否要选择一帧的二进制向量。这种类型的序列预测任务是针对语音识别,语言处理等问题的许多流行算法的基础。此类任务的最重要方面是,选择的决定不能本地且孤立地进行——依赖关系需要在考虑原始序列中的所有数据后做出决策。
For video summarization, the inter-dependency across video frames is complex and highly inhomogeneous. This is not entirely surprising as human viewers rely on high-level semantic understanding of the video contents (and keep track of the unfolding of storylines) to decide whether a frame would be valuable to keep for a summary. For example, in deciding what the keyframes are, temporally close video frames are often visually similar and thus convey redundant information such that they should be condensed. However, the converse is not true. That is, visually similar frames do not have to be temporally close. For example, consider summarizing the video “leave home in the morning and come back to lunch at home and leave again and return to home at night.” While the frames related to the “at home” scene can be visually similar, the semantic flow of the video dictates none of them should be eliminated. Thus, a summarization algorithm that relies on examining visual cues only but fails to take into consideration the high-level semantic understanding about the video over a long-range temporal span will erroneously eliminate important frames. Essentially, the nature of making those decisions is largely sequential – any decision including or excluding frames is dependent on other decisions made on a temporal line.
对于视频摘要,视频帧之间的相互依赖关系是复杂的和高度不均匀的。这并不完全令人惊讶,因为人类观众依赖于对视频内容的高级语义理解(并跟踪故事情节的展开)来决定一个帧是否有作为视频摘要的价值。例如,在确定关键帧是什么时,时间上接近的视频帧通常在视觉上是相似的,因此传递了冗余信息,因此应该对其进行压缩。然而,反过来就不正确了。也就是说,视觉上相似的帧不一定在时间上接近。例如,考虑对如下视频进行摘要:“早上离开家,回到家里吃午餐,然后再次离开,晚上回到家。”尽管与“在家”场景相关的帧在视觉上可以相似,但视频的语义流要求它们都不能被删除。因此,一个仅依赖于检查视觉线索而没有考虑到对视频在长时间跨度上的高级语义理解的摘要算法将错误地消除重要帧。本质上,做出这些决策的本质是顺序的——任何包括或排除帧的决策都依赖于在时间线上做出的其他决策。
Modeling variable-range dependencies where both short-range and long-range relationships intertwine is a long-standing challenging problem in machine learning. Our work is inspired by the recent success of applying long short-term memory (LSTM) to structured prediction problems such as speech recognition [19,20,21] and image and video captioning [22,23,24,25,26]. LSTM is especially advantageous in modeling long-range structural dependencies where the influence by the distant past on the present and the future must be adjusted in a data-dependent manner. In the context of video summarization, LSTMs explicitly use its memory cells to learn the progression of “storylines”, thus to know when to forget or incorporate the past events to make decisions.
在机器学习中,对短期和长期关系交织下的可变范围的依赖关系进行建模是一个长期存在的挑战性问题。我们的工作受到了最近将长短期记忆(LSTM)成功应用于结构化预测问题的启发,如语音识别[19,20,21]及图像和视频字幕[22,23,24,25,26]。LSTM在对长期结构依赖关系方面进行建模特别有优势,因为遥远的过去对现在和未来的影响必须以依赖数据的方式加以调整。在视频摘要的背景下,LSTMs明确地使用它的记忆细胞来学习故事情节的进展,从而知道何时忘记或合并过去的事件来做决定。
In this paper, we investigate how to apply LSTM and its variants to supervised video summarization. We make the following contributions. We propose vsLSTM, a LSTM-based model for video summarization (Sec. 3.3). Fig. 2 illustrates the conceptual design of the model. We demonstrate that the sequential modeling aspect of LSTM is essential; the performance of multi-layer neural networks (MLPs) using neighboring frames as features is inferior. We further show how LSTM’s strength can be enhanced by combining it with the determinantal point process (DPP), a recently introduced probabilistic model for diverse subset selection [2,27]. The resulting model achieves the best results on two recent challenging benchmark datasets (Sec. 4). Besides advances in modeling, we also show how to address the practical challenge of insufficient human-annotated video summarization examples. We show that model fitting can benefit from combining video datasets, despite their heterogeneity in both contents and visual styles. In particular, this benefit can be improved by “domain adaptation” techniques that aim to reduce the discrepancies in statistical characteristics across the diverse datasets.
在本文中,我们研究了如何将LSTM及其变体应用于监督视频摘要。我们做出以下贡献。我们提出了vsLSTM,一种基于LSTM的视频摘要模型(第3.3节)。模型的概念设计如图2所示。我们证明了LSTM在序列建模方面至关重要。以相邻帧作为特征的多层神经网络(MLP)的性能较差。我们进一步展示了如何通过将LSTM与确定性点过程(DPP)相结合来增强LSTM的强度,DPP是一种最近引入的用于不同子集选择的概率模型[2,27]。所得到的模型在最近两个具有挑战性的基准数据集上达到了最佳结果(第4节)。除了建模方面的进步之外,我们还展示了如何解决人工注释视频摘要示例不足的实际挑战。我们展示了模型拟合可以从视频数据集的组合中受益,尽管这些视频数据集在内容和视觉样式上具有异质性。特别是,这种好处可以通过旨在减少不同数据集统计特征差异的“领域适应”技术得到改善。
The rest of the paper is organized as follows. Section 2 reviews related work of video summarization, and Section 3 describes the proposed LSTM-based model and its variants. In Section 4, we report empirical results. We examine our approach in several supervised learning settings and contrast it to other existing methods, and we analyze the impact of domain adapation for merging summarization datasets for training (Section 4.4). We conclude our paper in Section 5.
本文的其余部分组织如下。第2节回顾了视频摘要的相关工作,第3节描述了提出的基于lstm的模型及其变体。在第4部分,我们报告实证结果。我们在几种有监督的学习环境中检查了我们的方法,并将其与其他现有方法进行了对比,并分析了领域适应对合并摘要数据集进行训练的影响(第4.4节)。我们在第5节中总结我们的论文。
2 Related Work
Techniques for automatic video summarization fall in two broad categories: unsupervised ones that rely on manually designed criteria to prioritize and select frames or subshots from videos [1,3,5,6,9,10,11,12,14,28,29,30,31,32,33,34,35,36] and supervised ones that leverage human-edited summary examples (or frame importance ratings) to learn how to summarize novel videos [2,15,16,17,18]. Recent results by the latter suggest great promise compared to traditional unupservised methods.
自动视频摘要的技术分为两大类:无监督的技术(该技术依赖于人工手动设计的标准来对视频中的帧或子镜头进行优先排序和选择[1、3、5、6、9、10、11、12、14、28、29 ,30、31、32、33、34、35、36])和监督的技术(该技术利用人工编辑的摘要示例(或视频帧重要性评级)来学习如何总结新视频[2,15,16,17,18])。最近的研究结果表明,与传统的未使用的方法相比,后者有很大的前景。
Informative criteria include relevance [10,13,14,31,36], representativeness or importance [5,6,9,10,11,33,35], and diversity or coverage [1,12,28,30,34]. Several recent methods also exploit auxiliary information such as web images [10,11,33,35] or video categories [31] to facilitate the summarization process.
信息标准包括相关性[10,13,14,31,36]、代表性或重要性[5,6,9,10,11,33,35]、多样性或覆盖率[1,12,28,30,34]。一些最近的方法也利用辅助信息,如web图像[10,11,33,35]或视频类别[31],以促进摘要过程。
Because they explicitly learn from human-created summaries, supervised methods are better equipped to align with how humans would summarize the input video. For example, a prior supervised approach learns to combine multiple hand-crafted criteria so that the summaries are consistent with ground truth [15,17]. Alternatively, the determinatal point process (DPP) — a probabilistic model that characterizes how a representative and diverse subset can be sampled from a ground set — is a valuable tool to model summarization in the supervised setting [2,16,18].
由于他们明确地从人工创建的摘要中学习,因此受监督的方法可以更好地适应人类对输入视频进行摘要的方式。 例如,先前的监督方法学会了结合多个手工制定的标准,以使摘要与基本事实保持一致[15,17]。 另外,确定点过程(DPP)是一种概率模型,它描述了如何从基础集合中抽取具有代表性的和多样性的子集。它是在有监督的环境中建模进行摘要的有价值的工具[2,16,18]。
None of above work uses LSTMs to model both the short-range and long-range dependencies in the sequential video frames. The sequential DPP proposed in [2] uses pre-defined temporal structures, so the dependencies are “hard-wired”. In contrast, LSTMs can model dependencies with a data-dependent on/off switch, which is extremely powerful for modeling sequential data [20].
以上工作均未使用LSTMs对于序列视频帧的短距离和长距离相关性进行建模。文献[2]中提出的序列DPP使用预定义的时间结构,因此依赖关系是“硬连接的”。相比之下,LSTMs可以使用依赖于数据的on/off开关来建模依赖关系,这对于建模顺序数据[20]非常强大。
LSTMs are used in [37] to model temporal dependencies to identify video highlights, cast as auto-encoder-based outlier detection. LSTMs are also used in modeling an observer’s visual attention in analyzing images [38,39], and to perform natural language video description [23,24,25]. However, to the best of our knowledge, our work is the first to explore LSTMs for video summarization. As our results will demonstrate, their flexibility in capturing sequential structure is quite promising for the task.
在文献[37]中使用LSTMs来对时间依赖性进行建模,以识别视频亮点,并转换为基于自动编码器的离群点检测(孤立点检测、异常值检测)。 LSTMs还用于在分析图像时对观察者的视觉注意力进行建模[38,39],并用于执行自然语言视频描述[23,24,25]。然而,就我们所知,我们的工作是第一次探索视频摘要的LSTMs。正如我们的结果将证明的那样,它们在捕获顺序结构方面的灵活性对于这项任务是很有前途的。
3 Approach
In this section, we describe our methods for summarizing videos. We first formally state the problem and the notations, and briefly review LSTM [40,41,42], the building block of our approach. We then introduce our first summarization model vsLSTM. Then we describe how we can enhance vsLSTM by combining it with a determinantal point process (DPP) that further takes the summarization structure (e.g., diversity among selected frames) into consideration.
在本节中,我们将介绍视频摘要的方法。首先正式地陈述问题和符号,并简要回顾LSTM[40,41,42],以及我们方法的组成部分。然后介绍我们的第一个总结模型vsLSTM。然后,我们描述了如何通过将vsLSTM与确定性点过程(DPP)结合来增强vsLSTM, DPP进一步考虑了摘要结构(例如,选择帧之间的多样性)。
3.1 Problem Statement
We use x = {x1, x2, · · · , xt, · · · , xT } to denote a sequence of frames in a video to be summarized while xt is the visual features extracted at the t-th frame.
我们用x = {x1, x2,···,xt,···,xT}表示要进行摘要的视频帧序列,xt是在第t帧提取的视觉特征。
The output of the summarization algorithm can take one of two forms. The first is selected keyframes [2,3,12,28,29,43], where the summarization result is a subset of (isolated) frames. The second is interval-based keyshots [15,17,31,35], where the summary is a set of (short) intervals along the time axis. Instead of binary information (being selected or not selected), certain datasets provide frame-level importance scores computed from human annotations [17,35]. Those scores represent the likelihoods of the frames being selected as a part of summary. Our models make use of all types of annotations — binary keyframe labels, binary subshot labels, or frame-level importances — as learning signals.1
摘要算法的输出可以有两种形式。首先是选定的关键帧[2,3,12,28,29,43],其中摘要结果是(孤立的)帧的子集。第二种是基于间隔的按键[15,17,31,35],其中摘要是沿时间轴的一组(短)间隔。不同于二进制信息(被选择或未被选择),某些数据集提供从人类注释中计算出的帧级重要性分数[17,35]。这些分数代表选择该帧作为摘要一部分的可能性。我们的模型利用了所有类型的注释(二进制关键帧标签、二进制子镜头标签或帧级重要性)作为学习信号.
Our models use frames as its internal representation. The inputs are frame-level features x and the (target) outputs are either hard binary indicators orframe-level importance scores (i.e., softened indicators).
我们的模型使用视频帧作为其内部表示。输入是帧级特征x,(目标)输出要么是硬二进制指标,要么是帧级重要性分数(即软化指标)。
3.2 Long Short-Term Memory (LSTM)
LSTMs are a special kind of recurrent neural network that are adept at modeling long-range dependencies. At the core of the LSTMs are memory cells c which encode, at every time step, the knowledge of the inputs that have been observed up to that step. The cells are modulated by nonlinear sigmoidal gates, and are applied multiplicatively. The gates determine whether the LSTM keeps the values at the gates (if the gates evaluate to 1) or discard them (if the gates evaluate to 0).
LSTMs是一种特殊的递归神经网络,擅长于建模远程依赖关系。在LSTMs的核心是记忆单元c,它在每一个时间步长对之前观察到的输入信息进行编码。单元采用非线性s形门调制,并进行乘法运算。门决定LSTM是将值保留在门上(如果门的值为1)还是丢弃它们(如果门的值为0)。
There are three gates: the input gate (i) controlling whether the LSTM considers its current input (xt), the forget gate (f) allowing the LSTM to forget its previous memory (ct), and the output gate (o) deciding how much of the memory to transfer to the hidden states (ht). Together they enable the LSTM to learn complex long-term dependencies–in particular, the forget date serves as a time-varying data-dependent on/off switch to selectively incorporating the past and present information. See Fig.1 for a conceptual diagram of a LSTM unit and its algebraic definitions [21].
有三个门:输入门(i)控制LSTM是否考虑它的当前输入(xt),遗忘门(f)允许LSTM忘记它以前的记忆(ct),和输出门(o)决定有多少记忆转移到隐藏状态(ht)。它们使LSTM能够学习复杂的长期依赖关系,特别是,遗忘日期作为一个时变数据依赖于 开/闭 开关,以选择性地合并过去和现在的信息。LSTM单元的概念图及其代数定义[21]见图1。
Fig.1
Fig. 1.The LSTM unit, redrawn from [21]. The memory cell is modulated jointly by the input, output and forget gates to control the knowledge transferred at each timestep. ⊙ denotes element-wise products.
图1所示。从[21]重画的LSTM单元。记忆单元由输入、输出和遗忘门共同调制,以控制在每个时间步的知识转移。按元素运算
3.3 vsLSTM for Video Summarization
Our vsLSTM model is illustrated in Fig.2. There are several differences from the basic LSTM model. We use bidirectional LSTM layers [44] for modeling better long-range dependency in both the past and the future directions. Note that the forward and the backward chains do not directly interact. We combine the information in those two chains, as well as the visual features, with a multi-layer perceptron (MLP). The output of this perceptron is a scalar
我们的vsLSTM模型如图2所示。与基本的LSTM模型有几个不同之处。我们使用双向LSTM层[44]来更好地建模过去和未来方向的长期依赖关系。请注意,正向链和反向链并不直接相互作用。我们使用多层感知器(MLP)将这两个链中的信息以及视觉特性结合起来。这个感知器的输出是一个标量
To learn the parameters in the LSTM layers and the MLP for fI(·), our algorithm can use annotations in the forms of either the frame-level importance scores or the selected keyframes encoded as binary indicator vectors. In the former case, y is a continuous variable and in the latter case, y is a binary variable. The parameters are optimized with stochastic gradient descent.
为了学习fI(·)的LSTM层和MLP中的参数,我们的算法可以使用帧级重要性分数或所选关键帧编码为二进制指示向量的形式进行注释。前一种情况下,y是连续变量,后一种情况下,y是二元变量。采用随机梯度下降法对参数进行优化。
Fig.2
Fig.2. Our vsLSTM model for video summarization. The model is composed of two LSTM (long short-term memory) layers: one layer models video sequences in the for-ward direction and the other the backward direction. Each LSTM block is a LSTM unit, shown in Fig.1.
The forward/backward chains model temporal inter-dependencies between the past and the future. The inputs to the layers are visual features extracted at frames. The outputs combine the LSTM layers’ hidden states and the visual features with a multi-layer perceptron, representing the likelihoods of whether the frames should be included in the summary. As our results will show, modeling sequential structures as well as the long-range dependencies is essential.
图2: 我们的vsLSTM模型(用于视频摘要)。该模型由两层LSTM(长短期存储器)组成:一层对视频序列进行正向建模,另一层对视频序列进行反向建模。每个LSTM块都是一个LSTM单元,如图1所示。
前向/后向链建模过去和未来之间的时间相互依赖关系。各层的输入是按帧提取的视觉特征。输出用多层感知器将LSTM层的隐藏状态和视觉特征结合起来,表示在摘要中是否包含该帧的可能性。正如我们结果将显示的那样,对顺序结构以及长期依赖关系进行建模是必要的。
3.4 Enhancing vsLSTM by Modeling Pairwise Repulsiveness
通过成对排斥建模增强vsLSTM
vsLSTM excels at predicting the likelihood that a frame should be included or how important/relevant a frame is to the summary. We further enhance it with the ability to model pairwise frame-level “repulsiveness” by stacking it with a determinantal point process (DPP) (which we discuss in more detail below). Modeling the repulsiveness aims to increase the diversity in the selected frames by eliminating redundant frames. The modeling advantage provided in DPP has been exploited in DPP-based summarization methods [2,16,18]. Note that diversity can only be measured “collectively” on a (sub)set of (selected) frames, not on frames independently or sequentially. The directed sequential nature in LSTMs is arguably weaker in examining all the fames simultaneously in the subset to measure diversity, thus is at the risk of having higher recall but lower precision. On the other hand, DPPs likely yield low recalls but high precisions. In essence, the two are complementary to each other.
vsLSTM擅长预测一个帧应该被包含的可能性,或者一个帧对摘要的重要性或相关性。通过将其与确定点过程(DPP)叠加(我们将在下面详细讨论),我们进一步增强了对帧级“排斥”进行建模的能力。排斥建模的目的是通过消除冗余帧来增加所选帧的多样性。基于DPP的摘要方法利用了DPP提供的建模优势[2,16,18]。注意,多样性只能在一组(选定的)帧上“集体地”测量,而不能在帧上独立地或顺序地测量。LSTMs的有向序列特性在同时检查子集中所有的fames(谣言?饥饿?)以测量多样性方面更弱,因此存在较高召回率但较低精度的风险。另一方面,DPPs可能产生较低的召回率但较高的精度。这两者在本质上是相辅相成的。
Fig.3
Fig.3.Our dppLSTMmodel. It combines vsLSTM (Fig.2) and DPP by modeling both long-range dependencies and pairwise frame-level repulsiveness explicitly.
图3.我们的dppLSTMmodel。它结合了vsLSTM(图2)和DPP,通过显式地建模远程依赖和成对帧级排斥。
**Determinantal point processes (DPP).**Given a ground set Z of N items(e.g., all frames of a video), together with an N×N kernel matrix that records the pairwise frame-level similarity, a DPP encodes the probability to sample any subset from the ground set [2,27]. The probability of a subset z is proportional to the determinant of the corresponding principal minor of the matrix Lz
确定性点过程(DPP)。给定一个包含N个元素的基础集合Z(比如视频的所有帧)以及记录帧级相似度的N×N核矩阵。DPP会对从基础集合中抽取的任何子集的概率进行编码[2,27]。子集z的概率正比于矩阵Lz的相应主子式的行列式
Where I is the N×N identity matrix. If two items are identical and appear in the subset, Lz will have identical rows and columns, leading to zero-valued determinant. Namely, we will have zero-probability assigned to this subset. A highly probable subset is one capturing significant diversity (i.e., pairwise dissimilarity)
其中I是N×N的单位矩阵。如果两个元素是相同的并且出现在子集中,Lz将具有相同的行和列,从而导致零值的行列式。也就是说,这个子集的赋值概率为零。一个高度可能的子集是一个捕获显著多样性的子集。两两不同)
搞不下去了,公式太多翻起来太麻烦,这部分后续的自己看吧。。。
3.5 Generating Shot-Based Summaries from Our Models
从模型中生成基于瞬间的摘要
Our vsLSTM predicts frame-level importance scores, i.e., the likelihood that a frame should be included in the summary. For our dppLSTM, the approximate MAP inference algorithm [46] outputs a subset of selected frames. Thus, for dppLSTM we use the procedure described in the Supplementary Material to convert them into key shot-based summaries for evaluation.
我们的vsLSTM预测帧级重要性分数,即某一帧被包含在摘要中的可能性。对于我们的dppLSTM,近似映射推理算法[46]输出选定帧的子集。因此,对于dppLSTM,我们使用补充材料中描述的过程将其转换为基于关键瞬间的摘要进行评估
4 实验结果
我们首先定义实验设置(数据集、特征、指标)。然后,我们提供关键的定量结果,证明我们的方法的优势,现有技术(第4.2节)。接下来,我们更深入地分析了我们的方法设计的影响(第4.3节),并探讨了领域适应在“同质化”不同摘要数据集中的使用(第4.4节)。最后,我们给出实例定性结果(第4.5节)
4.1 实验设置
数据集。我们在两个视频数据集上评估我们的模型的性能,SumMe[17]和TVSum[35]。SumMe由25个用户视频组成,记录各种各样的事件,如假期和运动。TVSum包含了从YouTube下载的50个视频,在TRECVid多媒体中定义了10个类别事件检测(地中海)。大多数视频都是1到5分钟的长度。
为了解决对大量注释数据的需求,我们使用了另外两个带有基于关键帧摘要注释的注释数据集:Youtube[28]和Open Video Project (OVP)[28,47]。我们将它们处理为[2],为每个视频创建关键帧的地基真值集(然后转换为帧级重要度分数的地基真值序列)。我们使用ground- truth in importance scores来训练vsLSTM,并将序列转换为选定的关键帧来训练dppLSTM。
为了进行评估,两个数据集都为每个视频提供了多个用户注释的摘要,要么以键击(SumMe)的形式,要么以帧级重要性分数(TVSum,可转换为基于键击的摘要)的形式。这种转换记录在补充材料中。
表1总结了这些数据集的主要特征。我们可以看到,这四个数据集在视觉风格和内容上都是异构的。
特性。对于大多数实验来说,每一帧的特征描述符都是通过提取GoogLeNet模型48的倒数第二层(pool 5)的输出来获得的。我们也用[35]中使用的相同的浅有限元法进行了实验。例如,颜色直方图,GIST, HOG,浓密的SIFT),以提供对深层特征的比较。
评价指标。遵循[15,17,35]协议,我们将生成的基于关键帧的摘要A限制在原始视频持续时间的15%以下(详见补充材料)。然后针对用户摘要B计算精度§和召回率®,根据两者在时间上的重叠:
以及他们的调和平均f分,
我们也遵循[15,35]计算的指标时,有多个人注释摘要的视频。
不同的监督学习设置。我们研究了几种监督学习的设置,总结在表2中:
1)规范。这是标准的监督学习设置,其中训练、验证和测试集来自相同的数据集,尽管它们是不相交的。
2)增强。在此设置中,对于给定的数据集,我们随机留下其中的20%用于测试,并使用其他三个数据集增加剩余的80%,从而形成一个扩充的训练和验证数据集。我们的假设是,尽管样式和内容是异构的,但由于增加了注释的数量,增强的数据集有助于提高模型的性能。
3)转移。在此设置中,对于给定的数据集,我们使用其他三个数据集来训练、验证和测试数据集上的学习模型。我们感兴趣的是研究现有的数据集是否能有效地将总和化模型转移到新的无注释的数据集。如果能成功转移就有可能对野生动物的大量视频进行总结,而野生动物几乎没有紧密对应的注释。
4.2 主要结果
表3总结了我们的方法的性能,并与之前的工作取得的结果进行了对比。红色的数字表示我们的dppLSTM在相应设置下获得了最佳性能。否则,最好的表演是加粗的。在“规范的”监督学习的常见设置中,对TVSum,我们的两种方法都超过了最先进的。然而,在我们的方法表现不佳,可能是由于较少的注释训练样本在SumMe。
特别有趣的是,当带注释的数据数量增加时,我们的方法可以得到显著改进。特别是在迁移学习的情况下,尽管这三个训练数据集与测试数据有很大的不同,我们的方法利用注解有效地提高了规范设置的准确性,在规范设置中,注解的训练数据是有限的。表现最好的设置是增广,我们将所有四个数据集组合在一起形成一个训练数据集。
结果表明,在有足够的注释数据的情况下,我们的模型可以更好地描述时间结构,而先前的方法既没有明确的节奏结构[11,15,17,30,35],也没有考虑预先定义的节奏结构[2,16]。更具体地说,双向LSTMs和DPPs有助于获得基于整个视频的不同结果,同时利用视频的顺序特性。进一步讨论请参阅补充材料。
4.3 分析
接下来,我们进一步分析几个感兴趣的设置。
序列建模有多重要?表4对比了基于lstm的方法vsLSTM和基于多层感知器的基线的性能。在这个基线中,我们学习了一个两隐藏层的MLP,它在每一层中都有相同数量的隐藏单元,就像我们模型中的一个MLP一样。
表3。各种视频摘要方法的性能(F-score)。已发表的结果以粗体斜体表示;我们的实现使用的是普通字体。空框表示设置不适用于测试的方法。
表4。使用LSTMs对视频数据进行建模是有益的。报告的数字是
通过各种总结方法得到f分。
由于MLP不能显式地捕获时间信息,为了与基于lstm的方法进行公平比较,我们考虑了两个变量。在第一种不同的MLP- shot中,我们使用投篮中的平均帧特征作为MLP的输入,并预测投篮水平的重要分数。地面真射击级的重要度得分是相应帧级重要度得分的平均值。然后使用预测的射击级重要度分数来选择关键射击,然后将结果的基于射击的摘要与用户注释进行比较。在第二个MLP-Frame中,我们将以每一帧为中心的K-frame(在我们的实验中K = 5)窗口内的所有视觉特征连接起来,作为预测帧级重要性得分的输入。
值得注意的是,在规范设置中,基于mlp的方法优于vsLSTM。然而,在anno-生产量增加的所有其他情况下,我们的vsLSTM能够超过基于mlp的方法
表5所示。摘要结果(F-score)由我们的dppLSTM使用浅层和深层特征。注意[35]报道TVSum有50.0%使用了相同的浅层特性。
表6所示。结果由vsLSTM对规范设置中的不同类型的注释,这证实了人们对LSTMs的普遍看法:尽管它们功能强大,但它们通常需要大量注释数据才能执行良好的性能。
浅层还是深层?我们还研究了对每一帧使用替代视觉特征的效果。从表5可以看出,深度特征能够略微提高浅层特征的性能。注意,我们的带有浅层特性的dppLSTM仍然优于[35],[35]报告了使用相同浅层特性(即浅层特性)在TVSum上的结果。,颜色直方图,GIST, HOG,浓密筛)。
哪种注释更有效?在视频摘要数据集中有两种常见的注释类型:一个帧是否被选择的二进制指示器和帧级重要性分数,它决定了一个帧在摘要中被包含的可能性有多大。虽然我们的模型可以采用这两种格式,但我们怀疑框架级重要性分数比二进制指标提供了更丰富的信息,因为它们代表了框架之间的相对良度。
表6演示了在规范设置中使用两种不同注释时vsLSTM模型的性能。使用框架级重要性得分具有一致的优势。
但是,这并不意味着不能利用二进制注释/关键帧注释。我们的dppLSTM利用帧级重要性分数和二进制信号。具体来说,dppLSTM首先使用帧级重要性分数来训练它的LSTM层,然后使用二进制指标来形成目标函数来进行精调(此阶段训练的细节参见第3节)。因此,比较表3、表4、表5和表6中的结果,我们可以看到dppLSTM通过利用这两种类型的注释得到了进一步的改进。
4.4 基于领域自适应的训练数据扩充
虽然表3清楚地表明了增加训练数据集的优势,但这些辅助数据集往往与目标数据集在内容和风格上有所不同。我们进一步改进总结,借鉴视觉域适应的思想用于对象识别[49-51]。主要思想是先消除数据分布的差异,然后再进行扩充。
表7显示了这种想法的有效性。我们使用简单的域映射技术[52]来减少四个数据集之间的数据分布差异,通过线性变换视觉特征,使四个数据集的covari矩阵彼此接近。同质化的数据集,当合并时(在转移和增强设置中),将导致一个改进的总结f分。对于较小的数据集SumMe,改进尤其明显。
表7所示。通过我们的模型在传输和增强设置中总结结果,可选地使用线性调整以减少跨数据集差异的可视化特征
4.5 定性结果
我们在图4中提供了视频摘要范例。我们举例说明了dppLSTM的时间mod能力,并与MLP-Shot进行了对比。
蓝色背景的高度表示视频的ground-truth帧级重要性得分。红色和绿色标记的间隔分别是dppLSTM和MLP-Shot选择作为汇总的间隔。dppLSTM可以捕获时间相关性,从而识别出视频中最重要的部分,即描述狗清洗耳朵的帧。然而,MLP-Shot完全没有选择这样的子镜头,即使这些子镜头比邻近的帧有更高的地基真值重要性得分。我们认为这是因为MLP-Shot没有正确地捕捉顺序语义流,并且缺乏这样的知识:如果相邻的帧很重要,那么中间的帧也可能很重要。
同样有趣的是,尽管DPP模型通常会消除相似的元素,dppLSTM仍然可以选择相似但重要的子镜头:两个带狗的人清洗狗的耳朵之前和之后的子镜头都被选择。这突出了dppLSTM自适应地建模远程(远程状态)依赖关系的能力。
图4。示例视频摘要由MLP-Shot和dppLSTM,连同地面真相重要性(蓝色背景)。详见正文。我们在[35]中索引视频。(颜色图在线)
图5.微型计算机体积很小。dppLSTM故障案例。详见正文。我们在[35]中索引视频。
图5显示了dppLSTM的一个失败案例。这是一个以自我为中心的户外视频,记录了非常丰富的内容。特别是在三明治店、建筑、食品和城市广场之间的场景变化。从总结的结果可以看出,dppLSTM仍然选择了不同的内容,但是没有捕捉到开始帧,这些帧都有很高的重要度,视觉上相似,但是时间上拥挤。在这种情况下,dppLSTM被迫删除其中一些,导致召回率较低。另一方面,MLP- Shot只需要预测重要性得分,而不需要多样性,这就导致了较高的召回率和f得分。有趣的是,MLP-Shot对视频结束时的预测很差,而dppLSTM建模的厌恶性为该方法提供了在视频结束时选择几帧的边缘。
总而言之,我们期望我们的方法在那些结构平稳变化(至少在一个短的间隔内)的视频上工作得很好,这样时间结构就能被很好地捕捉。对于变化快、内容多样的视频,可以辅以更高层次的语义提示(如[5,9]中所述的目标检测)。
5 结论
我们的工作是探索长短期记忆发展新的监督学习方法自动视频摘要。我们基于lstm的模型在两个具有挑战性的基准测试中优于其他方法。其中有几个关键因素:LSTMs捕捉可变范围内的相互依赖的建模能力,以及我们用DPP来补充LSTMs强度的想法,明确地建模帧间的排斥,以鼓励选择的帧多样化。虽然LSTMs需要大量的注释样例,但是我们展示了如何通过利用存在的其他anno- tated视频数据集来调节这种需求,尽管它们在风格和内容上是异构的。初步的结果是很有前途的,暗示了未来的研究方向,发展更复杂的技术,可以汇集大量可用的视频数据集,以进行视频摘要。特别是,通过学习编码视频内容的语义理解,并使用它们来指导可视化分析中的总结和其他任务,探索新的序列模型可以增强视频数据建模中的LSTMs能力,这将是非常有效的。