>>>この記事は、Pytorchがさらに洗練するために提供した公式チュートリアルを参照しています<<<
-
----以下は、Pytorchの公式教育Tensorboardで使用されている詳細なアドレスです。興味がある場合は、それを参照することもできます。
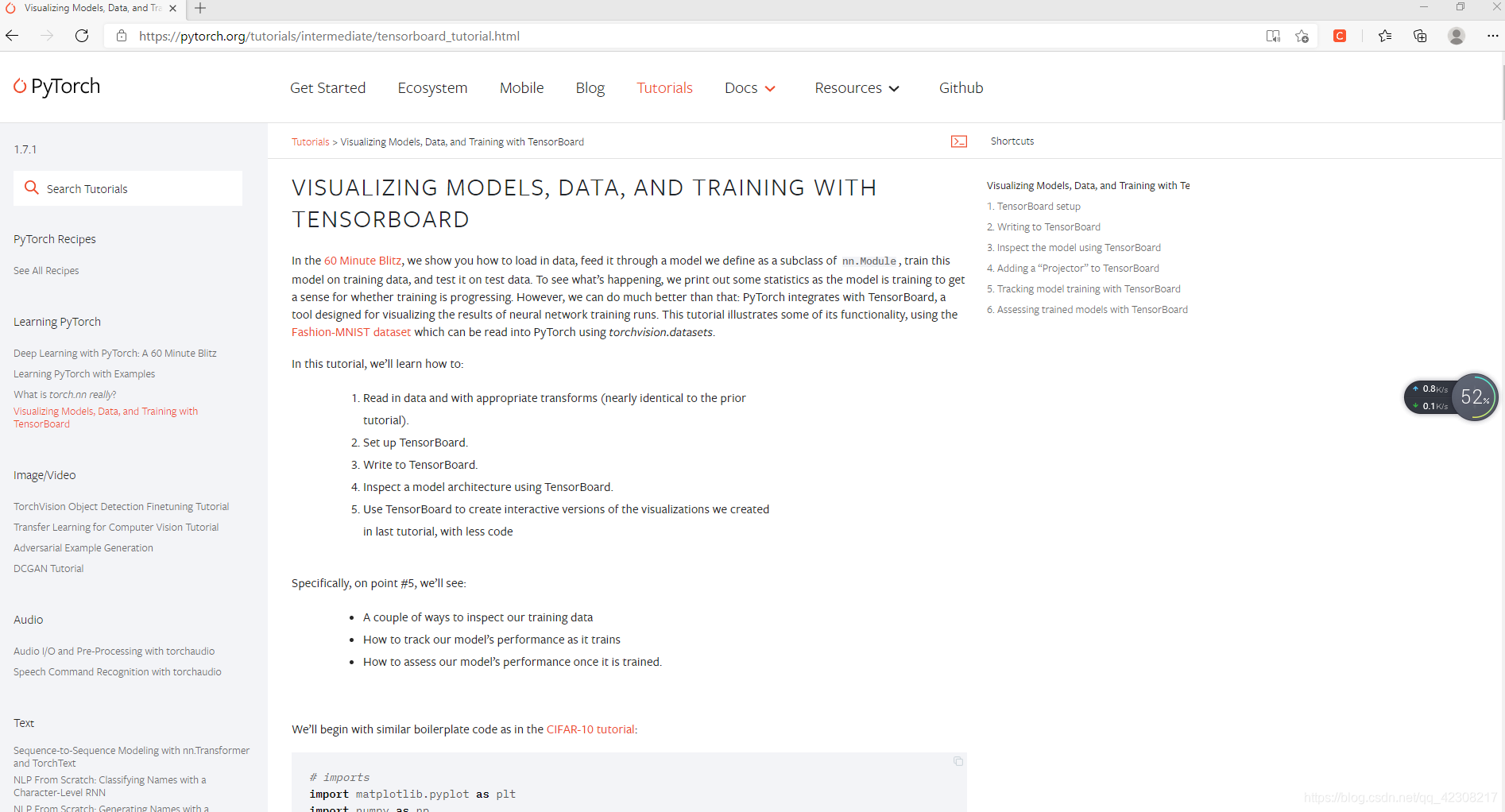
-
----私は通常、Tensorboardを使用するときにどの機能を使用するかわかりません。個人的には主に4つの機能を使っています:
>①ネットワークの構造図を保存する:TensorboardのGRAPHSには、モデルの構造図があり、モデル全体の各モジュールをはっきりと見ることができます。
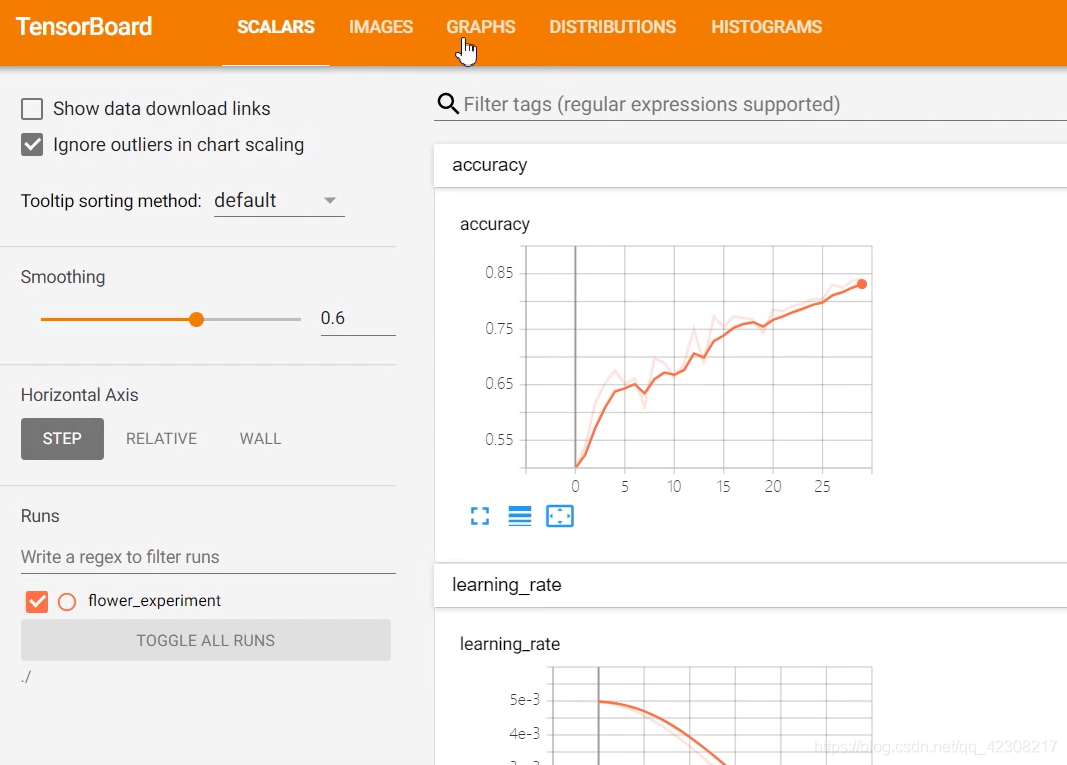
>②TensorboardのSCALARSにtraining_loss、validation set acc、learning_rateの変更を保存します。
>③TensorboardのHISTOGRAMSで各層構造の重み値の分布を確認します。
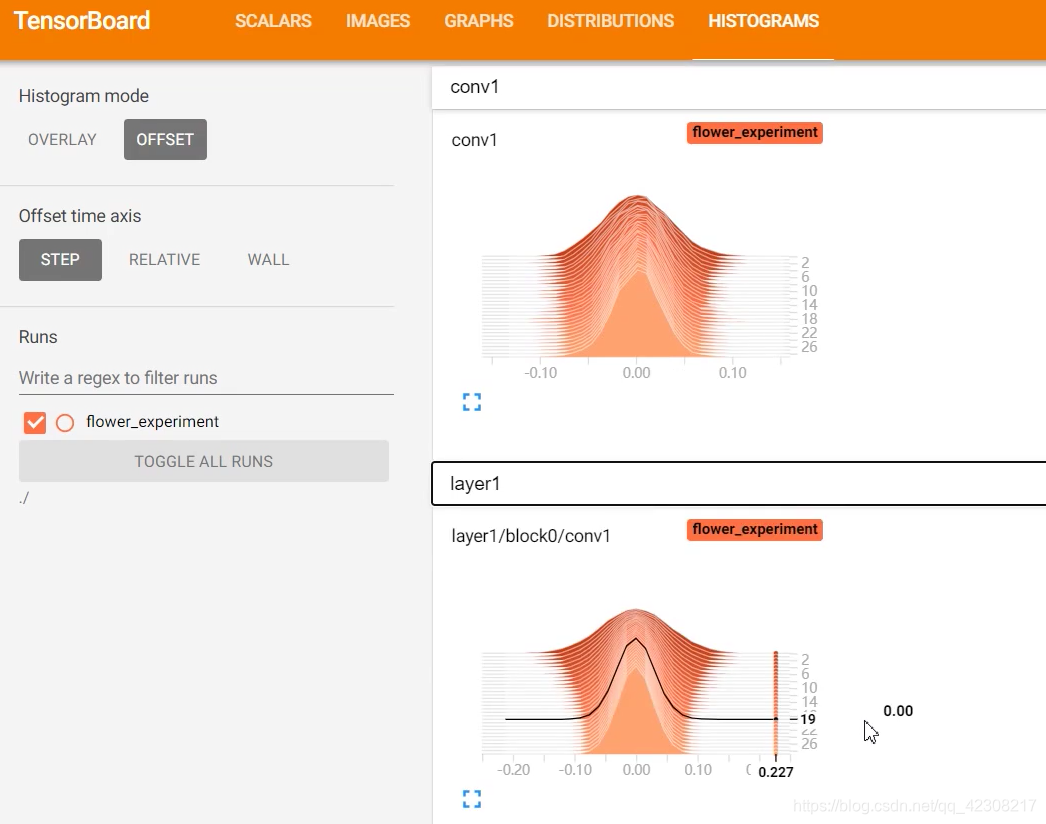
>④予測画像の情報を保存するテンソルボードの画像には、特定の画像の各ステップの予測結果が保存されます。
-
>>>この記事で使用されているネットワークモデルはResNetです。その原則とその構築方法については詳しく説明しません。次に、トピックに移りましょう<<<
最初にプロジェクトに入り、フォルダを作成する必要があります。そこに保存された画像はトレーニングプロセス中に予測され、結果がテンソルボードに追加されます。さらに、次の図に示すように、画像のラベルに対応するlabel.txtファイルも準備する必要があります。
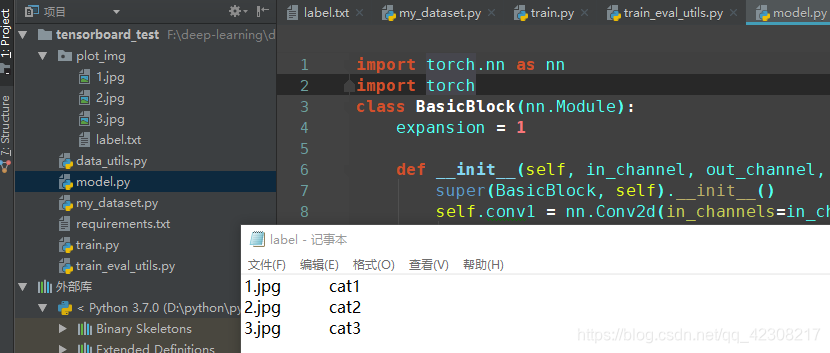
-> ResNet事前トレーニングウェイトのダウンロード方法:torchvision.models.resnet ctrlをインポートし、文中のresnetをクリックして、必要なウェイトをダウンロードします。ただし、この実験では事前トレーニングウェイトを使用していません、トレーニング前のウェイトを使用すると、accとlossは基本的に変更されておらず、トレーニングの最初のエポックの精度は97%に達していることがわかります。
テンソルボードステートメントのステップバイステップの説明:
-
SummaryWriterオブジェクトをインスタンス化します。パラメーターは、テンソルボードファイルが保存されるフォルダーです。ステートメントが実行されると、テンソルボードファイルが自動的に作成され、保存されます。
tb_writer = SummaryWriter(log_dir="runs/experiment")
-
モデルをインスタンス化した後、ゼロ行列を作成する必要があります。なぜこのゼロ行列を作成するのですか?:ネットワーク構造図を追加する場合は、順伝播のためにモデルに渡す必要があります。ネットワーク構造図は、マトリックスのサイズと写真は同じです。
model = resnet34(num_classes=args.num_classes).to(device)
init_img = torch.zeros((1, 3, 224, 224), device=device)
tb_writer.add_graph(model, init_img)
-
各エポックの後、つまり検証セットコードが実行された後、現在のエポックトレーニングセットの平均損失、検証セットacc、およびlearning_rateが保存されます。-------------注:tb_writer.add_scalarメソッドの使用:最初のパラメーターはラベルです。2番目のパラメーターはトレーニングプロセス中に収集されたデータです。ここでの値はテンソルではありません。ただし、浮動小数点データ。3番目のパラメーターは現在のトレーニングステップです。tb_writer.add_figureメソッドの使用:指定された画像の予測結果を追加し、それを画像に描画して、テンソルボードに保存します。最初のパラメーターは描画された画像のタイトル、2番目のパラメーターはFigureオブジェクト、そして3番目のパラメーターは現在のトレーニングステップです。
for epoch in range(args.epochs):
mean_loss = train_one_epoch(model=model,
optimizer=optimizer,
data_loader=train_loader,
device=device,
epoch=epoch)
scheduler.step()
acc = evaluate(model=model,
data_loader=val_loader,
device=device)
print("[epoch {}] accuracy: {}".format(epoch, round(acc, 3)))
tags = ["train_loss", "accuracy", "learning_rate"]
tb_writer.add_scalar(tags[0], mean_loss, epoch)
tb_writer.add_scalar(tags[1], acc, epoch)
tb_writer.add_scalar(tags[2], optimizer.param_groups[0]["lr"], epoch)
fig = plot_class_preds(net=model,
images_dir="./plot_img",
transform=data_transform["val"],
num_plot=5,
device=device)
if fig is not None:
tb_writer.add_figure("predictions vs. actuals",
figure=fig,
global_step=epoch)
tb_writer.add_histogram(tag="conv1",
values=model.conv1.weight,
global_step=epoch)
tb_writer.add_histogram(tag="layer1/block0/conv1",
values=model.layer1[0].conv1.weight,
global_step=epoch)
-
図はplot_class_preds()関数によって形成されます。そのパラメーター変換は検証セットで使用される画像前処理に対応し、パラメーター4は表示する画像の数です。
plot_class_preds(net,
images_dir: str,
transform,
num_plot: int = 5,
device="cpu"):
if not os.path.exists(images_dir):
print("not found {} path, ignore add figure.".format(images_dir))
return None
label_path = os.path.join(images_dir, "label.txt")
if not os.path.exists(label_path):
print("not found {} file, ignore add figure".format(label_path))
return None
json_label_path = './class_indices.json'
assert os.path.exists(json_label_path), "not found {}".format(json_label_path)
json_file = open(json_label_path, 'r')
flower_class = json.load(json_file)
class_indices = dict((v, k) for k, v in flower_class.items())
label_info = []
with open(label_path, "r") as rd:
for line in rd.readlines():
line = line.strip()
if len(line) > 0:
split_info = [i for i in line.split(" ") if len(i) > 0]
assert len(split_info) == 2, "label format error, expect file_name and class_name"
image_name, class_name = split_info
image_path = os.path.join(images_dir, image_name)
if not os.path.exists(image_path):
print("not found {}, skip.".format(image_path))
continue
if class_name not in class_indices.keys():
print("unrecognized category {}, skip".format(class_name))
continue
label_info.append([image_path, class_name])
if len(label_info) == 0:
return None
if len(label_info) > num_plot:
label_info = label_info[:num_plot]
num_imgs = len(label_info)
images = []
labels = []
for img_path, class_name in label_info:
img = Image.open(img_path).convert("RGB")
label_index = int(class_indices[class_name])
img = transform(img)
images.append(img)
labels.append(label_index)
images = torch.stack(images, dim=0).to(device)
with torch.no_grad():
output = net(images)
probs, preds = torch.max(torch.softmax(output, dim=1), dim=1)
probs = probs.cpu().numpy()
preds = preds.cpu().numpy()
fig = plt.figure(figsize=(num_imgs * 2.5, 3), dpi=100)
for i in range(num_imgs):
ax = fig.add_subplot(1, num_imgs, i+1, xticks=[], yticks=[])
npimg = images[i].cpu().numpy().transpose(1, 2, 0)
npimg = (npimg * [0.229, 0.224, 0.225] + [0.485, 0.456, 0.406]) * 255
plt.imshow(npimg.astype('uint8'))
title = "{}, {:.2f}%\n(label: {})".format(
flower_class[str(preds[i])],
probs[i] * 100,
flower_class[str(labels[i])]
)
ax.set_title(title, color=("green" if preds[i] == labels[i] else "red"))
return fig