
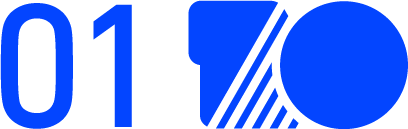
There are two recent news:
Sam Altman revealed the details of GPT-5 and publicly declared that the improvement of GPT-5 will be huge, and any company that underestimates it will be crushed. He also tweeted suggesting that OpenAI’s products this year will change human history.
Regarding the news about the first AI software engineer, the AI software engineer’s performance is already quite outstanding. He has the capabilities of overall planning, DevOp, and full project scanning. He is not far away from being a real programmer.
Although everything was expected, it still felt like it came a little faster.
Personally, I feel that the current progress of AI technology has at least two impacts:
At the level of national competition, with the blessing of AI, China’s previous major advantage over the United States—the number of outstanding college graduates—no longer exists. Who will win later will depend on who makes fewer mistakes.
At the level of personal jobs, it is expected that production methods will change in 2-5 years. If we do not keep up, we may suffer from dimensionality reduction. As a coder, it is imperative to learn the underlying principles of AI or participate in AI-related application practices.
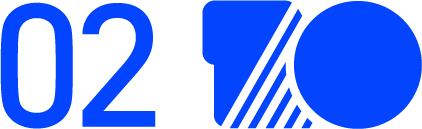
-
Explains the basic principles of neural networks and deep learning through a very simple network. -
Then I will give you some experience through a real neural network operation. -
Finally, a common question leads to the complex neural network that will be introduced in the following article.
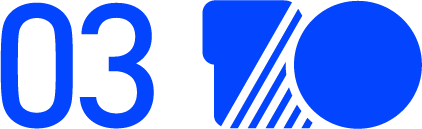
Key tips in this chapter:
Neural networks solve problems outside the training set by "learning" and storing the general rules of the training set.
Storage is ok. The neural network is divided into multiple layers, each layer has multiple neurons, and each neuron has N+1 parameters (where N is equal to the number of inputs connected to the neuron). The general rules "learned" are stored in these parameters (of all neurons).
The process is also certain. Because the "learning" process starts from any point in the high-dimensional parameter space (X parameters of all neurons) (the initial values of the parameters are randomly given), and gradually returns to the point with the lowest loss, it is a step-by-step adjustment of parameters. process. So if the formula for adjusting parameters is determined, then the "learning" process is determined. Yes, the formula for adjusting parameters is determined: when the model is compiled, the adjustment formula for each parameter of each neuron in each layer can be determined early based on the transitivity of partial derivatives.
▐What neural networks can do
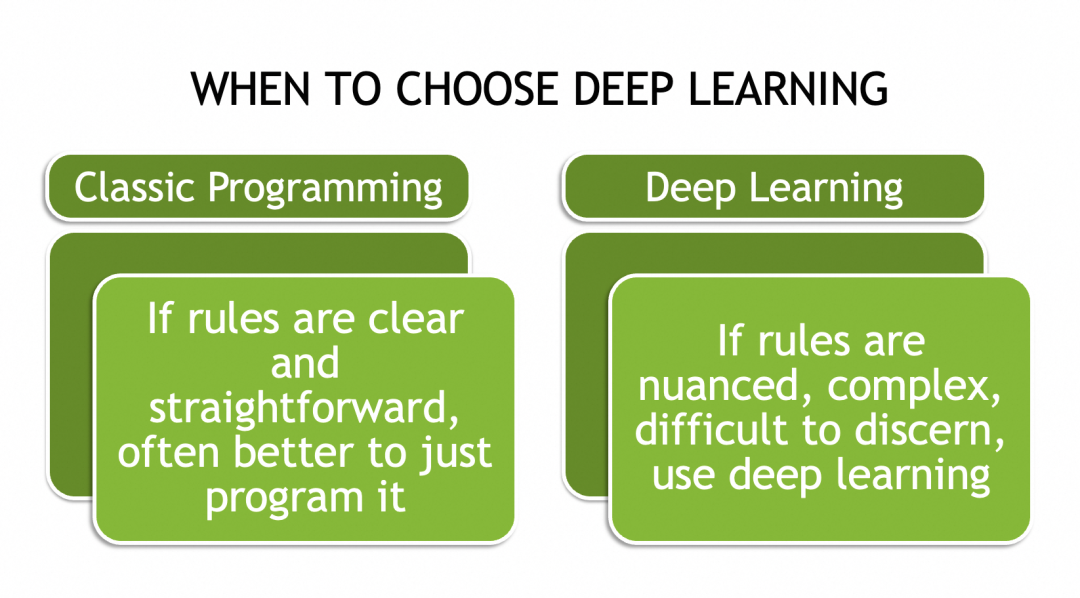
▐Why neural networks can solve complex problems
Complex problems are often not exhaustive (such as different cat pictures), so there is no set of rules that can be applied to all their instances. The only way is to abstract their general laws, or characteristics, or patterns.
For example, why text embedding can be used for natural language processing is because it does not (and cannot) record all text combinations, but records the pattern of a single text or token through a vector. This vector is the representation of the text. abstraction. If the vectors of two words are partially similar, this similarity may correspond to their common features in a specific context. We don't care about which vector value represents what feature. These are more like metadata of language and text.
Assume that after training, the vector representation of king is [0.3, 0.5, ..., 0.9], queen is [0.8, 0.5, ..., 0.2], and prince is [0.3, 0.7, ..., 0.5], In a certain context, such as xx is ruling the kingdom, xx can be filled with king or queen, then they may be determined by the second element of the vector, the common 0.5. In another context, such as xx is a Man, xx can be filled with king or prince, which may be determined by the first element.
As will be mentioned below, neural networks also have a mechanism for calculating and storing these abstract patterns, so neural networks are very suitable for solving complex problems involving abstract concepts.
▐How to implement neural network
Network 1
Neural network storage structure
Its structure is a single input, only one layer, and only one neuron in this layer.
Its parameters are the data trained on each neuron. There is only one neuron here, and it has two parameters, m and b.
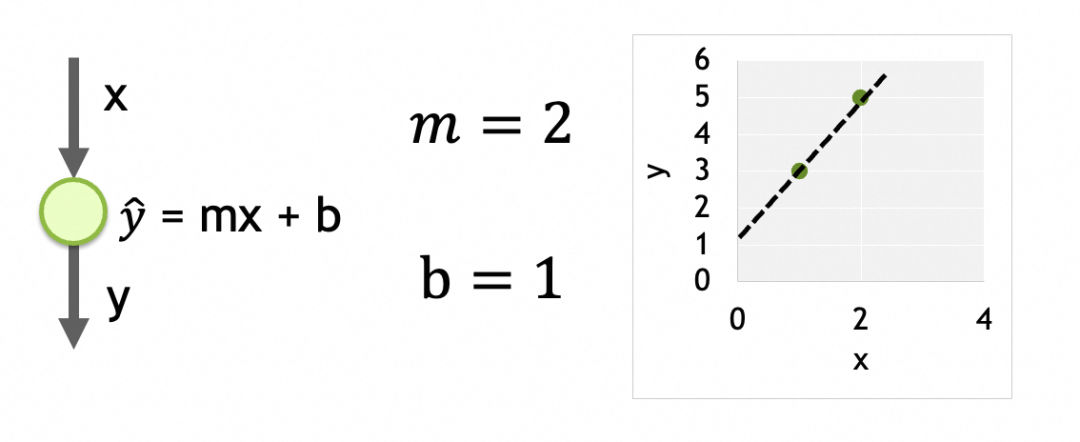
A network in which each layer is densely connected, each node is connected to all nodes in the upper layer, and all nodes in the upper layer are inputs to each node in the lower layer. The output of each node is the weighted sum of its inputs, the result after processing by the activation function: output = activation_function(W * X + b)
, where X is the input vector [x1, x2, ..., xn]
, W is the weights vector of each input [w1, w2, ..., wn]
, b is the bias constant, and activation_function is activation function. When the activation function is linear, output = W * X + b
. When a node has n inputs, the node has [w1, w2, ..., wn, b]
a total of n+1 parameters. There is a chain relationship between different layers of the network, and the results are passed downward until the last output layer. Any parameter of any neuron in any layer will affect the final result. So these parameters are the storage units of the neural network, used to store abstract patterns.
To give another example, [Network 2] in the figure below is a network with two inputs, two layers, 2 elements in the first layer, 1 element in the second layer, and the activation function of each layer is linear. The total number of parameters in the first layer of the network = (number of inputs + 1) the number of nodes = (2 + 1) 2 = 6, the total number of parameters in the second layer = (2 + 1) * 1 = 3, the total number of network parameters = 6 + 3 = 9, the abstracted pattern is stored in these parameters. (w111,w112,b11,w121,w122,b12,w21,w22,b2 in the picture below)
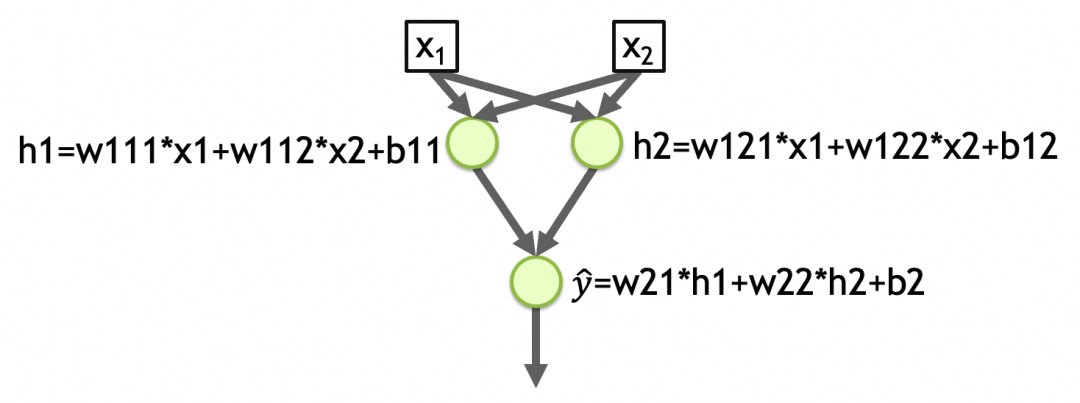
activation function
y_hat=w21*h1+w22*h2+b2=w21*(w111*x1+w112*x2+b11)+w22*(w121*x1+w122*x2+b12)+b2
最终可以化简为
y_hat=w1'*x1+w2'*x2+b'
。而一个线性函数(直线、平面、…)是没法拟合/抽象现实问题的复杂度的。
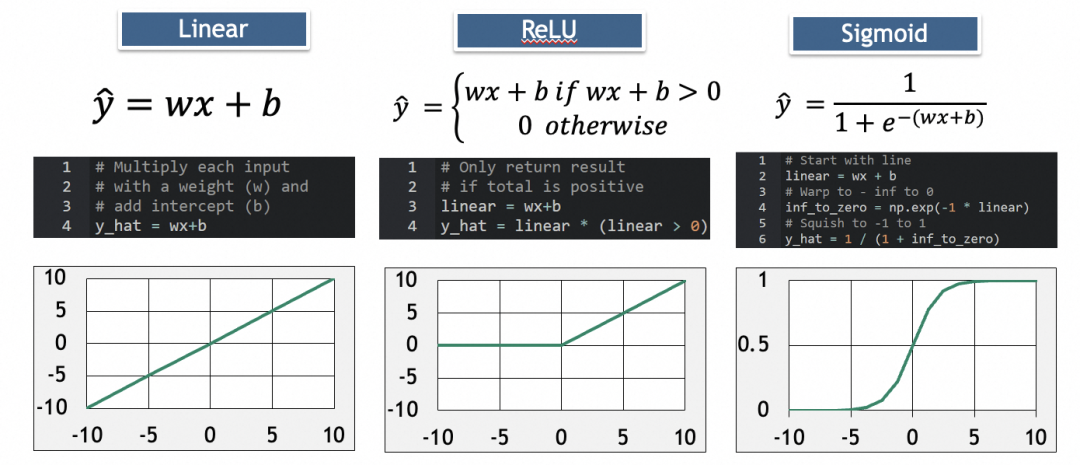
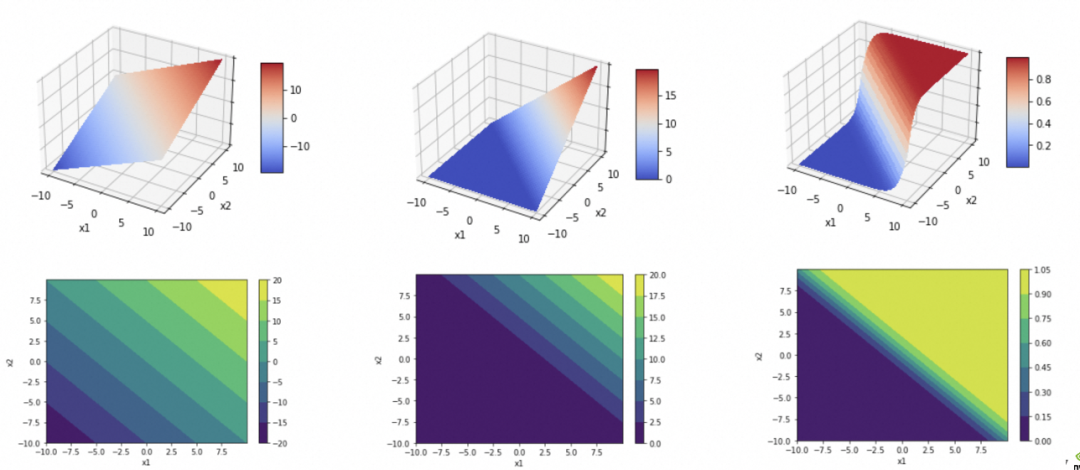
其中,ReLU用于消除负值,Sigmoid用于增加弧度。
深度学习过程
y_hat
。因为多层网络的计算是从上往下,所以称为前向传播:
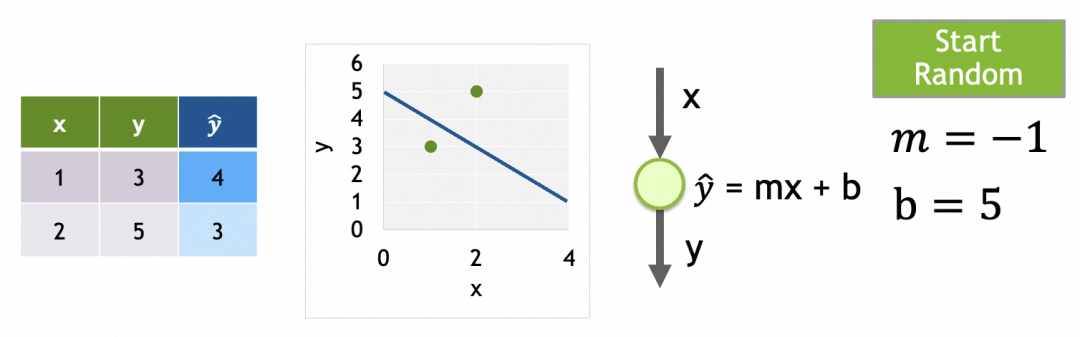
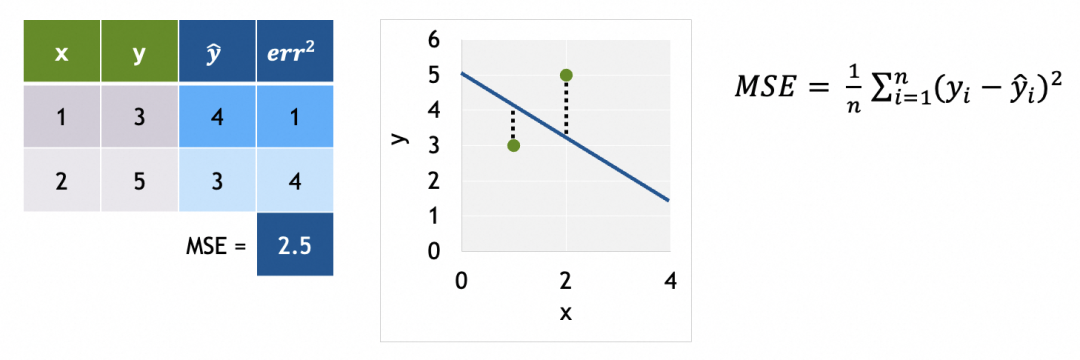
第4步、通过反向传播算法计算各参数的梯度(gradient)。
各参数的梯度,即单个参数的变化会多大程度影响损失结果的变化。计算各参数的梯度,再将参数往损失结果变小的方向调整,即可在高维参数空间中找到损失最小值点。
为了计算梯度,我们需要先了解损失曲面。
例子中只有m和b两个参数,如果将m和b作为x和y轴,损失函数结果作为z轴,可以得出损失曲面。(如果存在更多参数,形成高维参数空间,道理类似)
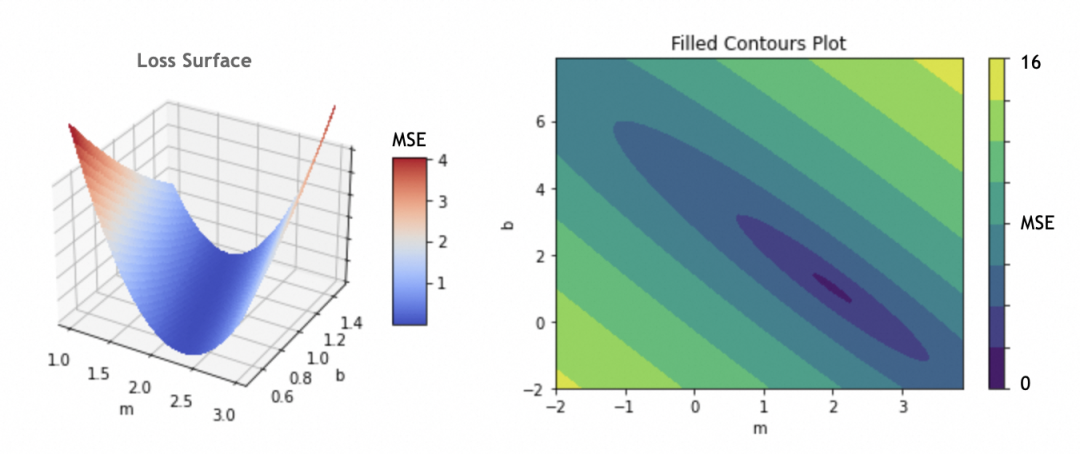
我们的目标是找出损失函数结果最小的m和b,这里即m=2,b=1。具体做法是将参数的当前值m=-1,b=5分次进行一定偏移,往m=2,b=1靠拢。
偏移具体怎么算呢?这就涉及到计算梯度,数学上就是计算偏导数。
对于一个曲面,当前在高处,要往低处移动,可以分别计算x、y两个方向(即m和b两个参数),在当前位置的梯度,即偏导数(partial derivative),即几何上的切线斜率,即单个参数的变化会多大程度影响损失结果的变化(注意梯度是向上的,用的话要取反)。
幸运的是,对于任何损失函数,都有对应公式算出对于某个参数的偏导数。例如,激活函数是y_hat = m * x + b
,损失函数是L = (1/N) * Σ(y - y_hat)^2
,则对于m的偏导数是∂L/∂w = (1/N) * Σ -2x(y - y_hat) = (1/N) * Σ -2x(y - (m * x + b))
。这里仅仅是说明能解,因为模型会提供这个能力,所以我们一般不人为关注。
不仅如此,神经网络还有传导性,拿[网络2]举例,如果我们希望算出第一层参数w111的梯度,则可以按∂L/∂w111 = ∂L/∂y_hat * ∂y_hat/∂h1 * ∂h1/∂w111
一层一层反向传播算回去,得到w111梯度具体的公式。
第5步、按梯度更新各参数:
因为偏导数是向上的,往低处走、梯度下降要取反。
拿m举例,m的新值m = m - learning_rate * ∂L/∂m
。
注意learning_rate的选择,过大会导致越过最低点,过小会导致单次变化太小,训练时间太长。不过一般模型会提供自动渐进式的算法,我们无需人为关注。
第6步、网络的训练过程是迭代的,在每个训练周期(epoch)中,网络将通过梯度下降的方式逐步调整其参数。整个过程会重复多个epoch,直到模型的表现不再显著提高或满足特定的停止条件。
每个训练周期,即会用训练集进行训练,也会用验证集进行验证。通过对比训练集和验证集的结果,能发现是否存在过拟合现象。
关于全局最优解和局部最优解:
如果损失曲面比较复杂,比如有多个低洼,从某个点渐进式移动不一定能找到最低的那个,这种情况会拿到局部最优解而不是全局最优解。
关于训练batch:如果训练集非常大,每个训练周期不一定会拿全部训练集进行训练,而是会随机选一批数据进行。常见的方式有Stochastic Gradient Descent(SGD),一次选一个样例,这样的好处不仅计算量大大减少,还容易从局部最优解跳出,缺点是不太稳定。另一种方式是Mini-Batch Gradient Descent,这种一次选10-几百个样例。具有SGD的优点且比SGD稳定,比较常用。
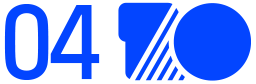
MNIST数据集是深度学习领域的一个经典数据集,它包含了大量的手写数字图像,对于验证深度学习算法的有效性具有标志性意义。
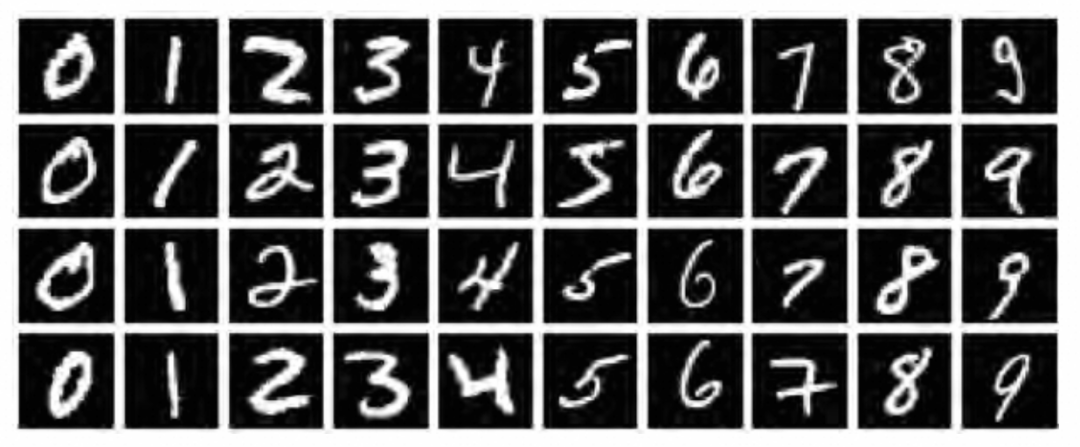
有了前面章节的理论,接下来,我们将通过一个实际案例来展示如何使用神经网络来解决图像分类问题。
下面的操作会用到jupyter平台和tensorflow2深度学习框架,但本文重点解释原理,平台和框架的使用就不多置笔墨了。
加载和观察数据集
在使用图像进行深度学习时,我们既需要图像本身(通常表示为 "X"),也需要这些图像的正确标签(通常表示为 "Y")。此外,我们需要一组X
和Y
来训练模型,然后还需要一组单独的X
和Y
来验证训练后模型的表现。因此,MNIST数据集需要分成4份:
x_train
:用于训练神经网络的图像y_train
:x_train
图像的正确标签,用于评估模型在训练过程中的预测结果x_valid
:模型训练完成后用于验证模型表现的图像y_valid
:x_valid
图像的正确标签,用于评估模型训练后的预测结果
x_train
和验证图像集
x_valid
:可以看到是分别是60000张和10000张28x28 pixel的灰阶图像(灰度0-255):
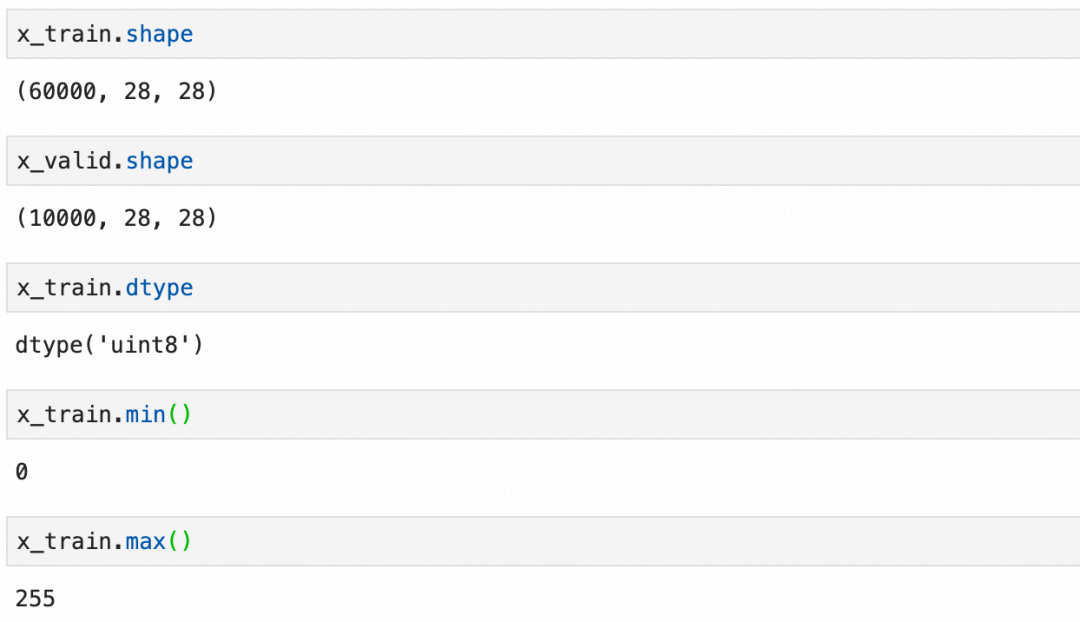
每个图像是一个图像grid的二维数组,下图是二维数组的内容和可视化图像:
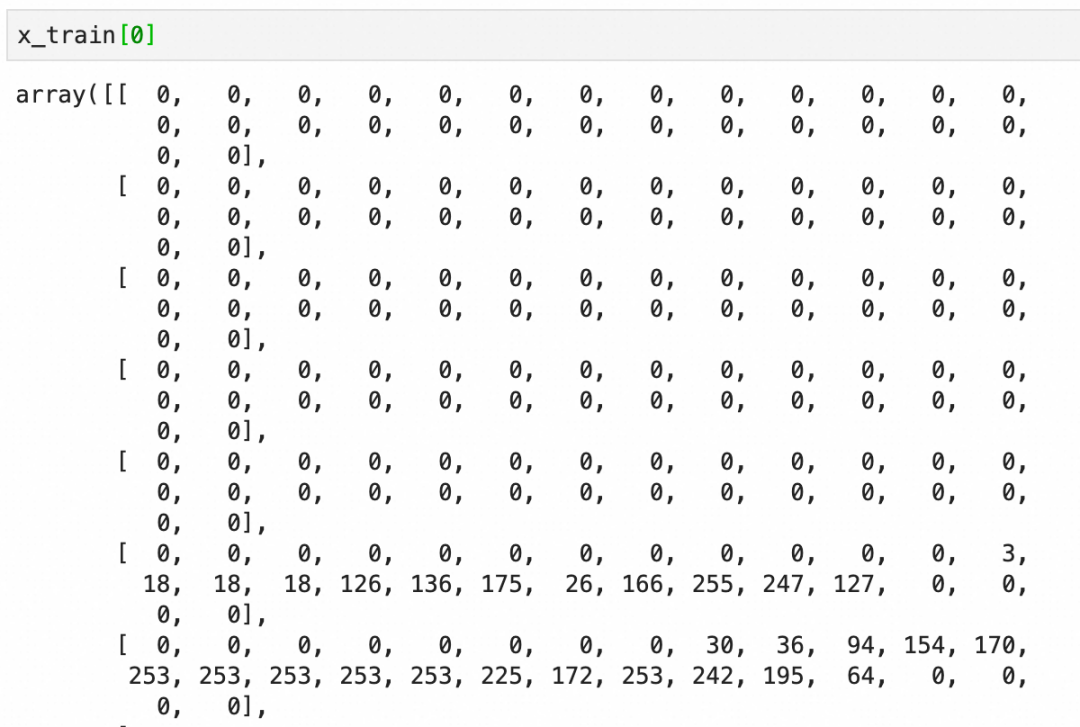
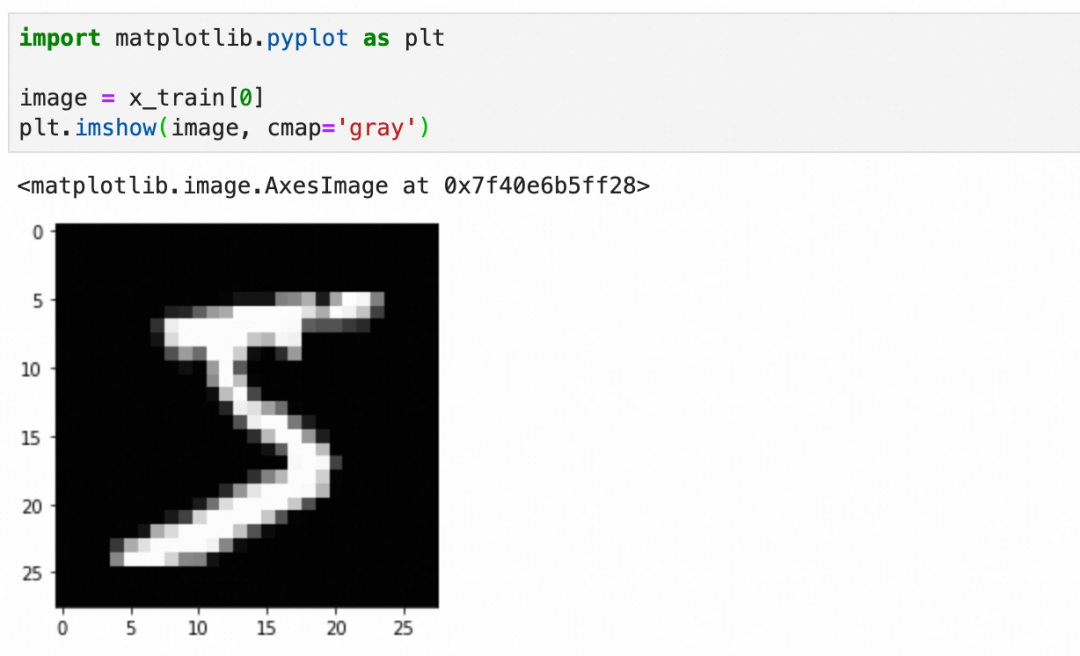
标签y_train
比较简单,就是图像对应的数字:
预加工数据集
在将数据输入到神经网络之前,通常需要进行一些预处理。这包括将图像数据展平为一维向量,标准化像素值到0-1之间,并将标签转换为适合分类任务的格式,通常是one-hot编码。
展平:
标准化:
one-hot编码:
虽然这里的标签是0-9的连续整数,但不要把问题看作是一个数值问题(考虑一下这种情况,假设我们不是在识别手写0-9的图片,而是在识别各种动物的图片)。
这里本质上是在处理分类,分类问题的输出在深度学习框架里适合用one-hot编码来表达(一个一维向量,长度为总分类数,所属分类的值为1,其它值为0)。
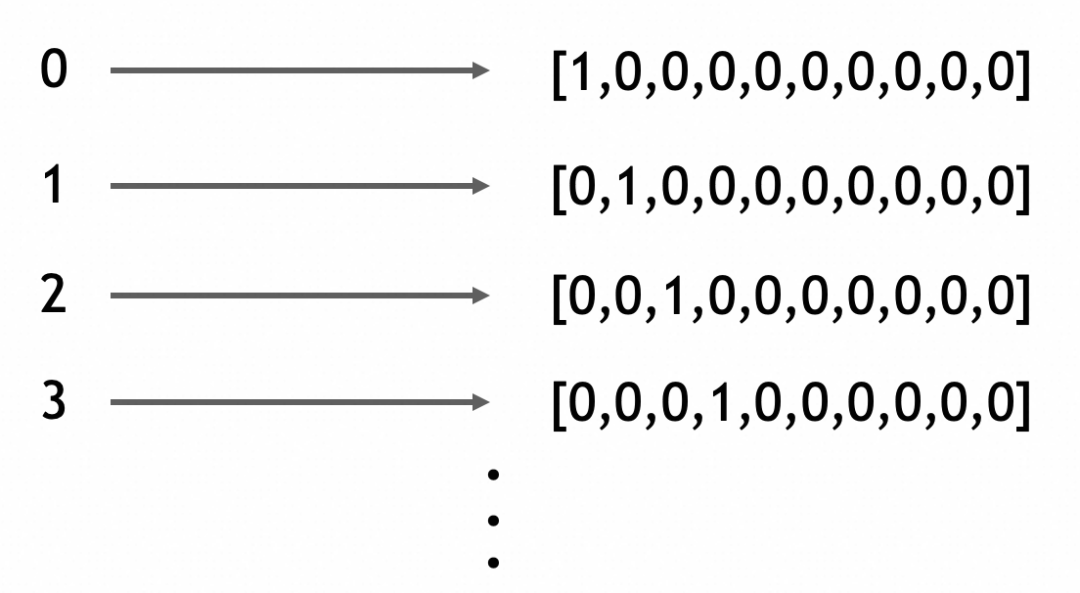
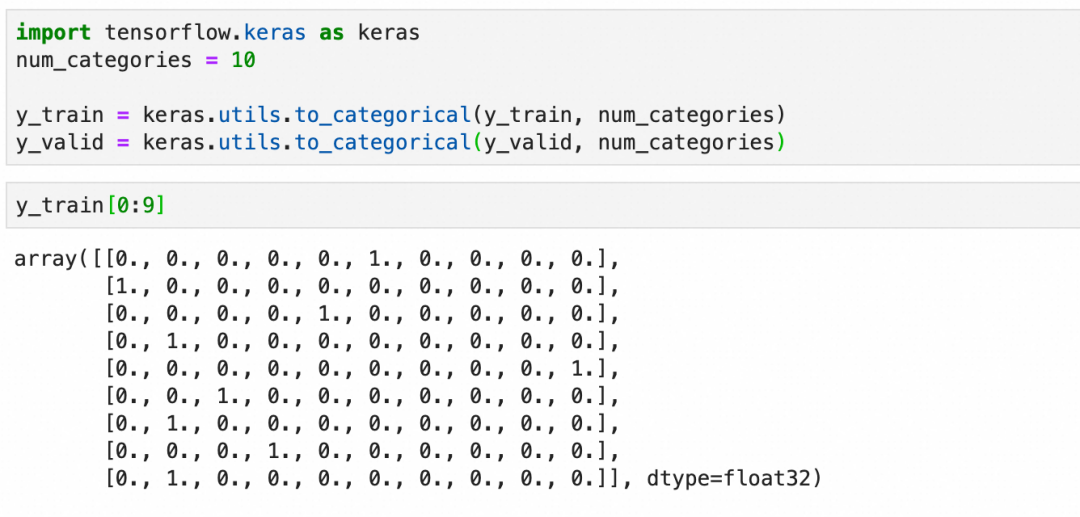
创建模型
创建一个有效的模型通常需要一定的探索或者经验。对于MNIST数据集,一个常见的起点是构建一个包含以下层的网络:
784个输入(对应于28x28像素的输入图像)。
第一层是输入层,有512个神经元,激活函数是ReLU。
第二层隐藏层,使用512个神经元和ReLU激活函数。
第三层是输出层,10个神经元(对应于10个数字类别),使用Softmax激活函数以输出概率分布。
激活函数ReLU上面已经介绍过了,这里的Softmax需要介绍一下:Softmax函数确保输出层的输出值总和为1,从而可以被解释为概率分布。这对于多类分类问题非常有用。例如,输出层的10个输出值为[0.9, 0.0, 0.1, 0.0, ..., 0.0]可以解释为90%概率属于第1类,10%概率属于第3类。
按此创建模型并查看摘要:
-
编译模型
模型在编译时需要指定损失函数和优化器。对于多分类问题,损失函数通常选择交叉熵(Categorical Crossentropy),它的特征可以看参考公式:当实际属于某类(y_actual
=1)时,损失等于log(y_hat)
,否则损失等于log(1-y_hat)
。这个损失函数会惩罚错误猜测,使其损失接近∞,可以有效地量化预测概率分布与实际分布之间的差距。优化器则负责调整网络参数以最小化损失函数。

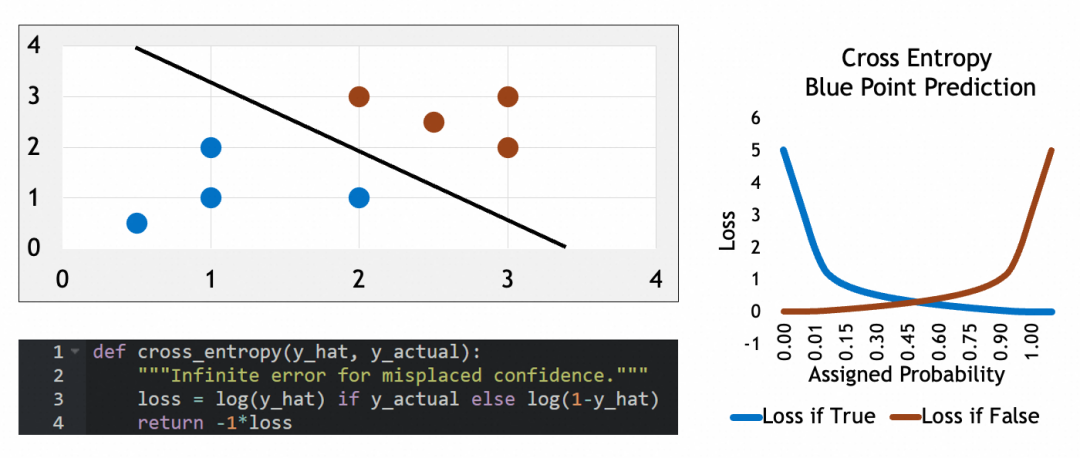
训练并观察准确率
训练模型时,我们会在多个训练周期内迭代更新参数,每个训练周期会经历一次完整的前向传递计算输出、损失函数评估结果和后向传递更新参数的过程。我们观察训练集和验证集上的准确率,以评估模型的表现和泛化能力。
注意损失曲面、梯度下降等概念和原理,如果不清楚可以回顾一下【深度学习过程】。
下图中的accuracy
是训练集的准确率,val_accuracy
是验证集的准确率,准确率符合预期。
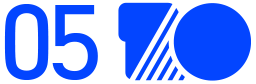
如果模型在训练集上准确率很高,但在验证集上准确率低,可能出现了过拟合现象(overfitting)。过拟合意味着模型过于复杂或训练过度,以至于学习了训练数据中的噪声而非潜在规律。
训练集,左边损失非常小:
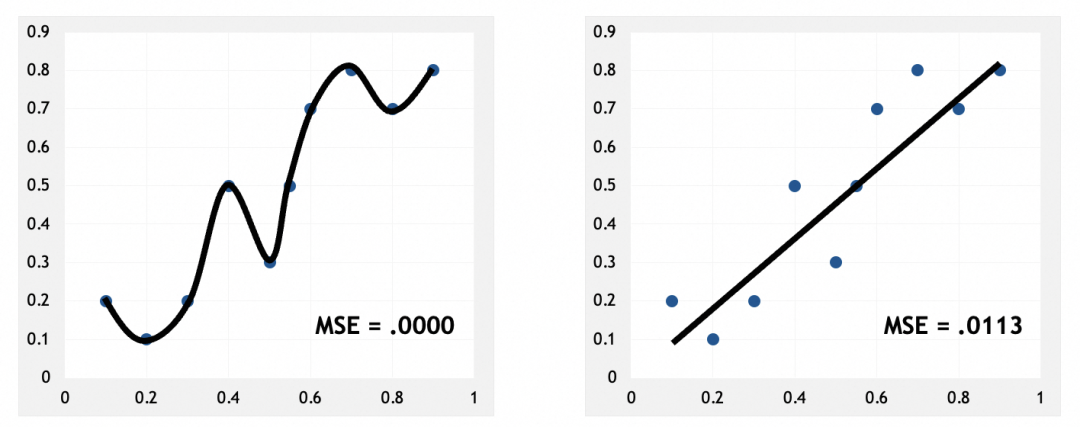
验证集,左边损失反而更大:
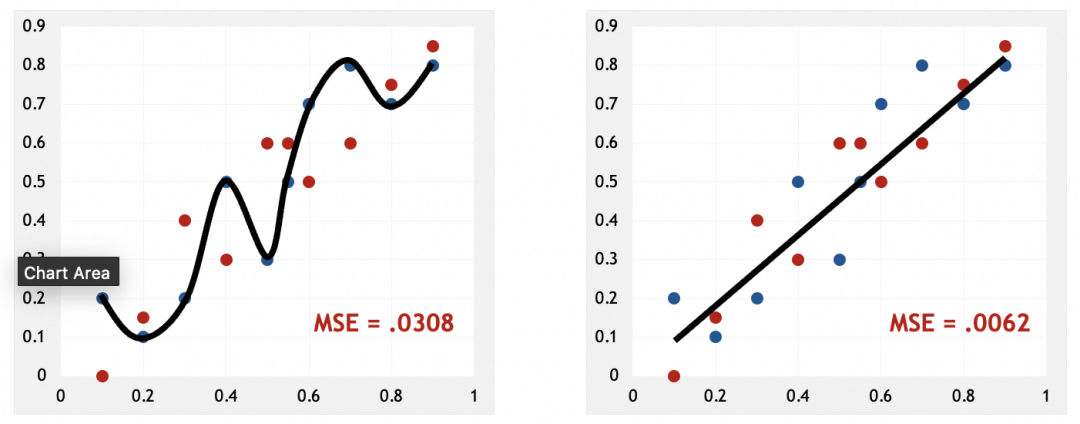
出现过拟合说明什么
左图可以看到,模型几乎硬套了训练集。和人脑类似,硬套部分实例,说明模型只是在记忆这些实例,只有找出它们的规律、特征、模式,才是真正的抽象。这也应证了我前面的说法,神经网络是在通过抽象解决问题。
为什么会出现过拟合
从被训练数据来看,过拟合说明训练集可能特征不明显,不容易抽象。比如被识别的图像模糊、亮度不高。
从模型来看,过拟合通常由于模型过于复杂或训练时间过长造成,走向了死记硬背。
如何解决过拟合
AI技术经过多年的发展,现在已经有比较成熟的方案来解决过拟合了,比如卷积神经网络、递归神经网络,这些我会在后面的文章里进行介绍。
本文大部分素材来自Nvidia在线课程Getting Started with Deep Learning。
(Getting Started with Deep Learning地址:https://learn.nvidia.com/courses/course-detail?course_id=course-v1:DLI+S-FX-01+V1)
团队介绍
天猫国际是中国领先的进口电商平台, 也是阿里巴巴-淘天集团电商技术体系中链路最完整且最为复杂的技术产品之一,也是淘天集团拥有最完整业务形态(平台+直营、跨境、大贸、免税等多业务模式)的业务。在这里我们参与到阿里电商体系的绝大部分核心系统(导购、商家、商品、交易、营销、履约等),同时借助区块链、大数据、AI算法等前沿技术助力业务高速增长。作为贴近业务前沿的技术团队,我们对于电商行业特性、跨境市场研究、未来交易趋势以及未来技术布局等都有着深度的理解。
本文分享自微信公众号 - 大淘宝技术(AlibabaMTT)。
如有侵权,请联系 [email protected] 删除。
本文参与“OSC源创计划”,欢迎正在阅读的你也加入,一起分享。