The era of AI empowering everything has arrived. On May 28th, GOTC 2023 "
AI
is Everywhere
Special Forum" will be held in Zhangjiang Science Hall in Shanghai, with Yang Xuan, vice president of Asia Pacific of the Linux Foundation, as the producer.
The Global Open-source Technology Conference (GOTC for short) is jointly sponsored by Open Atom Open Source Foundation, Linux Foundation Asia Pacific, Shanghai Pudong Software Park and Open Source China, a grand open source technology conference for global developers feast.
GOTC 2023 will last for two days. The conference will be presented in the form of industry exhibitions, keynote speeches, special forums, and sub-forums. Participants will discuss popular technical topics such as metaverse, 3D and games, eBPF, Web3.0, and blockchain. And hot topics such as open source community, AIGC, automotive software, AI programming, open source education and training, cloud native, etc., to discuss the future of open source and help open source development.
AI has changed the world, and the GOTC 2023
registration channel is now open
:
https://www.bagevent.com/event/8387611
,
come on, let’s walk into
the AI
is Everywhere
special forum to discuss the future of open source AI.
Conference highlights:
-
AI and data, the future of databases
-
Training framework, inference model, algorithm mass production
-
Detailed explanation of PyTorch 2.0 architecture
-
Large model and multi-modal technology, AI application practice
Producer: Yang Xuan
He is currently the vice president of the Asia Pacific region of the Linux Foundation. He has more than 20 years of experience in the software industry and has held senior management positions in large international software companies such as Saba, Sumtotal, and Computer Associates. He has rich experience in enterprise-level software application and development, as well as practical experience in software open source and digital transformation.
Topic: Welcome and Introduction
Speech time:
9:00-9:20
Speaker:
Tan Zhongyi | Chairman of LF AI Outreach
Topic: Keynote
Speech time:
9:20-9:40
Speaker:
Ibrahim Haddad | Executive Director of LF AI & Data Foundation, Executive Director of PyTorch Foundation
议题:AI & Data: painpoints and the future.
Speech Time:
9:40-10:00
Speaker:
Du Junping | LF AI & Data Board Chair, ASF Member
Introduction to the topic:
At present, the large language model revolution detonated by ChatGPT is having a profound impact. As one of the most scarce resources in the intelligent age, the importance of data is beyond doubt, and it often becomes the bottleneck of model development and tuning for major enterprises and research institutions. This topic focuses on the data pain points behind the large model and the future-oriented solutions.
Topic: Application of Large-Scale Language Models in Intelligent Document Question Answering: Solutions Based on Langchain and Langchain-serve
Speech Time:
10:00-10:20
Speaker:
Wang Nan | Co-founder and CTO of Jina AI
Introduction to the topic:
The task of a document question answering system is to find answers related to user questions from document data. Due to the increasing number of documents, traditional search can no longer meet people's needs. With the development of deep learning models, document question answering systems have migrated from character matching-based methods to vector representation-based methods. However, they still can only return passages related to the question and cannot give the answer to the question directly, especially for whether or not questions. Recently, the capabilities of large-scale language models have improved, providing a solution to the problem of answer generation for document question answering systems. The new generation of document question answering system integrates traditional models, deep learning question answering models and large-scale language model technologies to provide users with more complete document question answering services. This talk will introduce how to use the Langchain development framework and the Langchain-serve deployment tool to develop an intelligent document question answering system.
Topic: One-stop easy-to-use practice of Shengsi large model
Speech time:
10:20-10:40
Speaker:
He Luwei | Huawei Senior Open Source Engineer
Topic introduction:
Artificial intelligence has developed to this day, and it has gradually moved from a "big refining model" to a "big refining model". Compared with traditional models trained for specific application scenarios, large models have strong generalization capabilities and are no longer limited to a single specific scenario. Therefore, they require a larger and wider amount of data to be "fed" and require stronger computing power. Training requires huge costs, which most developers cannot afford. How to reduce the training and application threshold of large models has become a new problem. In this topic, we will bring the one-stop easy-to-use practice sharing of Shengsi large model, and introduce the one-stop large model platform created by the Shengsi MindSpore community, which integrates model selection, online reasoning, and online training. It supports the online experience and fine-tuning of large models, allowing developers to have zero-distance contact with large-scale model applications such as Zidong · Taichu uses Wen to generate text, Wukong Huahua uses Wen to generate diagrams, and Luojia remote sensing detection.
Topic: Application Practice of AI Database OpenMLDB
Speech time:
10:40-11:00
Speaker:
Chen Dihao | Architect of 4Paradigm
Introduction to the topic:
AI has become an indispensable part of computer infrastructure, and databases optimized for AI scenarios have emerged as the times require. The AI database not only needs to meet the online requirements of feature engineering and machine learning models functionally, but also has higher requirements for offline and online performance. This sharing will take the OpenMLDB project as an example to introduce the application scenarios and performance optimization of AI databases in depth, so as to realize the rapid implementation of specific AI scenarios and the performance improvement of several times or even dozens of times.
Topic: Vector Database: Massive Memory for AIGC
Speech Time:
11:00-11:20
Speaker:
Guo Rentong | Zilliz Partner, Product Director
Introduction to the topic:
In the era of AIGC fire, vector databases are playing an increasingly important role in the processing of massive unstructured data. This sharing will focus on how vector databases can empower AI in the AIGC wave.
Topic: PyTorch 2.0: Bringing compiler technology into the PyTorch kernel
Speech time:
11:20-11:55
Speaker:
Peng Wu | Engineering Manager supporting the PyTorch compiler team
Topic Introduction:
PyTorch 2.0 leverages the compiler for faster training and inference without sacrificing PyTorch's flexibility and ease of use. This talk will provide an overview of the technology stack behind the new torch.compile() API and discuss key features of PyTorch 2.0, including its full backwards compatibility and 43% faster model training. We will introduce various stack components such as TorchDynamo, AOTAutograd, PrimTorch, and TorchInductor, and how they work together to simplify the model development process. Attendees will gain a deeper understanding of the PyTorch 2.0 architecture and the benefits of integrating compiler technology in deep learning frameworks.
Topic: Paddle , an open source deep learning framework, and its open source community
Speech Time:
13:30-14:00
Introduction to the topic:
This report combines the latest development trend of generative AI and Baidu's practice, and introduces the progress of Baidu's deep learning platform + large model core technology research and development, product innovation and ecological construction. At the same time, the report shared thoughts
on
the open source and open platform of Flying Paddle's industrial-level deep learning and the construction of an integrated education ecology under the new trend.
Topic: When federated learning meets large language models
Speech time:
14:00-14:20
Speaker:
Peng Lin | Senior Researcher, VMware CTO Office
Introduction to the topic:
Federated learning enables multiple data sources to collaboratively train a model without sharing data. In recent years, large-scale language models based on transformers have become increasingly popular. However, these models pose challenges due to their high computational resource requirements and complex algorithms. In this talk, we present FATE's recent efforts in applying federated learning to large language models such as GPT-J, ChatGLM-6B, GLM, and LLaMA in financial use cases. FATE combines the distributed training mechanism of federated learning with a large-scale model, allowing computing investment based on the actual data volume of each participant while keeping sensitive data of all parties limited to the local domain. This enables to jointly train large-scale models with mutual benefit. We also explore technical and practical considerations, real-world use cases, and the need for privacy-preserving mechanisms.
Topic: Model Reasoning Optimization, Exploring the Potential of AI Implementation
Speech time:
14:20-14:40
Speaker:
Yuan Liya | ZTE Standards and Open Source Senior Engineer
Introduction to the topic:
The trend of large models has become unstoppable, and how to improve the efficiency of model reasoning has become an urgent problem to be solved. This report will introduce the technical status and trends of model inference optimization, and share the practice of the Adlik project in this field.
Topic: Xtreme1 Next Generation Multimodal Open Source Training Data Platform
Speech time:
14:40-15:00
Speaker:
Wang Jiajun | R&D Director of Beisai Technology
Topic introduction:
UBS Global research report found that AI engineers now spend 70%-90% of their time on training data. Many algorithms have been very good in practice, and data has become a new bottleneck for AI model development. Based on the above status quo, the Beisai Technology team has developed the Xtreme1 training data platform, and is committed to building the most accessible open source Data-Centric MLOps infrastructure to connect people, models and data. Xtreme1 introduces Ontology for the first time to penetrate the problem abstraction of different AI customers. It is the world's first open source tool that supports multi-modal data annotation. It fully follows the principles of cloud-native architecture to ensure the scalability of service performance and the flexibility of deployment scale. , and service resilience under failure conditions.
Topic: Exploration and Practice of OPPO Mobile Graphics Technology——O3DE Mobile WG and shaderNN
Speech Time:
15:00-15:20
Speaker:
Peng Zhouhu | Head of OPPO Open Source Office
Introduction to the topic:
In recent years, with the continuous improvement of mobile terminal computing power and the rapid development of deep learning research, especially the continuous maturity of small network models and the increasing requirements for data security, more and more applications that were originally executed in the cloud Reasoning is transferred to the mobile terminal for implementation. Deep learning reasoning on mobile platforms involves hardware platforms, drivers, compilation optimization, model compression, operator algorithm optimization, and deployment. An efficient reasoning framework suitable for system business development has become an urgent need and development focus of the industry.
Based on the high-efficiency AI reasoning requirements for mobile graphics and image post-processing, in order to reduce the cost of business integration and improve performance, we developed ShaderNN, an efficient reasoning engine based on GPU Shader. It performs efficient inference directly based on GPU textures to save I/O time, does not rely on third-party libraries, supports mainstream deep learning training frameworks across different hardware platforms, and can be customized to facilitate optimization, integration, deployment, and upgrades.
Topic: Intel's PyTorch Journey: AI Computing Power Improvement and Open Source Software Optimization
Speech time:
15:40-16:00
Speaker:
Ma Mingfei | Senior Deep Learning Software Engineer
Introduction to the topic:
PyTorch is one of the most popular frameworks for deep learning and machine learning, and Intel has been a long-term contributor and advocate for the PyTorch community. In this talk, we will share our experience contributing to PyTorch in the core framework and its ecosystem of libraries. We detail our optimizations in torch.compile, the flagship new feature of PyTorch 2.0, and demonstrate its performance benefits on CPU. We will show how to make AI applications more popular through the improvement of hardware computing power and the optimization of open source software, such as diffusion-based generative AI and large language models. We will also introduce some of the PyTorch ecosystem projects we have participated in in the past, such as HuggingFace, DeepSpeed, PyG and many more. Finally, we will discuss future plans and visions to continue working with the PyTorch Foundation to drive deep learning and machine learning in a better direction.
Topic: DeepRec: A High-Performance Deep Learning Framework for Recommendation Scenarios
Speech time:
16:00-16:20
Speaker: Speaker:
Ding Chen | Alibaba Cloud PAI Technical Expert
Introduction to the topic:
DeepRec is an open-source high-performance deep learning framework for recommendation scenarios of the Alibaba Cloud machine learning platform PAI. A series of functions such as elastic features, dynamic elastic dimensions, adaptive EmbeddingVariable, incremental model export and loading, etc. DeepRec is used within the Alibaba Group on Taobao, Tmall, Alimama, AutoNavi, Taot, AliExpress, Lazada, etc., and supports ultra-large-scale sparse training of hundreds of billions of features and trillions of samples in its core business. DeepRec has been open sourced for more than a year, and has been widely used in dozens of companies' search and promotion business scenarios, bringing huge business value.
Topic: Megvii Algorithm Mass Production and MegEngine Ecological Construction
Speech Time:
16:20-16:40
Speaker:
Chen Qiyou | MegEngine Team Leader
Introduction to the topic:
At present, the application of AI technology has been verified in various fields, and it has higher productivity than traditional algorithms. However, with the demand for a large number of AI algorithms, the traditional algorithm generation method for data collection, labeling, model training, verification, and delivery for a specific scenario has become a bottleneck for AI implementation. Around the MegEngine training framework, the MegEngine team proposes a method based on standardized algorithm mass production at each stage to reduce the threshold of AI landing. In order to achieve algorithm mass production, MegEngine has developed a series of components, which together form the MegEngine algorithm mass production The ecology is gradually being open sourced.
Topic: Primus - a general distributed training scheduling framework
Speech time:
16:40-17:00
Speaker:
Xu Hebang | R&D Engineer of ByteDance Infrastructure Computing Framework
Introduction to the topic:
In recent years, machine learning technology has been deeply rooted in various application fields and has successfully brought significant improvements. Facing the ever-increasing training data and model scale, in order to meet the needs of more efficient model training, the concept of distributed training was born accordingly. As a general distributed training scheduling framework, Primus provides a common interface to bridge distributed training tasks and physical computing resources, allowing data scientists to focus on the design of learning algorithms, and allowing distributed training tasks to run on different types of Compute clusters such as Kubernetes and YARN. On this basis, Primus also provides the fault tolerance and data scheduling capabilities required for distributed training tasks, which in turn improves the ease of use of distributed training. This topic will share the current situation and practice of Primus in ByteDance, the challenges related to Primus and distributed training, and the future prospects.
Topic: Transparent Backend Graph Compiler Seamlessly Improves ML Upstream Frameworks
Speech Time:
17:00-17:20
Speaker:
Tiejun Chen | Sr. Technical lead
Topic Introduction:
There is currently an emerging trend: Observability is shifting from the cloud to the edge, where AI workloads are often managed and orchestrated through high-level ML frameworks such as Ray. But at the same time, AI accelerators from various vendors (such as Nvidia GPU series, Intel Movidius VPU, Google TPU, etc.) have achieved AI acceleration. You can actually see many ASIC-based AI accelerators. On the other hand, there are various graph compilers (such as TVM, Intel OpenVINO, TensorRT, etc.) to improve ML performance, but the fragmentation is serious. Therefore, users face challenges in endowing these heterogeneous AI accelerators with different software accelerations in the real world due to the lack of a common unified framework to support them naturally. This speech will share the introduction of transparent backend acceleration technology to automatically improve ML performance on heterogeneous AI accelerators on popular ML upstream frameworks (such as Tensorflow, Pytorch, TorchServe, Tensorflow Serving, etc.), and seamlessly integrate with those mainstream ML graph compilers combined. With our zero-code-change approach to mainstream ML frameworks, users can see improved ML/AI performance on their native AI applications.
Topic: OpenGPT: LMM Multimodal Large Model Reasoning Framework
Speech time:
17:20-17:40
Speaker:
Wang Feng | Jina AI Senior Algorithm Engineer
Introduction to the topic:
Large language models and multimodal technologies have become a trend. The improvement of AI capabilities represented by GPT-4 has changed from achieving monotonous text interaction to accepting images and text as input. More and more multimodal technologies based on large models have emerged, but there are still many challenges in the process of landing actual industrial products. Especially for model reasoning problems, there are more new problems to be solved. This sharing will take the OpenGPT project as an example to introduce Jina AI's practice in solving the problem of large model product landing.
The Global Open-source Technology Conference (GOTC for short) is jointly sponsored by Open Atom Open Source Foundation, Linux Foundation Asia Pacific, Shanghai Pudong Software Park and Open Source China, a grand open source technology conference for global developers feast.
GOTC 2023 will be held in Shanghai Zhangjiang Science Hall from May 27th to 28th. The conference will be presented in the form of industry exhibitions, keynote speeches, special forums, and sub-forums. Participants will discuss popular technical topics such as Metaverse, 3D and games, eBPF, Web3.0, and blockchain, as well as open source communities, AIGC, Hot topics such as automotive software, AI programming, open source education and training, cloud native, etc., discuss the future of open source and help open source development.
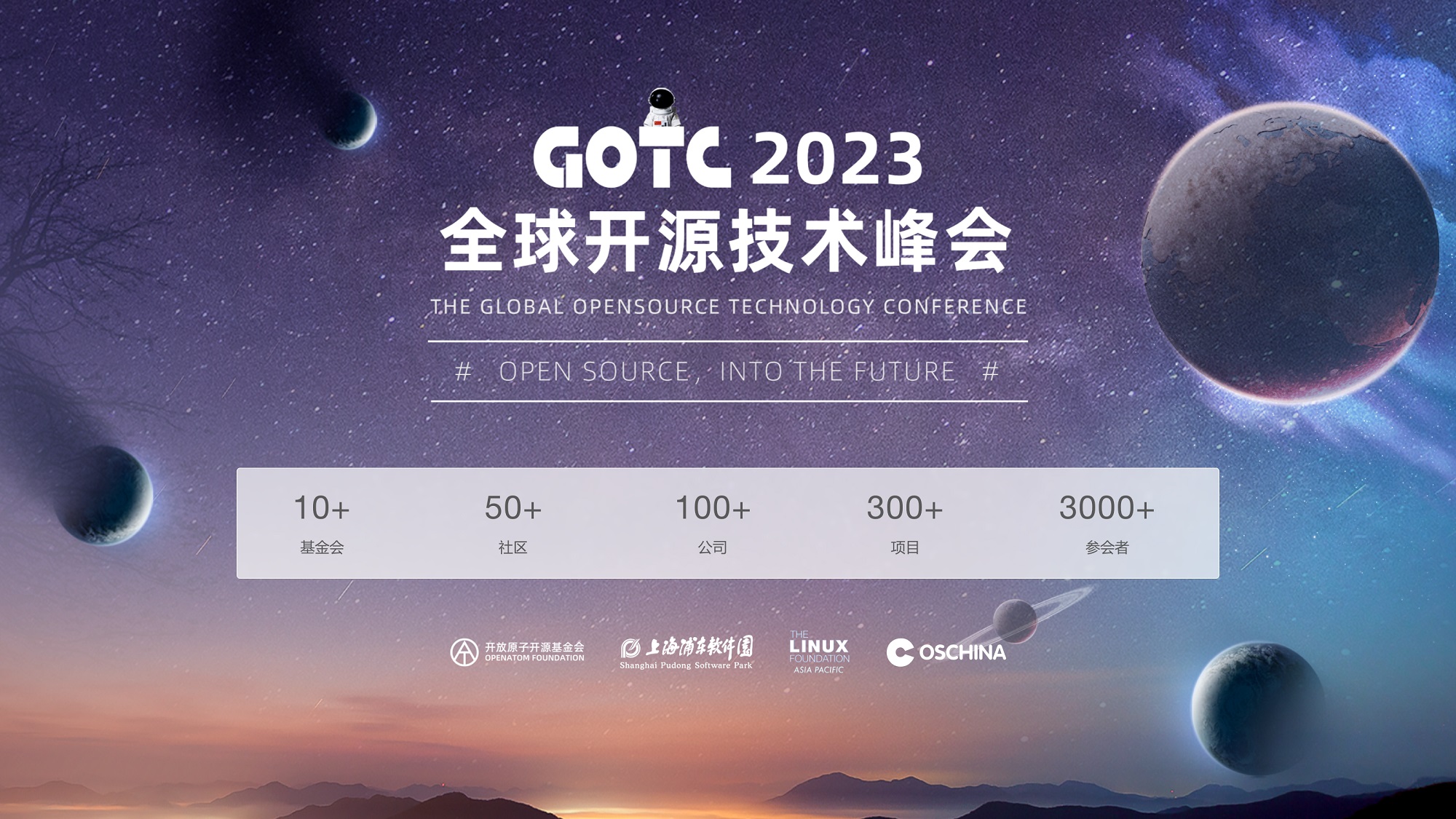
The registration channel for GOTC 2023 is now open, and open source enthusiasts in various technical fields around the world are sincerely invited to join in the grand event!
For conference registration, please visit: https://www.bagevent.com/event/8387611
Enter the official website for more information, please visit: https://gotc.oschina.net