TensorFlow 卷积神经网络系列案例(1):猫狗识别
TensorFlow 系列案例(2):自然语言处理-TensorFlow + Word2Vec https://blog.csdn.net/duan_zhihua/article/details/81257323
使用卷积神经网络进行猫狗识别的步骤:
1. 加载猫狗训练图片数据。
2. 创建卷积神经网络层次。
3. 对猫狗图片进行训练测试,保存模型。
4. 加载训练好的模型,对新的图片进行猫、狗预测。
本节案例代码来源于网上资料,在此致谢网上各位AI大牛的贡献!
- 数据处理:加载猫狗训练图片数据。
数据集来源于Kaggle,这里从数据集中选取整理了1000只猫和1000只狗的图片,图片放在training_data目录下,training_data目录包括cats目录和dogs目录。链接:https://pan.baidu.com/s/124AY6eN2580eTWmryQ1-_A 密码:9uqc
dataset.py代码:先安装OpenCV、Pillow第三方模块。在代码中导入cv2。
import numpy as np
import os
import glob
from sklearn.utils import shuffle
import cv2
def load_train(train_path, img_size, classes):
images = []
labels = []
img_names = []
cls = []
print("读取训练图片...")
#classes传入一个列表:<class 'list'>: ['dogs', 'cats']
for fields in classes:
index = classes.index(fields)
print("Now going to read {} files (Index:{})".format(fields, index))
#路径读入格式:'D:/PycharmProjects/Tensorflow_2018_test/catAndDog/training_data\\dogs\\*g'
path = os.path.join(train_path, fields, '*g')
#file内容:'D:/PycharmProjects/Tensorflow_2018_test/catAndDog/training_data\\dogs\\dog.0.jpg'
files = glob.glob(path)
print(files)
for fl in files:
print(fl)
#img_size 图片的大小(如 64),原始图片可能是大图片,也可能是小图片,以下转换为统一格式。
image = cv2.imread(fl)
#转换为大小:<class 'tuple'>: (64, 64, 3),这里是彩色图片RGB,通道数为3.
image = cv2.resize(image, (img_size, img_size), 0, 0, cv2.INTER_LINEAR)
image = image.astype(np.float32)
# 归一化处理,将数据乘以 1/255,转换为(0,1)之间的范围。
image = np.multiply(image, 1.0 / 255.0)
images.append(image)
label = np.zeros(len(classes))
# 猫狗二分类打标签,如[1. 0.]
label[index] = 1.0
labels.append(label)
flbase = os.path.basename(fl)
img_names.append(flbase)
cls.append(fields)
images = np.array(images)
labels = np.array(labels)
img_names = np.array(img_names)
cls = np.array(cls)
return images, labels, img_names, cls
class DataSet(object):
def __init__(self, images, labels, img_names, cls):
self._num_examples = images.shape[0]
self._images = images
self._labels = labels
self._img_names = img_names
self._cls = cls
self._epochs_done = 0
self._index_in_epoch = 0
def images(self):
return self._images
def labels(self):
return self._labels
def img_names(self):
return self._img_names
def cls(self):
return self._cls
def num_examples(self):
return self._num_examples
def epochs_done(self):
return self._epochs_done
def next_batch(self, batch_size):
start = self._index_in_epoch
self._index_in_epoch += batch_size
if self._index_in_epoch > self._num_examples:
self._epochs_done += 1
start = 0
self._index_in_epoch = batch_size
assert batch_size <= self._num_examples
end = self._index_in_epoch
return self._images[start:end], self._labels[start:end], self._img_names[start:end], self._cls[start:end]
def read_train_sets(train_path, image_size, classes, validation_size):
class DataSets(object):
pass
data_sets = DataSets()
images, labels, img_names, cls = load_train(train_path, image_size, classes)
#调用sklearn.utils的 shuffle方法,打散猫狗图片数据。
images, labels, img_names, cls = shuffle(images, labels, img_names, cls)
# 这里读入了2002张猫狗图片,validation_size等于0.2,因此验证集validation_size 为400个
#images:<class 'tuple'>: (2002, 64, 64, 3)
if isinstance(validation_size, float):
validation_size = int(validation_size * images.shape[0])
validation_images = images[:validation_size]
validation_labels = labels[:validation_size]
validation_img_names = img_names[:validation_size]
validation_cls = cls[:validation_size]
train_images = images[validation_size:]
train_labels = labels[validation_size:]
train_img_names = img_names[validation_size:]
train_cls = cls[validation_size:]
data_sets.train = DataSet(train_images, train_labels, train_img_names, train_cls)
data_sets.valid = DataSet(validation_images, validation_labels, validation_img_names, validation_cls)
return data_sets
- 创建卷积神经网络层次。卷积神经网络(CNN)由输入层、卷积层(激活函数)、池化层、全连接层(神经网络的隐藏层)组成,即INPUT(输入层)-CONV(卷积层 -RELU激活函数)-POOL(池化层)-FC(全连接层)。在每一层转换中需清楚shape的转换,以下是卷积神经网络输出size大小的计算:
卷积计算输出的大小:
out_height =((input_height - filter_height + padding_top + padding_bottom) /stride_height) +1
out_width =((input_width - filter_width + padding_left + padding_right) /stride_width) +1
但我们通常都使用方阵:
out_height = out_width
input_height = input_width
filter_height filter_width
padding_top =padding_bottom=padding_left= padding_right
stride_height=stride_width
1)因此,如果padding 不等于“SAME”,
输入图片大小:W*W
filter内核大小:F*F
步长 S
padding像素数P
卷积计算输出的大小简化为:N= (W-F+2P)/S +1
2)如果padding =“SAME”:
卷积计算公式输出大小简化为:W/S 向上取整。
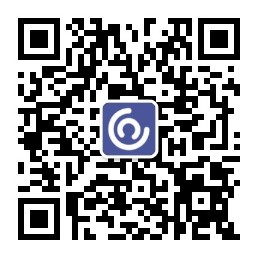
计算例子:
① padding = "value", stride = 4, (227 - 11 + 2*0)/ 4 + 1 = 55
② padding = "value", stride = 2, (55 - 3 + 2*0)/ 2 + 1 = 27
③ padding = "same", stride = 1, 27 / 1 = 27
④ padding = "value",stride = 2, (27 - 3 + 2*0) / 2 + 1 = 13
本案例中的计算示例:layer_conv1 的卷积计算,从输入层到卷积层。
layer_conv1 = create_convolution_layer(input=x,
num_input_channels=num_channels,
conv_filter_size=filter_size_conv1,
num_filters=num_filters_conv1)
def create_convolution_layer(input,
num_input_channels,
conv_filter_size,
num_filters):
weights = create_weights(shape=[conv_filter_size, conv_filter_size, num_input_channels, num_filters])
biases = create_biases(num_filters)
layer = tf.nn.conv2d(input=input, filter=weights, strides=[1, 1, 1, 1], padding='SAME')
layer += biases
layer = tf.nn.relu(layer)
layer = tf.nn.max_pool(value=layer, ksize=[1, 2, 2, 1], strides=[1, 2, 2, 1], padding='SAME')
return layer
layer_conv1 的卷积计算,从输入层到卷积层。
在代码layer = tf.nn.conv2d(input=input, filter=weights, strides=[1, 1, 1, 1], padding='SAME')
tf.nn.conv2d方法的输入tensor的shape形状: `[batch, in_height, in_width, in_channels]`
tf.nn.conv2d方法的filter内核tensor 的shape形状:`[filter_height, filter_width, in_channels, out_channels]`
其中:
input=x 的shape:batchsize 64 64 3
weights内核的shape: 3 3 3 32 这里的第三个参数3是 num_input_channels,需等于输入层的通道数3
stride步长: 1 1 1 1,这里的strides[0] = strides[3] 需等于1
卷积计算输出大小W/S=64/1=64 ,其shape为: batchsize 64 64 32
这里卷积层的池化计算中:
layer = tf.nn.max_pool(value=layer, ksize=[1, 2, 2, 1], strides=[1, 2, 2, 1], padding='SAME')
输入为layer,其shape为: batchsize 64 64 32
输出大小为W/S=64/2=32,其shape为:batchsize 32 32 32
其他的卷积层和池化层的大小计算依此类推。
数据训练的整个代码train.py:
import dataset
import tensorflow as tf
import numpy as np
from numpy.random import seed
seed(10)
from tensorflow import set_random_seed
set_random_seed(20)
batch_size = 32
classes = ['dogs', 'cats']
num_classes = len(classes)
validation_size = 0.2
img_size = 64
num_channels = 3
train_path = "D:/PycharmProjects/Tensorflow_2018_test/catAndDog/training_data"
data = dataset.read_train_sets(train_path, img_size, classes, validation_size)
session = tf.Session()
x = tf.placeholder(tf.float32, shape=[None, img_size, img_size, num_channels], name='x')
y_true = tf.placeholder(tf.float32, shape=[None, num_classes], name='y_true')
y_true_cls = tf.argmax(y_true, dimension=1)
filter_size_conv1 = 3
num_filters_conv1 = 32
filter_size_conv2 = 3
num_filters_conv2 = 32
filter_size_conv3 = 3
num_filters_conv3 = 64
# 全连接层的输出
fc_layer_size = 1024
def create_weights(shape):
return tf.Variable(tf.truncated_normal(shape, stddev=0.05))
def create_biases(size):
return tf.Variable(tf.constant(0.05, shape=[size]))
def create_convolution_layer(input,
num_input_channels,
conv_filter_size,
num_filters):
weights = create_weights(shape=[conv_filter_size, conv_filter_size, num_input_channels, num_filters])
biases = create_biases(num_filters)
layer = tf.nn.conv2d(input=input, filter=weights, strides=[1, 1, 1, 1], padding='SAME')
layer += biases
layer = tf.nn.relu(layer)
layer = tf.nn.max_pool(value=layer, ksize=[1, 2, 2, 1], strides=[1, 2, 2, 1], padding='SAME')
return layer
def create_flatten_layer(layer):
layer_shape = layer.get_shape()
num_features = layer_shape[1:4].num_elements()
layer = tf.reshape(layer, [-1, num_features])
return layer
def create_fc_layer(input,
num_inputs,
num_outputs,
use_relu=True):
weights = create_weights(shape=[num_inputs, num_outputs])
biases = create_biases(num_outputs)
layer = tf.matmul(input, weights) + biases
layer = tf.nn.dropout(layer, keep_prob=0.7)
if use_relu:
layer = tf.nn.relu(layer)
return layer
layer_conv1 = create_convolution_layer(input=x,
num_input_channels=num_channels,
conv_filter_size=filter_size_conv1,
num_filters=num_filters_conv1)
layer_conv2 = create_convolution_layer(input=layer_conv1,
num_input_channels=num_filters_conv1,
conv_filter_size=filter_size_conv2,
num_filters=num_filters_conv2)
layer_conv3 = create_convolution_layer(input=layer_conv2,
num_input_channels=num_filters_conv2,
conv_filter_size=filter_size_conv3,
num_filters=num_filters_conv3)
layer_flat = create_flatten_layer(layer_conv3)
layer_fc1 = create_fc_layer(input=layer_flat,
num_inputs=layer_flat.get_shape()[1:4].num_elements(),
num_outputs=fc_layer_size,
use_relu=True)
layer_fc2 = create_fc_layer(input=layer_fc1,
num_inputs=fc_layer_size,
num_outputs=num_classes,
use_relu=False)
y_pred = tf.nn.softmax(layer_fc2, name='y_pred')
y_pred_cls = tf.argmax(y_pred, dimension=1)
session.run(tf.global_variables_initializer())
cross_entropy = tf.nn.softmax_cross_entropy_with_logits(logits=layer_fc2, labels=y_true)
cost = tf.reduce_mean(cross_entropy)
optimizer = tf.train.AdamOptimizer(learning_rate=1e-4).minimize(cost)
correct_prediction = tf.equal(y_pred_cls, y_true_cls)
accuracy = tf.reduce_mean(tf.cast(correct_prediction, tf.float32))
session.run(tf.global_variables_initializer())
def show_progress(epoch, feed_dict_train, feed_dict_validate, val_loss, i):
acc = session.run(accuracy, feed_dict=feed_dict_train)
val_acc = session.run(accuracy, feed_dict=feed_dict_validate)
print("epoch:", str(epoch + 1) + ",i:", str(i) +
",acc:", str(acc) + ",val_acc:", str(val_acc) + ",val_loss:", str(val_loss))
total_iterations = 0
saver = tf.train.Saver()
def train(num_iteration):
global total_iterations
for i in range(total_iterations, total_iterations + num_iteration):
x_batch, y_true_batch, _, cls_batch = data.train.next_batch(batch_size)
x_valid_batch, y_valid_batch, _, valid_cls_batch = data.valid.next_batch(batch_size)
feed_dict_tr = {x: x_batch, y_true: y_true_batch}
feed_dict_val = {x: x_valid_batch, y_true: y_valid_batch}
session.run(optimizer, feed_dict=feed_dict_tr)
examples = data.train.num_examples()
if i % int(examples / batch_size) == 0:
val_loss = session.run(cost, feed_dict=feed_dict_val)
epoch = int(i / int(examples / batch_size))
show_progress(epoch, feed_dict_tr, feed_dict_val, val_loss, i)
saver.save(session, './dogs-cats-model/dog-cat.ckpt', global_step=i)
total_iterations += num_iteration
train(num_iteration=100)
train(num_iteration=5000) 运行结果如下:
......
D:/PycharmProjects/Tensorflow_2018_test/catAndDog/training_data\cats\cat.998.jpg
D:/PycharmProjects/Tensorflow_2018_test/catAndDog/training_data\cats\cat.999.jpg
2018-07-22 19:47:35.412691: W c:\l\tensorflow_1501918863922\work\tensorflow-1.2.1\tensorflow\core\platform\cpu_feature_guard.cc:45] The TensorFlow library wasn't compiled to use SSE instructions, but these are available on your machine and could speed up CPU computations.
2018-07-22 19:47:35.413146: W c:\l\tensorflow_1501918863922\work\tensorflow-1.2.1\tensorflow\core\platform\cpu_feature_guard.cc:45] The TensorFlow library wasn't compiled to use SSE2 instructions, but these are available on your machine and could speed up CPU computations.
2018-07-22 19:47:35.413607: W c:\l\tensorflow_1501918863922\work\tensorflow-1.2.1\tensorflow\core\platform\cpu_feature_guard.cc:45] The TensorFlow library wasn't compiled to use SSE3 instructions, but these are available on your machine and could speed up CPU computations.
2018-07-22 19:47:35.414036: W c:\l\tensorflow_1501918863922\work\tensorflow-1.2.1\tensorflow\core\platform\cpu_feature_guard.cc:45] The TensorFlow library wasn't compiled to use SSE4.1 instructions, but these are available on your machine and could speed up CPU computations.
2018-07-22 19:47:35.414523: W c:\l\tensorflow_1501918863922\work\tensorflow-1.2.1\tensorflow\core\platform\cpu_feature_guard.cc:45] The TensorFlow library wasn't compiled to use SSE4.2 instructions, but these are available on your machine and could speed up CPU computations.
2018-07-22 19:47:35.416029: W c:\l\tensorflow_1501918863922\work\tensorflow-1.2.1\tensorflow\core\platform\cpu_feature_guard.cc:45] The TensorFlow library wasn't compiled to use AVX instructions, but these are available on your machine and could speed up CPU computations.
2018-07-22 19:47:35.416710: W c:\l\tensorflow_1501918863922\work\tensorflow-1.2.1\tensorflow\core\platform\cpu_feature_guard.cc:45] The TensorFlow library wasn't compiled to use AVX2 instructions, but these are available on your machine and could speed up CPU computations.
2018-07-22 19:47:35.417239: W c:\l\tensorflow_1501918863922\work\tensorflow-1.2.1\tensorflow\core\platform\cpu_feature_guard.cc:45] The TensorFlow library wasn't compiled to use FMA instructions, but these are available on your machine and could speed up CPU computations.
epoch: 1,i: 0,acc: 0.625,val_acc: 0.4375,val_loss: 0.7436454
epoch: 2,i: 50,acc: 0.65625,val_acc: 0.40625,val_loss: 0.7284967
epoch: 3,i: 100,acc: 0.5625,val_acc: 0.40625,val_loss: 0.7191855
epoch: 4,i: 150,acc: 0.5625,val_acc: 0.5,val_loss: 0.7015336
epoch: 5,i: 200,acc: 0.625,val_acc: 0.59375,val_loss: 0.6757284
epoch: 6,i: 250,acc: 0.5625,val_acc: 0.5625,val_loss: 0.7258785
epoch: 7,i: 300,acc: 0.6875,val_acc: 0.5625,val_loss: 0.6614233
epoch: 8,i: 350,acc: 0.59375,val_acc: 0.59375,val_loss: 0.605597
epoch: 9,i: 400,acc: 0.625,val_acc: 0.53125,val_loss: 0.67095697
epoch: 10,i: 450,acc: 0.625,val_acc: 0.5,val_loss: 0.66302484
epoch: 11,i: 500,acc: 0.65625,val_acc: 0.53125,val_loss: 0.66088706
epoch: 12,i: 550,acc: 0.6875,val_acc: 0.59375,val_loss: 0.5763863
epoch: 13,i: 600,acc: 0.625,val_acc: 0.625,val_loss: 0.68232787
epoch: 14,i: 650,acc: 0.8125,val_acc: 0.78125,val_loss: 0.5594511
epoch: 15,i: 700,acc: 0.71875,val_acc: 0.75,val_loss: 0.6684464
epoch: 16,i: 750,acc: 0.75,val_acc: 0.59375,val_loss: 0.64405406
epoch: 17,i: 800,acc: 0.75,val_acc: 0.53125,val_loss: 0.63719827
epoch: 18,i: 850,acc: 0.71875,val_acc: 0.6875,val_loss: 0.5434316
epoch: 19,i: 900,acc: 0.78125,val_acc: 0.65625,val_loss: 0.7148928
epoch: 20,i: 950,acc: 0.75,val_acc: 0.78125,val_loss: 0.57082707
epoch: 21,i: 1000,acc: 0.8125,val_acc: 0.65625,val_loss: 0.63966876
epoch: 22,i: 1050,acc: 0.75,val_acc: 0.75,val_loss: 0.5933325
epoch: 23,i: 1100,acc: 0.75,val_acc: 0.625,val_loss: 0.6802487
epoch: 24,i: 1150,acc: 0.75,val_acc: 0.625,val_loss: 0.6392723
epoch: 25,i: 1200,acc: 0.78125,val_acc: 0.625,val_loss: 0.6674799
epoch: 26,i: 1250,acc: 0.78125,val_acc: 0.75,val_loss: 0.59081894
epoch: 27,i: 1300,acc: 0.71875,val_acc: 0.5,val_loss: 0.67688525
epoch: 28,i: 1350,acc: 0.8125,val_acc: 0.59375,val_loss: 0.6042088
epoch: 29,i: 1400,acc: 0.78125,val_acc: 0.53125,val_loss: 0.70344555
epoch: 30,i: 1450,acc: 0.75,val_acc: 0.75,val_loss: 0.5876309
epoch: 31,i: 1500,acc: 0.875,val_acc: 0.71875,val_loss: 0.6333902
......
运行结果将训练好的模型进行持久化。
- 加载训练好的模型,对新的图片进行猫、狗预测。这里仍使用training_data目录的数据来进行图片预测。
predict.py
import glob
import tensorflow as tf
import numpy as np
import os, cv2
image_size = 64
num_channels = 3
images = []
path = "D:/PycharmProjects/Tensorflow_2018_test/catAndDog/training_data"
direct = os.listdir(path)
for file in direct:
path = os.path.join(path, file, '*g')
files = glob.glob(path )
print(files)
for fl in files:
print(fl)
image = cv2.imread(fl)
image = cv2.resize(image, (image_size, image_size), 0, 0, cv2.INTER_LINEAR)
images.append(image)
images = np.array(images, dtype=np.uint8)
images = images.astype('float32')
images = np.multiply(images, 1.0 / 255.0)
for img in images:
x_batch = img.reshape(1, image_size, image_size, num_channels)
sess = tf.Session()
# step1网络结构图
saver = tf.train.import_meta_graph('./dogs-cats-model/dog-cat.ckpt-3050.meta')
# step2加载权重参数
saver.restore(sess, './dogs-cats-model/dog-cat.ckpt-3050')
# 获取默认的图
graph = tf.get_default_graph()
y_pred = graph.get_tensor_by_name("y_pred:0")
x = graph.get_tensor_by_name("x:0")
y_true = graph.get_tensor_by_name("y_true:0")
y_test_images = np.zeros((1, 2))
feed_dict_testing = {x: x_batch, y_true: y_test_images}
result = sess.run(y_pred, feed_dict_testing)
res_label = ['dog', 'cat']
print(res_label[result.argmax()])
预测结果如下:
......
2018-07-22 20:15:19.835048: W c:\l\tensorflow_1501918863922\work\tensorflow-1.2.1\tensorflow\core\platform\cpu_feature_guard.cc:45] The TensorFlow library wasn't compiled to use SSE instructions, but these are available on your machine and could speed up CPU computations.
2018-07-22 20:15:19.835668: W c:\l\tensorflow_1501918863922\work\tensorflow-1.2.1\tensorflow\core\platform\cpu_feature_guard.cc:45] The TensorFlow library wasn't compiled to use SSE2 instructions, but these are available on your machine and could speed up CPU computations.
2018-07-22 20:15:19.836264: W c:\l\tensorflow_1501918863922\work\tensorflow-1.2.1\tensorflow\core\platform\cpu_feature_guard.cc:45] The TensorFlow library wasn't compiled to use SSE3 instructions, but these are available on your machine and could speed up CPU computations.
2018-07-22 20:15:19.836836: W c:\l\tensorflow_1501918863922\work\tensorflow-1.2.1\tensorflow\core\platform\cpu_feature_guard.cc:45] The TensorFlow library wasn't compiled to use SSE4.1 instructions, but these are available on your machine and could speed up CPU computations.
2018-07-22 20:15:19.837650: W c:\l\tensorflow_1501918863922\work\tensorflow-1.2.1\tensorflow\core\platform\cpu_feature_guard.cc:45] The TensorFlow library wasn't compiled to use SSE4.2 instructions, but these are available on your machine and could speed up CPU computations.
2018-07-22 20:15:19.838874: W c:\l\tensorflow_1501918863922\work\tensorflow-1.2.1\tensorflow\core\platform\cpu_feature_guard.cc:45] The TensorFlow library wasn't compiled to use AVX instructions, but these are available on your machine and could speed up CPU computations.
2018-07-22 20:15:19.839484: W c:\l\tensorflow_1501918863922\work\tensorflow-1.2.1\tensorflow\core\platform\cpu_feature_guard.cc:45] The TensorFlow library wasn't compiled to use AVX2 instructions, but these are available on your machine and could speed up CPU computations.
2018-07-22 20:15:19.906125: W c:\l\tensorflow_1501918863922\work\tensorflow-1.2.1\tensorflow\core\platform\cpu_feature_guard.cc:45] The TensorFlow library wasn't compiled to use FMA instructions, but these are available on your machine and could speed up CPU computations.
cat
cat
cat
......
“我设想在未来,我们可能就相当于机器人的宠物狗狗,到那时我也会支持机器人的。” ——克劳德·香农
“全面化人工智能可能意味着人类的终结...”机器可以自行启动,并且自动对自身进行重新设计,速率也会越来越快。受到漫长的生物进化历程的限制,人类无法与之竞争,终将被取代。“ ——史蒂芬·霍金