深度学习有三个瓶颈
-
算力:前几篇文章或多或少提了些,也比较容易理解,后续会写更细
-
算法:在深度学习从入门到不想放弃的专栏里会一直写
-
数据: 今天写点
前几天DeepMind发表了一篇文章,发现如果把CNN给做成和Trasformer一样大的结构,那得到的训练结果实际上差不太多,其实很早就有论点说深度学习里面会认为数据和算力才配是决定性因素,我对这个观点不置可否(至少LSTM因为串行化,再怎么弄也很难做的和transfomer,CNN一样有效率),但是如果换个角度说算法决定神经网络限,算力和数据决定神经网络上限(有些场景反而得倒过来说),那我是双手赞成的。
先看一篇论文:
Training_language_models_to_follow_instructions_with_human_feedback.pdf (openai.com)
这篇论文是Openai阐述其RLHF的整个流程,相信论文里的图大家可能或多或少在一些PPT里都看过一点,比如下图:
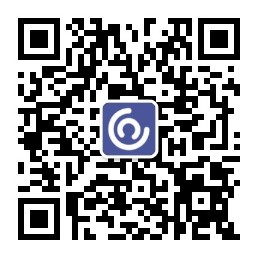
但是这文章如果细看你会发现很多有用的东西,包括彩蛋,建议有条件的详细读一下全文,其实RLHF这东西最早是Openai联合Deepmind在2017年就提出来了,想法很有用也很朴实,就是人类去标注再重新强化学习,但是为什么从2017年到2023年这6年里,只有Openai在ChatGPT上做出来了,难道别人不理解这论文吗?
不是的,我们来看一下论文里提到的非常意思的一段:
To produce our demonstration and comparison data, and to conduct our main evaluations, we hired a team of about 40 contractors on Upwork and through ScaleAI. Compared to earlier work that collects human preference data on the task of summarization (Ziegler et al., 2019; Stiennon et al., 2020; Wu et al., 2021), our inputs span a much broader range of tasks, and can occasionally include controversial and sensitive topics. Our aim was to select a group of labelers who were sensitive to the preferences of different demographic groups, and who were good at identifying outputs that were potentially harmful. Thus, we conducted a screening test designed to measure labeler performance on these axes. We selected labelers who performed well on this test; for more information about our selection procedure and labeler demographics, see Appendix B.1.
是的,大家没看错,OpenAI为了做RLHF和PPO,光雇佣Contractors公司来做数据标注和alignment就雇佣了40个公司来做,想象一下那是多少人来一起完成的数据集的创建...
事实上RLHF真正难点不是技术,而是钱,毕竟人力成本才是最贵的!
那我没有钱,雇佣不起那么多公司和人,我可以训练大模型吗,可以获得数据集吗?当然可以,