持续创作,加速成长!这是我参与「掘金日新计划 · 6 月更文挑战」的第24天,点击查看活动详情
ShowMeAI日报系列全新升级!覆盖AI人工智能 工具&框架 | 项目&代码 | 博文&分享 | 数据&资源 | 研究&论文 等方向。点击查看 历史文章列表,在公众号内订阅话题 #ShowMeAI资讯日报,可接收每日最新推送。点击 专题合辑&电子月刊 快速浏览各专题全集。
1.工具&框架
工具:Unclutter - Immersive Reading Mode,排除干扰信息专注阅读的浏览器插件
'Unclutter - Immersive Reading Mode - A reader mode browser extension to remove distractions from web articles.' by lindylearn
GitHub: github.com/lindylearn/…
工具库:scikit-opt - 一个纯Python群体智能算法库
包含很多算法(差分进化算法、遗传算法、粒子群算法、模拟退火算法、蚁群算法、鱼群算法、免疫优化算法),特点是轻量、易部署,支持GPU运算。
GitHub: github.com/guofei9987/…
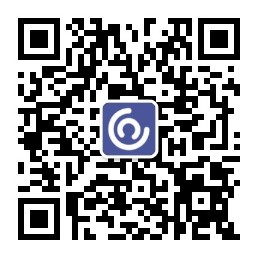
工具:Hayabusa - 基于sigma的Windows事件日志分析工具
它协助安全人员快速找到安全威胁。
GitHub: github.com/Yamato-Secu…
工具:Gifsicle - 一个在浏览器里进行gif编辑的工具。
Gifsicle可以对Gif图片进行压缩,旋转,裁剪等操作
GitHub: github.com/renzhezhilu…
工具库:AREkit - 文档级属性关系提取工具包
'AREkit - Document level Attitude and Relation Extraction toolkit (AREkit) for mass-media news and analytical articles' by Nicolay Rusnachenko
GitHub: github.com/nicolay-r/A…
2.博文&分享
课程:新加坡国立大学《3D计算机视觉》
《3D Computer Vision | National University of Singapore - YouTube》
Link: www.youtube.com/playlist?li…
博文:Vim 命令、操作、快捷键全集
Link: weibo.com/ttarticle/p…
3.数据&资源
资源列表:深度学习3D视觉最新论文列表
'Trending-in-3D-Vision - An on-going paper list on new trends in 3D vision with deep learning' by Xiaolong
GitHub: github.com/dragonlong/…
书籍:《Python Data Science Handbook》Python数据科学
介绍数据科学和应用的书籍。内容覆盖:① 数据科学家需要的计算环境:IPython和Jupyter ② NumPy工具库与科学计算 ③ Pandas与数据处理 ④ Matplotlib与数据可视化 ⑤ Scikit-Learn与机器学习。
英文原版地址: jakevdp.github.io/PythonDataS…
非官方中文翻译地址: github.com/wangyingsm/…
4.研究&论文
公众号回复关键字 日报,免费获取整理好的6月论文合辑。
论文:Automatic Prosody Annotation with Pre-Trained Text-Speech Model
论文标题:Automatic Prosody Annotation with Pre-Trained Text-Speech Model
论文时间:16 Jun 2022
所属领域:语音
对应任务:Speech Synthesis,Text-To-Speech Synthesis,语音合成,文本到语音合成
论文作者:Ziqian Dai, Jianwei Yu, Yan Wang, Nuo Chen, Yanyao Bian, Guangzhi Li, Deng Cai, Dong Yu
论文简介:Prosodic boundary plays an important role in text-to-speech synthesis (TTS) in terms of naturalness and readability./就自然性和可读性而言,韵律边界在文本到语音合成 (TTS) 中起着重要作用。
论文摘要:Prosodic boundary plays an important role in text-to-speech synthesis (TTS) in terms of naturalness and readability. However, the acquisition of prosodic boundary labels relies on manual annotation, which is costly and time-consuming. In this paper, we propose to automatically extract prosodic boundary labels from text-audio data via a neural text-speech model with pre-trained audio encoders. This model is pre-trained on text and speech data separately and jointly fine-tuned on TTS data in a triplet format: {speech, text, prosody}. The experimental results on both automatic evaluation and human evaluation demonstrate that: 1) the proposed text-speech prosody annotation framework significantly outperforms text-only baselines; 2) the quality of automatic prosodic boundary annotations is comparable to human annotations; 3) TTS systems trained with model-annotated boundaries are slightly better than systems that use manual ones.
就自然性和可读性而言,韵律边界在文本到语音合成 (TTS) 中起着重要作用。然而,韵律边界标签的获取依赖于人工标注,成本高且耗时。在本文中,我们建议通过带有预训练音频编码器的神经文本语音模型从文本音频数据中自动提取韵律边界标签。该模型分别在文本和语音数据上进行预训练,并在三元组格式的 TTS 数据上联合微调:{语音、文本、韵律}。自动评估和人工评估的实验结果表明:1)所提出的文本语音韵律注释框架显着优于纯文本基线; 2)自动韵律边界标注的质量与人工标注相当; 3) 使用模型标注边界训练的 TTS 系统比使用手动边界的系统稍好。
论文:Level 2 Autonomous Driving on a Single Device: Diving into the Devils of Openpilot
论文标题:Level 2 Autonomous Driving on a Single Device: Diving into the Devils of Openpilot
论文时间:16 Jun 2022
所属领域:计算机视觉
对应任务:无人驾驶,自动驾驶
论文作者:Li Chen, Tutian Tang, Zhitian Cai, Yang Li, Penghao Wu, Hongyang Li, Jianping Shi, Junchi Yan, Yu Qiao
论文简介:Equipped with a wide span of sensors, predominant autonomous driving solutions are becoming more modular-oriented for safe system design./配备广泛的传感器,主要的自动驾驶解决方案正变得更加模块化,以实现安全系统设计。
论文摘要:Equipped with a wide span of sensors, predominant autonomous driving solutions are becoming more modular-oriented for safe system design. Though these sensors have laid a solid foundation, most massive-production solutions up to date still fall into L2 phase. Among these, Comma.ai comes to our sight, claiming one $999 aftermarket device mounted with a single camera and board inside owns the ability to handle L2 scenarios. Together with open-sourced software of the entire system released by Comma.ai, the project is named Openpilot. Is it possible? If so, how is it made possible? With curiosity in mind, we deep-dive into Openpilot and conclude that its key to success is the end-to-end system design instead of a conventional modular framework. The model is briefed as Supercombo, and it can predict the ego vehicle's future trajectory and other road semantics on the fly from monocular input. Unfortunately, the training process and massive amount of data to make all these work are not publicly available. To achieve an intensive investigation, we try to reimplement the training details and test the pipeline on public benchmarks. The refactored network proposed in this work is referred to as OP-Deepdive. For a fair comparison of our version to the original Supercombo, we introduce a dual-model deployment scheme to test the driving performance in the real world. Experimental results on nuScenes, Comma2k19, CARLA, and in-house realistic scenarios verify that a low-cost device can indeed achieve most L2 functionalities and be on par with the original Supercombo model. In this report, we would like to share our latest findings, shed some light on the new perspective of end-to-end autonomous driving from an industrial product-level side, and potentially inspire the community to continue improving the performance. Our code, benchmarks are at github.com/OpenPercept…
主要的自动驾驶解决方案配备了广泛的传感器,在安全系统设计方面正变得更加模块化。尽管这些传感器已经奠定了坚实的基础,但迄今为止大多数量产解决方案仍处于 L2 阶段。其中,Comma.ai 出现在我们的视线中,声称一款售价 999 美元的售后设备安装了单个摄像头和板卡,具有处理 L2 场景的能力。加上 Comma.ai 发布的整个系统的开源软件,该项目被命名为 Openpilot。可能吗?如果是这样,它是如何实现的?带着好奇心,我们深入研究了 Openpilot,并得出结论,它成功的关键是端到端的系统设计,而不是传统的模块化框架。该模型简称为 Supercombo,它可以从单目输入动态预测自我车辆的未来轨迹和其他道路语义。不幸的是,所有这些工作的训练过程和大量数据都没有公开。为了进行深入调查,我们尝试重新实现训练细节并在公共基准上测试管道。在这项工作中提出的重构网络被称为 OP-Deepdive。为了将我们的版本与原始 Supercombo 进行公平比较,我们引入了双模型部署方案来测试现实世界中的驾驶性能。 nuScenes、Comma2k19、CARLA 和内部真实场景的实验结果验证了低成本设备确实可以实现大多数 L2 功能,并且与原始 Supercombo 模型相当。在本报告中,我们想分享我们的最新发现,从工业产品层面阐明端到端自动驾驶的新视角,并可能激励社区继续提高性能。我们的代码和基准位于 github.com/OpenPercept…
论文:Discrete Contrastive Diffusion for Cross-Modal and Conditional Generation
论文标题:Discrete Contrastive Diffusion for Cross-Modal and Conditional Generation
论文时间:15 Jun 2022
所属领域:计算机视觉
对应任务:Contrastive Learning,Denoising,Image Generation,Music Generation,对比学习,去噪,图像生成,音乐生成
论文作者:Ye Zhu, Yu Wu, Kyle Olszewski, Jian Ren, Sergey Tulyakov, Yan Yan
论文简介:To this end, we introduce a Conditional Discrete Contrastive Diffusion (CDCD) loss and design two contrastive diffusion mechanisms to effectively incorporate it into the denoising process./为此,我们引入了条件离散对比扩散 (CDCD) 损失,并设计了两种对比扩散机制,以有效地将其纳入去噪过程。
论文摘要:Diffusion probabilistic models (DPMs) have become a popular approach to conditional generation, due to their promising results and support for cross-modal synthesis. A key desideratum in conditional synthesis is to achieve high correspondence between the conditioning input and generated output. Most existing methods learn such relationships implicitly, by incorporating the prior into the variational lower bound. In this work, we take a different route -- we enhance input-output connections by maximizing their mutual information using contrastive learning. To this end, we introduce a Conditional Discrete Contrastive Diffusion (CDCD) loss and design two contrastive diffusion mechanisms to effectively incorporate it into the denoising process. We formulate CDCD by connecting it with the conventional variational objectives. We demonstrate the efficacy of our approach in evaluations with three diverse, multimodal conditional synthesis tasks: dance-to-music generation, text-to-image synthesis, and class-conditioned image synthesis. On each, we achieve state-of-the-art or higher synthesis quality and improve the input-output correspondence. Furthermore, the proposed approach improves the convergence of diffusion models, reducing the number of required diffusion steps by more than 35% on two benchmarks, significantly increasing the inference speed.
扩散概率模型 (DPMs) 已成为一种流行的条件生成方法,因为它们具有可喜的结果和对跨模态合成的支持。条件合成中的一个关键要求是在条件输入和生成的输出之间实现高度对应。大多数现有方法通过将先验结合到变分下限中来隐式地学习这种关系。在这项工作中,我们采取了不同的路线 - 我们通过使用对比学习最大化它们的互信息来增强输入-输出连接。为此,我们引入了条件离散对比扩散(CDCD)损失,并设计了两种对比扩散机制,以有效地将其纳入去噪过程。我们通过将 CDCD 与传统的变分目标联系起来来制定 CDCD。我们展示了我们的方法在评估三种不同的多模态条件合成任务中的有效性:舞蹈到音乐生成、文本到图像合成和类条件图像合成。在每一个方面,我们都实现了最先进或更高的合成质量,并改善了输入-输出的对应关系。此外,所提出的方法提高了扩散模型的收敛性,在两个基准上将所需的扩散步骤数量减少了 35% 以上,显着提高了推理速度。
论文:GLIPv2: Unifying Localization and Vision-Language Understanding
论文标题:GLIPv2: Unifying Localization and Vision-Language Understanding
论文时间:12 Jun 2022
所属领域:计算机视觉,自然语言处理
对应任务:Contrastive Learning,Image Captioning,Instance Segmentation,Language Modelling,Masked Language Modeling,object-detection,Object Detection,Phrase Grounding,Referring Expression Segmentation,Semantic Segmentation,Visual Question Answering,VQA,对比学习,图像字幕,实例分割,语言建模,蒙面语言建模,物体检测,物体检测,短语接地,参考表达分割,语义分割,视觉问答
论文作者:Haotian Zhang, Pengchuan Zhang, Xiaowei Hu, Yen-Chun Chen, Liunian Harold Li, Xiyang Dai, Lijuan Wang, Lu Yuan, Jenq-Neng Hwang, Jianfeng Gao
论文简介:We present GLIPv2, a grounded VL understanding model, that serves both localization tasks (e. g., object detection, instance segmentation) and Vision-Language (VL) understanding tasks (e. g., VQA, image captioning)./我们提出了 GLIPv2,一种基于 VL 的理解模型,它同时服务于本地化任务(例如,对象检测、实例分割)和视觉语言 (VL) 理解任务(例如,VQA、图像字幕)。
论文摘要:We present GLIPv2, a grounded VL understanding model, that serves both localization tasks (e.g., object detection, instance segmentation) and Vision-Language (VL) understanding tasks (e.g., VQA, image captioning). GLIPv2 elegantly unifies localization pre-training and Vision-Language Pre-training (VLP) with three pre-training tasks: phrase grounding as a VL reformulation of the detection task, region-word contrastive learning as a novel region-word level contrastive learning task, and the masked language modeling. This unification not only simplifies the previous multi-stage VLP procedure but also achieves mutual benefits between localization and understanding tasks. Experimental results show that a single GLIPv2 model (all model weights are shared) achieves near SoTA performance on various localization and understanding tasks. The model also shows (1) strong zero-shot and few-shot adaption performance on open-vocabulary object detection tasks and (2) superior grounding capability on VL understanding tasks. Code will be released at github.com/microsoft/G…
我们提出了 GLIPv2,一个基于 VL 的理解模型,它服务于本地化任务(例如,目标检测、实例分割)和视觉语言(VL)理解任务(例如,VQA、图像字幕/看图说话)。 GLIPv2 优雅地将定位预训练和视觉语言预训练 (VLP) 与三个预训练任务相结合:短语接地作为检测任务的 VL 重构,区域-词对比学习作为新的区域-词级对比学习任务,以及掩码语言建模。这种统一不仅简化了之前的多阶段 VLP 程序,而且实现了定位和理解任务之间的互相促进。实验结果表明,单个 GLIPv2 模型(所有模型权重共享)在各种定位和理解任务上实现了接近 SoTA 的性能。该模型还展示了(1)在开放词汇目标检测任务上的强大的零样本和少样本适应性能和(2)在 VL 理解任务上的出色接地能力。代码将在 github.com/microsoft/G… 发布。
论文:Degradation-Aware Unfolding Half-Shuffle Transformer for Spectral Compressive Imaging
论文标题:Degradation-Aware Unfolding Half-Shuffle Transformer for Spectral Compressive Imaging
论文时间:20 May 2022
所属领域:计算机视觉
对应任务:Compressive Sensing,Image Reconstruction,Image Restoration,压缩感知,图像重建,图像恢复
论文作者:Yuanhao Cai, Jing Lin, Haoqian Wang, Xin Yuan, Henghui Ding, Yulun Zhang, Radu Timofte, Luc van Gool
论文简介:In coded aperture snapshot spectral compressive imaging (CASSI) systems, hyperspectral image (HSI) reconstruction methods are employed to recover the spatial-spectral signal from a compressed measurement./在编码孔径快照光谱压缩成像 (CASSI) 系统中,采用高光谱图像 (HSI) 重建方法从压缩测量中恢复空间光谱信号。
论文摘要:In coded aperture snapshot spectral compressive imaging (CASSI) systems, hyperspectral image (HSI) reconstruction methods are employed to recover the spatial-spectral signal from a compressed measurement. Among these algorithms, deep unfolding methods demonstrate promising performance but suffer from two issues. Firstly, they do not estimate the degradation patterns and ill-posedness degree from the highly related CASSI to guide the iterative learning. Secondly, they are mainly CNN-based, showing limitations in capturing long-range dependencies. In this paper, we propose a principled Degradation-Aware Unfolding Framework (DAUF) that estimates parameters from the compressed image and physical mask, and then uses these parameters to control each iteration. Moreover, we customize a novel Half-Shuffle Transformer (HST) that simultaneously captures local contents and non-local dependencies. By plugging HST into DAUF, we establish the first Transformer-based deep unfolding method, Degradation-Aware Unfolding Half-Shuffle Transformer (DAUHST), for HSI reconstruction. Experiments show that DAUHST significantly surpasses state-of-the-art methods while requiring cheaper computational and memory costs. Code and models will be released at github.com/caiyuanhao1…
在编码孔径快照光谱压缩成像 (CASSI) 系统中,采用高光谱图像 (HSI) 重建方法从压缩测量中恢复空间光谱信号。在这些算法中,深度展开方法表现出良好的性能,但存在两个问题。首先,它们没有从高度相关的 CASSI 中估计退化模式和不适定度来指导迭代学习。其次,它们主要是基于 CNN 的,在捕获远程依赖方面表现出局限性。在本文中,我们提出了一个原则性的退化感知展开框架(DAUF),它从压缩图像和物理掩码中估计参数,然后使用这些参数来控制每次迭代。此外,我们定制了一种新颖的 Half-Shuffle Transformer (HST),它同时捕获本地内容和非本地依赖项。通过将 HST 插入 DAUF,我们建立了第一个基于 Transformer 的深度展开方法,即 Degradation-Aware Unfolding Half-Shuffle Transformer (DAUHST),用于 HSI 重建。实验表明,DAUHST 显着超越了最先进的方法,同时所需计算量和内存成本也降低了。代码和模型将在 github.com/caiyuanhao1… 发布
论文:HumanNeRF: Free-viewpoint Rendering of Moving People from Monocular Video
论文标题:HumanNeRF: Free-viewpoint Rendering of Moving People from Monocular Video
论文时间:CVPR 2022
所属领域:计算机视觉
论文作者:Chung-Yi Weng, Brian Curless, Pratul P. Srinivasan, Jonathan T. Barron, Ira Kemelmacher-Shlizerman
论文简介:Our method optimizes for a volumetric representation of the person in a canonical T-pose, in concert with a motion field that maps the estimated canonical representation to every frame of the video via backward warps./我们的方法优化了人在标准 T 姿势中的体积表示,与运动场相一致,该运动场通过向后扭曲将估计的标准表示映射到视频的每一帧。
论文摘要:We introduce a free-viewpoint rendering method -- HumanNeRF -- that works on a given monocular video of a human performing complex body motions, e.g. a video from YouTube. Our method enables pausing the video at any frame and rendering the subject from arbitrary new camera viewpoints or even a full 360-degree camera path for that particular frame and body pose. This task is particularly challenging, as it requires synthesizing photorealistic details of the body, as seen from various camera angles that may not exist in the input video, as well as synthesizing fine details such as cloth folds and facial appearance. Our method optimizes for a volumetric representation of the person in a canonical T-pose, in concert with a motion field that maps the estimated canonical representation to every frame of the video via backward warps. The motion field is decomposed into skeletal rigid and non-rigid motions, produced by deep networks. We show significant performance improvements over prior work, and compelling examples of free-viewpoint renderings from monocular video of moving humans in challenging uncontrolled capture scenarios.
我们介绍了一种自由视点渲染方法 - HumanNeRF - 它适用于人类执行复杂身体运动的给定单目视频,例如:来自 YouTube 的视频。我们的方法可以在任何帧暂停视频,并从任意新的摄像机视点甚至是该特定帧和身体姿势的完整 360 度摄像机路径渲染主体。这项任务特别具有挑战性,因为它需要合成身体的逼真细节,从输入视频中可能不存在的各种摄像机角度看,以及合成精细的细节,如布料褶皱和面部外观。我们的方法优化了典型 T 姿势中人的体积表示,与运动场相一致,该运动场通过向后扭曲将估计的典型表示映射到视频的每一帧。运动场被分解为由深度网络产生的骨骼刚性和非刚性运动。我们展示了相对于先前工作的显着性能改进,以及在具有挑战性的不受控制的捕获场景中移动人类的单目视频的自由视点渲染示例。
论文:SemanticStyleGAN: Learning Compositional Generative Priors for Controllable Image Synthesis and Editing
论文标题:SemanticStyleGAN: Learning Compositional Generative Priors for Controllable Image Synthesis and Editing
论文时间:CVPR 2022
所属领域:计算机视觉
对应任务:Disentanglement,Facial Editing,Image Generation,Transfer Learning,解缠结,人脸编辑,图像生成,迁移学习
论文作者:Yichun Shi, Xiao Yang, Yangyue Wan, Xiaohui Shen
论文简介:When combined with editing methods designed for StyleGANs, it can achieve a more fine-grained control to edit synthesized or real images./当与为 StyleGAN 设计的编辑方法结合使用时,它可以实现更细粒度的控制来编辑合成或真实图像。
论文摘要:Recent studies have shown that StyleGANs provide promising prior models for downstream tasks on image synthesis and editing. However, since the latent codes of StyleGANs are designed to control global styles, it is hard to achieve a fine-grained control over synthesized images. We present SemanticStyleGAN, where a generator is trained to model local semantic parts separately and synthesizes images in a compositional way. The structure and texture of different local parts are controlled by corresponding latent codes. Experimental results demonstrate that our model provides a strong disentanglement between different spatial areas. When combined with editing methods designed for StyleGANs, it can achieve a more fine-grained control to edit synthesized or real images. The model can also be extended to other domains via transfer learning. Thus, as a generic prior model with built-in disentanglement, it could facilitate the development of GAN-based applications and enable more potential downstream tasks.
最近的研究表明,StyleGAN 为图像合成和编辑的下游任务提供了有前途的先验模型。然而,由于 StyleGAN 的潜在代码旨在控制全局样式,因此很难实现对合成图像的细粒度控制。我们提出了 SemanticStyleGAN,其中一个生成器被训练来分别对局部语义部分进行建模,并以组合的方式合成图像。不同局部部分的结构和纹理由相应的潜在代码控制。实验结果表明,我们的模型在不同的空间区域之间提供了强大的解耦。当与为 StyleGAN 设计的编辑方法相结合时,它可以实现更细粒度的控制来编辑合成或真实图像。该模型还可以通过迁移学习扩展到其他领域。因此,作为具有内置解缠结的通用先验模型,它可以促进基于 GAN 的应用程序的开发和支撑更多潜在的下游任务。
论文:3D-aware Image Synthesis via Learning Structural and Textural Representations
论文标题:3D-aware Image Synthesis via Learning Structural and Textural Representations
论文时间:CVPR 2022
所属领域:计算机视觉
对应任务:3D-Aware Image Synthesis,Image Generation,3D感知图像合成,图像生成
论文作者:Yinghao Xu, Sida Peng, Ceyuan Yang, Yujun Shen, Bolei Zhou
论文简介:The feature field is further accumulated into a 2D feature map as the textural representation, followed by a neural renderer for appearance synthesis./特征场进一步积累成二维特征图作为纹理表示,然后是神经渲染器进行外观合成。
论文摘要:Making generative models 3D-aware bridges the 2D image space and the 3D physical world yet remains challenging. Recent attempts equip a Generative Adversarial Network (GAN) with a Neural Radiance Field (NeRF), which maps 3D coordinates to pixel values, as a 3D prior. However, the implicit function in NeRF has a very local receptive field, making the generator hard to become aware of the global structure. Meanwhile, NeRF is built on volume rendering which can be too costly to produce high-resolution results, increasing the optimization difficulty. To alleviate these two problems, we propose a novel framework, termed as VolumeGAN, for high-fidelity 3D-aware image synthesis, through explicitly learning a structural representation and a textural representation. We first learn a feature volume to represent the underlying structure, which is then converted to a feature field using a NeRF-like model. The feature field is further accumulated into a 2D feature map as the textural representation, followed by a neural renderer for appearance synthesis. Such a design enables independent control of the shape and the appearance. Extensive experiments on a wide range of datasets show that our approach achieves sufficiently higher image quality and better 3D control than the previous methods.
使生成模型具有 3D 感知能力在 2D 图像空间和 3D 物理世界之间架起一座桥梁,但仍然具有挑战性。最近的尝试为生成对抗网络 (GAN) 配备了神经辐射场 (NeRF),它将 3D 坐标映射到像素值,作为 3D 先验。然而,NeRF 中的隐函数具有非常局部的感受野,使得生成器很难意识到全局结构。同时,NeRF 建立在体绘制之上,其成本太高而无法产生高分辨率结果,从而增加了优化难度。为了缓解这两个问题,我们提出了一种称为 VolumeGAN 的新颖框架,用于通过显式学习结构表示和纹理表示来进行高保真 3D 感知图像合成。我们首先学习一个特征量来表示底层结构,然后使用类似 NeRF 的模型将其转换为特征场。特征场进一步累积成 2D 特征图作为纹理表示,然后是用于外观合成的神经渲染器。这样的设计能够独立控制形状和外观。在广泛的数据集上进行的大量实验表明,我们的方法比以前的方法实现了更高的图像质量和更好的 3D 控制。
我们是 ShowMeAI,致力于传播AI优质内容,分享行业解决方案,用知识加速每一次技术成长!点击查看 历史文章列表,在公众号内订阅话题 #ShowMeAI资讯日报,可接收每日最新推送。点击 专题合辑&电子月刊 快速浏览各专题全集。