Thanks for participation of Dr Chang Xu and assistant professor Ran He from The University of Sydney and National Laboratory of Pattern Recognition separately.
Unconditional Generative Models
Generative Adversarial Network [Goodfellow et al, 2014]
Variational Autoencoder [Kingma et al, 2014]
Autoregressive Models (PixelRNN, PixelCNN) [Oord et al, 2016]
Reversible Flow [Dinh et al, 2014]
Unconditional Dual Generative Models
CoGAN [Liu et al, 2016] MERL
DVG [Fu et al, 2019] NLPR
Conditional Generative Models
Class-conditional
Conditional Image-to-image Translation
Conditional Image/Video-to-video Generation
Applications
`face generation and translation
inpainting/expression/rotation/aging/attribute
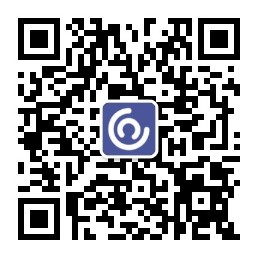
`style transfer
`super resolution
`text to image
`audio/video generation
`pose-based human image generation
A Siarohin, E Sangineto, Deformable GANs for Pose-based Human Image Generation CVPR2018.
`Human Motion Transfer
C Chan, S Ginosar, T Zhou, AA Efros, Everybody Dance Now, Siggraph 2018.
`Adversarial Domain Adaptation
Unsupervised domain adaptation by backpropagationICML 2015
Multiple source domain adaptation. [NeurIPS 2018]
Structured domain adaptation (e.g. segmentation). [CVPR 2018]
Conditional domain discriminator. [NeurIPS 2018]
Domain classifier →Task-specific classifier. [CVPR 2018]
Feature augmentation via another GANs. [CVPR 2018]
`Adversarial Examples
Goodfellow et al., 2015; Carlini and Wagner, 2017; Liu et al., 2017