Article directory
ChatGPT is not all you need, read all SOTA generative AI models in one article: a full review of 21 models in 9 categories from 6 major companies (1)
In the past two months, we have been screened by ChatGPT. It is no exaggeration to say that its development speed is like a rocket. With its excellent performance, since the open source of Stable Diffusion and the open interface of ChatGPT, the industry has become more enthusiastic about generative models. However, the release speed of generative SOTA models is so fast and there are so many types that it is difficult for us not to miss every model.
Last month, researchers from Comillas Pontifical University in Spain submitted a review paper " ChatGPT is not all you need. A State of the Art Review of large Generative AI models ", which will generate models According to the task modality and domain, it is divided into 9 categories, and the capabilities and limitations of 21 generative models released in 2022 are summarized. These limitations include the lack of large datasets for specific tasks and the need for expensive computing resources.
Application:ChatGPT is not all you need. A State of the Art Review of Large Generative AI Models
Presented
by: Roberto Gozalo-Brizuela, Eduardo C. Garrido-Merch ́an
: https://arxiv.org/pdf /2301.04655.pdf
First of all, the models can be divided into 9 categories according to the input and output data types, as shown in Figure 1 below.
The main focus of the article is to describe the latest developments in generative AI models. In order to give readers an overall understanding, all published models are given in Figure 2.
In addition, behind these published large models, only 6 companies (OpenAl, Google, DeepMind, Meta, runway, Nvidia) as shown in Figure 3 below, with the help of acquired startups and cooperation with academia, successfully deployed these state-of-the-art generative AI models. The main reason behind this fact is that in order to be able to estimate the parameters of these models, it is necessary to have a lot of computing power and a team skilled and experienced in data science and data engineering.
At the level of major companies involved in startups, Microsoft invested $10 billion in OpenAI and helped them develop models. Additionally, Google acquired Deepmind in 2014.
In terms of universities, VisualGPT was developed by King Abdullah University of Science and Technology (KAUST), Carnegie Mellon University and Nanyang Technological University; Human Motion Diffusion model was developed by Tel Aviv University in Israel.
At the level of cooperation between companies and universities, for example, Stable Diffusion is jointly developed by Runway, Stability AI and the University of Munich; Soundify is jointly developed by Runway and Carnegie Mellon University; DreamFusion is jointly developed by Google and the University of California, Berkeley.
The article introduces the 9 categories described in Figure 1 in detail from the third chapter, and for each category, the detailed information of the model is displayed accordingly.
Text-to-Image model
Let's first look at the Text-to-Image model, that is, a model in which the input is a text prompt and the output is an image.
FROM-E 2
DALL-E 2, developed by OpenAI, is capable of generating original, real, lifelike images and art from cues consisting of textual descriptions at a 4x higher resolution than DALL-E 1. OpenAI has provided an API to access the model.
The special feature of DALL-E 2 is that it can combine concepts, attributes and different styles. Its ability comes from the language-image pre-training model CLIP neural network, which can indicate the most relevant text fragments in natural language.
CLIP is a work of OpenAI in early 2021: " Learning Transferable Visual Models From Natural Language Supervision ". CLIP is a set of models with 9 image encoders, 5 convolutional encoders and 4 transformer encoders. It is a zero-shot visual classification model, and the pre-trained model has achieved good transfer results on downstream tasks without fine-tuning. The author tested on more than 30 data sets, covering tasks such as OCR, motion detection in videos, and coordinate positioning. See https://github.com/openai/CLIP for details .
Specifically, CLIP embedding has several desirable properties: it is able to perform stable transformations on image distributions; it has a strong zero-shot capability; and it achieves state-of-the-art results after fine-tuning. To obtain a complete image generative model, the CLIP image embedding decoder module is combined with a prior model to generate relevant CLIP image embeddings from a given text caption.
Therefore, the image generated by DALL-E 2 cleverly combines different and unrelated elements semantically. For example, entering prompt: a bowl of soup that is a portal to another dimension as digital art generates the image below.
IMAGE
Imagen is a text-to-image diffusion model that can generate more realistic images. This is built on a large transformer language model. Google has provided an API to access the model.
Imagen mainly uses the T5 model as a pre-training model, and uses 800GB of training corpus for pre-training. After the pre-training is over, it is then frozen, input into the Text-to-Image diffusion Model, and then upsampled to make the picture generate a high-definition image. The specific model structure is as follows:
At the same time, Google found that general large-scale language models (such as T5) pre-trained on plain text corpora are surprisingly effective in encoding text for image synthesis. Instead of increasing the size of the diffusion model, but increasing the size of the language model, the generated effect will be more realistic.
To sum up, there are several main findings when using Imagen:
- Large pretrained frozen text encoders are very effective for text-to-image generation tasks.
- Increasing the size of the language model improves sample fidelity and image-text alignment more than increasing the size of the image diffusion model.
- Introduces a new efficient U-Net architecture that is more computationally efficient, more memory efficient, and faster to converge.
- The model achieved the highest effect without using the COCO dataset for training.
In addition, Google researchers launched DrawBench, a more challenging test benchmark than COCO, which contains various tricky prompt words. DrawBench is a multidimensional evaluation of image-to-text (text to image) models. It contains 11 categories with about 200 text cues aimed at exploring different semantic properties of the model.
Stable Diffusion
Stable Diffusion is a text-to-image model based on Latent Diffusion Models (LDMs). If you want to have a deeper understanding of the technical principles of Stable Diffusion, you can read the paper "High-Resolution Image Synthesis with Latent Diffusion Models", which was published at CVPR2022 and was developed by the Machine Vision and Learning Research Group of the University of Munich, Germany. Stability AI has officially practiced its open source commitment, and has released version 2.0 of Stable Diffusion, project address: https://github.com/Stability-AI/stablediffusion.
Compared with other models, the main difference of Stable Diffusion is the use of Latent Diffusion Models, which generates images by iterating "denoising" data in a latent representation space, and then decodes the representation results into complete images, so that text generation can On consumer-grade GPUs, images can be generated in 10 seconds, which greatly reduces the barriers to implementation and brings a fire in the field of text and image generation.
The overall framework of Latent Diffusion Models is shown in the figure below. First, you need to train a self-encoder model (AutoEncoder), so that you can use the encoder to compress the image, then do the diffusion operation on the latent representation space, and finally use the decoder to restore the original pixels. The space is sufficient, and the paper calls this method Perceptual Compression (Perceptual Compression).
Muse
The text-image generation model Muse released by Google does not use the current popular diffusion model (diffusion model), but uses the classic Transformer model to achieve the most advanced image generation performance, compared to diffusion or autoregressive (autoregressive) models , the efficiency of the Muse model is also greatly improved.
Muse is trained on a discrete token space as a masked modeling task: Given a text embedding extracted from a pretrained large language model (LLM), the training process of Muse is to predict randomly masked image tokens.
Compared with the diffusion model of pixel space (such as Imagen and DALL-E 2), since Muse uses discrete tokens and requires fewer sampling iterations, the efficiency is significantly improved. Compared to Parti (an autoregressive model), Muse is more efficient due to parallel decoding. Muse is 10 times faster than Imagen-3B or Parti-3B in inference time, and 3 times faster than Stable Diffusion v1.4.
The framework of the Muse model contains multiple components. The training pipeline consists of a T5-XXL pre-trained text encoder, a base model and a super-resolution model, as shown in the figure below.
Text-to-3D model
The current text image generation models such as DALL-E 2, Imagen, etc. still remain in two-dimensional creation (that is, pictures), and cannot generate 360-degree 3D models without dead ends. It is very difficult to directly train a text-to-3D model, because the training of models such as DALL-E 2 needs to swallow billions of image-text pairs, but there is no such large-scale labeled data for 3D synthesis, and there is no An efficient model architecture for denoising 3D data.
But now the model trained with 2D data can also generate 3D images. As long as you enter simple text prompts, you can generate 3D models with elements such as density and color.
Dreamfusion
DreamFusion, developed by Google Research, uses a pre-trained 2D text-to-image diffusion model for text-to-3D synthesis. Specifically, DreamFusion first uses a pre-trained 2D diffusion model to generate a two-dimensional image based on text prompts, and then introduces a loss function based on probability density distillation to optimize a randomly initialized neural radiation field NeRF model through gradient descent. It replaces CLIP with a new loss calculation method: the loss is calculated by the Imagen diffusion model from text to image.
The trained model can generate a model based on a given text prompt in any angle, any lighting condition, and any 3D environment. The whole process does not require 3D training data, nor does it need to modify the image diffusion model. It completely relies on the pre-trained diffusion model as the first step. test.
Compared with other methods that mainly sample pixels, sampling in parameter space is much more difficult than sampling in pixel space, DreamFusion uses a differentiable generator that focuses on creating 3D models that render images from random angles.
Magic3D
Magic3D is a text-to-3D model developed by NVIDIA Corporation. Although the Dreamfusion model achieves remarkable results, this method suffers from two problems: long processing time and low quality of generated images. However, Magic3D addresses these issues using a two-stage optimization framework.
First, Magic3D builds a low-resolution diffusion prior, then, it accelerates it using a sparse 3D hash grid structure. Using this, the textured 3D mesh model is further optimized with efficient differentiable rendering. After human evaluation comparing Dreamfusion and Magic3D, the Magic3D model achieved better results, the results indicated that 61.7% of people prefer Magic3D to DreamFusion. As shown in Figure 9 below, Magic3D achieves higher quality 3D shapes in terms of geometry and texture compared to DreamFusion.
Friends please continue to pay attention to my public account " HsuDan ", I will continue to update the remaining 7 in this generative AI model review " ChatGPT is not all you need. A State of the Art Review of large Generative AI models " Large categories of models: Image-to-Text model, Text-to-Video model, Text-to-Audio model, Text-to-Text model, Text-to-Code model, Text-to-Science model, etc.
Welcome everyone to pay attention to my personal public account: HsuDan , I will share more about my learning experience, pit avoidance summary, interview experience, and the latest AI technology information.
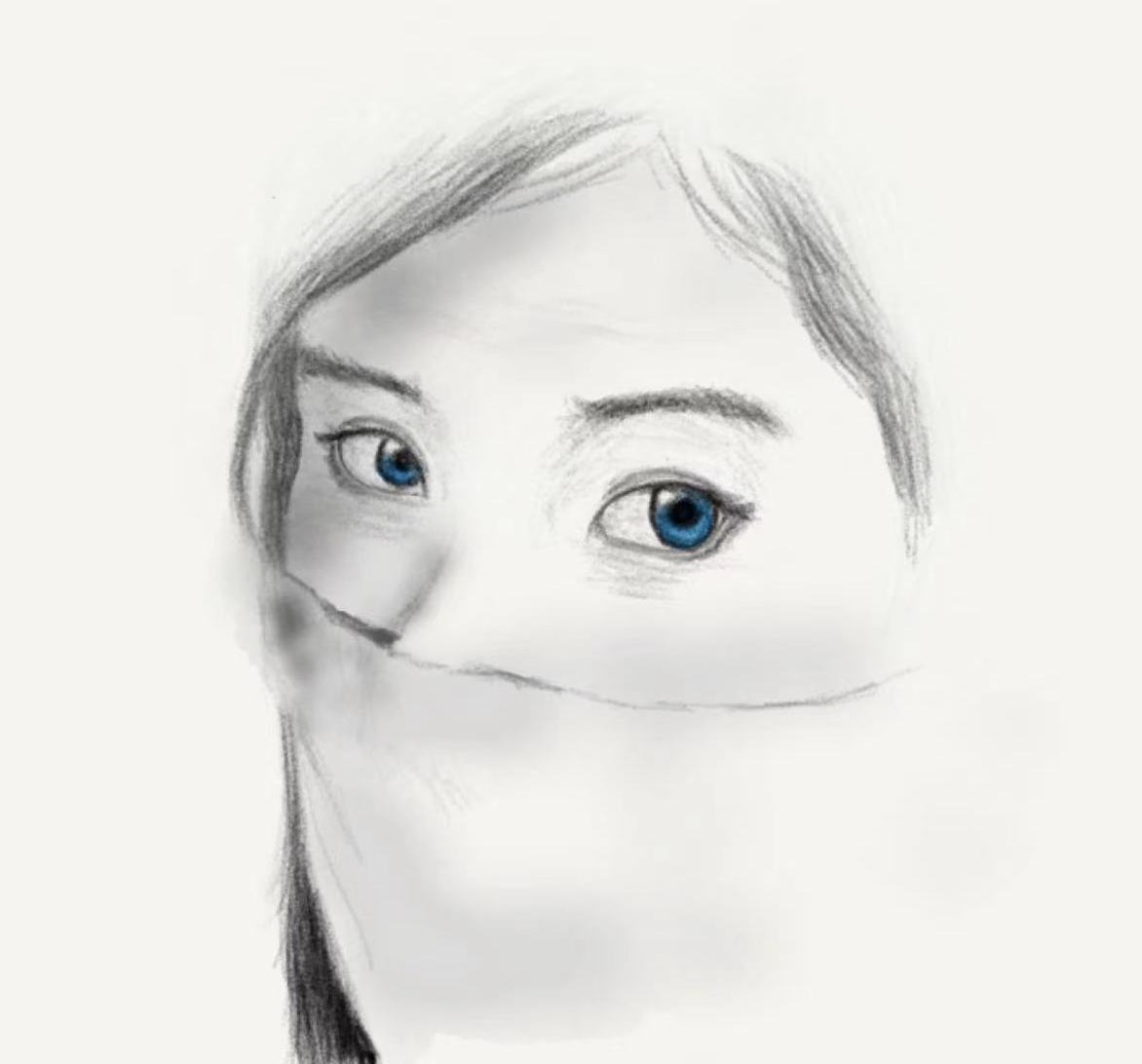