Article directory
ChatGPT is not all you need, read all SOTA generative AI models in one article: a full review of 21 models in 9 categories from 6 major companies (2)
AI painting has become a hot technology topic in 2022, thanks to the new interactive method of "text-to-image" or text-to-3D (text-to-3D). In August 2022, Stable Diffusion will be officially opened, which will undoubtedly further bring the recent enthusiasm to AI creation.
Just like when machine learning first took off, AI generative techniques didn’t just appear out of thin air. Only in the past one or two years, the quality and calculation speed of works have been increasing rapidly, which makes us ignore the equally long history of AI painting.
On January 27, Google released a new AI model - MusicLM. Through the AI model MusicLM, text can directly generate high-fidelity music. Following the popularity of text-generated AI models in the field of painting creation, the music field is about to be captured by Jukebox (a music creation model driven by artificial intelligence), etc. It is not difficult to see that the generative AI track is ushering in an explosion.
Today we continue to study the review paper " ChatGPT is not all you need. A State of the Art Review of large Generative AI models " submitted by researchers from Comillas Pontifical University in Spain .
Application:ChatGPT is not all you need. A State of the Art Review of Large Generative AI Models
Presented
by: Roberto Gozalo-Brizuela, Eduardo C. Garrido-Merch ́an
: https://arxiv.org/pdf /2301.04655.pdf
You can review the content of the first part:
Portal: ChatGPT is not all you need, read all SOTA generative AI models in one article: a full review of 21 models in 9 categories from 6 major companies (1)
In this second part, let's look at some details of the Image-to-Text, Text-to-Video, and Text-to-Audio models.
Image-to-text models
Sometimes it is also useful to get the text describing the image, which is equivalent to the inverse version of image generation.
Flamingo
Flamingo is a visual language model developed by Deepmind. On open visual language tasks, few-shot learning can be performed only through some input/output examples.
Specifically, the input of Flamingo includes an autoregressive text generation model under visual conditions, which can receive a sequence of text tokens interleaved with images or videos, and generate text as output. The Flamingo model leverages two complementary models: a vision model that analyzes visual scenes, and a large language model that performs basic forms of reasoning. Language models are trained on large amounts of text data.
Building models that can be quickly adapted to numerous tasks using only a small number of annotated examples is an open challenge in multimodal machine learning research. However, Flamingo has exactly this ability and has innovated in architecture: (i) connecting powerful pre-trained visual and language models, (ii) processing arbitrary interleaved visual and text data sequences, and (iii) seamlessly ingesting image or video as input. Due to their flexibility, Flamingo models can be trained on large-scale multimodal web corpora containing arbitrarily interleaved text and images, which is the key to endowing them with contextual few-shot learning capabilities.
Users can enter a query into the model, attach a photo or a video, and the model will answer with a text answer. As shown in Figure 10 below.
VisualGPT
VisualGPT is an image-text model developed by OpenAI. Based on the pre-trained language model GPT-2, a new attention mechanism is proposed to bridge the semantic differences between different modalities. It does not require a large amount of image-text data training. It can improve the efficiency of text generation. OpenAI has provided an API to access the model.
To more effectively fuse visual information into different layers of a language model, we can consider a specially designed cross-attention fusion mechanism to balance the mixing of text generation ability and visual information. Therefore, an innovation of VisualGPT is to adopt a self-reviving encoder-decoder attention mechanism to quickly adapt a pre-trained LM using a small amount of in-domain image-text data.
Image Captioning tasks require computers to describe the visual content of a picture in natural language. The current image description model is mainly based on the Encoder-Decoder architecture, which is trained on a large amount of paired graphic data to obtain more accurate and detailed image descriptions. However, the acquisition of large-scale manually labeled training data is expensive, and there are inevitably some errors after cleaning the automatically crawled data on the Internet, and some specific fields such as medical imaging reports do not have the conditions to construct large-scale data sets.
The biggest advantage of VisualGPT is that it proposes for the first time to adapt the pre-trained language model PLM to image description tasks in various fields to alleviate the problems existing in the data. By modifying the structure of gpt as a decoder, inserting the self-resurrection activation gate (SRAU), balancing the language knowledge and input image information learned by PLM in advance, so as to better solve the description problem of new objects, and finally generate higher quality images. image description.
Figure 11 below contains examples of three text cues generated by the model that relate to the three images that were input to the model.
Text-to-Video model
In the second half of 2022, we saw some text-to-video models, looking forward to models with higher resolution and frame rate.
Phenaki
Following Meta's Make-A-Video, Google has released two video models, Imagen Video and Phenaki. The two emphasize different functions such as video quality and length respectively.
Phenaki, developed by Google Research, is a model capable of photorealistic video synthesis given a series of text cues. Google has provided an API to access the model.
Phenaki is the first model that can generate videos from open-domain temporally variable cues.
In order to solve the problem of less training data, Google also expanded the available range of video data sets by jointly training on a large image-text pair corpus and a small number of video-text examples. Primarily image-text datasets tend to have billions of input data, while text-video datasets are much smaller and computing on videos of different lengths is also a challenge.
The Phenaki model consists of three parts: C-ViViT Encoder, Training Transformer and Video Generator.
Phenaki compresses video into discrete embeddings based on a new codec architecture, C-ViViT. After converting the input token to embedding, it then passes through the timing Transformer and space Transformer, and then uses a single linear projection without activation to map the token back to the pixel space.
The final model can generate temporally coherent and diverse videos conditioned on open-domain cues, and is even able to handle some novel concepts that do not exist in the dataset. Videos can be several minutes long, and the model is trained on 1.4-second videos. Some examples of creating a video from a series of text prompts and from a series of text prompts and images are shown in Figures 12 and 13 below.
Phenaki can convert detailed text prompts into videos longer than two minutes, but the downside is lower video quality.
Soundify
In video editing, sound is half the story. Skilled video editors overlay sound (such as effects and environments) over footage, add character to objects or immerse viewers in spaces. However, for professional video editing, the problem comes from finding the right sound, aligning sound, video, and tuning parameters, a process that can be tedious and time-consuming.
To solve this problem, Soundify is a system developed by Runway to match sound effects to video, with the purpose of producing sound effects. By leveraging a library of labeled studio sound effects and extending CLIP, a neural network with impressive Zero-Shot image classification capabilities, into a "Zero-Shot Detector", Soundify is able to Produce high-quality results in learning or audio generation situations.
Specifically, Soundify includes three modules: classification, synchronization, and mix. First, the model matches the effect with the video by classifying the sound, then compares the effect with each frame, and inserts the corresponding sound effects. This classification matches the effect by classifying the sound emitters in the video. To reduce different sound emitters, Soundify segments videos based on absolute color histogram distance. In the synchronization part, gaps are identified by comparing effect labels to each frame and thresholded to pinpoint consecutive matches. In the blending section, the effect is divided into roughly one-second chunks, and crucially, the chunks are stitched by cross-stitching.
Text-to-Audio model
Compared with Text-to-Image, which has popular AI painting, Text-to-Audio also has AI composition, and has a wide range of TTS (Text-to-speech) scenarios. TTS technology can be applied to the content creation of popular songs, musical compositions, and audio books, as well as the creation of soundtracks in the fields of video, games, and film and television, which greatly reduces the procurement cost of music copyrights. Among them, AI composition can be simply understood as "using the language model (currently represented by Transformer, such as Google Megenta, OpenAI Jukebox, AIVA, etc.) as an intermediary to perform two-way conversion of music data (via MIDI and other conversion paths)".
Images aren't the only important unstructured data format. For video, music, and many environments, audio can be critical.
AudioLM
AudioLM was developed by Google and can be used to generate high-quality audio with long-distance consistency.
AudioLM consists of three parts:
- A token generative model that maps a sequence of sounds into a discrete sequence of tokens. This step also reduces the size of the sequence (about 300 times less sampling rate).
- A decoder-only transformer that maximizes the likelihood of predicting the next token in the sequence. The model contains 12 layers, 16 attention heads, embedding dimension is 1024, and feed-forward layer dimension is 4096.
- A detokenized model that converts predicted tokens into audio tokens.
The special feature of AudioLM is that it maps the input audio into a discrete token sequence, converts the audio generation into a language modeling task, and learns to produce natural and coherent timbres based on prompt words. In the human assessment, 51.2% of the voices are considered to be human voices, which is close to the ratio of synthetic voices, indicating that the synthesis effect is close to that of real people. As with other models, the API can be found via GitHub.
Trained on a large number of raw audio waveforms, AudioLM successfully learns to generate natural coherent continuous speech given short cues. This method can even be extended to speech beyond the human voice, such as continuous piano music, etc., without adding symbolic representations during training.
Because audio signals involve multiple scales of abstraction, it is very challenging to achieve high audio quality while displaying consistency across multiple scales during audio synthesis. The AudioLM model is achieved by combining recent advances in neural audio compression, self-supervised representation learning, and language modeling.
Jukebox
Jukebox A music composition model developed by OpenAI that generates music with lyrics. However, the current model is still limited to English. As with other models, the API can be found via GitHub.
One of the early methods of automatically generating music was the note generator, which generates a score that can be played, but the biggest limitation of the note generator is that it cannot capture the human voice and other musical details such as timbre, dynamics and expressiveness.
There is another approach, directly modeling the music as raw audio. But because the audio sequence is very long, it is very difficult to generate music at the audio level. Taking 44 kHz, 16-bit CD music as an example, a 4-minute song may take 10 million hours. Therefore, to learn the high-level semantics of music, the model needs to be able to handle extremely long dependencies.
In general, training an automatically generated music model requires a challenge: the spatial dimension of the original audio is very high, and a large amount of information needs to be modeled. The key bottleneck is that modeling raw audio directly introduces extremely long-range dependencies, making it computationally difficult to understand the high-level semantics of music. The special feature of Jukebox is that it tries to solve it through the layered VQ-VAE architecture, compressing the audio into a discrete space, and the loss function is designed to retain the largest amount of information, which is used to solve the problem that AI is difficult to learn advanced features in audio. This mode is limited to English songs. Specifically, its training data set comes from LyricWiki's 1.2 million songs, of which 600,000 are English songs. VQ-VAE has 5 billion parameters and is trained for 3 days on 9-second audio clips.
In order to solve the problem of lyrics processing correspondence, Jukebox researchers also added new neural network-based tools:
- Spleeter, which can extract vocals from songs for speech recognition;
- NUS AutoLyricsAlign, align lyrics with songs;
- The attention mechanism allows the decoded music to pay attention to the changes in the position of the lyrics code as the playback progresses.
The Chinese of Jukebox is a jukebox, which probably means what to sing. As long as you provide the neural network with the type of music (genre), reference singers and lyrics as input, it can automatically generate the corresponding music: melody, harmony , and the lyrics to the song. Thanks to a Transformer-based architecture similar to GPT-2, Jukebox can generate diverse and coherent music, offering multiple renditions of the same song, giving users multiple options.
Whisper
Whisper is an automatic speech recognition model developed by OpenAI. According to OpenAI, the model is robust to accents, background noise, and technical language. In addition, it supports transcription and translation from 99 different languages to English. As with other models, the API can be found via GitHub.
First of all, the biggest feature of Whisper is the large-scale training set it uses: it uses 680,000 hours of multilingual, multi-task supervised data collected from the Internet for training. This results in a very diverse dataset, covering audio from many different environments, recording devices, and languages.
Second, the Whisper architecture is a simple end-to-end approach, specifically Transformer's encoder-decoder format.
The input audio is split into 30-second segments, converted into log-Mel spectrograms, and passed to the encoder.
The decoder is trained to predict the corresponding text captions, mixed with special tokens that instruct a single model to perform tasks such as language recognition, multilingual speech transcription, and English speech translation.
Compared with other models, English speech recognition is Whisper's core competitiveness.
reference:
https://baijiahao.baidu.com/s?id=1746191197239679569&wfr=spider&for=pc
https://roll.sohu.com/a/634763268_121207965
https://baijiahao.baidu.com/s?id=1756520241186383004&wfr=spider&for=pc
https://openai.com/blog/whisper/
Welcome everyone to pay attention to my personal public account: HsuDan , I will share more about my learning experience, pit avoidance summary, interview experience, and the latest AI technology information.
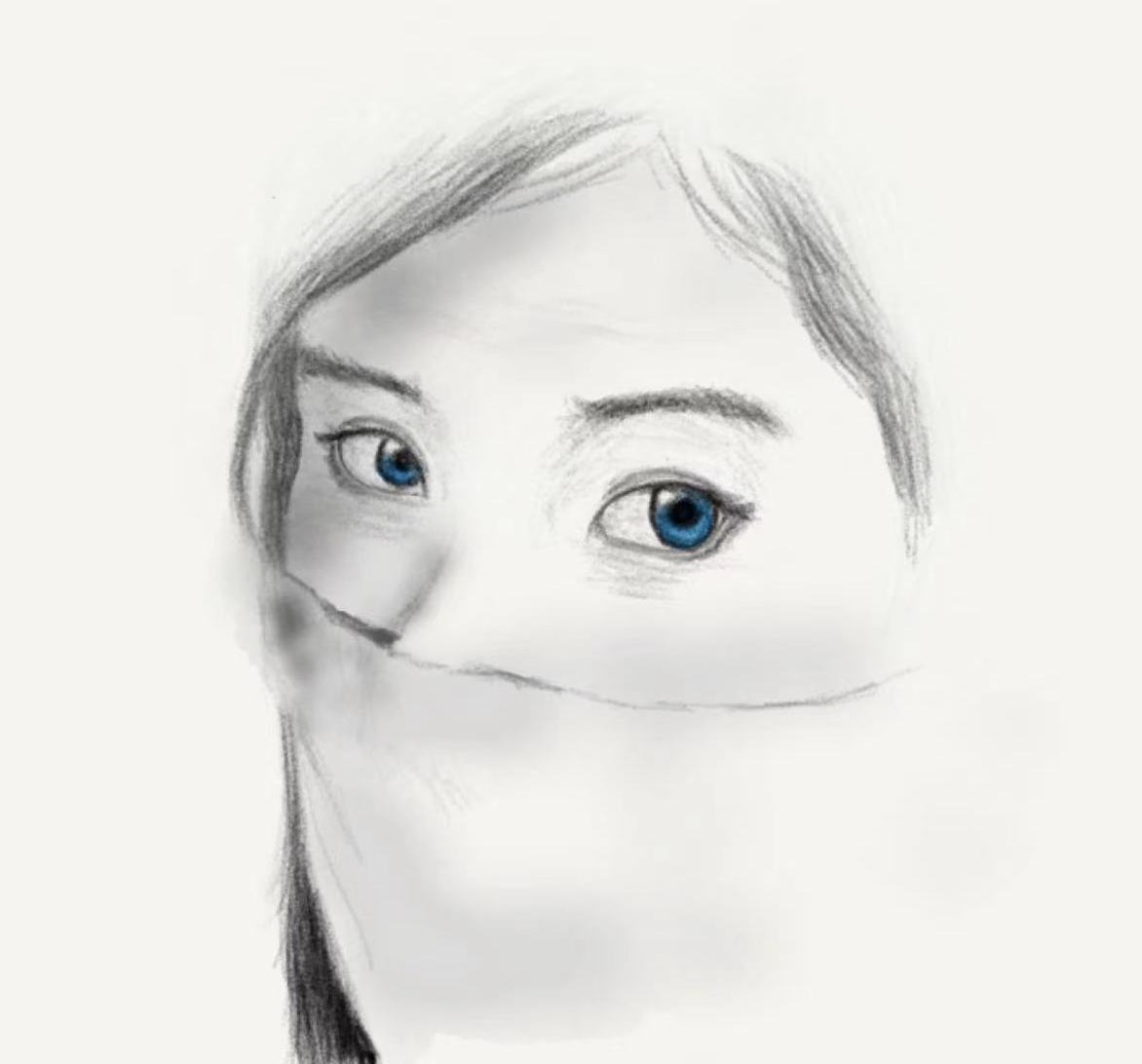