这个在GitHub上拿到34k星的项目?难道CSDN的程序员都只逛百度,不逛GitHub?
这个项目堪称传奇,在GitHub上被无数人称赞为新手必学项目,却偏偏又可以无需任何修改,就在任何平台、版本的IDE上运用,真正的0成本接入,堪称有史以来最伟大的工程,没有之一!
为了尊重原作者,请大家去项目中查看,绝对是计算机历史上的一座丰碑。
我上面的话,可不是我自己评价的,都是网友们的留言:
这也是我见过第一款,被中外老哥用花式语言夸奖的项目,比如Book思议(不可思议),甚至很多人用:道可道,非常道、无极、凝视深渊这里很哲理的话来夸奖
整个GitHub,甚至往大了说,全网你都找不到第二个这样的项目了!
项目名称:nocode
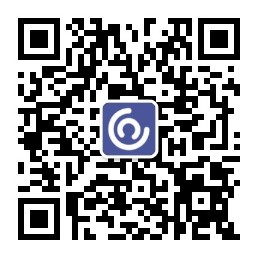
项目地址:https://github.com/kelseyhightower/nocode
等你心平气和,仔细看完项目就会明白,这个项目贯彻了git这个词的本意。
而且程序员其实有三重境界:
1.眼中有码,敲键盘如有神助。
2.心中有码,无码也有码,对于代码的理解近乎于道。
3.心中无码,有码也无码,他就是道。
只有到达第三重境界才能迈过35岁这个坎
我看完这个项目之后的感想:
再给大家来个硬核项目:99行代码实现《冰雪奇缘》!
《冰雪奇缘》没有真人出演,预算却高达1.5亿美元,每一秒的镜头都是经费在燃烧。一般人想用电脑做出CG特效简直不可想象。
然而,最近一位来自中国的MIT博士,开发了物理模拟编程语言:Taichi
大大降低了成本。
计算机图形学知名学者、北大教授陈宝权给出很高的评价:
#import taichi as ti
quality = 1 # Use a larger value for higher-res simulations
n_particles, n_grid = 9000 * quality ** 2, 128 * quality
dx, inv_dx = 1 / n_grid, float(n_grid)
dt = 1e-4 / quality
p_vol, p_rho = (dx * 0.5)**2, 1
p_mass = p_vol * p_rho
E, nu = 0.1e4, 0.2 # Young's modulus and Poisson's ratio
mu_0, lambda_0 = E / (2 * (1 + nu)), E * nu / ((1+nu) * (1 - 2 * nu)) # Lame parameters
x = ti.Vector(2, dt=ti.f32, shape=n_particles) # position
v = ti.Vector(2, dt=ti.f32, shape=n_particles) # velocity
C = ti.Matrix(2, 2, dt=ti.f32, shape=n_particles) # affine velocity field
F = ti.Matrix(2, 2, dt=ti.f32, shape=n_particles) # deformation gradient
material = ti.var(dt=ti.i32, shape=n_particles) # material id
Jp = ti.var(dt=ti.f32, shape=n_particles) # plastic deformation
grid_v = ti.Vector(2, dt=ti.f32, shape=(n_grid, n_grid)) # grid node momemtum/velocity
grid_m = ti.var(dt=ti.f32, shape=(n_grid, n_grid)) # grid node mass
ti.cfg.arch = ti.cuda # Try to run on GPU
@ti.kernel
def substep():
for i, j in ti.ndrange(n_grid, n_grid):
grid_v[i, j] = [0, 0]
grid_m[i, j] = 0
for p in range(n_particles): # Particle state update and scatter to grid (P2G)
base = (x[p] * inv_dx - 0.5).cast(int)
fx = x[p] * inv_dx - base.cast(float)
# Quadratic kernels [http://mpm.graphics Eqn. 123, with x=fx, fx-1,fx-2]
w = [0.5 * ti.sqr(1.5 - fx), 0.75 - ti.sqr(fx - 1), 0.5 * ti.sqr(fx - 0.5)]
F[p] = (ti.Matrix.identity(ti.f32, 2) + dt * C[p]) @ F[p] # deformation gradient update
h = ti.exp(10 * (1.0 - Jp[p])) # Hardening coefficient: snow gets harder when compressed
if material[p] == 1: # jelly, make it softer
h = 0.3
mu, la = mu_0 * h, lambda_0 * h
if material[p] == 0: # liquid
mu = 0.0
U, sig, V = ti.svd(F[p])
J = 1.0
for d in ti.static(range(2)):
new_sig = sig[d, d]
if material[p] == 2: # Snow
new_sig = min(max(sig[d, d], 1 - 2.5e-2), 1 + 4.5e-3) # Plasticity
Jp[p] *= sig[d, d] / new_sig
sig[d, d] = new_sig
J *= new_sig
if material[p] == 0: # Reset deformation gradient to avoid numerical instability
F[p] = ti.Matrix.identity(ti.f32, 2) * ti.sqrt(J)
elif material[p] == 2:
F[p] = U @ sig @ V.T() # Reconstruct elastic deformation gradient after plasticity
stress = 2 * mu * (F[p] - U @ V.T()) @ F[p].T() + ti.Matrix.identity(ti.f32, 2) * la * J * (J - 1)
stress = (-dt * p_vol * 4 * inv_dx * inv_dx) * stress
affine = stress + p_mass * C[p]
for i, j in ti.static(ti.ndrange(3, 3)): # Loop over 3x3 grid node neighborhood
offset = ti.Vector([i, j])
dpos = (offset.cast(float) - fx) * dx
weight = w[i][0] * w[j][1]
grid_v[base + offset] += weight * (p_mass * v[p] + affine @ dpos)
grid_m[base + offset] += weight * p_mass
for i, j in ti.ndrange(n_grid, n_grid):
if grid_m[i, j] > 0: # No need for epsilon here
grid_v[i, j] = (1 / grid_m[i, j]) * grid_v[i, j] # Momentum to velocity
grid_v[i, j][1] -= dt * 50 # gravity
if i < 3 and grid_v[i, j][0] < 0: grid_v[i, j][0] = 0 # Boundary conditions
if i > n_grid - 3 and grid_v[i, j][0] > 0: grid_v[i, j][0] = 0
if j < 3 and grid_v[i, j][1] < 0: grid_v[i, j][1] = 0
if j > n_grid - 3 and grid_v[i, j][1] > 0: grid_v[i, j][1] = 0
for p in range(n_particles): # grid to particle (G2P)
base = (x[p] * inv_dx - 0.5).cast(int)
fx = x[p] * inv_dx - base.cast(float)
w = [0.5 * ti.sqr(1.5 - fx), 0.75 - ti.sqr(fx - 1.0), 0.5 * ti.sqr(fx - 0.5)]
new_v = ti.Vector.zero(ti.f32, 2)
new_C = ti.Matrix.zero(ti.f32, 2, 2)
for i, j in ti.static(ti.ndrange(3, 3)): # loop over 3x3 grid node neighborhood
dpos = ti.Vector([i, j]).cast(float) - fx
g_v = grid_v[base + ti.Vector([i, j])]
weight = w[i][0] * w[j][1]
new_v += weight * g_v
new_C += 4 * inv_dx * weight * ti.outer_product(g_v, dpos)
v[p], C[p] = new_v, new_C
x[p] += dt * v[p] # advection
import random
group_size = n_particles // 3
for i in range(n_particles):
x[i] = [random.random() * 0.2 + 0.3 + 0.10 * (i // group_size), random.random() * 0.2 + 0.05 + 0.32 * (i // group_size)]
material[i] = i // group_size # 0: fluid 1: jelly 2: snow
v[i] = [0, 0]
F[i] = [[1, 0], [0, 1]]
Jp[i] = 1
import numpy as np
gui = ti.GUI("Taichi MLS-MPM-99", res=512, background_color=0x112F41)
for frame in range(20000):
for s in range(int(2e-3 // dt)):
substep()
colors = np.array([0x068587, 0xED553B, 0xEEEEF0], dtype=np.uint32)
gui.circles(x.to_numpy(), radius=1.5, color=colors[material.to_numpy()])
gui.show() # Change to gui.show(f'{frame:06d}.png') to write images to disk
这段代码用Python 3运行。运行前要根据操作系统和CUDA版本(如果有CUDA的话)安装taichi:
# CPU 版本 (支持Linux, OS X and Windows)
python3 -m pip install taichi-nightly
# GPU (CUDA 10.0) (只支持Linux)
python3 -m pip install taichi-nightly-cuda-10-0
# GPU (CUDA 10.1) (只支持Linux)
python3 -m pip install taichi-nightly-cuda-10-1
这99行代码虽然很短,其背后的故事却很长。
虽然语法看起来是Python,其计算部分却会被一整套编译系统接管,最后输出可执行的x86_64或者PTX instructions,能够在CPU/GPU上高效运行。