Article Directory
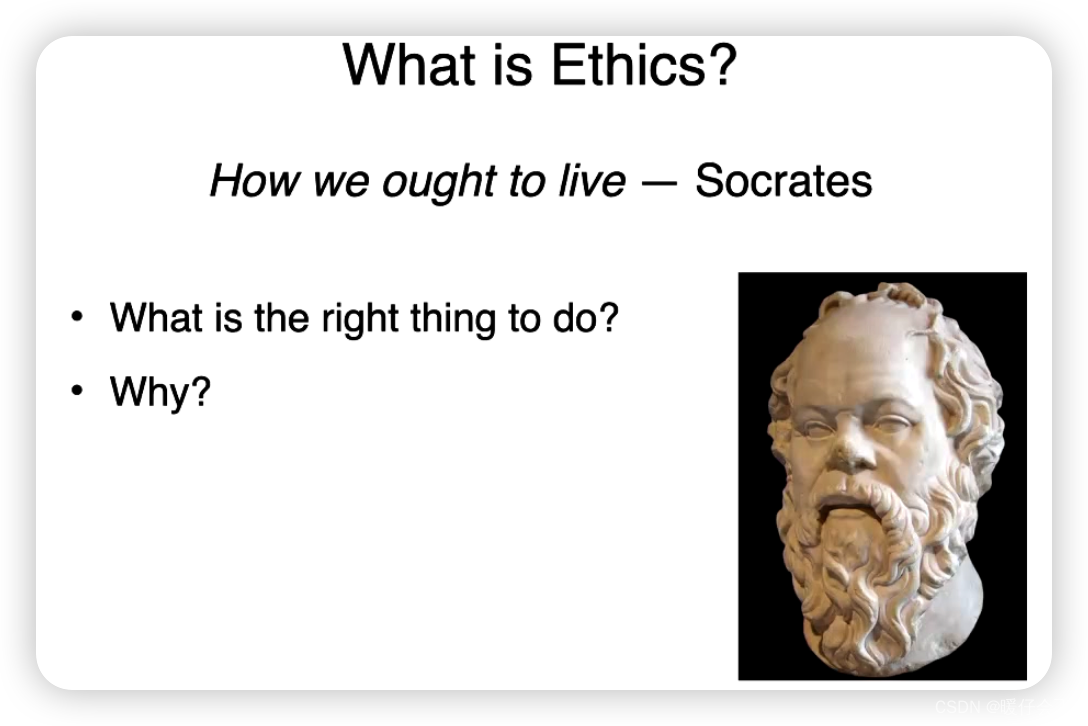
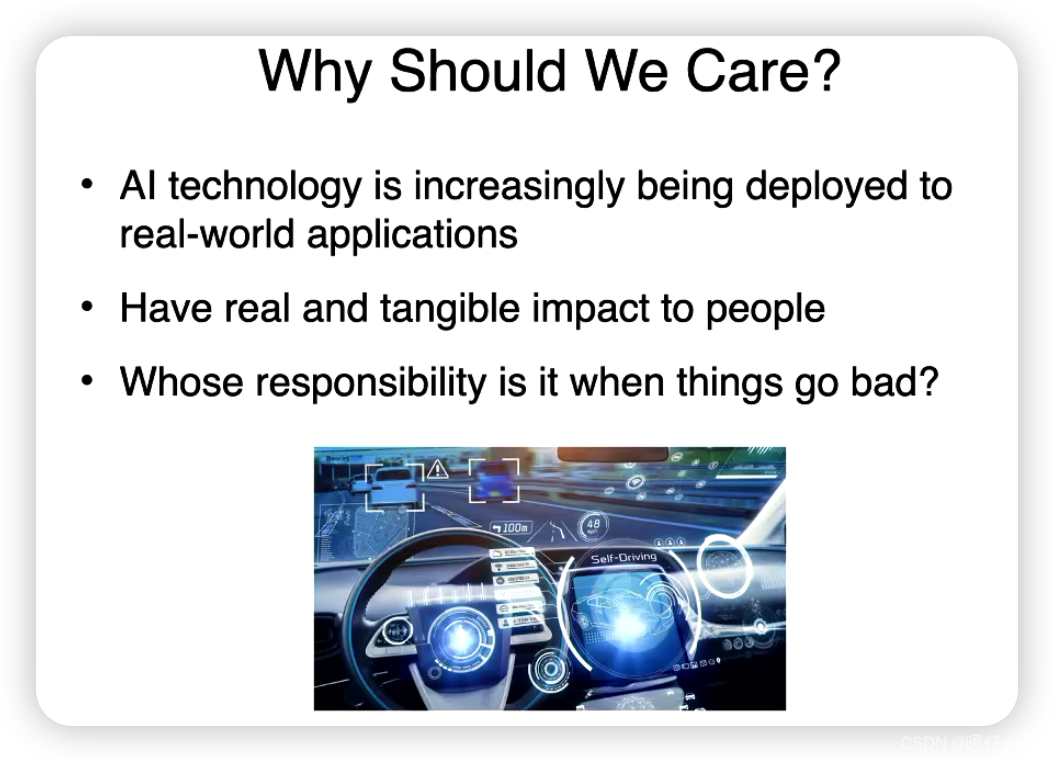
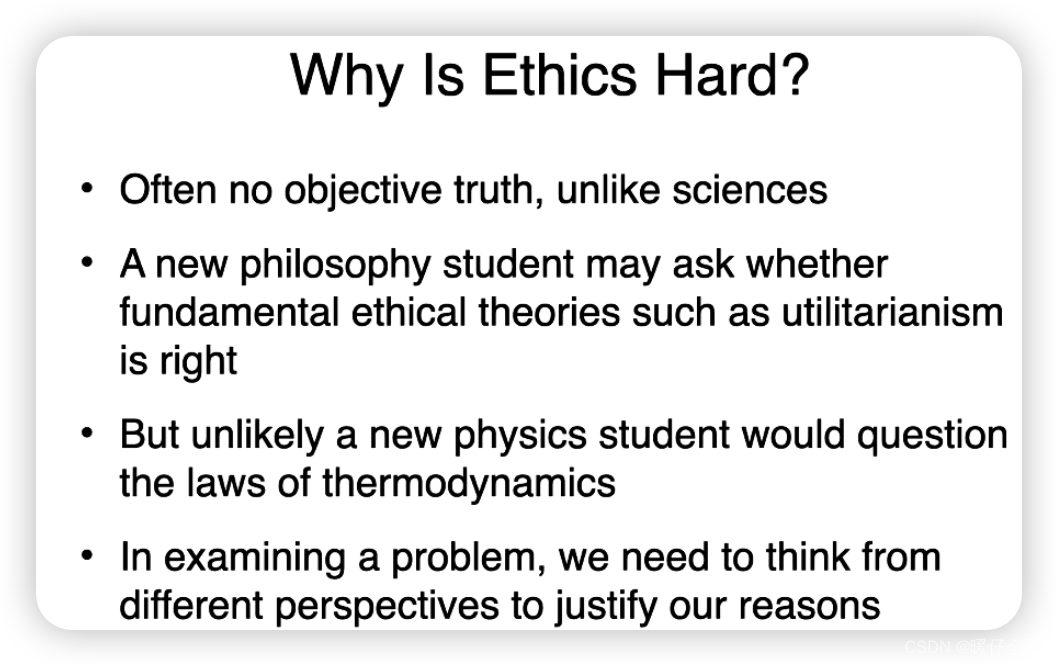
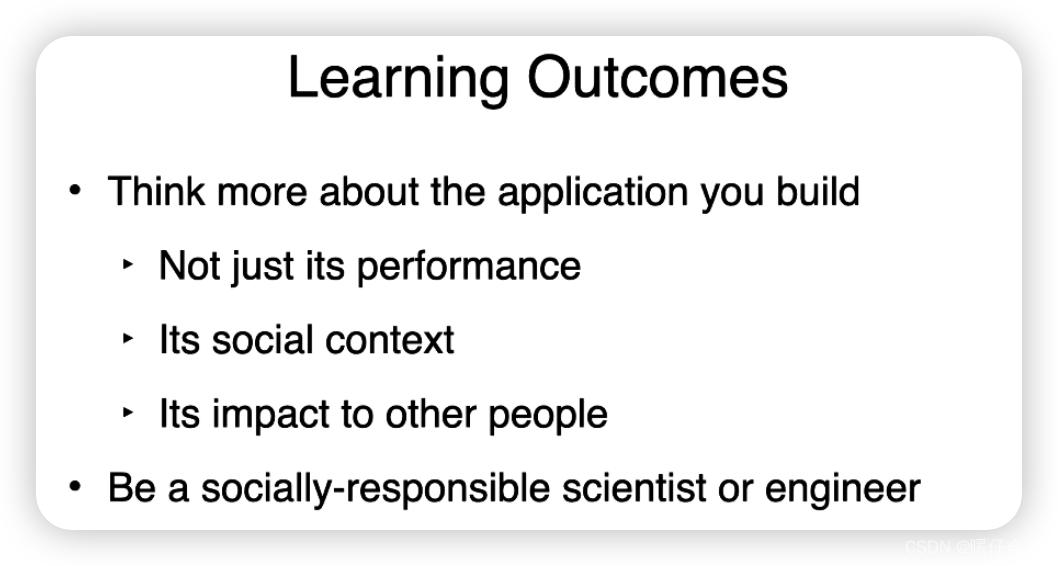
Core NLP ethics concepts
The ethics of natural language processing (NLP) is an increasingly important field that touches on many issues related to issues of fairness, transparency, privacy, and bias. Here are some major ethical issues:
-
Data bias: NLP systems are usually trained by learning a large amount of language data. If these data contain biases, such as gender, race, age, religion, etc., these biases may be learned and amplified by the NLP system, thereby affecting the decision-making and recommendation of the system.
-
Privacy protection: In NLP, the user's language data is usually used to train the model. If it is not properly processed and protected, the user's private information may be leaked.
-
Transparency and Interpretability: NLP models, especially deep learning models, are often viewed as “black boxes,” making it difficult to understand how they work and make decisions. This can lead to an inability to effectively audit and govern the model's decisions.
-
Ethical responsibility for generated content: With the development of generative models such as GPT, NLP systems can now generate very realistic text. This may be used to create fake news, false information, etc., and have a negative impact on society.
-
Fairness: NLP systems need to ensure that all users are treated fairly, and that different cultures, languages, and dialects should be equally valued and handled.
bias
privacy
Group discussion
Automatic Prison Term Prediction
- Bias: According to different training data, the sentence of a person may be judged differently
- Transparency: the model cannot provide specific reasons why such judgment criteria are set
Automatic CV Processing
- bias: This thing may be racially biased
- Transparency: Also need to explain why a candidate was eliminated
- privacy: The company that designed the model will get the data of these past candidates, which is detrimental to the privacy of others
Language Community Classification
Privacy and Labeling: An individual's sexual orientation is sensitive information that should not be made public or revealed without others knowing. Although the goal of this tool may be to understand how the languages of the two communities differ, there may be a risk of misuse, leading to some people's sexual orientation being exposed.
Prejudice and Discrimination: Labeling of language can trigger and reinforce prejudice and discrimination. If a tool labels specific language idioms or expressions as LGBTQ or straight, this could lead to inaccurate or biased perceptions of people who speak those languages.
Oversimplification and stereotyping: Sexual orientation is not the only factor that affects an individual's language use. Classifying language as "LGBTQ" or "heterosexual" may oversimplify the complexity and diversity of language, ignoring the differences between individuals, as well as the influence of other factors such as culture, geography, age, education, etc.
Misinterpretation and misclassification: Tools may not understand and classify language with complete accuracy, which can lead to misunderstanding and misclassification, which can lead to a series of problems, such as misleading research, causing misunderstandings, or harming the misclassified people.