Click on the card below to follow the " CVer " public account
AI/CV heavy-duty information, delivered as soon as possible
Click to enter -> [Image Segmentation and Transformer] communication group
Author: Uno Whoiam (Source: Zhihu, authorized) | Editor: CVer public account
https://zhuanlan.zhihu.com/p/656570195
Reply in the background of CVer WeChat public account: Dark light instance segmentation, you can download the pdf, code and data set of this paper
Instance Segmentation in the Dark
Unit: Beijing Institute of Technology & Princeton
Author: Linwei Chen · Ying Fu · Kaixuan Wei · Dezhi Zheng · Felix Heide
Paper: arxiv.org/abs/2304.14298
https://link.springer.com/article/10.1007/s11263-023-01808-8
Code: https://github.com/Linwei-Chen/LIS
TL;DR Too long to read
This paper opens up a new research direction on instance segmentation in low-light conditions. It is the first paper that systematically establishes a training and testing verification framework for instance segmentation under low-light conditions (emphasis added, it can be a new direction for volume!).
This paper collects and produces a Low-light Instance Segmentation (LIS) data set, which includes four sets of data of low light, normal exposure, paired JPEG and RAW, and provides 8 types of instance pixel annotations, which can be used for instance segmentation, Target detection task. (New dataset!)
This paper observes that RAW images have better potential than JPEG images to achieve higher instance segmentation accuracy, and the author further analyzes that this is related to the fact that RAW can provide more bit-depth information (RAW is all you need!).
This paper observes that under dark light conditions, image noise will cause high-frequency disturbances to the features in deep neural networks, which is an important reason why existing instance segmentation methods perform poorly under dark light conditions (Noise is the key !).
How's the effect? Using the method framework of this paper, based on the results of Mask R-CNN-ResNet50, compared with the large model Segment Anything trained on a large amount of data, the method proposed in this paper still performs well.
Summary
Existing instance segmentation techniques mainly target high-quality image input under normal lighting, but their performance drops significantly in extremely low-light environments. In this work, we delve into instance segmentation in low-light conditions and introduce several techniques that significantly improve low-light inference accuracy. The proposed method is based on the observation that noise in low-light images introduces high-frequency interference to the feature maps of neural networks, thereby significantly degrading performance. To suppress this "feature noise", we propose a novel learning method that relies on adaptive weighted downsampling layers, smooth directional convolution blocks, and interference suppression learning. These components effectively reduce feature noise during downsampling and convolution operations, enabling the model to learn perturbation-resistant features. Additionally, we found that high-bit-depth RAW images better retain richer scene information in low-light conditions than typical camera sRGB output, thus supporting the use of RAW input algorithms. Our analysis shows that high bit depth is crucial for low-light instance segmentation. To alleviate the scarcity of annotated RAW datasets, we utilize a low-light RAW compositing pipeline to generate realistic low-light data. Furthermore, to facilitate further research in this direction, we capture a real-world low-light instance segmentation dataset consisting of more than two thousand paired low-light/normal-light images with instance-level pixel-level annotations. Notably, without any image pre-processing, we achieve satisfactory instance segmentation performance (4% higher AP than state-of-the-art competitors) in very low light conditions while providing future Research opens up new opportunities. Our code and datasets are publicly available to the community (https://github.com/Linwei-Chen/LIS).
1. Observation and motivation
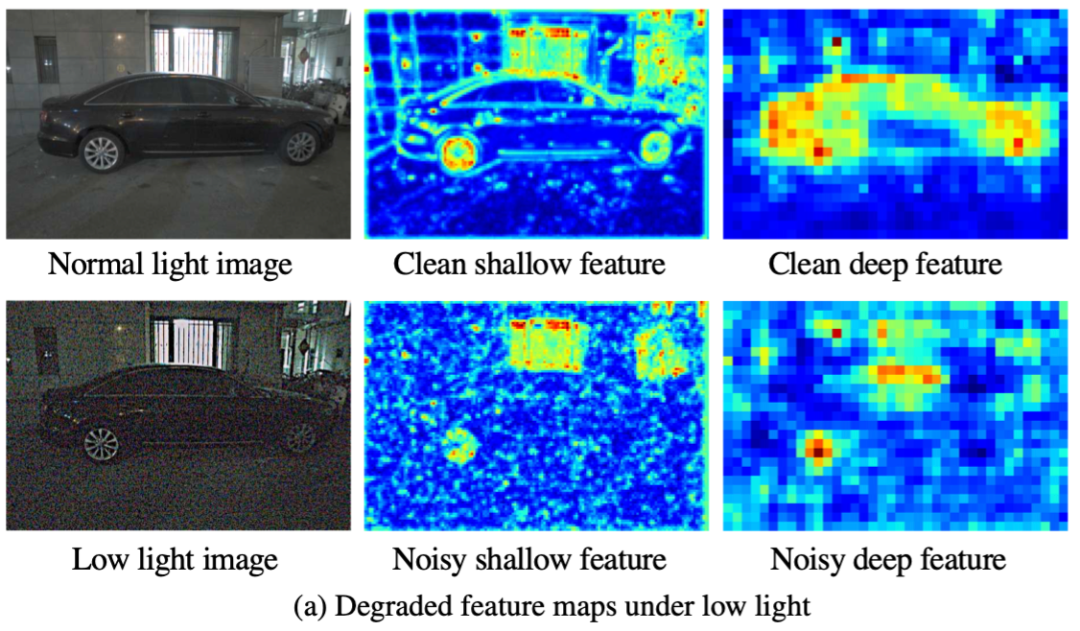
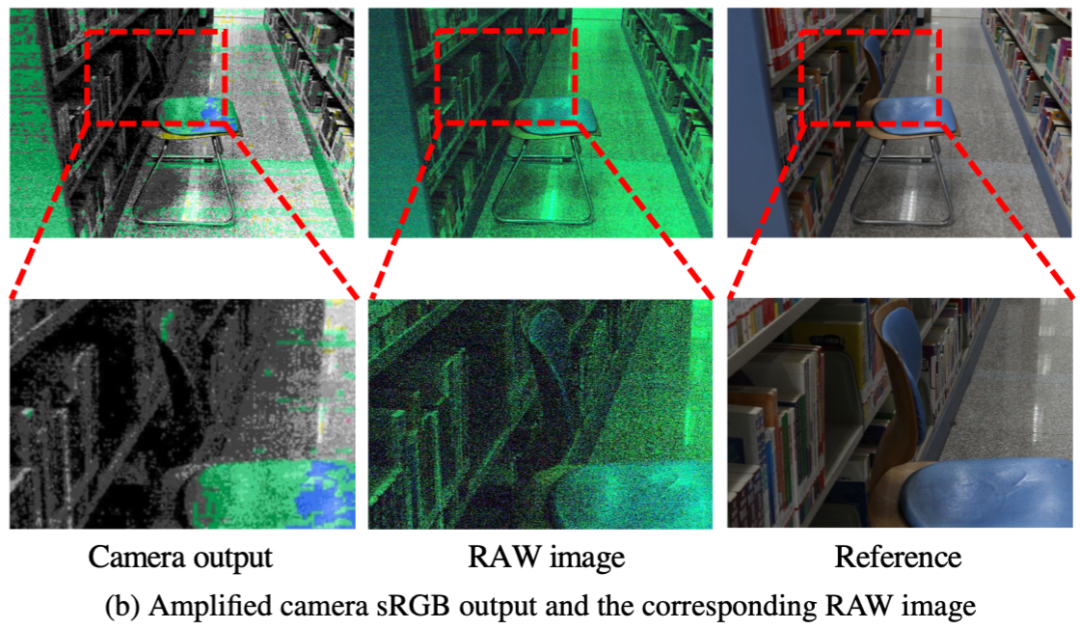
Two key observations:
a. Feature map degradation under low light. For clear normal light images, the instance segmentation network is able to clearly capture both low-level (e.g., edges) and high-level (i.e., semantic responses) features of objects in both shallow and deep layers. However, in noisy low-light images, shallow features may be contaminated and full of noise, while deep features are less semantically responsive to objects.
b. Comparison between camera’s sRGB output and RAW image in darkness. Due to the significantly reduced signal-to-noise ratio, the 8-bit camera output loses a lot of scene information, for example, the seat back structure is almost unrecognizable in the camera output, while it is still identifiable in the RAW image (zoomed in for better detail).
Illustration of our key observations under dark regimes that drive our method design: a Degraded feature maps under low light. For clean normal-light images, the instance segmentation network is able to clearly capture the low-level ( e.g., edges) and high-level ( i.e., semantic responses) features of objects in shallow and deep layers, respectively. However, for noisy low-light images, shallow features can be corrupted and full of noise, and the deep features show lower semantic responses to objects. b Comparison between camera sRGB output and RAW image in the dark. Due to significantly low SNR, the 8-bit camera output loses much of the scene information, for example, the seat backrest structure is barely discernible, whereas is still recognizable in the RAW counter- part (Zoom in for better details)
2. Challenges and methods
The overall approach is as follows
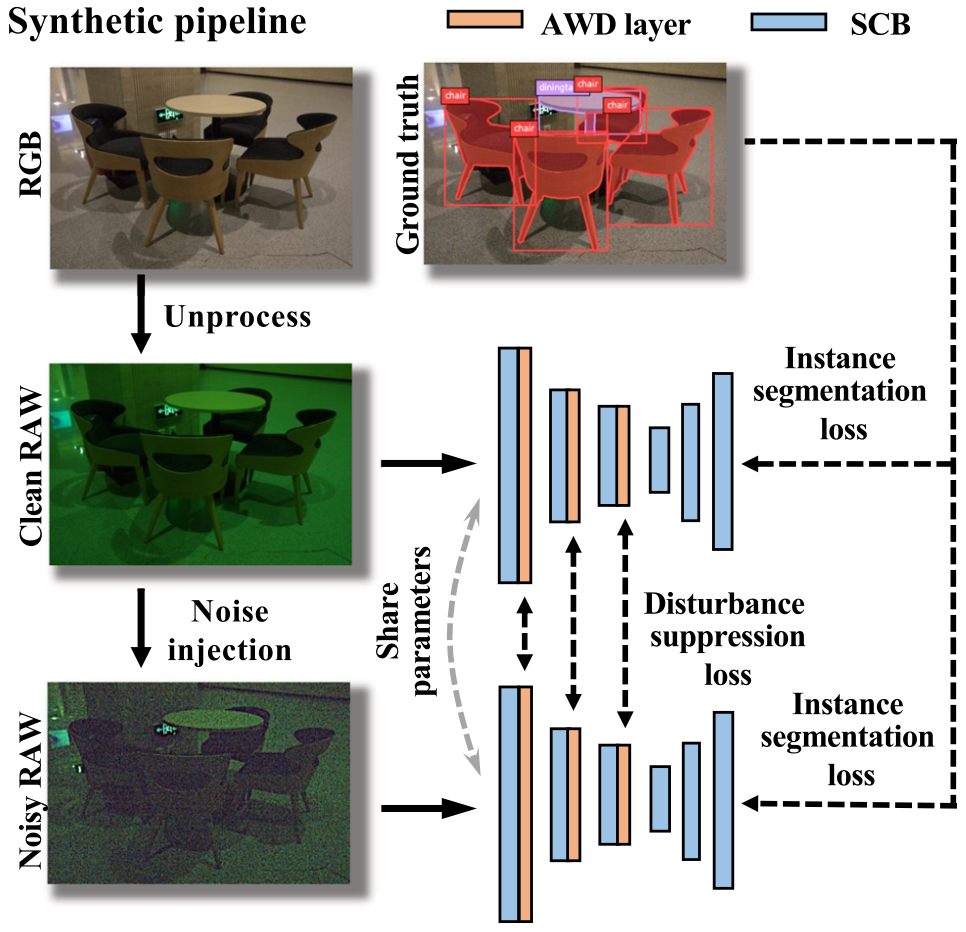
2.1 Low-Light RAW Synthetic Pipeline
Challenge: Training a segmentation model requires massive data with instance segmentation annotations, and currently there is no such data set. It is expensive to collect additional dark-light images and label large-scale dark-light data sets. At the same time, existing RAW data is also very scarce.
Solution: Design a pipeline from RGB images to noisy dark-light RAW images, so that the existing instance segmentation data set can be used to train an instance segmentation model for RAW input under dark-light conditions at zero cost.
Our low-light RAW compositing pipeline consists of two steps, raw and noise injection:
Reverse processing. Collecting large-scale RAW image datasets is expensive and time-consuming, so we considered leveraging existing sRGB image datasets (Everingham et al., 2010; Lin et al., 2014). sRGB images are obtained through a series of image transformation operations of the camera's internal image signal processing (ISP), such as tone mapping, gamma correction, color correction, white balance, and demosaicing. Through unprocessed operations (Brooks et al. 2019), we can invert these image processing transformations and thus obtain RAW images. In this way, we can create a RAW dataset at zero cost.
Noise injection. After obtaining a clean RAW image through unprocessed operations, in order to simulate a real noisy low-light image, we need to inject noise into the RAW image. To produce more accurate complex noise results, we adopt a recently proposed physics-based noise model (Wei et al., 2020, 2021) instead of the widely used Poissonian-Gaussian noise model (i.e., the heteroscedastic Gaussian model (Foi et al., 2008)). The model can accurately describe the real noise structure, taking into account many noise sources, including photon shooting noise, read noise, strip pattern noise and quantization noise.
Our low-light RAW synthetic pipeline consists of two steps, i.e., unproccessing and noise injection. We introduce them one by one.
Unprocessing. Collecting a large-scale RAW image dataset is expensive and time-consuming, hence we consider utilizing existing sRGB image datasets (Everingham et al., 2010; Lin et al., 2014). The sRGB image is obtained from RAW images by a series of image transformations of on-camera image signal processing (ISP), e.g., tone mapping, gamma correction, color correction, white balance, and demosaicking. With the help of the unprocessing operation (Brooks et al., 2019), we can invert these image processing transforma- tions, and RAW images can be obtained. In this way, we can create a RAW dataset with zero cost.
Noise injection. After obtaining clean RAW images by unprocessing, to simulate real noisy low-light images, we need to inject noise into RAW images. To yield more accurate results for real complex noise, we employ a recently proposed physics-based noise model (Wei et al., 2020, 2021), instead of the widely used Poissonian-Gaussian noise model (i.e., heteroscedastic Gaussian model (Foi et al., 2008)). It can accurately characterize the real noise structures bytakingg into account many noise sources, including photon shot noise, read noise, banding pattern noise, and quantization noise.
2.2 Adaptive Weighted Downsampling Layer
Challenge: How to solve the characteristic "noise disturbance" caused by image noise
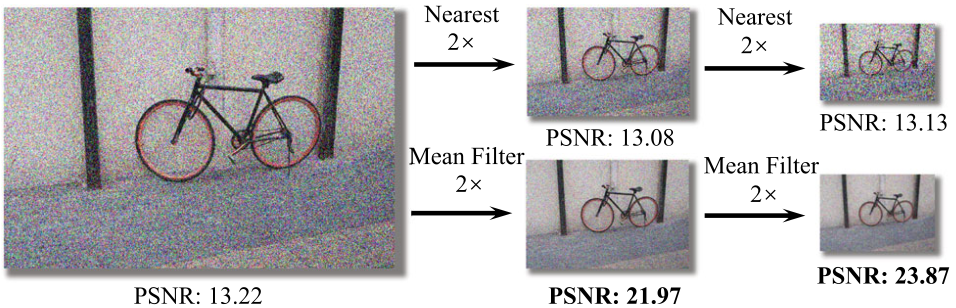
A simple observation is that downsampling the image using low-pass filtering reduces the noise level due to the smooth a priori nature of the image.
Considering that existing networks often have multiple downsampling layers, wouldn't it be possible to take full advantage of these downsampling processes? Experiments have shown that simply inserting a mean filter can achieve almost no-cost performance in dark-light instance segmentation.
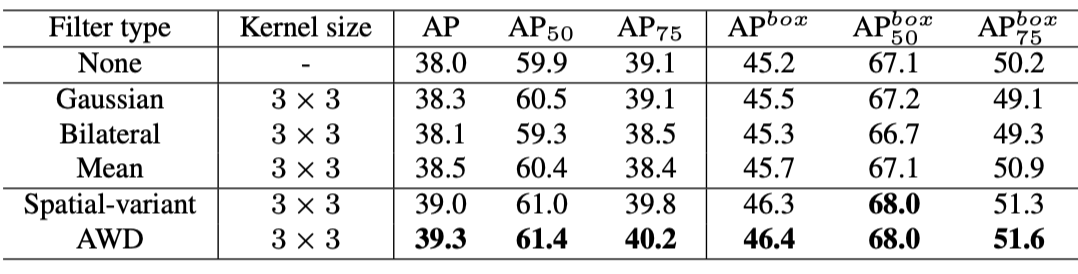
Although effective, fixed filters such as mean filter cannot be adaptively adjusted according to features, and thus may erase detailed information. In this regard, the author proposes Adaptive Weighted Downsampling Layer, AWD, which adaptively predicts low-pass filtering on features channel by channel and point by point. This increases the intensity of the low-pass in the noisy areas, while reducing the low-pass level in the detail areas to preserve details.
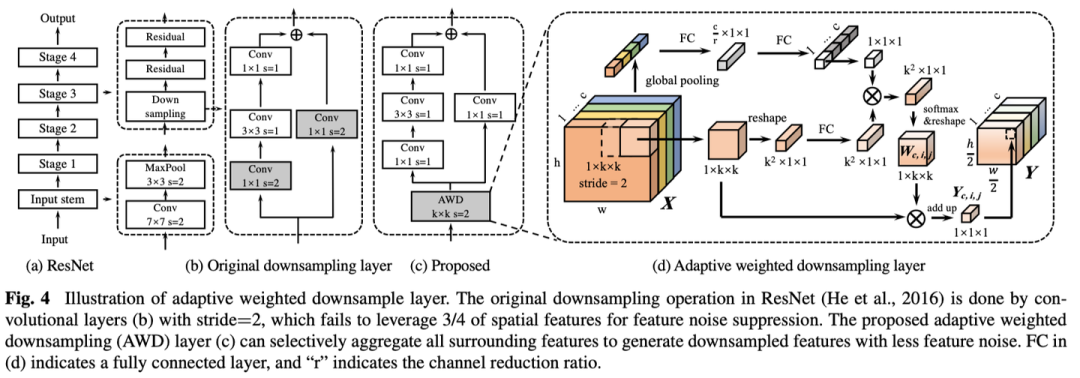
After looking at the source code, FC is replaced by Depth-wise, and the effect is equivalent. The formulas are not listed here. If you are interested, you can read the original text.
2.3 Smooth-Oriented Convolutional Block
In order to further reduce the disturbance of features by high-frequency noise in images, the author also proposed to use re-parameterization technology to simultaneously train a set of smooth convolution kernels during training and fuse them into the original convolution kernels during inference. In this way Convolution is more robust in the face of noise. It is worth noting that this does not increase the amount of parameters and calculations during inference! Pure prostitution!
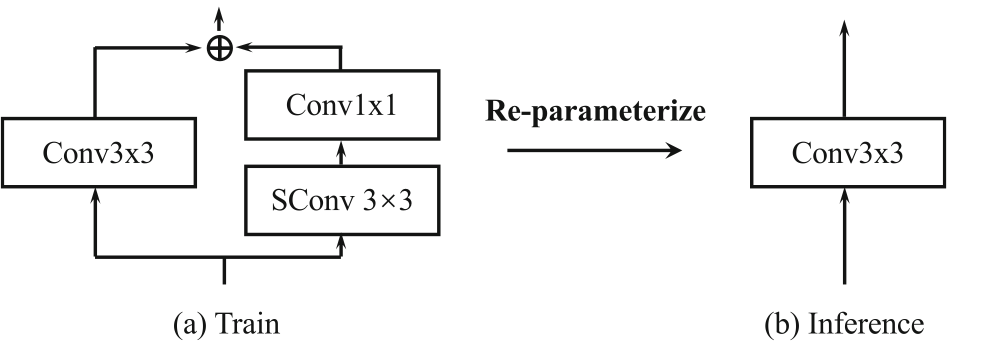
2.4 Disturbance Suppression Learning
At the same time, the author also made some adjustments during model learning, allowing the model to learn clean and noisy images at the same time, and constraining the features of the input to be noisy images to be closer to clean images, which is somewhat similar to knowledge distillation, but does not require a teacher. This not only improves the robustness of the model under dark light, but also improves images with normal lighting. This is very consistent with the actual application scenario, that is, a model can cope with both day and night applications.
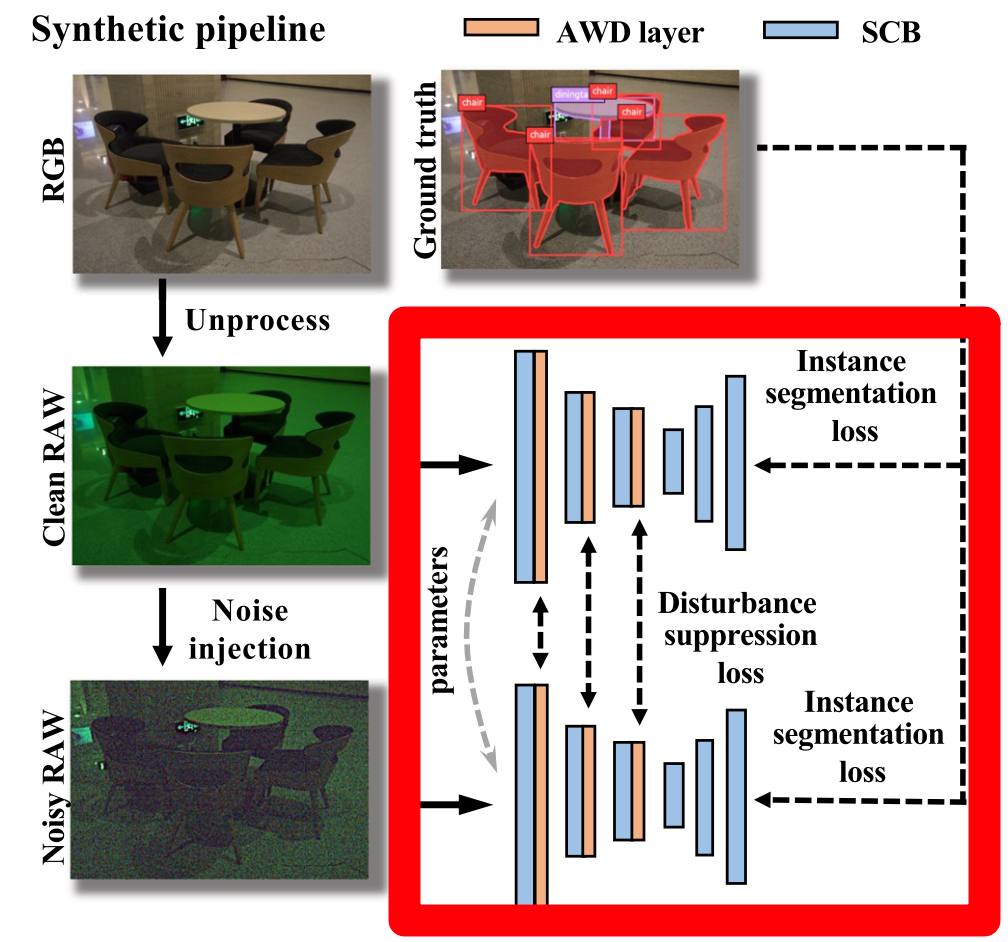
3. LIS data set
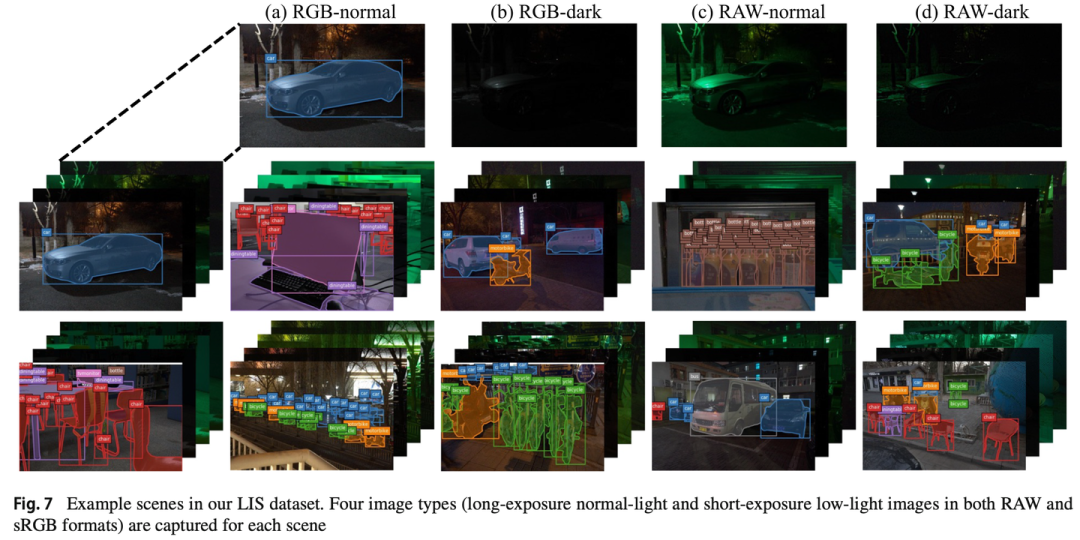
The data set was captured by Canon EOS 5D Mark IV and has the following characteristics:
- Paired samples. In the LIS dataset, we provide images in sRGB-JPEG (typical camera output) and RAW formats, each format including paired short-exposure low-light and corresponding long-exposure normal-light images. We refer to these four types of images as sRGB-Dark, sRGB-Normal, RAW-Dark and RAW-Normal. To ensure they were aligned at the pixel level, we mounted the camera on a sturdy tripod and controlled it remotely via a mobile app to avoid vibration.
- Diverse scenarios. The LIS dataset consists of 2230 pairs of images collected in various scenes, including indoors and outdoors. To add variety to low-light conditions, we shot long-exposure reference images using a range of ISO levels (e.g. 800, 1600, 3200, 6400), and deliberately through a range of low-light factors (e.g. 10, 20, 30, 40, 50 , 100) Reduce exposure time and shoot short exposure images to simulate very low light conditions.
- Instance-level pixel-level labels. For each pair of images, we provide accurate instance-level pixel-level labels annotating instances of the 8 most common object categories in our daily lives (bicycles, cars, motorcycles, buses, bottles, chairs, dining tables, televisions) . We note that LIS contains images taken in different scenes (indoor and outdoor) and under different lighting conditions. In Figure 7, object occlusion and densely distributed objects make LIS more challenging outside of low-light conditions.
– Paired samples. In the LIS dataset, we provide images in both sRGB-JPEG (typical camera output) and RAW formats, each format consists of paired short-exposure low-light and corresponding long-exposure normal-light images. We term these four types of images as sRGB- dark, sRGB-normal, RAW-dark, and RAW-normal. To ensure they are pixel-wise aligned, we mount the camera on a sturdy tripod and avoid vibrations by remote control via a mobile app.
– Diverse scenes. The LIS dataset consists of 2230 image pairs, which are collected in various scenes, including indoor and outdoor. To increase the diversity of low-light conditions, we use a series of ISO levels ( e.g., 800, 1600, 3200, 6400) to take long-exposure reference images, and we deliberately decrease the exposure time by a series of low-light factors ( e.g., 10, 20, 30, 40, 50, 100) to take short-exposure images for simulating very low-light conditions.
– Instance-level pixel-wise labels. For each pair of images, we provide precise instance-level pixel-wise labels annotate instances of 8 most common object classes in our daily life (bicycle, car, motorcycle, bus, bottle, chair, dining table, tv). We note that LIS contains images captured in different scenes (indoor and outdoor), and different illuminationconditionss. In Fig.7, object occlusion and densely distributed objects make LIS more challenging besides the low light.
4. Experimental results
3.1 Ablation
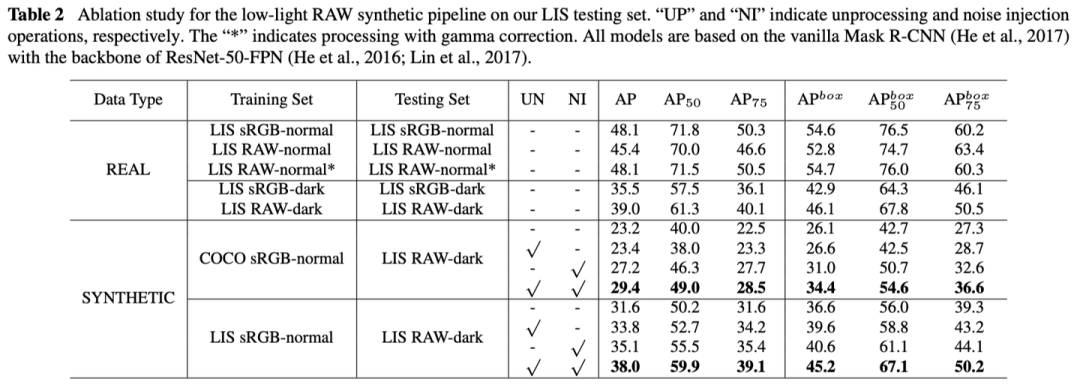
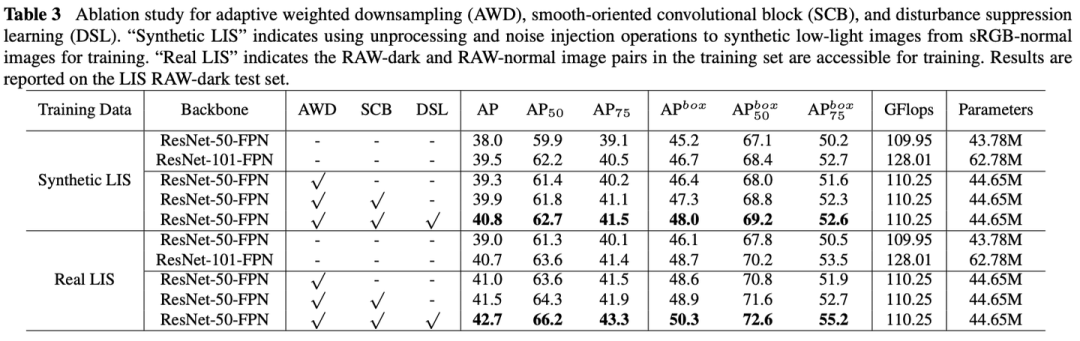
3.2 Main results
It provides the use of Mask R-CNN, PointRend, Mask2Former, Faster R-CNN methods and the backbone network ResNet-50, Swin-Transformer, and ConvNeXt to prove the effectiveness on two tasks: instance segmentation and target detection:
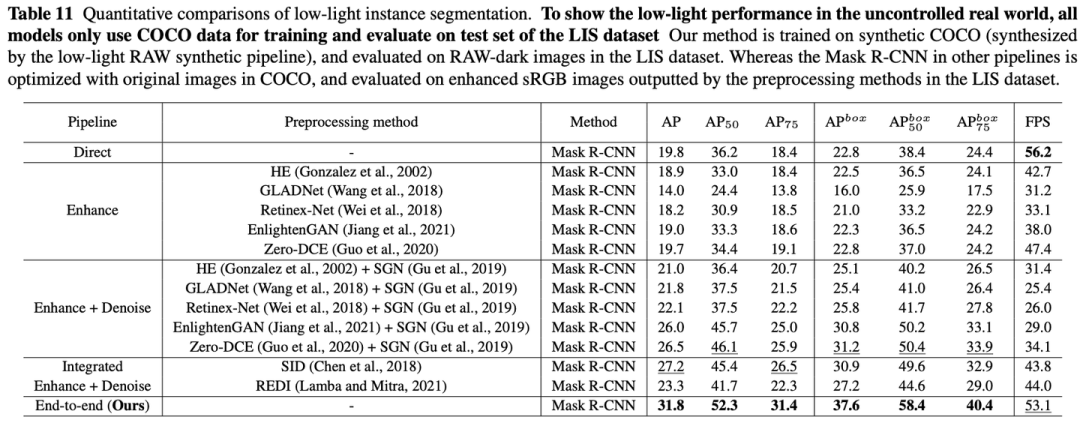
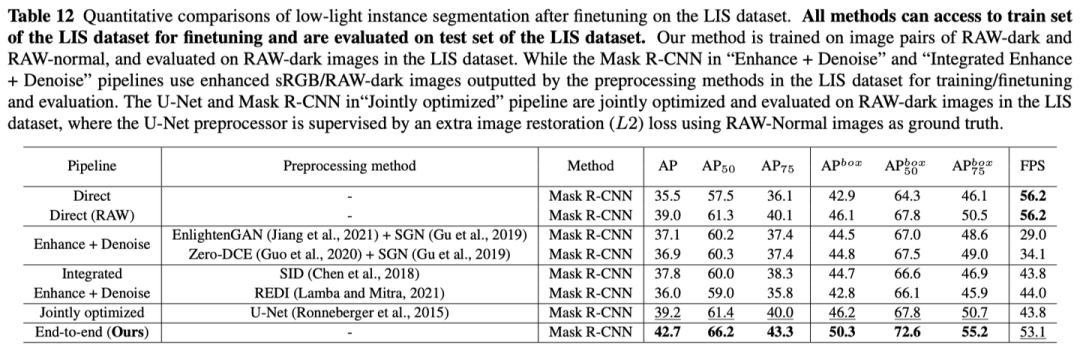
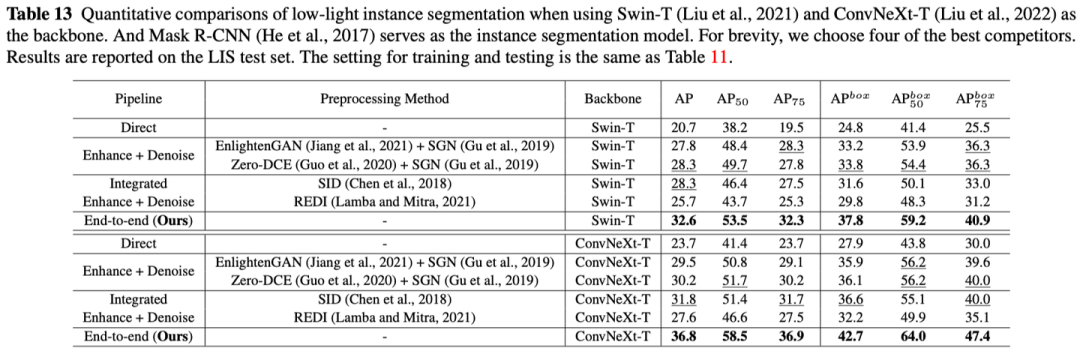
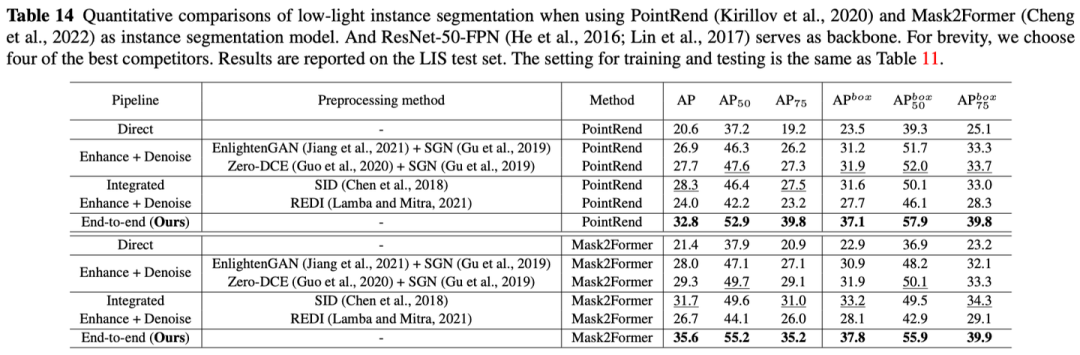
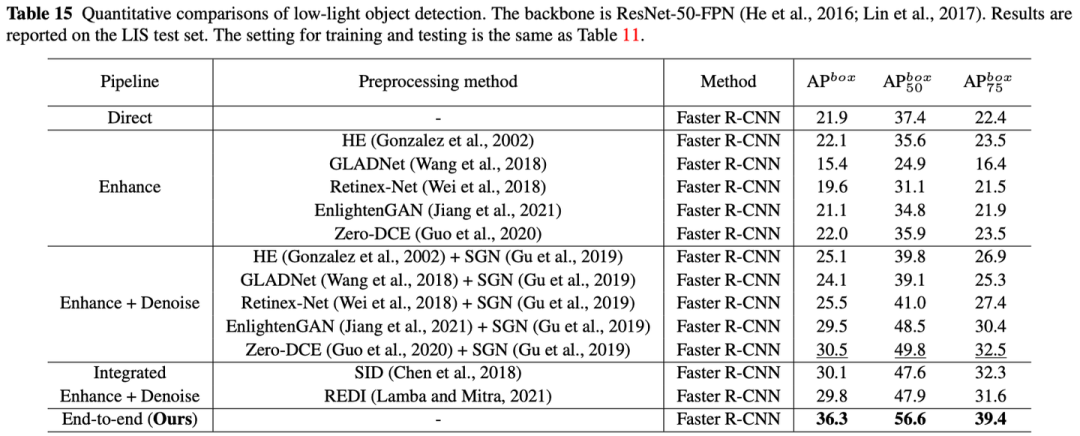
3.3 Visualization results
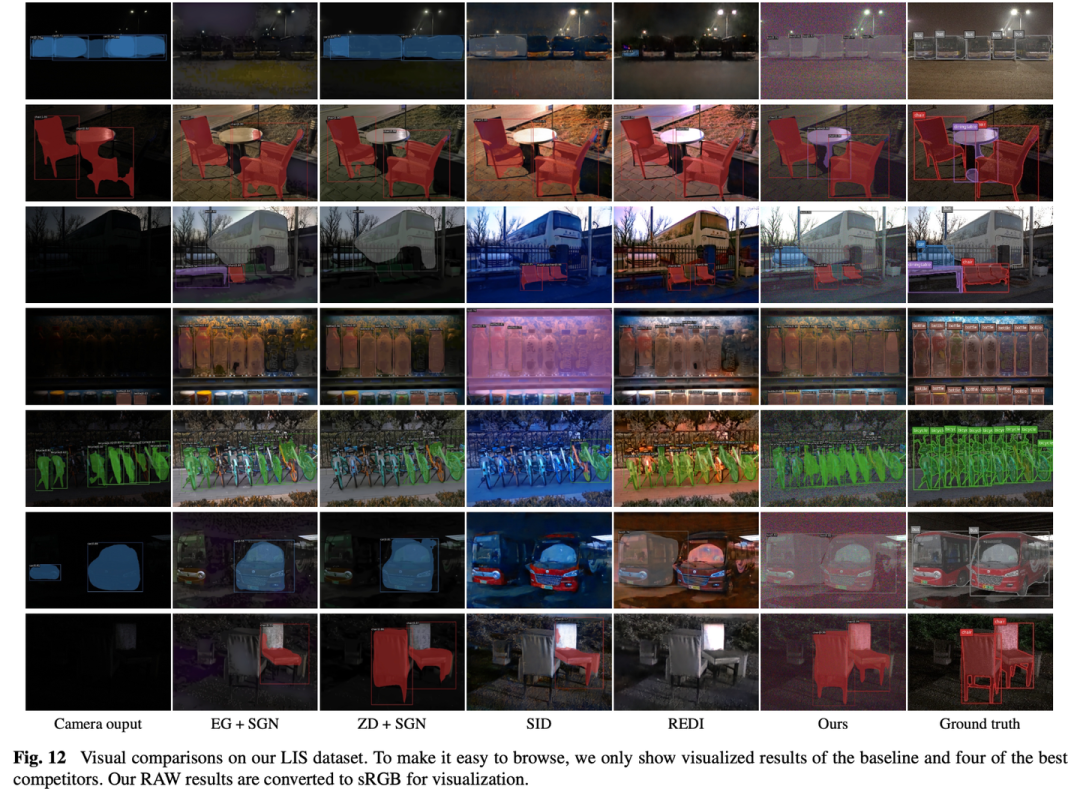
5. Inspiration
Detection and segmentation is a hot mainstream research direction, and papers are emerging one after another. If you want to explore in the red ocean, the best way is to create a blue ocean yourself. This paper finds a new application scenario for instance segmentation, builds a framework for instance segmentation training and verification under dark light conditions, and obtains a series of simple but effective methods from the observation of key phenomena. It can be seen that observation is the basis of scientific research. Good observation can allow people to discover interesting phenomena and problems, thereby leading to new problems and opening up new paths.
Reply in the background of CVer WeChat public account: Dark light instance segmentation, you can download the pdf, code and data set of this paper
Click to enter -> [Image Segmentation and Transformer] communication group
ICCV/CVPR 2023 paper and code download
Backstage reply: CVPR2023, you can download the collection of CVPR 2023 papers and code open source papers
后台回复:ICCV2023,即可下载ICCV 2023论文和代码开源的论文合集
图像分割和Transformer交流群成立
扫描下方二维码,或者添加微信:CVer333,即可添加CVer小助手微信,便可申请加入CVer-图像分割或者Transformer 微信交流群。另外其他垂直方向已涵盖:目标检测、图像分割、目标跟踪、人脸检测&识别、OCR、姿态估计、超分辨率、SLAM、医疗影像、Re-ID、GAN、NAS、深度估计、自动驾驶、强化学习、车道线检测、模型剪枝&压缩、去噪、去雾、去雨、风格迁移、遥感图像、行为识别、视频理解、图像融合、图像检索、论文投稿&交流、PyTorch、TensorFlow和Transformer、NeRF等。
一定要备注:研究方向+地点+学校/公司+昵称(如图像分割或者Transformer+上海+上交+卡卡),根据格式备注,可更快被通过且邀请进群
▲扫码或加微信号: CVer333,进交流群
CVer计算机视觉(知识星球)来了!想要了解最新最快最好的CV/DL/AI论文速递、优质实战项目、AI行业前沿、从入门到精通学习教程等资料,欢迎扫描下方二维码,加入CVer计算机视觉,已汇集数千人!
▲扫码进星球
▲点击上方卡片,关注CVer公众号
It’s not easy to organize, please like and watch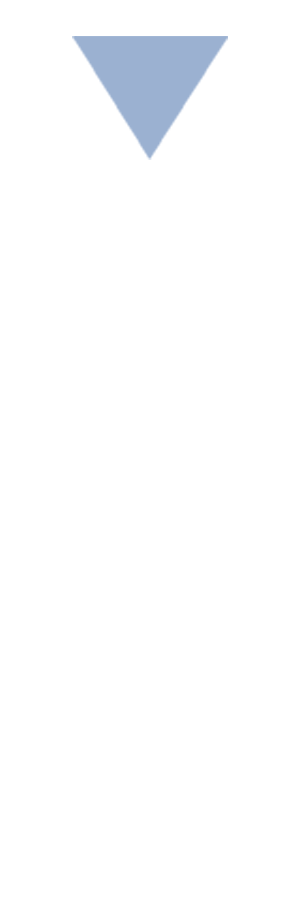