Summary
Since the tutorial is relatively long, it is not recommended to practice it, just read it to understand and learn.
Before running a likelihood-based phylogenetic analysis, the user needs to decide which free parameters should be included in the model: whether a single rate should be assumed for all substitutions (like the Jukes-Cantor model of sequence evolution) or whether different transition rates and disruptions should be allowed. Exchange rate (such as HKY model). Or should different ratios be used for all substitutions (as in the GTR model) should the frequencies of the four nucleotides ("state frequencies") be estimated or assumed to be all equal? The optimal number of free model parameters depends on the available data and can be chosen based on criteria such as the Akaike Information Criterion (AIC), which seeks to balance improvement in model fit with the number of additional parameters required for model fit. .
In this tutorial [1] I will describe how to select alternative models for phylogenetic analysis using the software PAUP* (Swofford 2003), a popular and versatile tool for various types of phylogenetic analyses.
data set
The data used in this tutorial is a filtered version of the alignment generated for the 16s and RAG1 sequences in the tutorial multiple sequence alignment. Since PAUP* requires Nexus format alignment as input, use the files 16s_filtered.nex and rag1_filtered.nex.
PAUP*
Originally developed in the late 1980s, this software is one of the oldest phylogenetic analysis programs, and although it has been around for a long time, its author Dave Swofford never released a final version. Although PAUP* has long been surpassed in speed by other programs for likelihood-based phylogenetic inference, it remains important for the variety of other functions it contains. Not long ago, PAUP* could only be purchased from Sinauer Associates for around $100. Since 2015, Dave Swofford has been distributing the updated version of PAUP* 4.0 for free as a trial version on his new PAUP* website. These trials expire after a few months, so you may need to download them again if you want to use PAUP in the future. This situation may only be temporary, as development of PAUP 5 is ongoing and the product will be at least partially commercially distributed.
Although the descriptions in this tutorial assume that you have installed the graphical user interface (GUI) version of PAUP* for Mac OS X or Windows, you can also install the command line version of PAUP*, which is required on Linux or Mac OS X Catalina or newer, as no GUI currently exists for these systems. If you are using the command line version, you may need to look up the equivalent command; this can always be done through PAUP*'s help screen after starting PAUP*, which can be displayed by typing "?". and press Enter. The screenshot below shows the help screen for the command line version of PAUP*.
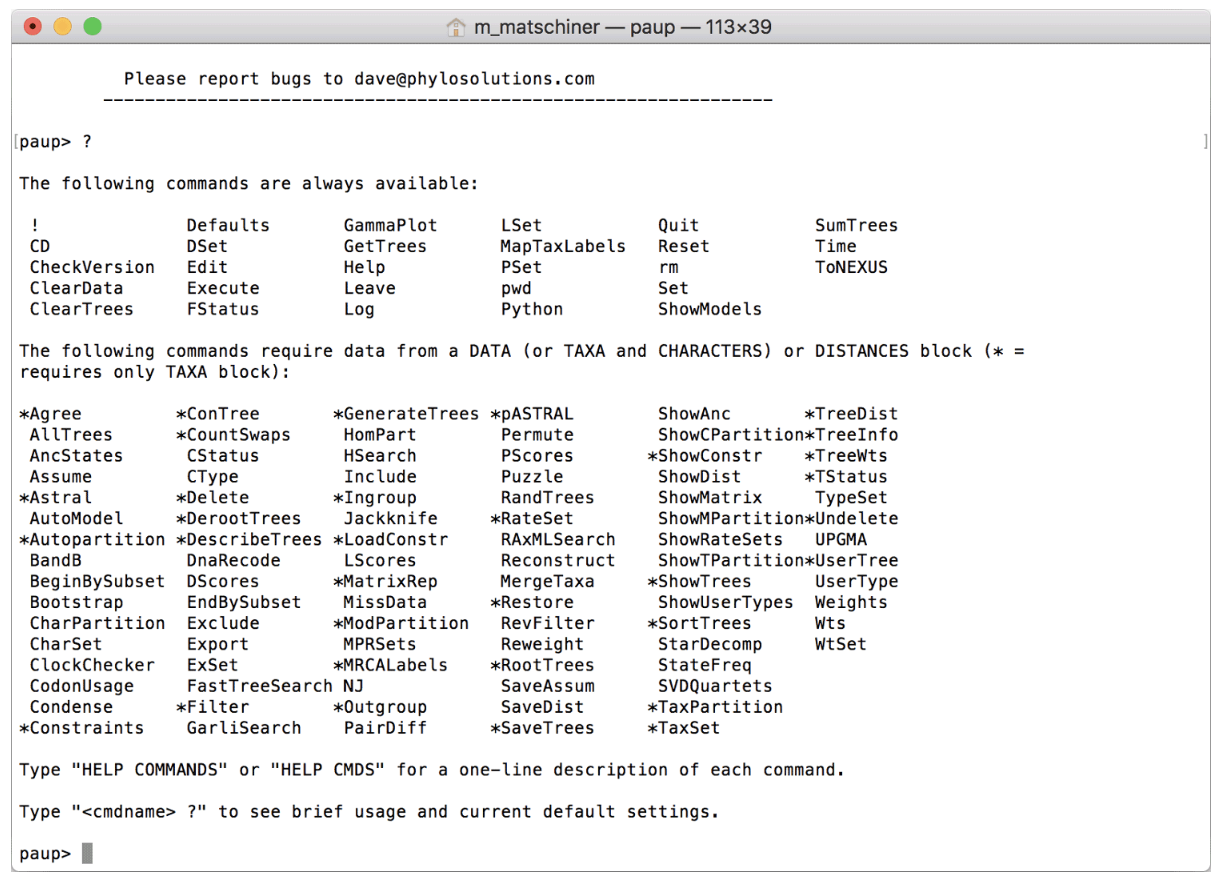
Model selection and phylogenetic inference
Comparisons of the fit of substitution-based models to sequence data have been implemented in a variety of tools and are most commonly performed using the program jModelTest. But since automatic selection of alternative models has recently been implemented in PAUP*, and the other tutorials in this repository require PAUP to be installed anyway , I'm using PAUP here instead of jModelTest for model selection. In fact, the model selection between the two programs is very similar.
-
单击 PAUP* 的“文件”菜单中的“打开...”。确保在打开的窗口底部选择“执行”作为初始模式,如下一个屏幕截图所示。选择 Nexus 格式的 16s 序列对齐文件 (16s_filtered.nex),然后单击“打开”。 PAUP* 将给出其对该文件的解释的简短报告,包括在比对中发现的物种(分类单元)和字符的数量。
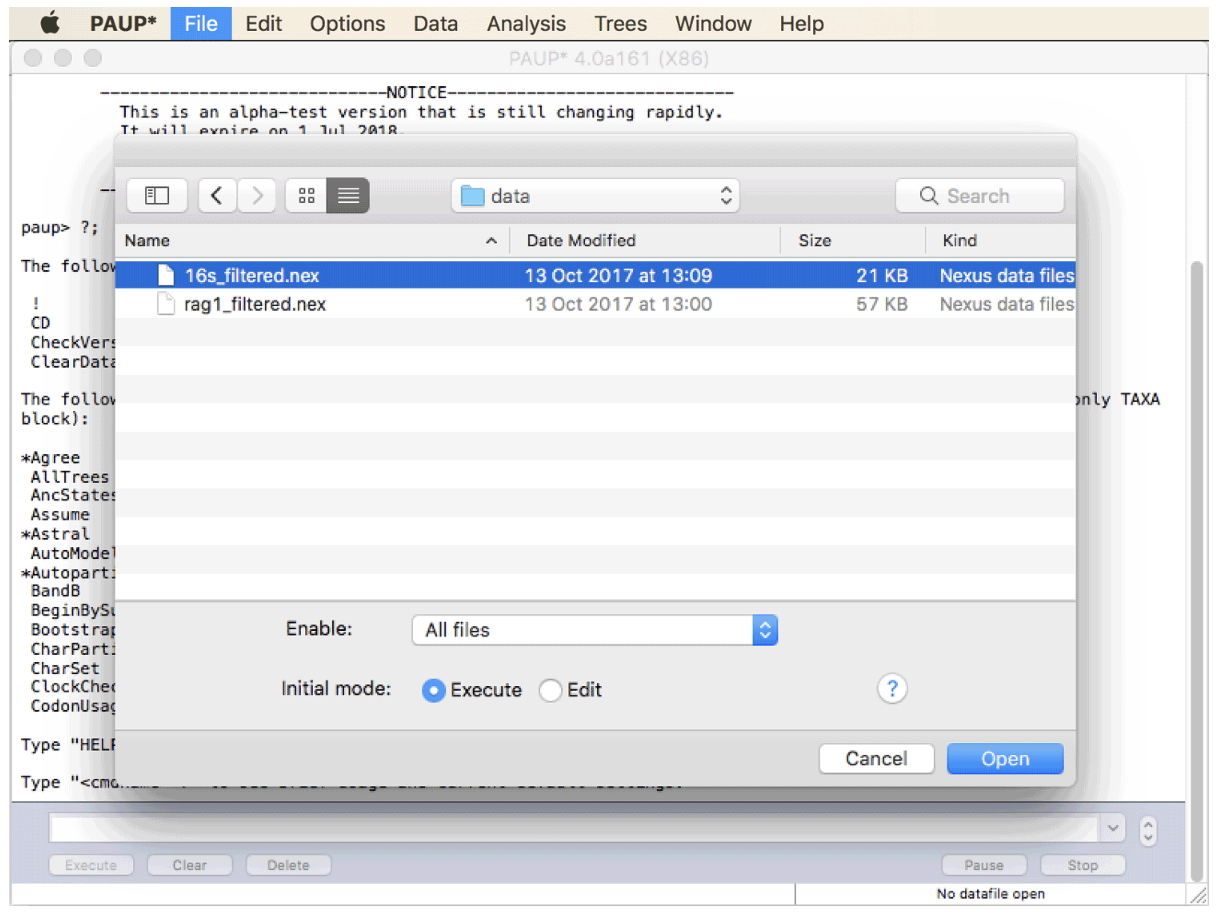
-
“自动模型选择”选项可以在 PAUP* 的“分析”菜单中找到。但是,当您单击它时,您会看到为了运行此模型选择,需要系统发育。虽然这可能看起来可能会导致循环推理(选择替代模型是最大似然系统发育分析所必需的,但也取决于系统发育),但这在实践中不是问题,因为模型选择的结果并不强烈依赖于正确的系统发育;因此,任何合理的系统发育都会导致相似的模型选择结果。因此,最好的解决方案是使用 Neighbor-Joining 算法运行快速系统发育分析,该算法也可以在 PAUP* 中方便地实现。
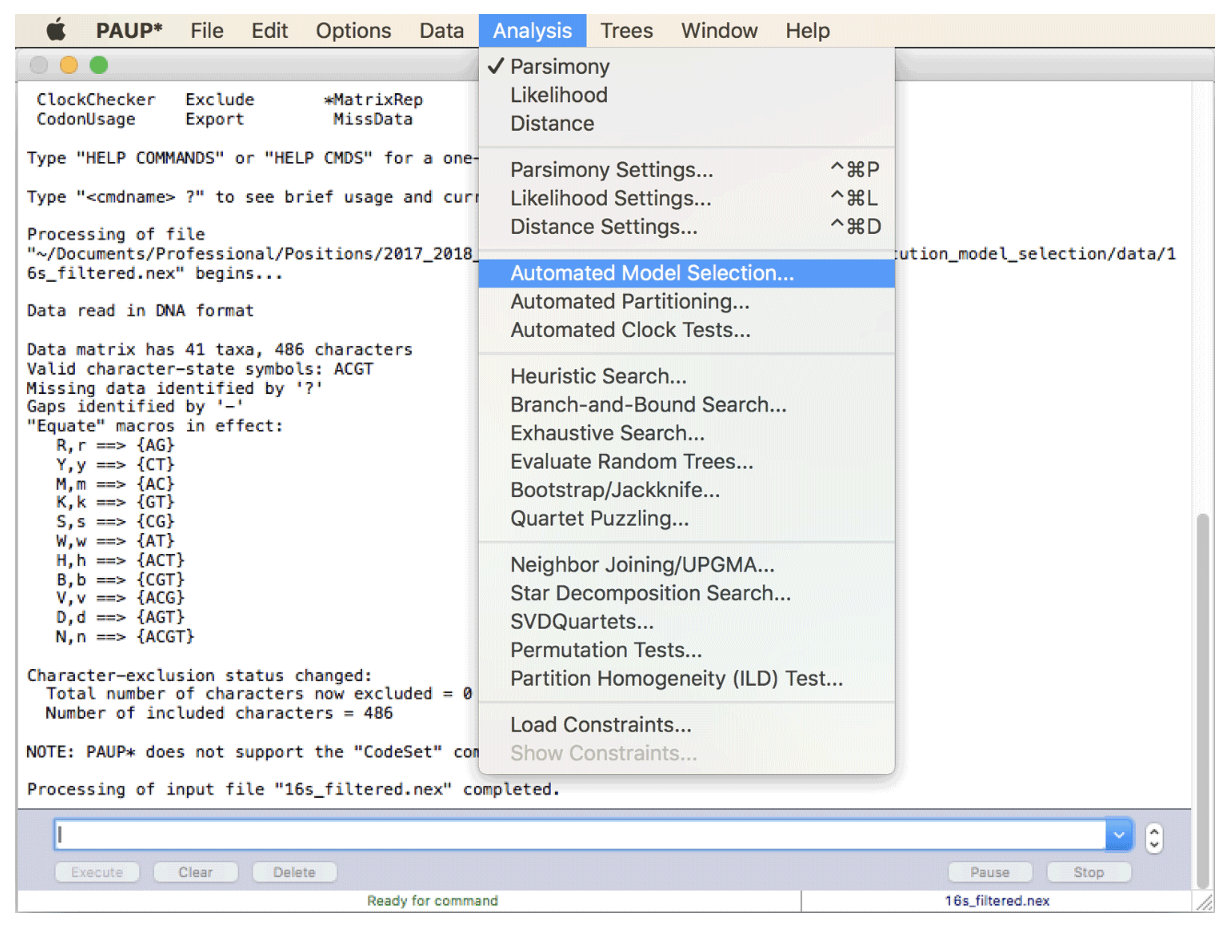
-
要从邻接系统发育分析的可用设置中进行选择,请单击 PAUP* 的“分析”菜单中的“邻接/UPGMA...”,如下面的屏幕截图所示。
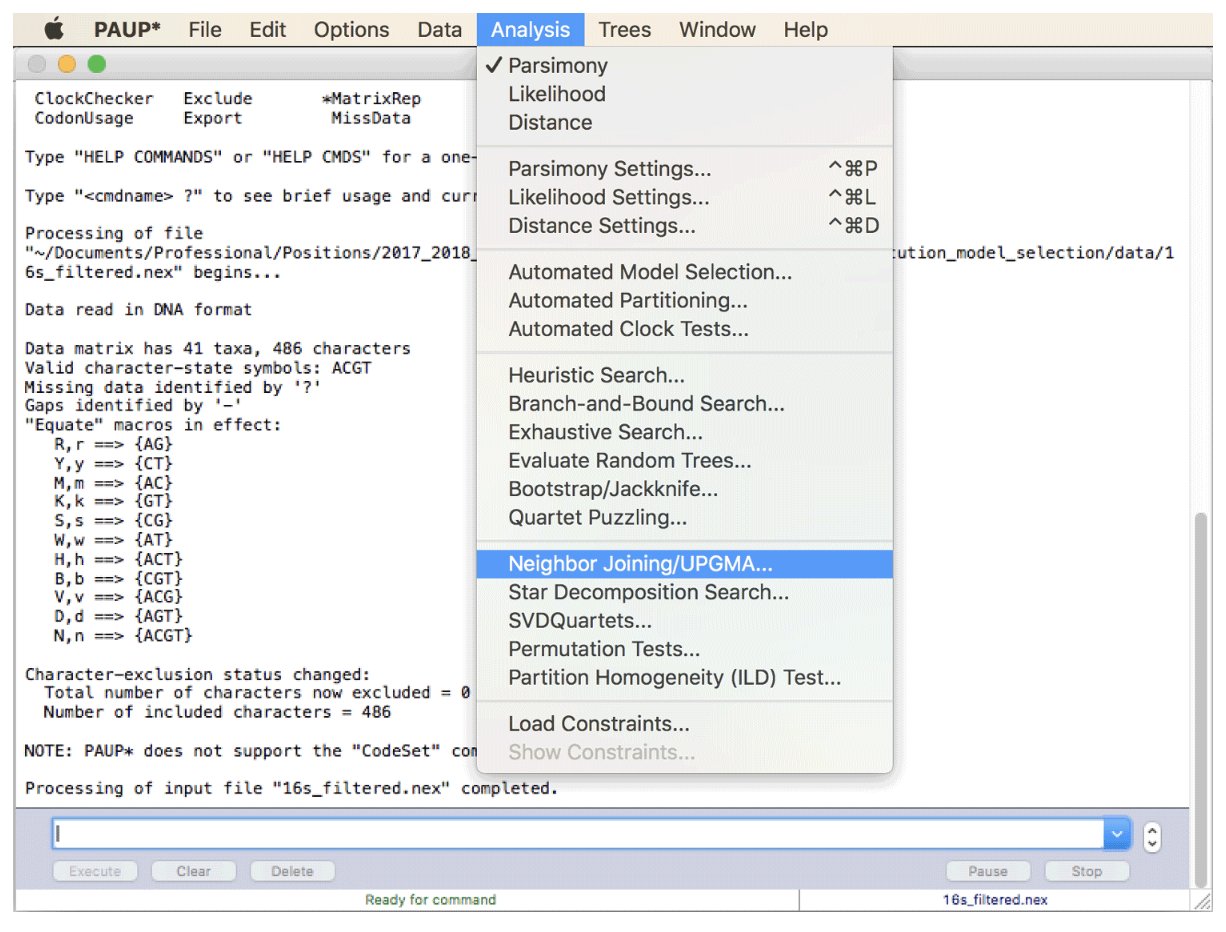
-
在新打开的弹出窗口中,保留所有默认选项并单击“确定”(PAUP* 命令行版本中的等效命令只是 NJ;)。 -
再次单击“分析”菜单中的“自动模型选择...”。使用邻接生成的树将已被选择用于模型选择,弹出窗口现在将为您提供用于此模型选择的多个选项。模型选择的可用标准称为“AIC”、“AICc”、“BIC”和“DT”。这些与似然比检验类似,但优点是它们可用于比较非“嵌套”模型(如果其中一个模型具有其他模型的所有参数加上附加参数,则两个模型是嵌套的)。 “AIC”代表“Akaike信息准则”,“AICc”是“针对小样本量校正的Akaike信息准则”,“BIC”是“贝叶斯信息准则”,“DT”是“决策理论”标准。其中最常用的是 Akaike 信息准则。每个模型的 AIC 独立计算为 AIC = 2 k −2 log(L),其中 k 是模型中自由参数的数量,L 是所有自由参数优化后数据的可能性(即最大可能性)。通常,如果一个模型的 AIC 分数比另一个模型的 AIC 分数好(= 小)至少 4 分,则该模型被认为优于另一个模型。设置“AIC”旁边的勾号,但删除“AICc”、“BIC”和“DT”旁边的勾号。另请选择“应用选择模型的设置:”右侧的“AIC”。作为“模型集”,选择数字“3”。这意味着将测试具有相等替代率的模型(例如 Jukes-Cantor 模型)、具有单独的转换和颠换替代率的模型(例如 HKY 模型)以及具有六个独立替代率的模型(GTR 模型)。保留“等速率”和“gamma”旁边的勾号(允许站点间速率变化的伽玛分布),但删除“invar.sites”和“两者”的勾号。我建议这样做,因为不变位点比例(“+I”)和位点间速率变化(“+G”)的参数很混乱,因为对一组位点应用特别低的速率几乎具有相同的效果。考虑到这些站点的效果完全不变。保留“显示每个模型的输出”旁边的勾号,并设置“显示每个模型的参数估计”旁边的勾号。确保设置面板如下面的屏幕截图所示,然后单击“确定”。
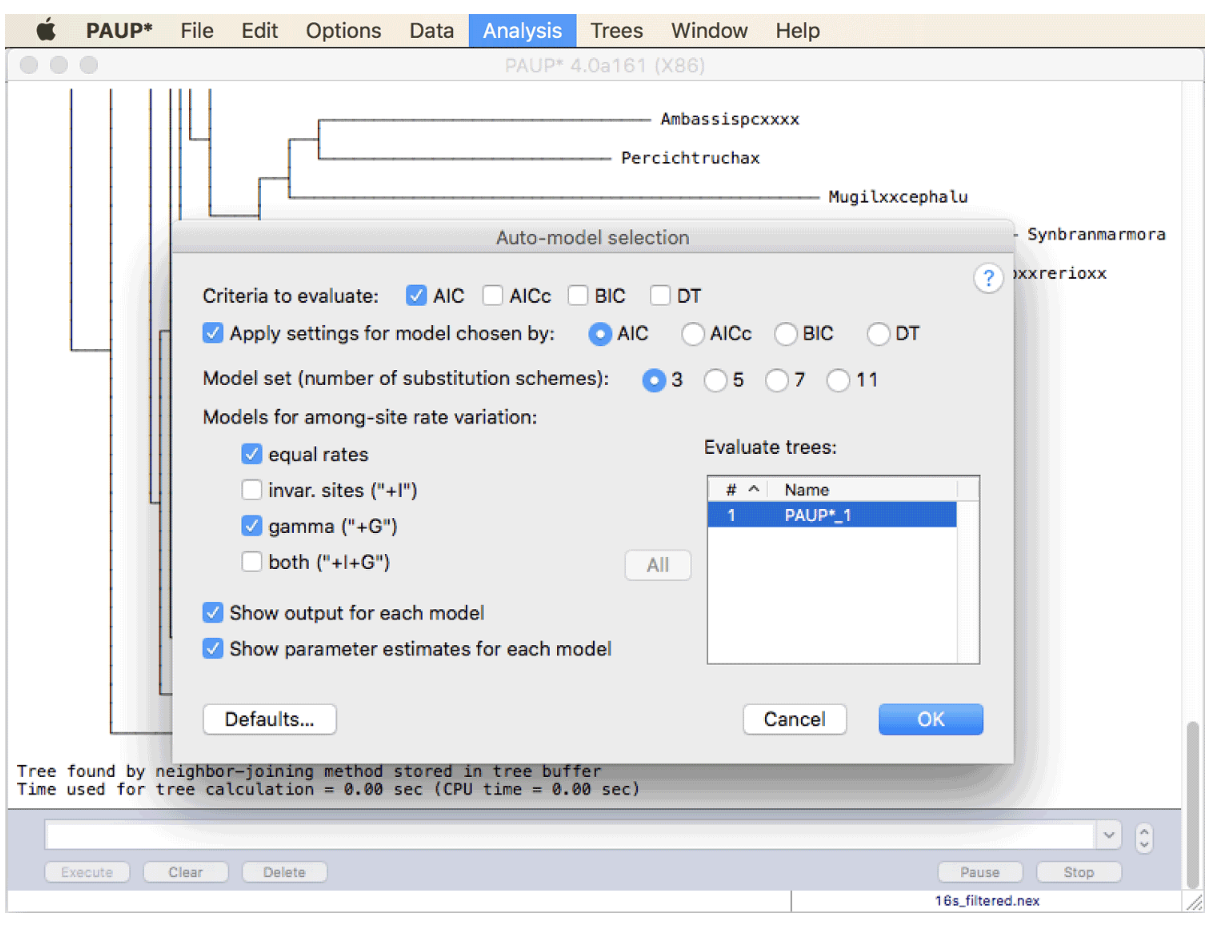
-
PAUP* 将在三个表中报告模型选择的输出。在第一个部分(在“评估树 1 的模型”下),您将看到已比较的 12 个模型的列表,如下所示(“JC”代表 Jukes-Cantor 模型)。
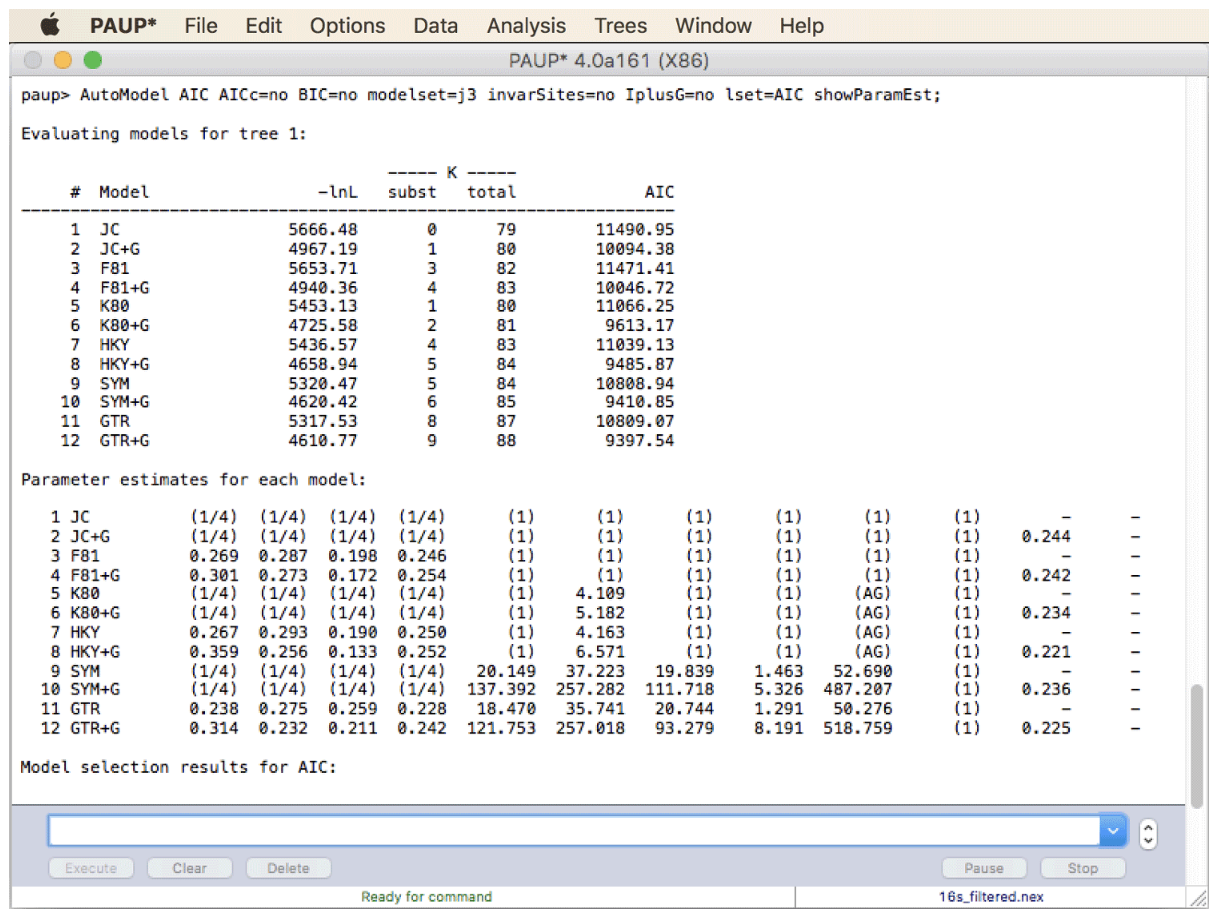
-
在同一个表的第 4 列和第 5 列中,您将看到 k,即模型中自由参数的数量。第 4 列列出了与最简单模型相比额外的自由参数的数量,第 5 列列出了自由参数的总数。第二个表列出了每个模型的参数估计值。每个型号的编号和名称后面有九列数字。最后,第三个表再次列出了模型,但这次是按 AIC 分数排名。
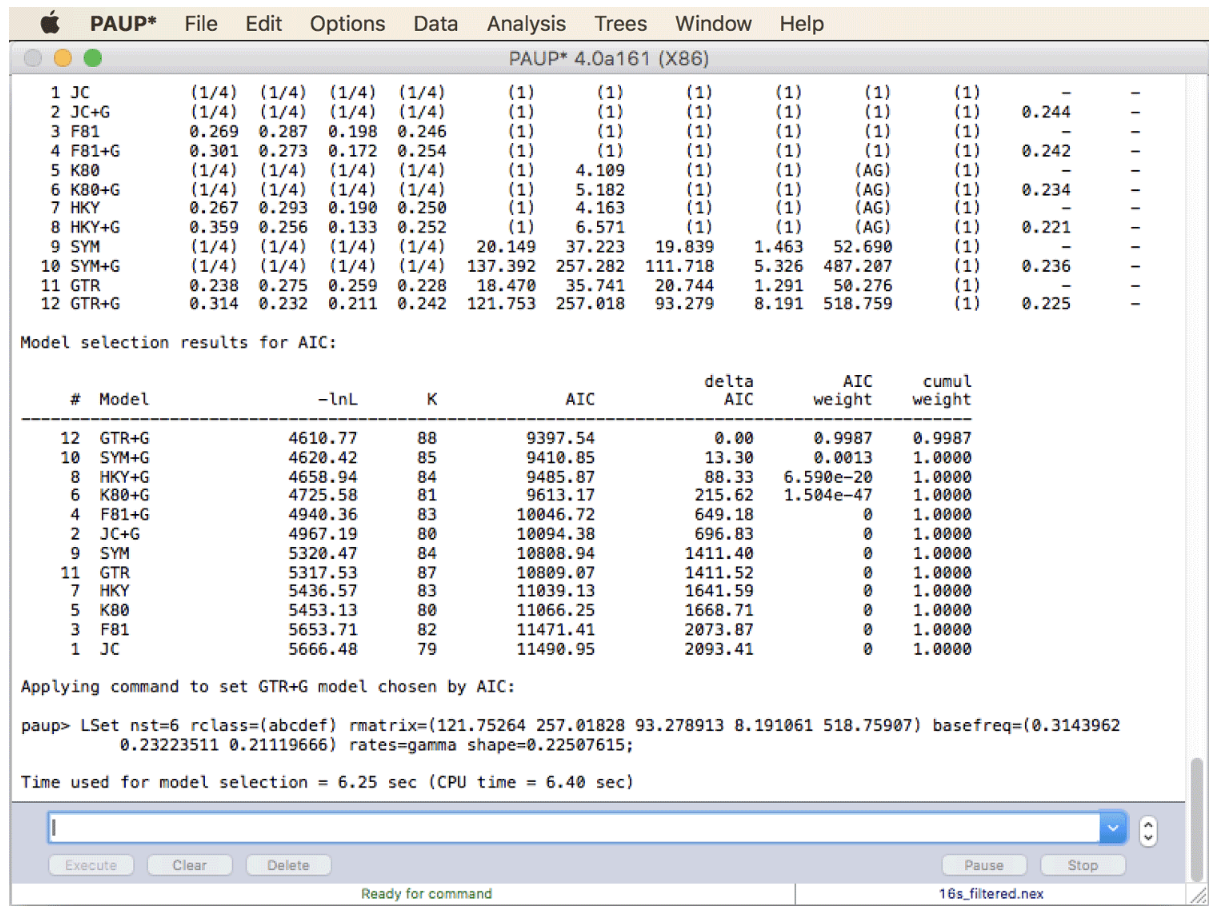
-
重复替换模型与 RAG1 序列比对 (rag1_filtered.nex) 的比较。
动动您发财的小手点个赞吧!
Reference
Source: https://github.com/mmatschiner/tutorials/blob/master/substitution_model_selection/README.md
本文由 mdnice 多平台发布