UniIVAL, the first unified model capable of supporting image, video, and audio-text tasks!
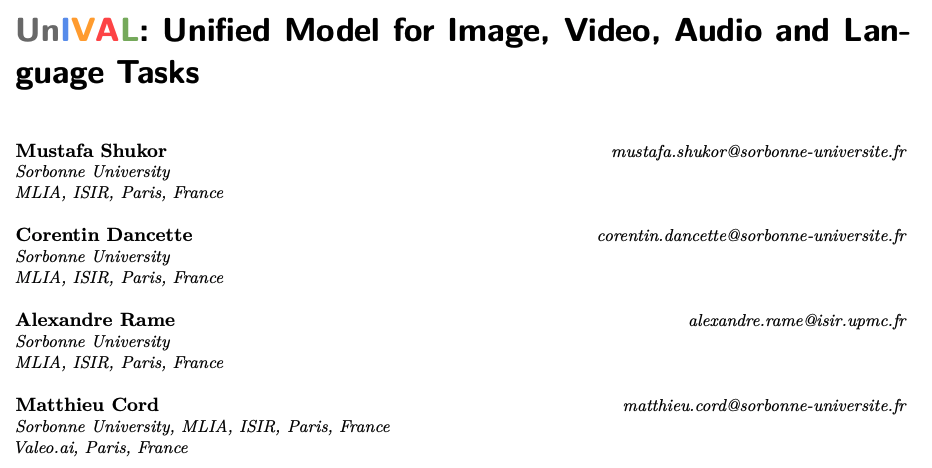
Enter the NLP group —> join the NLP exchange group
Large language models (LLMs) make the ambitious quest for generalist agents no longer a fantasy.
A key obstacle to building such general models is the diversity and heterogeneity of tasks and modalities.
One promising solution is Unification, allowing support for countless tasks and modes within a unified framework.
While large models such as Flamingo (Alayrac et al., 2022) trained on massive datasets can support more than two modalities, current small to medium unified models are still limited to 2 modalities, usually image-text or video-text.
The question we ask is: Is it possible to efficiently build a unified model that can support all modalities?
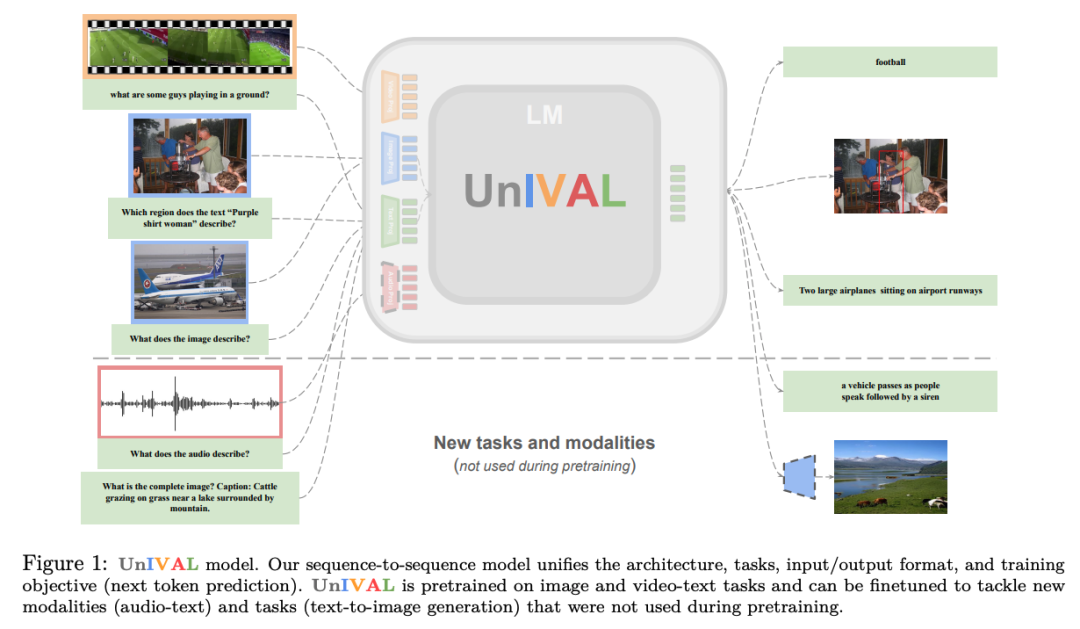
To answer this question, we propose UniIVAL , a step towards this ambitious goal.
Without relying on fancy dataset sizes or model billions of parameters, the ~0.25B parameter UniVAL model transcends both modalities, unifying text, images, video, and audio into a single model.
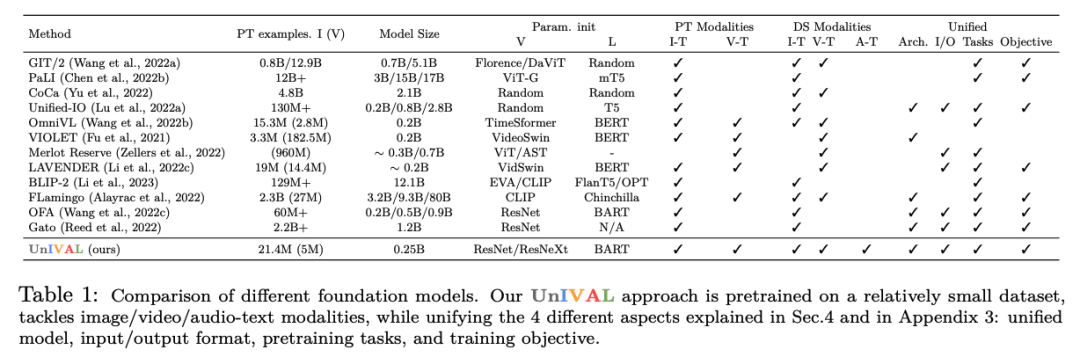
Our model is effectively pretrained on many tasks based on task balancing and multimodal curriculum learning.
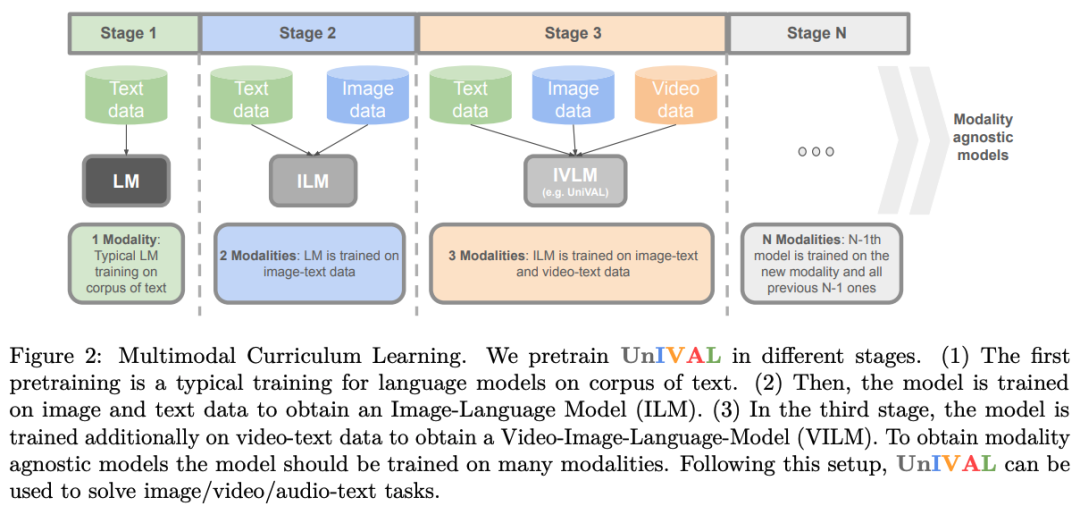

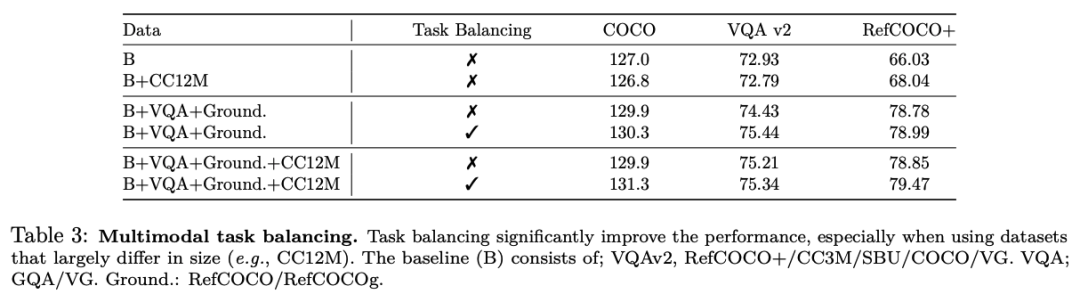
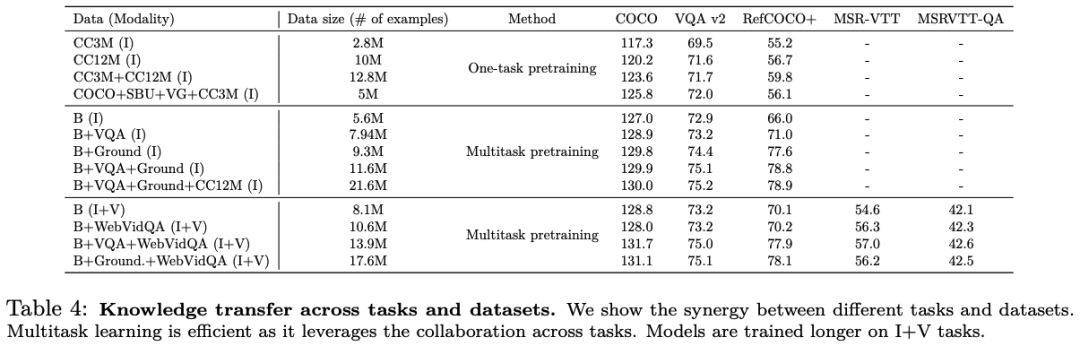
UniIVAL shows competitive performance of existing state-of-the-art methods across image and video-text tasks.
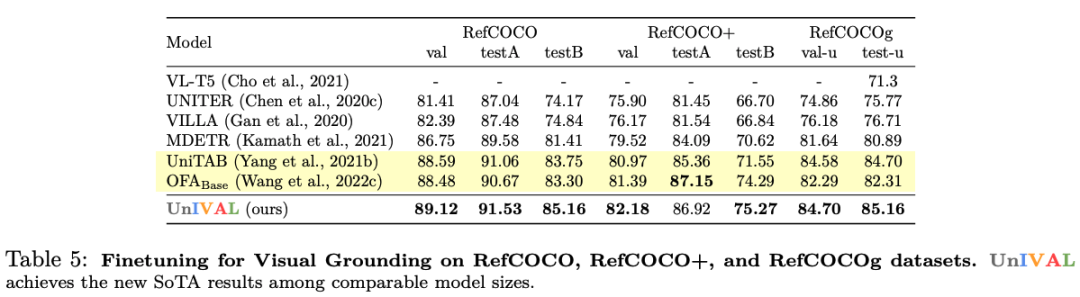
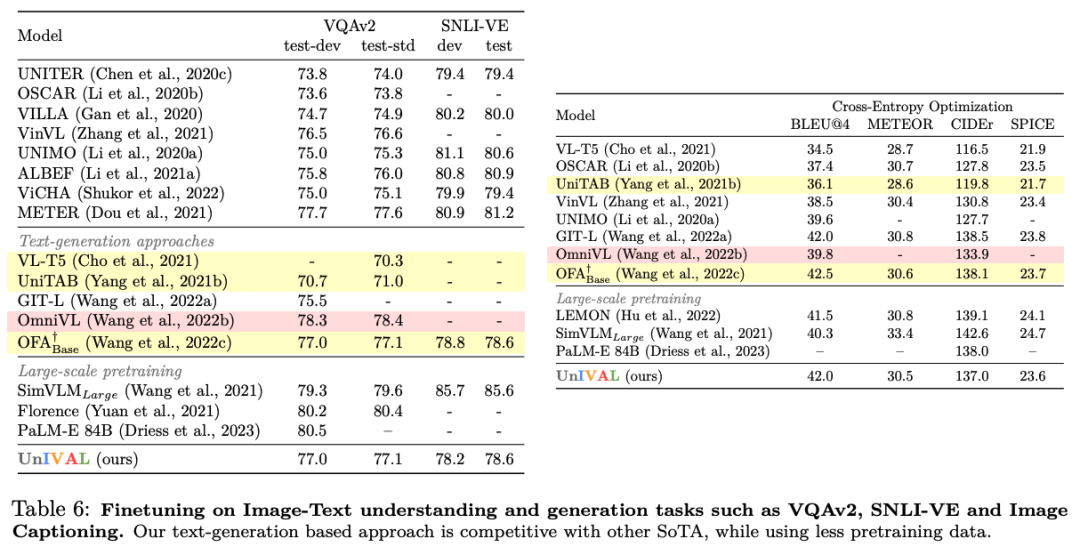
Feature representations learned from image and video-text patterns allow models to achieve competitive performance when fine-tuned on audio-text tasks, despite not being pretrained on audio.
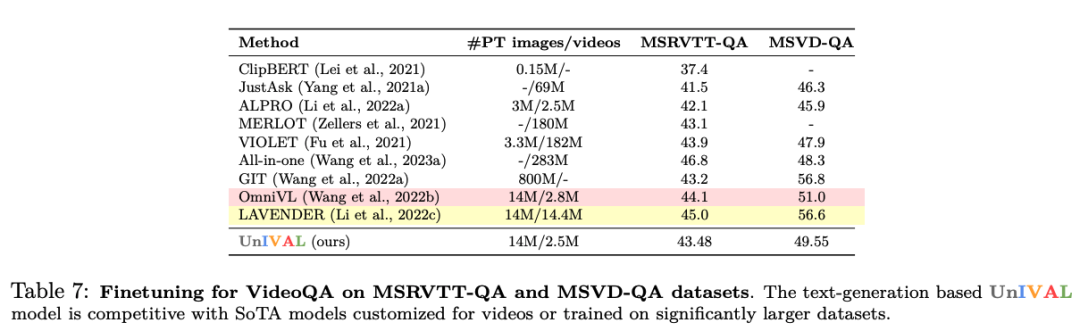
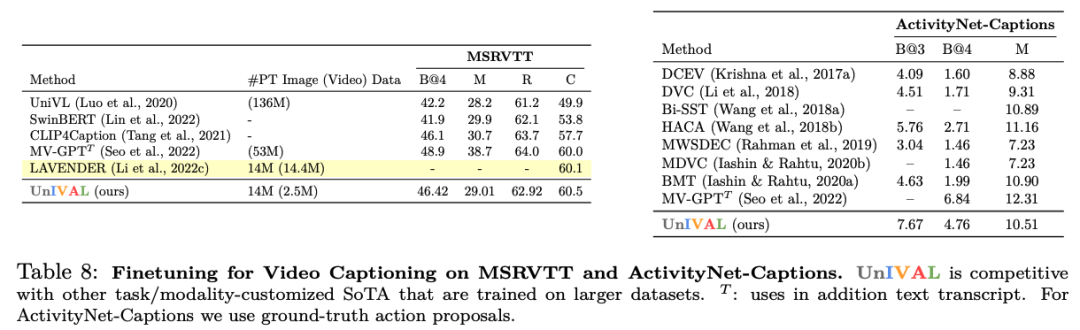
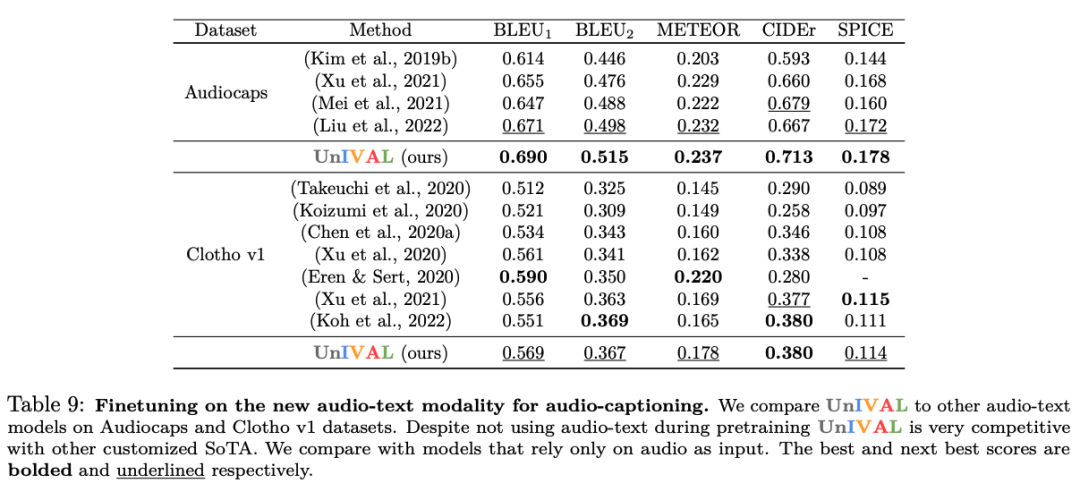
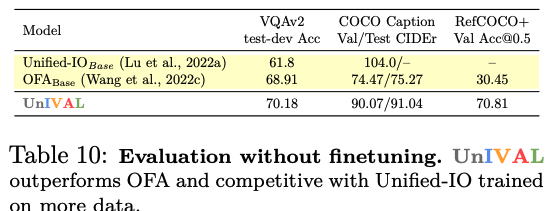
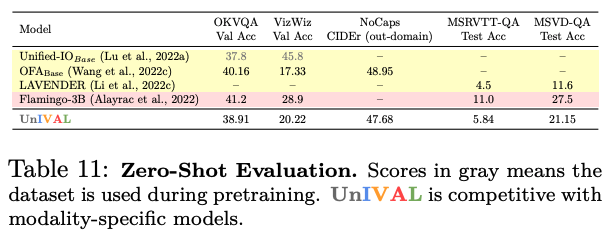
Benefiting from the unified model, we present a novel study of multimodal model merging via weight interpolation of models trained on different multimodal tasks, showing their benefits especially for out-of-distribution generalization.
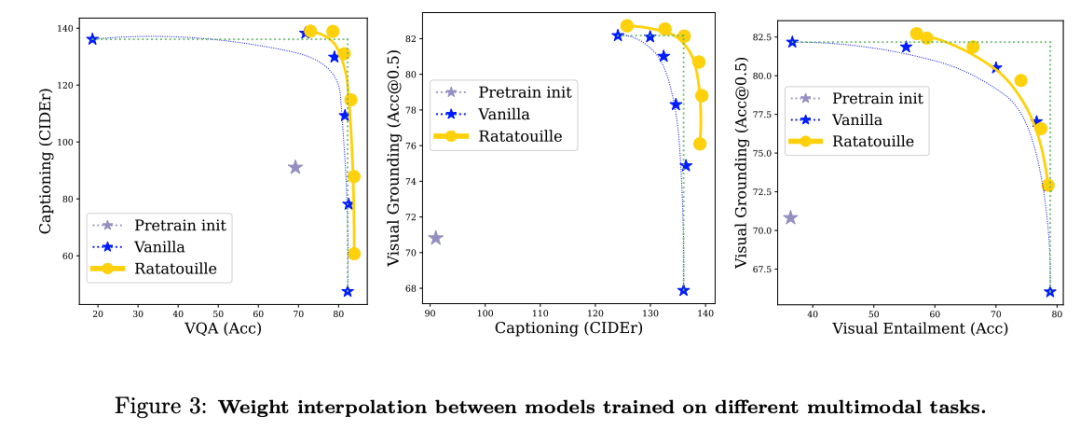
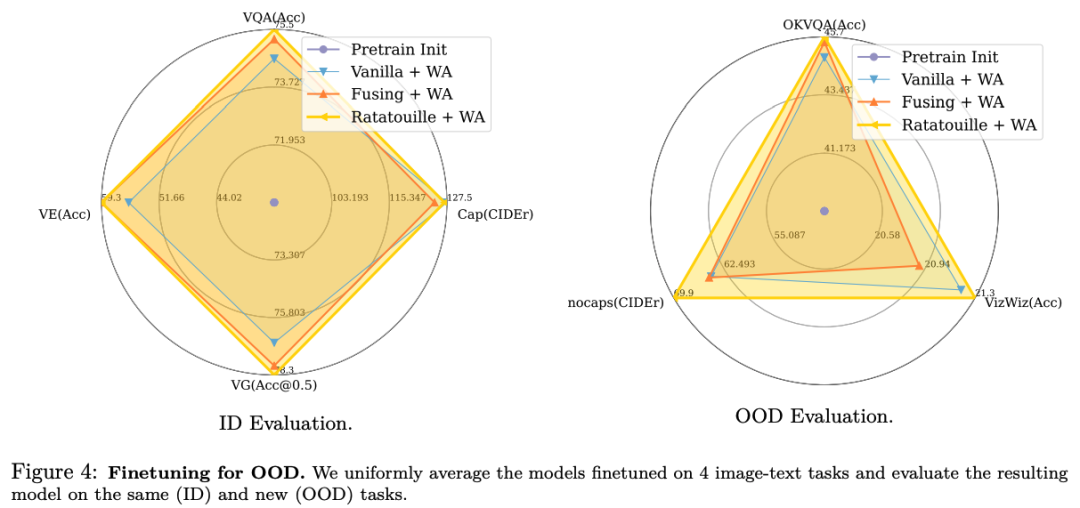
Finally, we incentivize unification by demonstrating synergy between tasks.
Summarize
In this study, we introduce UniIVAL, the first unified model capable of supporting image, video, and audio-text tasks.
We do this with a relatively small model with ~0.25B parameters on a relatively small dataset.
Our unified system is multi-task pre-trained with multiple advantages. It exploits the synergy between different tasks and modalities, enables more efficient data training, and exhibits strong generalization ability to novel modalities and tasks.
The unifying aspect of our strategy paves the way for interesting techniques for merging models fine-tuned on different multimodal tasks: we demonstrate that, in addition to multi-task pre-training, task diversity can be further exploited by weight interpolation merging.
Ultimately, we hope that our work will inspire the research community and accelerate progress in building modality-independent generalist assistant agents.
Enter the NLP group —> join the NLP exchange group