Source: Journal of Automation
Authors: Liu Huaping, Guo Di, Sun Fuchun, Zhang Xinyu
Summary
Embodied intelligence emphasizes that intelligence is influenced by the brain, body, and environment, and pays more attention to the "interaction" between the agent and the environment. Therefore, in the study of embodied intelligence, the relationship between the physical form of the agent and perception, learning, and control plays an important role. At present, embodied intelligence has comprehensively absorbed relevant research results in the field of mechanism science on form and structure, machine learning on perception and learning, and robotics on behavior and control, forming a relatively complete and independent And it is still a vigorously developing subject branch. However, there is no literature that completely combs the research progress of form-based embodied intelligence. From this perspective, this paper focuses on behavior generation based on morphological computation, morphological control based on learning, and The three aspects of learning form optimization summarize important research progress, condense relevant scientific issues, and summarize future development directions, which can provide reference for the research of embodied intelligence.
Key words
Embodied Intelligence / Morphological Intelligence / Morphological Computing / Morphological Control / Morphological-Control Co-Optimization
Modern artificial intelligence originated from the Dartmouth conference in the 1950s. For a period of time after that, the research on artificial intelligence was mainly limited to the symbol processing paradigm (also known as symbolism). However, the limitations of symbolism The nature of the theory was quickly exposed in practical applications, and it stimulated the development of connectionism, forming a multi-layer perceptron, feed-forward neural network, recurrent neural network, deep neural network, which is popular in academia and industry today, etc. This method of simulating the cognitive process with artificial neural networks has indeed made great progress in adaptation, generalization and learning, but it has not really solved the problem of the interaction between the agent and the real physical world. , robustness and other aspects are also facing great challenges [1]. In fact, the discussion on the problems of symbolism and connectionism has attracted great attention in the 1970s and 1980s. Hans · The "Moravec Paradox" proposed by Hans Moravec, Rodney Brooks and Marvin Minsky (generally and popularly expressed as: To let the computer It is relatively easy for an adult to play chess, but it is quite difficult or even impossible for a computer to have the perception and action ability of a one-year-old child), which reflects the concerns of many scholars. In response to this problem, Minsky From the perspective of behavioral learning, the concept of "reinforcement learning" was proposed. From the perspective of cybernetics, Brooks emphasized that intelligence is embodied (Embodied) and contextualized (Contextlized) [2]. In other words, an agent must have a "body" In order to enter the real world from the virtual world, and develop or evolve intelligence through the interaction with the real world. He developed a series of bionic robots under the guidance of the idea that "intelligence needs a body" [3], and pointed out that even if the research Simple behaviors such as walking and moving can also promote the understanding of the embodied nature of intelligence. In his book How the Body Shapes the Way We Think[4], Rolf Pfeifer analyzed the impact of "how the body affects intelligence" on "the embodied nature of intelligence". made a clear description of "embodied" and clarified the profound impact of "embodied" on the understanding of the nature of intelligence and the study of artificial intelligence systems. These works have laid the foundation for the third school of artificial intelligence—behaviorism represented by embodied intelligence. a solid technical foundation.
Different from symbolism's emphasis on "representation" and connectionism's emphasis on "computation", embodied intelligence pays more attention to "interaction". The physical operation process is generated continuously and dynamically. The research on embodied intelligence can be traced back to Aristotle’s discussion on the subjectivity of the body at the level of perception and movement in ancient Greece. Further, including Charles Darwin (Charles Darwin) Scientists including Robert Darwin, Maurice Merleau-Ponty, Claude Bernard and Martin Heidegger studied biology, philosophy, physiology and In the 1960s, Swiss psychologist Jean Piaget clearly pointed out that movement is the source of cognition in the process of studying child psychology. The basis of knowledge and knowledge, appropriate action behavior can help babies gradually form cognition about the world. American visual psychologist James Gibson (James Gibson) also emphasized the interaction between human and environment in his research on human vision and machine vision The close relationship between embodied perception and behavior in the process. Due to its inherent philosophy, psychology, physiology and cognitive science foundation, research results related to embodied intelligence are distributed in bionic/developmental/evolutionary robotics, artificial life, universal In many disciplines and directions such as computing [5-7]. The first issue of Nature Machine Intelligence also published a special paper on embodied intelligence; literature [8-9] comprehensively expounded the relationship between embodied intelligence and robots; Massachusetts Institute of Technology A research team named "Embodied Intelligence" (https://ei.csail.mit.edu) has also been established; internationally renowned companies including Google, Meta, etc. are also investing heavily in research related to embodied intelligence At present, the research on embodied intelligence has been widely expanded to the fields of education[10], materials[11-12], energy[13], etc., and it has become an important window for breakthroughs in the theory and application of the new generation of artificial intelligence in the future.
In order to distinguish it from embodied intelligence, this paper classifies the methods emphasizing "representation" and "computation" into the category of "disembodied intelligence". Although the two research paradigms of embodied intelligence and disembodied intelligence have different starting points, and There have been many confrontations in history, but it has to be admitted that such confrontations have effectively advanced people's understanding and simulation of intelligence. Literature [14] discussed the embodied cognition characteristics of analog computing, and explained the analog computer as an example The relationship between embodied cognition and intelligence based on computation and representation. Generally speaking, driven by big data, GPU and other resources, out-of-body intelligence has achieved great success in the field represented by Internet information processing; Body intelligence involves fields such as mechanisms and materials, and has become the core foundation of intelligent robots. In fact, it is now generally accepted that embodied intelligence and out-of-body intelligence are not mutually exclusive, especially at the method level, including deep learning, Reinforcement learning and other methods have become important tools to solve the problems of out-of-body intelligence and embodied intelligence. with extensions.
At present, embodied intelligence has comprehensively absorbed research results related to form and structure in the field of mechanism, perception and learning in the field of machine learning, and behavior and control in the field of robotics, forming a relatively complete, independent and rapidly developing discipline. branch, and has made great progress in the aspects of human-inspired perception, decision-making, control and system design[15]. Although there are currently some treatises with the theme of "Overview of Embodied Intelligence"[16-17], Its content is mainly limited to the joint learning of perception and behavior, ignoring the influence of physical form. In embodied intelligence, the relationship between the physical form of an agent and perception, learning, and control plays a vital role, but there is no The literature comprehensively sorts out the research progress in the form of embodied intelligence. This paper comprehensively analyzes and summarizes relevant important research progress on this issue, in order to provide reference for the development of this field. Philosophical discussions related to embodied intelligence, psychology, Research progress in physiology and materials science is beyond the scope of this article.
The structure of this paper is as follows: Section 1 summarizes the architecture of embodied intelligence, clarifies the position of form-based embodied intelligence research in the entire embodied intelligence research system, and generally introduces the development trend of related fields; Sections 2 and 3 Sections 1 and 4 respectively review in detail the three important issues of morphological computation-based behavior generation, learning-based morphological control, and learning-based morphological optimization; Section 5 specifically introduces the relevant cutting-edge developments for the typical form of soft robots; Section 6 is the summary and outlook.
1. Morphology-based embodied intelligence architecture
A core element of embodied intelligence is the design, control and optimization of the agent's own form. The research work in this area is closely related to the fields of mechanism and machine learning, and has strong crossover, so there are few systematic review literatures. This section summarizes the research progress and key scientific issues in this field according to the three modules of form, behavior and learning by analyzing the characteristics of embodied intelligence. There is a close relationship between these three modules (see Figure 1). Connections are manifested as different contents of embodied intelligence. It is worth mentioning that the content that this paper focuses on comprehensively considers the relationship between form, behavior, and learning. This is different from the "physical intelligence" mentioned in literature [9, 18] (Physical intelligence) has an important difference. The latter emphasizes the functions formed by the structural characteristics of the ontology itself.
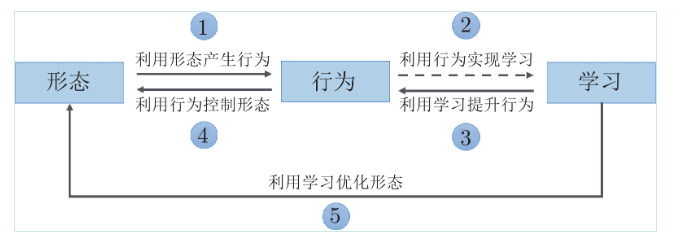
According to the architecture shown in Figure 1, in form-based embodied intelligence, the relationship between form, behavior and learning can be summarized in the following aspects:
-
1) Behavior generation by using morphology: Emphasis is placed on using the morphological characteristics of embodied agents to subtly implement specific behaviors, so as to achieve the purpose of partially replacing "computation". Behavioral generation of ".
-
2) Using behavior to realize learning: Emphasis is placed on actively acquiring learning samples and labeling information using the behavioral capabilities of embodied agents such as exploration and operation, so as to achieve the purpose of autonomous learning. This part of the work is relatively cutting-edge, and related achievements have not yet formed a relatively complete system , but it is an important research direction in the future, so this article will review it in the conclusion and prospect section.
-
3) and 4) respectively emphasize the use of learning to improve behavior and use behavior to control form. There are many ways to realize the latter, but the current work of using learning methods to improve behavior and then control form emerged after the development of modern artificial intelligence technology In particular, the technology based on reinforcement learning has become a current hot method. Therefore, Section 3 of this paper, "Learning-based Morphological Control" focuses on the specific review from this perspective.
-
5) Optimizing shape by learning: emphasizing the use of advanced learning optimization technology to realize the optimal design of the shape of the embodied agent. The relevant research progress of this part will be carried out in Section 4 of this paper, "Learning-based shape optimization".
2. Behavior Generation Based on Morphological Computation
The body of organisms gradually forms its own specific morphological structure in the process of adapting to the environment. This morphological structure plays a vital role in the survival of organisms. Literature [19] reveals the body shape of fish through the study of fish that have lost their lives. Natural movement in water can be produced only by interacting with the environment. This phenomenon abounds in human life. For example, when a person takes an object, he does not need to know the material, shape, size, and posture of the object It can be easily realized by precise estimation. This kind of intelligent behavior realized by structural shape alone has been paid attention to early, and it is called "morphological computing" or "morphological intelligence" in different occasions. This paper will uniformly use "Morphological computing" describes the mechanism of using the shape, material and dynamic characteristics of the body to improve computing efficiency and further realize the control of body behavior. Through morphological computing, part of the computing work that needs to be done by the "brain" can be transferred to the "body". "Complete, so that the interaction between the "body" and the environment is used to generate behavior. Morphological computing has great advantages in simulation-physical migration and low-power green computing [20], and is even regarded as the most advanced in embodied intelligence. The core and important content[21]. In recent years, with the development of precision mechanisms, soft materials and other fields, morphological computing has ushered in new development opportunities, including Artificial Life, Advanced Robotics, IEEE RAM, etc. album, which has strongly promoted the development of this field[22-23].
Since morphological computing is closely related to the study of biomimetic robotics, the relationship between the two may even cause some confusion. In fact, morphological computing is more concerned with using morphology to generate behavior than realistically imitating certain biological forms .Many bionic robots achieve some functional breakthroughs by imitating the shape of creatures. For example, legged robots can gain the ability to climb stairs compared with wheeled robots. But in terms of behavior control, they have not fully utilized the advantages of the shape itself, and still need Design complex controllers. These situations are not included in the morphological computing discussed in this paper. In addition, the morphological computing discussed in this paper is not the same as the concept of "embodied computing" used in [24]. The latter emphasizes Wearable, absorbable, implantable, and embeddable explicit computing devices in the human body can also be called "body-centered computing", while "morphological computing" emphasizes the use of the characteristics of the structure itself to realize implicit calculation of .
The history of using morphological calculation to realize automatic control can be traced back to Watt’s invention of the steam engine centrifugal governor, which is also the first controller oriented to practical industrial applications in history (see Figure 2). The invention of the centrifugal governor makes the steam engine Towards large-scale application, and helped the first industrial revolution. Watt is even called "the father of the steam engine". From the perspective of behavior control, the centrifugal governor actually uses the mechanism system to realize the analog operation of feedback control , but this control structure is quite different from the “controller-sensor” feedback control structure of modern control systems. By analyzing the structure of the centrifugal governor, it can be found that its form contains rich calculations and representations. The core is not contradictory to the traditional artificial intelligence field, but only uses the form to realize calculation and representation. However, with the rapid development of digital computing equipment, computers are gradually used to realize complex controller operations, making this use of mechanism form to achieve Methods of behavioral control have been largely neglected in the field of automatic control.

Nevertheless, researchers in the field of robotics have not completely given up the exploration of this problem. In the past thirty years, a large number of morphological computing devices including passive walking robots and related Theoretical model. This section focuses on combing the relevant important developments from the two perspectives of morphological computing devices and theoretical models, and will specifically introduce the relevant morphological computing methods for soft robots in Section 5.
2.1 Development of Morphological Computing Devices
A typical example of using morphological computing to realize complex behavior control is the passive walking robot in the 1990s. Literature [25] analyzed the human walking mechanism by using the motion characteristics of this mechanism, and explained the realization of this mechanism by using passive dynamics. , and proved that it can achieve a stable gait on a slight slope without power. In 2005, four scholars from the University of Michigan, Cornell University, Massachusetts Institute of Technology and Delft University in the Netherlands jointly worked on the The article [26] on Science pointed out that by introducing a weak power source (used to compensate for gravity), passive walking robots can achieve a natural gait similar to humans on a plane (see Figure 3(a)). They constructed three types The walking ability of the robot platform is completely generated through the interaction between the robot body and the environment (gravity and slope) (see Figure 3(b)). This work abandons the strict control of robot joints in the traditional dynamics modeling and control field. According to the requirements, the robot can realize the control of the overall behavior completely relying on its own shape, which becomes the evidence of using morphological computing ability to realize complex behavioral intelligence.
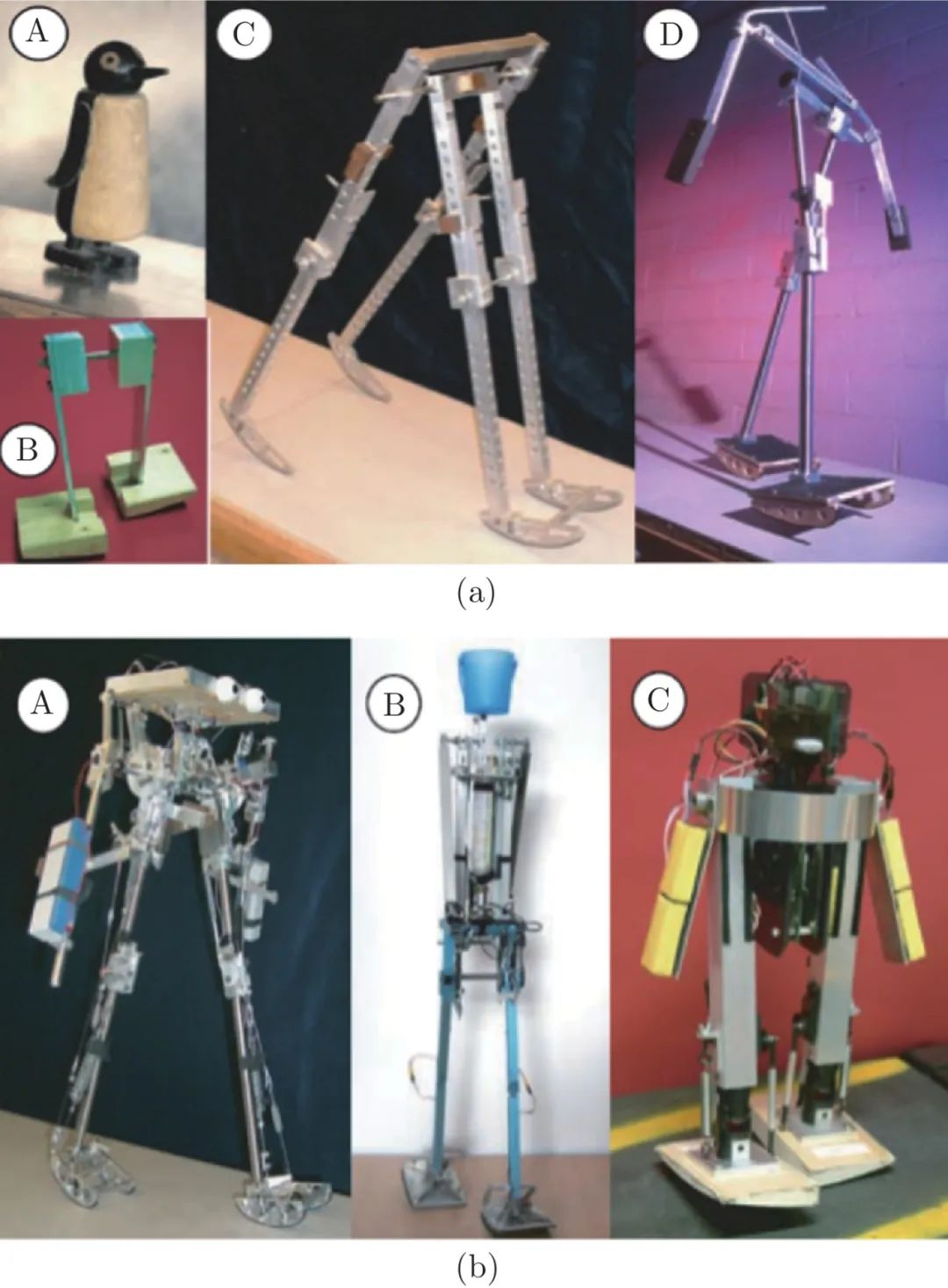
After successfully simulating biped robots, a series of biomimetic robots using morphological computing appeared in academia. For example, the quadruped robot Puppy with active and passive joint joints can easily perceive terrain information[27], and the artificial fish Wanda can use the least The control quantity realizes navigation in three-dimensional space[28]. Further, literature[29-30] lists and analyzes in detail the embodied shape calculation used to realize functions such as moving target detection, operation grasping, four-legged walking, and underwater navigation. device.
In recent years, with the rapid development of mechanisms, materials and other fields, related new forms have also emerged. For example, literature [31] discussed the use of morphological computing to achieve high-speed motion of quadruped robots. Literature [32] used dynamic morphological computing to generate Periodic gait of snake-like robots. Literature [33] expounds the morphological calculation in tactile perception for natural and artificial systems, oriented to tactile display, sensing and interaction. Inspired by desert locusts, literature [34] studied the animal leg Adapt to different ground control methods, and carry out robot dynamic adhesion experiments on surfaces including glass, sandstone, wood and grid. In addition, the "wind-powered bionic beast" invented by Dutch kinetic sculpture artist Theo Jansen can rely on mechanical principles It moves forward with natural wind, and its ingenious structure lies in the rational use of balance to transform physical variables, which has a very high energy conversion rate[18]. The combination of creation is very close, and most of the artificial design elements are present. At present, there is still a lack of guidance from systematic artificial intelligence technology.
2.2 Theoretical Model of Morphological Computation
Regarding the essence of "morphological computing", it is generally believed that it emphasizes the realization of computing, rather than the computing mechanism like the Turing machine model. Due to the huge potential of morphological computing, many scholars have been exploring its internal mechanism, hoping to use This will further promote the research on morphological computing. Considering the important role of "XOR" operation in the history of neural network development, literature [35] explores the possibility of using robot morphology to realize XOR operation, and constructs some thought experiments to preliminarily It is confirmed that the dynamic coupling of the physical body can effectively reduce the difficulty of controller design. Literature [36] established a formal analysis method for morphological computation from the perspective of programmable dynamic systems, pointing out that morphological computation is not only suitable for robots, but also can be widely used in Chemical systems, statistical physics and other scientific fields.
Although the theoretical system of morphological computing has not yet been established, and its theoretical model is not complete, there are currently two relatively mature methods: the dynamic system method and the information theory method. The two are not mutually exclusive, but can complement each other. The former The representative model of the latter is the calculation model of the reserve pool, and the representative method of the latter is mainly the controller complexity analysis method. The progress of these two aspects will be introduced respectively in the following.
2.2.1 Dynamic system analysis method for morphological calculation
The dynamic system method is mainly based on the reserve computing (Reservoir computing, RC) model, that is, the shape is understood as a physical reserve computing device. The reserve computing model is a kind of middle layer whose parameters are randomly fixed, and only needs to train the neural network of the output layer parameters. Network structure. Since its adjustable parameters only need to solve linear optimization problems, network training is very convenient. On the other hand, reserve pool calculation has a strong ability to describe dynamic systems, so it has become a powerful tool for analyzing morphological calculations[37].
Literature [38] used the random link form between multiple springs and masses to construct a reserve pool calculation model, and analyzed its performance in time series approximation by using nonlinear filter approximation theory (see Figure 4(a)) , pointing out that the Volterra series can be used
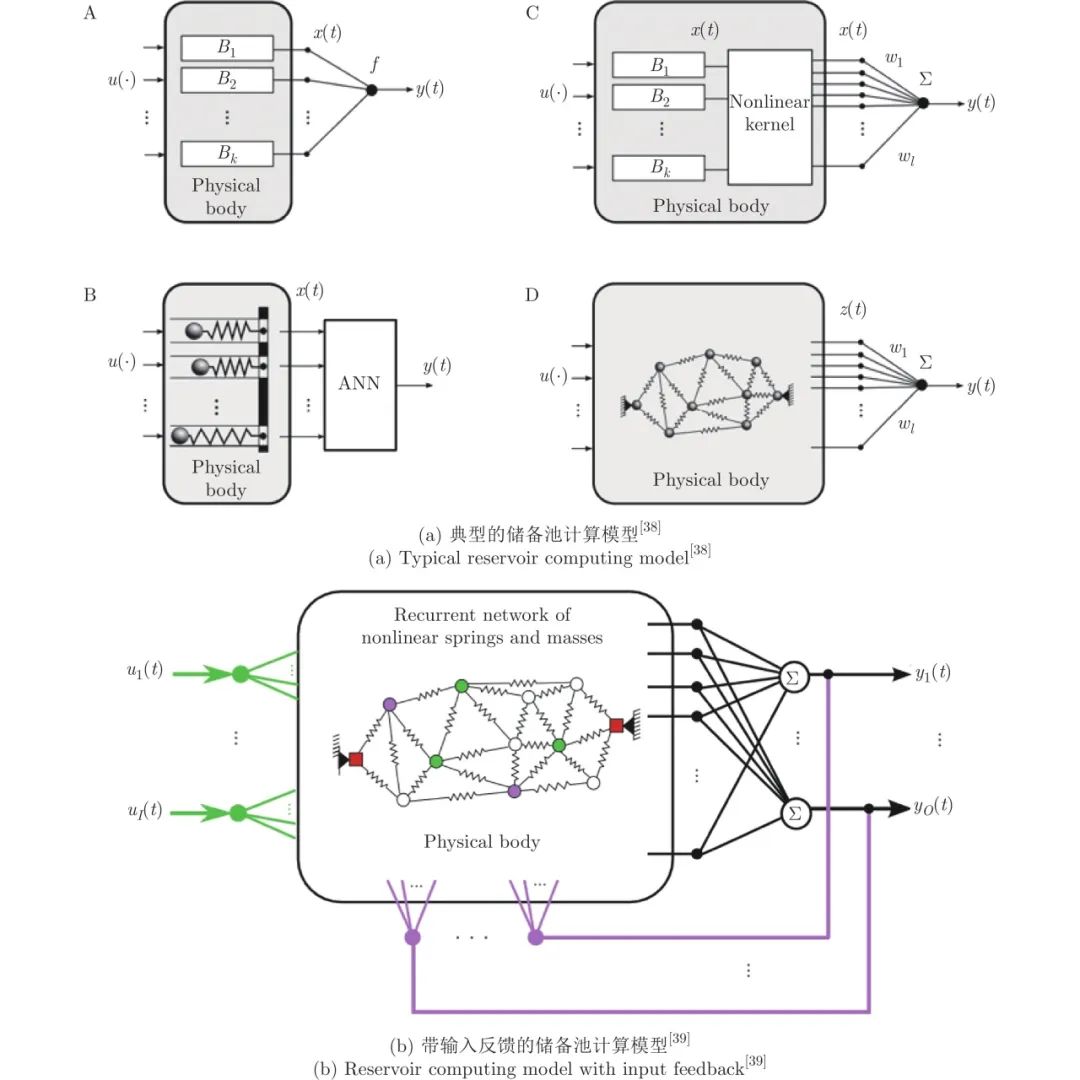
to represent the process of morphological calculation, where y(t) is the output sequence, and hd is the integral kernel that transforms the input u(t−τ1),⋯,u(t−τd) at different times in the past. Since Volterra Series can be used to represent continuous and nonlinear dynamic systems, so it can be conveniently used to describe many morphological calculation processes.
Furthermore, literature [39] discusses the role of output feedback in morphological computation. By introducing feedback from the output, the network can realize more complex behaviors such as robot walking (see Figure 4(b)). Literature [40] discusses this type of morphological The ability of the calculation model in robot walking learning was further analyzed. The literature [41] established the input and output equations of the morphological calculation for this spring mass model, and thus established the algebraic method of using the robot dynamics to realize the morphological calculation.
The above work provides a general reserve pool calculation model for morphological computing. Generally speaking, in order to achieve the desired computing power, the corresponding reserve pool needs to meet conditions including input separability and fading memory. Therefore, the follow-up work is mainly to construct different physically realizable computing models of reserve pools to describe and realize the morphological computing capabilities. In this sense, the research on computing models of reserve pools can effectively guide the design and A typical example of analysis is: if the mass in the spring mass in the above work is changed to a rigid rod, a tensegrity structure can be formed. This structure is composed of several separated compression members and continuous The self-stress-balanced stable space structure formed by the interconnection of tension members has the characteristics of both rigid structure and flexible structure. It can not only prevent the overall structure from collapsing by applying a certain tension force to the structure, but also has good flexibility. To absorb the impact of collisions. The tensegrity robot adds an active driving force to the structure, and changes the position of the center of gravity through its own deformation to achieve movement. It has the characteristics of light weight and foldability. It has achieved great development since it was proposed in the middle of the last century. [42]. Literature [35] uses four control inputs to control a tensegrity structure robot with 24 degrees of freedom, which embodies the "calculation" function of the tensegrity shape (see Figure 5). Literature [43-44] A linear feedback controller is introduced to achieve complex gait generation. It is pointed out that only a simple linear transformation is needed to generate a stable walking strategy. The core reason is that the shape of this type of robot shares some of the complex control calculation functions. Literature [45] further studied the Hebbian-type learning strategy for this kind of structure. For the recent development of tensegrity robot mechanism, please refer to [46-47].
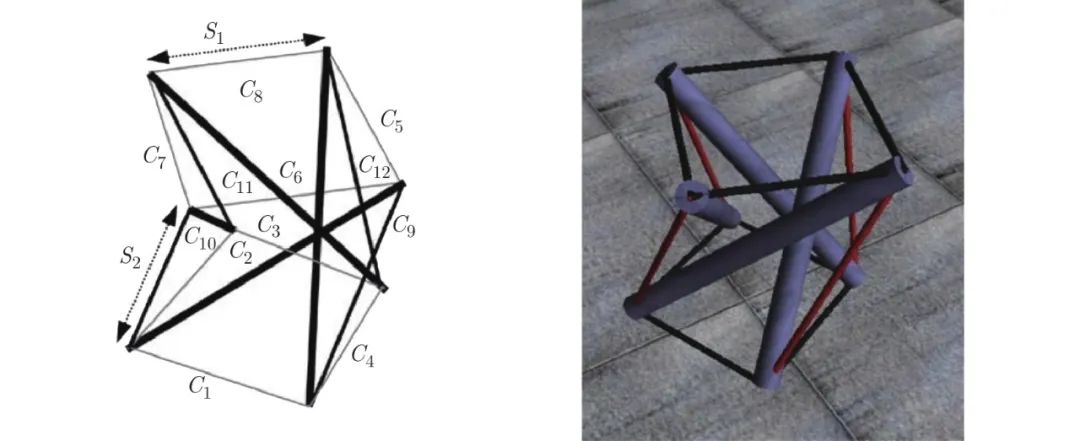
In addition, when the literature [48] applied the traditional reserve pool model to the morphological calculation of quadruped robot gait control, it was found that it is difficult to achieve complex morphological control by using simple linear transformation directly in the reserve pool calculation model. This paper introduces a nonlinear transformation layer, which significantly improves the description ability of the model (see Figure 6(a)). Literature [49] verifies that the robotic fish system also satisfies the Echo state property (Echo state property, That is, the system output only depends on the previous input, and has nothing to do with the initial state), so the use of the swimming characteristics of the robotic fish can significantly improve the morphological computing capability (see Figure 6(b)). Literature [50] pointed out that the origami structure It can also have sufficient dynamic performance, so that it has a strong embodied computing ability to simulate high-order nonlinear systems. In this paper, this phenomenon is used to realize a new robot crawling strategy (see Figure 6(c)). In addition, the literature [51] simulated the morphological computing ability of human skin by using the reserve pool calculation. Reference [52] used the dynamics of the robot itself to assist in the detection of wind speed. The recent development of the reserve pool calculation is very fast, and it has been applied in many fields. Especially the physical reserve Pool calculation, that is, the storage pool calculation realized by physical devices, has a direct and natural connection with embodied form calculation. Literature [53-54] made a detailed review on the recent progress of physical storage pool calculation.
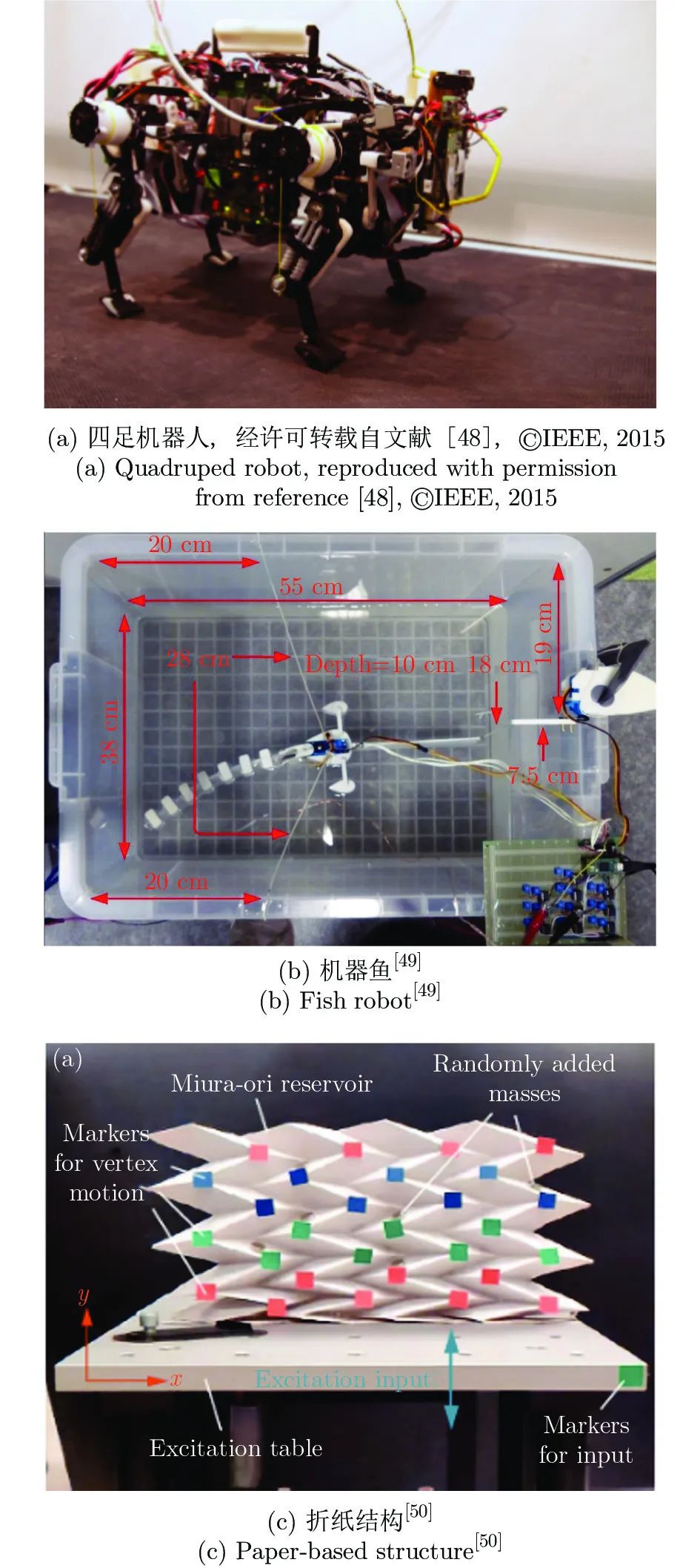
In addition to the calculation of physical reserve pools, the current methods of using various physical forms to realize neural network learning have also attracted great attention from researchers in different disciplines. For example, the physical perception training method proposed in [55] uses backpropagation to train controllable Physical systems. By training various physical neural networks based on optics, mechanics, and electronics, tasks such as audio and image classification can be achieved. Physical neural networks have the potential to perform machine learning faster and more energy-efficiently than traditional electronic processors, for The embodied morphological computing of robots provides a wealth of research ideas [56].
Although methods based on dynamical systems, especially reserve pool computing models, have achieved great success so far and have formed a good complement to other disciplines, such methods mainly aim at qualitatively simulating embodied morphological computing and lack quantitative evaluation. However, the ability of morphological calculations faces great difficulties in further analyzing the mechanism of morphological calculations, while the information theory method has obvious advantages in this respect.
2.2.2 Information Theoretic Analysis Method for Morphological Computing
Morphological computing is generally closely related to the specific physical realization, and its performance effect is highly visualized, and most of its performance is reflected in the form of physical experiments, resulting in a situation where qualitative analysis is more work and quantitative analysis is difficult. In fact, to measure the performance of a morphological computing system , the most important thing is to analyze how much computing power should be borne by the "brain" in its "body" (that is, the physical form). If the analysis can be carried out from this perspective, it is expected to obtain a quantitative analysis of the morphological calculation in general in conclusion.
Inspired by the above ideas, literature [57] pointed out that the quantitative analysis of morphological computing needs to establish a causal model of the cognitive system including the brain, execution, environment, and sensing (see Figure 7). A world state (World state) ww is introduced on the basis of learning the Markov decision process (MDP), which is used to describe the influence of the system's body and environment. By defining W as the set of world states, S is the sensor state Set, C is the set of internal states of the controller, A is the action set, ΔW, ΔS, ΔC, ΔA represent the probability distribution on the set W, S, C and A respectively, the embodied shape model can be defined as:
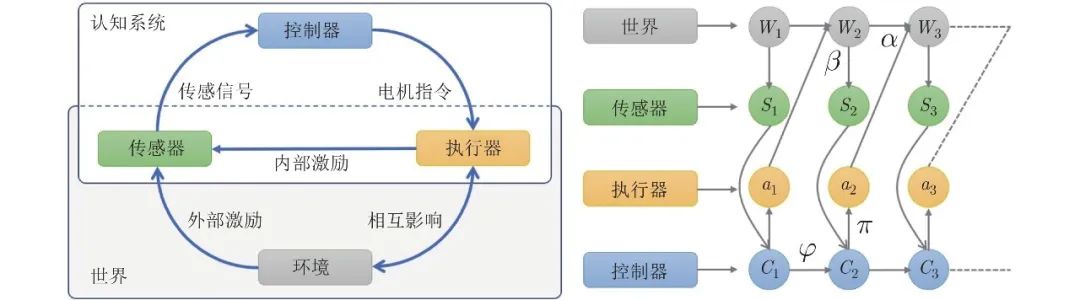
Among them, β(s∣w):W→ΔS describes how the agent perceives the environment, φ(c′∣s,c):S×C→ΔC reflects the evolution of memory c, π(a∣c):C →ΔA embodies the control strategy, α(w′∣w,a):W×A→ΔW is the evolution law of the world model, that is, the next state w′w′ of the entire environment will be determined by the current state w and the executed action a Influence. If the memory c is ignored, that is, φ(c′∣s,c):S×C→ΔC is not considered, the control strategy is described as:
The model degenerates into a memoryless reactive system in conventional reinforcement learning.
Based on this model, in order to describe the role of morphological calculations, the degenerate case of α(w′∣w,a) can be considered
. In this degenerate situation, since the next state of the environment is only affected by the action, the morphological computation plays no role. Therefore, it is possible to evaluate
The difference between them is used to judge the role of morphological intelligence. A typical measure is Kullback-Leibler divergence:
Among them, p(w′,w,a) is the joint distribution that can be estimated by frequency counting. Based on this model, literature [58] studied the application of morphological computation in different levels of control systems in the neural-muscular-skeletal model The role of morphological computation in different sensor configurations in mobile robots was analyzed in [59].
In addition, there are also some works to evaluate morphological intelligence from the perspective of analyzing the control complexity. For example, literature [60] uses the probabilistic optimal control method to analyze how much computing work can be performed by the robot’s morphological intelligence by optimizing the controller complexity. Commitment. Literature [61] compared the entropy of controllers corresponding to different shapes to analyze the effect of shape on behavior. Literature [62] studied the method of using entropy to describe the complexity of the body for micro-robots. Cheap control (Cheap control) also provides a new idea for morphological intelligence. The basic idea is that in the process of pursuing cheap control, the system must fully exploit the role of form-based embodied intelligence. Literature [63] further introduces this idea into reinforcement learning , constructing a new optimization objective as:
Among them, I(S;A) reflects the complexity of the control strategy, while Q(s,a) is the long-term cumulative return in conventional reinforcement learning, and γ is the weighting parameter. By optimizing the above indicators, the complexity of the controller will be significantly reduced, and this part will be automatically undertaken by morphological calculations. Compared with the framework in [57], these works are all indirectly analyzing the role of morphological effects by analyzing the complexity of the controller. In addition, the current quantitative Most of the analysis results are theoretical explorations independent of the actual physical form, and how to combine specific physical forms to achieve quantitative analysis is still a big challenge.
2.3 Summary
The main scientific problem of using form to generate behavior is how to transfer part of the computing tasks that should be handled by the "brain" to the "body", and how to accurately evaluate the morphological calculations undertaken by the body. The research work in this area combines the mechanism, material In addition to artificially designing structures with various functional forms, great progress has been made in theoretical models of morphological calculations, including reserve pool calculation models and information theory analysis methods. It is used to guide the design of morphological calculations. The "curse of dimension" (Curse of dimension) problem commonly encountered in the field of high-dimensional robot behavior control may be effectively overcome by relying on the advantages brought by morphologies, so we can also call it correspondingly "Bless of morphology".
Nevertheless, the current research work on morphological calculations is mostly limited to relatively simple structures, and morphological calculations are mostly used in the research work, but there are few studies on the feedback control of the morphological structure itself. In particular, most of the existing work only uses the limit However, it is far from enough for flexible control tasks to obtain the equilibrium state by means of loops.
Finally, it can be noticed that the structural shape design methods in this section mainly rely on the designer’s inspiration, and it is difficult to form a systematic design idea. With the development of artificial intelligence technology, if efficient optimization methods and machine learning can be used It will be a very potential research direction to optimize the shape design by using technology, and the research work in this area has received extensive attention. The next two sections of this paper will summarize the related progress around the control and automatic generation of shapes.
3. Learning-Based Morphological Control
The mainstream method of using learning to achieve shape control is reinforcement learning. Its core idea is to use the interaction between agents and links to learn strategies. However, embodied intelligence has high requirements on the structure of agents. When designing a controller for such a complex shape, traditional reinforcement learning ignores the shape characteristics, and often simply splices the observations of different parts and directly outputs all the control variables, which leads to a large search space and is difficult to identify in different parts. At present, the main problem facing the learning-based morphological control is how to effectively integrate the morphological information of the agent into the learning process of the controller to improve the learning efficiency. This section first introduces the reinforcement learning for specific morphologies The basic issues that the method focuses on include how to introduce morphological information into reinforcement learning, how to realize the migration between morphologies, and how to deal with faulty morphologies, etc. (Section 3.1). ) and Transformer (Section 3.3) methods, and introduce their research progress.
3.1 Reinforcement Learning Approaches for Morphological Control
Due to the different forms of embodied agents, it is very difficult to design a controller that can adapt to different forms. Literature [64] proposes a method to decompose robot forms and tasks in the policy network of reinforcement learning. In order to design a unified policy , literature [65] pointed out that the strategy should determine the action behavior at the next moment according to the current state and the ability of the agent itself. Therefore, the control strategy πθ(⋅) parameterized by θ should not only be related to the state st , should also be related to the Morphological latent embeddings (denoted as vh)vh) of the agent’s morphology. In order to solve the vector representation of the morphology, the author proposes a strategy of explicit encoding and implicit encoding. Explicit encoding is to convert the embodied The relative poses of different joints of the agent are concatenated one by one to form a representation vector, which is convenient for modeling the kinematic structure. This encoding method uses some prior information, but its encoding method is limited to simple splicing, so it is only suitable for serial forms. More complex forms are difficult to apply. The method of implicit encoding is to optimize the strategy πθ(at∣st,vh) in the process of optimizing the iterative learning strategy πθ, and also iteratively optimize the representation vh of the form, that is, the algorithm not only searches The optimal mapping from the state to the action, and the optimal representation of the shape can also be found. Although this method has a good migration ability on the same type of agents with different degrees of freedom, it needs synchronous iterative optimization during the learning process. Computing the embedded representation of the shape not only brings new optimization difficulties, but also does not make full use of the agent's own shape prior information.
The main purpose of using the reinforcement learning method to realize morphological control is to solve the migration problem between different morphologies. For agents of different morphologies, since the corresponding state space and action space are different, direct policy transfer is difficult to implement. Therefore, literature [66] proposes to use the strategy of hierarchical decomposition, only the high-level strategy is transferred, and the underlying strategy is still learned independently. However, due to the coupling of the high-level strategy and the bottom strategy, there is a problem in this method, that is, if different forms of intelligence If the underlying strategies of agents are significantly different, the transfer of high-level strategies is also difficult to succeed. Therefore, the author introduces mutual information to minimize the difference between the behavior of the shape and the bottom layer, so as to achieve the alignment of the bottom layer strategies of different forms of agents.
In addition, literature [67] regards tools as part of the body (Tool-as-embodiment), and uses the same representation space to represent the relationship between hands-objects and tools-objects, so a single strategy can be used to recursively manipulate objects . Literature [68] proposed a method of adversarial reinforcement learning for the problem of morphological faults.
Generally speaking, integrating morphological information into the reinforcement learning mechanism to achieve transferable policy learning has become a key research issue in morphological control. Affected by the complexity of robot morphologies, the early research work is relatively scattered. In recent years, graph neural network, Transformer The rapid development of tools such as , provides new and effective ideas for the efficient learning of embodied form control.
3.2 Morphology Learning Control Based on Graph Neural Network
In the field of reinforcement learning, there are many works that introduce graph structure to improve learning efficiency, but most of them are limited to using graph structure to describe the environment of the agent, rather than the shape of the agent itself[69-70]. In fact, many robots, Even the body shape of animals can be described as a discrete graph structure G=(V,E). The nodes v∈V of the graph can represent the joints of the agent, and the edges e∈E can represent the dependencies between the joints (which can be is physical or non-physical). A powerful tool to describe this relationship is the graph neural network (Graph neural network, GNN)[71]. In the graph neural network, each node uses its own historical state and received Messages from other nodes are used to update its own state, so it can use the synchronous message passing mechanism similar to the distributed computing architecture to realize forward reasoning, which has great advantages in dealing with different forms (corresponding to different state dimensions and action space dimensions). This feature has attracted the attention of many scholars. Literature [72] proposed a model called NerveNet to integrate the morphological structure information of the agent into the controller learning (see Figure 8(a)). NerveNet Each node in obtains the input information it needs from the observation vector, and then passes it to the neighbor nodes as a message after processing it, and updates the hidden state of each node. Assume that the strategy corresponding to the kth actuator for
, then the combination of output models of all nodes produces a control strategy:
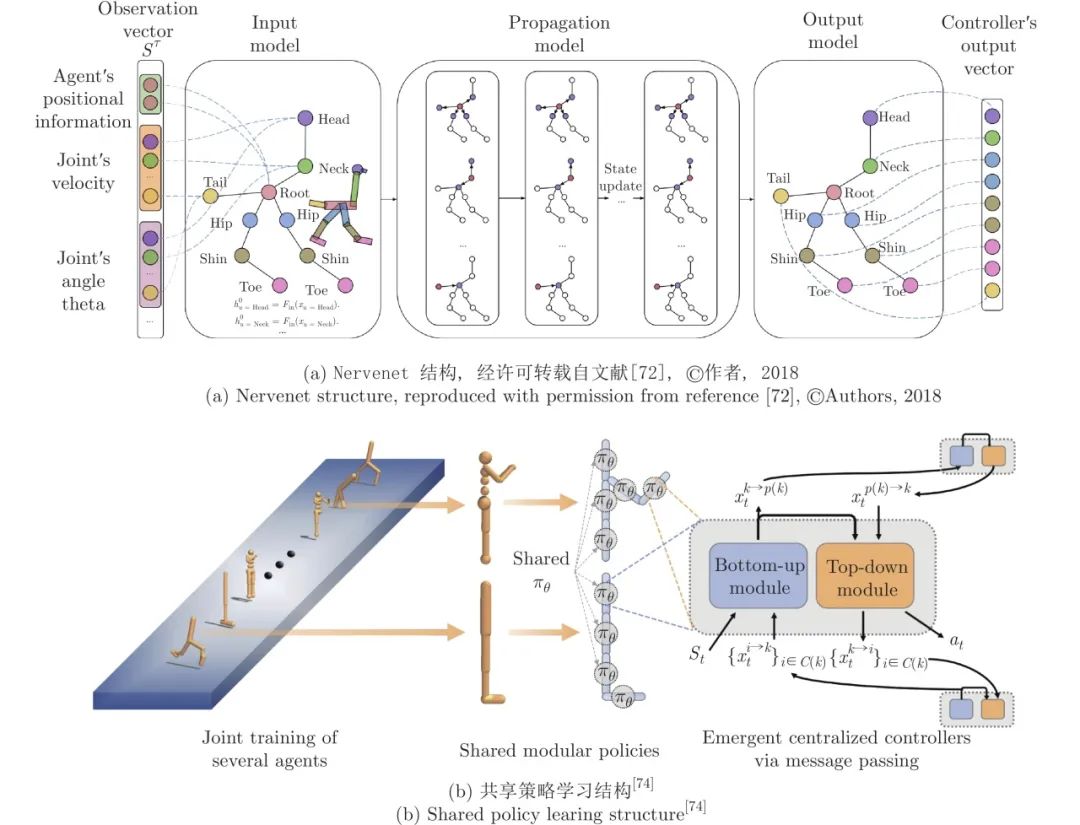
Among them, the set O is the set of output nodes. The whole learning process can be described as a reinforcement learning problem. This method of fully integrating the morphological structure is conducive to the realization of structural transfer learning (including the transfer of morphological size, and structural faults, etc.). 9 is the graph structure corresponding to the robot form in some typical MuJoCo and OpenAI Gym simulation environments. Literature [73] introduces parameter freezing technology to train graph neural network on the basis of NerveNet to solve high-dimensional continuous control problems.
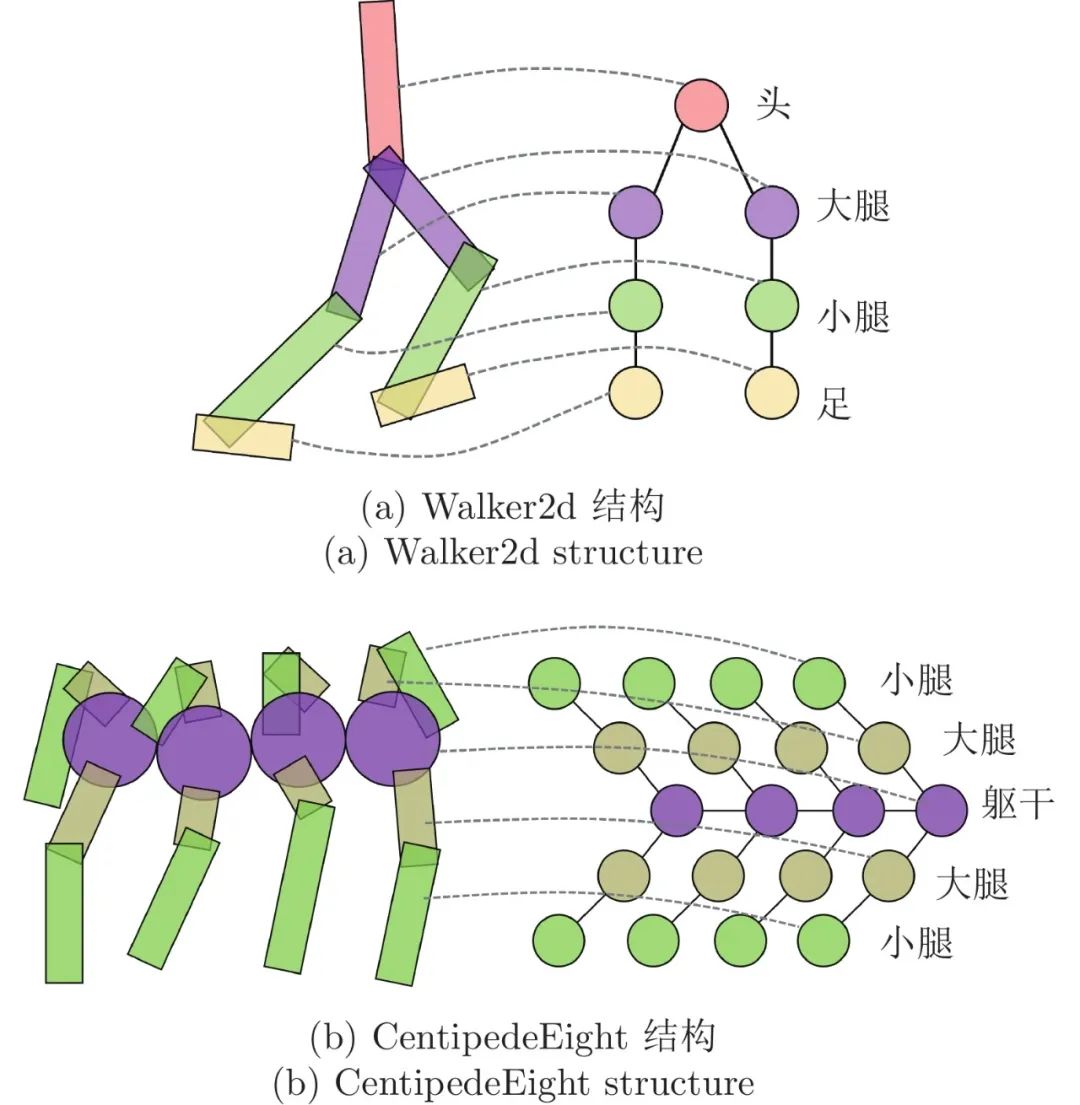
Although the method proposed in literature [72] effectively utilizes morphological information, different strategies need to be designed for each different node in the graph structure (actually corresponding to physical parts such as necks and legs). Literature [74] uses the graph structure to propose The shared policy learning structure of different forms of agents is shown (see Figure 8(b)). The core is to decompose the form of agents into different independent modules. That is, the behavior of the strategy is expressed as πθ(st,vh), where the parameter θ is shared by all modules. The strategy of the kth module is expressed as:
in,
Recorded as the state corresponding to the kth module,
record the messages of all nodes in its neighborhood node set C(k) inputting node k, and f(⋅) is a self-defined message aggregation function. This design can independently design control strategies for independent modules, so that it can be used for different forms It realizes unified control and provides a useful idea for the pre-training model of controller design.
Recently, literature [75] also used graph neural network to describe the type structured information between modules (such as legs, wheels, torso, etc.), pointing out that similar structure modules can share control strategies, and incorporate them into model-based reinforcement During learning, the search space is significantly reduced and applied to actual physical verification (see Figure 10).
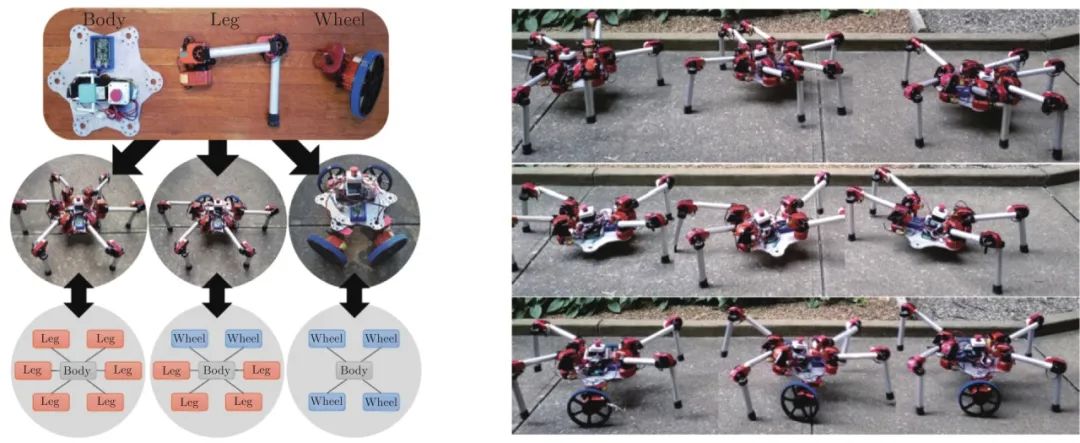
On the whole, the basic starting point of using the graph neural network is that the shape of the agent can generate an inductive bias (Inductive bias) that is beneficial to the learning of the controller. Further research is needed on the efficient migration between different morphologies.
3.3 Transformer-based morphological learning control
Although the graph structure plays an important role in the shape control, since the shape of the embodied agent is generally a sparse graph structure, a lot of key information is easily submerged in multiple rounds of message passing, which leads to "over-smoothing" (Over-smoothing) ) problem. In recent years, Transformer, as a model based on self-attention mechanism, has received high attention [76]. If the attention is designed as an edge-to-vertex aggregation operator, Transformer can be regarded as a graph on a fully connected graph Neural network. Therefore, the literature [77] directly uses the Transformer to realize the message transmission between different components (see the dotted arrow in Fig. This method fully embodies the potential of the Transformer, but it ignores the influence of the real physical form of the robot. Literature [78] further revealed the role of the position information of the agent node on the self-attention mechanism, and the form (mainly position) The information embedding Transformer model (see Figure 11(b)), which is used for joint policy learning of heterogeneous forms, overcomes the over-smoothing problem caused by the sparse structure in conventional graph neural networks.
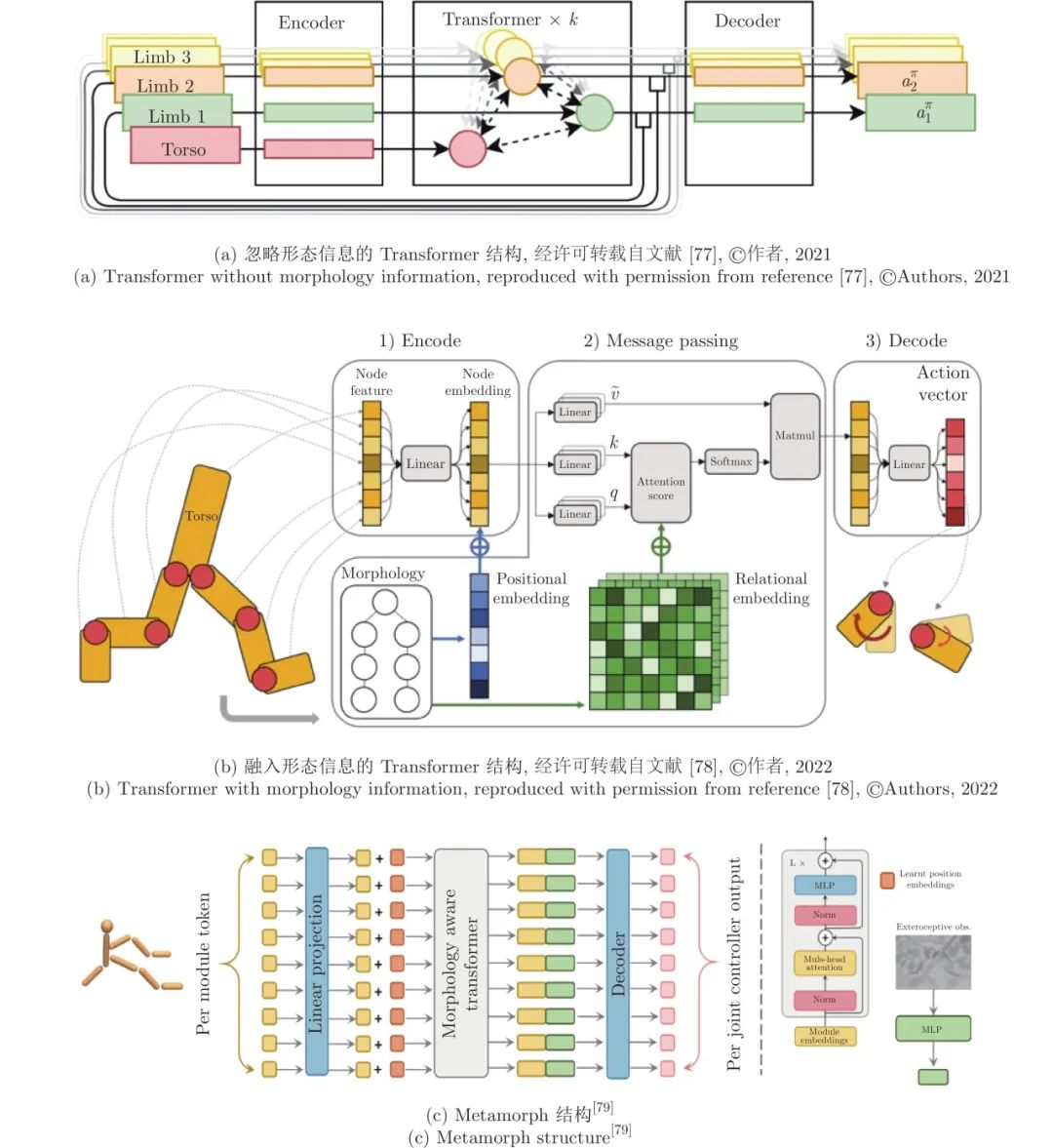
Further, literature [79] aims at the large-scale agent morphological control problem, regards the morphological form as an input Transformer modality, and learns a general strategy by constructing a "metamorphic" (MetaMorph) to solve a large number of different morphological control problems at the same time ( See Figure 11(c)), laying the foundation for large-scale pre-training of embodied morphological learning.
3.4 Summary
Affected by factors such as high dimensionality, nonlinearity, and strong coupling, it is a very challenging task to realize the morphological control of complex agents using reinforcement learning methods. However, the shape of the robot itself provides very important and useful prior information, which can significantly Landing constrains the search space. Therefore, the main scientific problem in this area is how to introduce the shape information into the learning algorithm in an appropriate way to improve the learning efficiency, and to ensure the performance when migrating to other unknown shapes, so as to reflect the "morphological blessing". The current representative methods in this regard include using the graph neural network to represent the morphological structure, and using the Transformer structure to describe the morphological characteristics. At present, these works are still mainly concentrated in the simulation environment, and the learned strategies will encounter problems when they are transferred to the physical system. On the other hand, although it is very attractive to design a unified morphological controller according to the characteristics of different morphological robots, it is also quite difficult. Inspired by the pre-training of large models in the fields of language and vision in recent years, Whether a unified pre-training large model can be established for different forms of agents is also an important development direction in the future.
4. Learning-based morphology optimization
The above two sections respectively reviewed the important developments in morphological calculation and morphological control. Among them, the morphological calculation part requires manual design of delicate structural shapes, which is a very challenging task for designers. If the process of morphological design can be automated Realization can significantly promote the research of morphological intelligence. On the other hand, the morphological control part mainly integrates morphological information in different forms under the learning framework to improve the learning efficiency and generalization ability of the controller. This learning-based controller The design idea can also be naturally extended to the shape design, so as to realize the joint optimization learning of shape and control.
The realization of brain-body co-evolution by using learning ideas has received sufficient attention in the early research of embodied intelligence [4], and is sometimes called evolutionary robots, artificial life, etc. However, early research mainly focused on using evolutionary learning algorithms to optimize specific The control strategy of the morphological robot does not affect the shape of the robot [80].
Literature [81] was the first to use the evolutionary learning framework to realize the collaborative optimization of shape and controller in a virtual environment. This literature expressed the structure of a three-dimensional rigid robot as a directed graph gene representation, and used the evolutionary algorithm on the graph to optimize the shape design of the robot . This work has received extensive attention because it can help robots search for shapes that better match the environment and tasks[82-88]. In particular, literature[83-84] utilizes variable-length cylindrical Evolutionary robot (see Figure 12). In this paper, the walking ability is used as the evaluation of the fitness function, and the evolution can be completed after about 300 to 600 generations of iterations in the simulation environment, and the commercialized rapid prototyping technology is used to transform it into a physical system (the motor needs additional installation). This study also gave some interesting findings. For example, there is no specification of morphological symmetry in the algorithm, but the final generated morphologies show strong symmetry to a certain extent. Because Without the use of sensors, the designed robot can only produce different modes, shapes, and actions, but cannot interact with the environment.
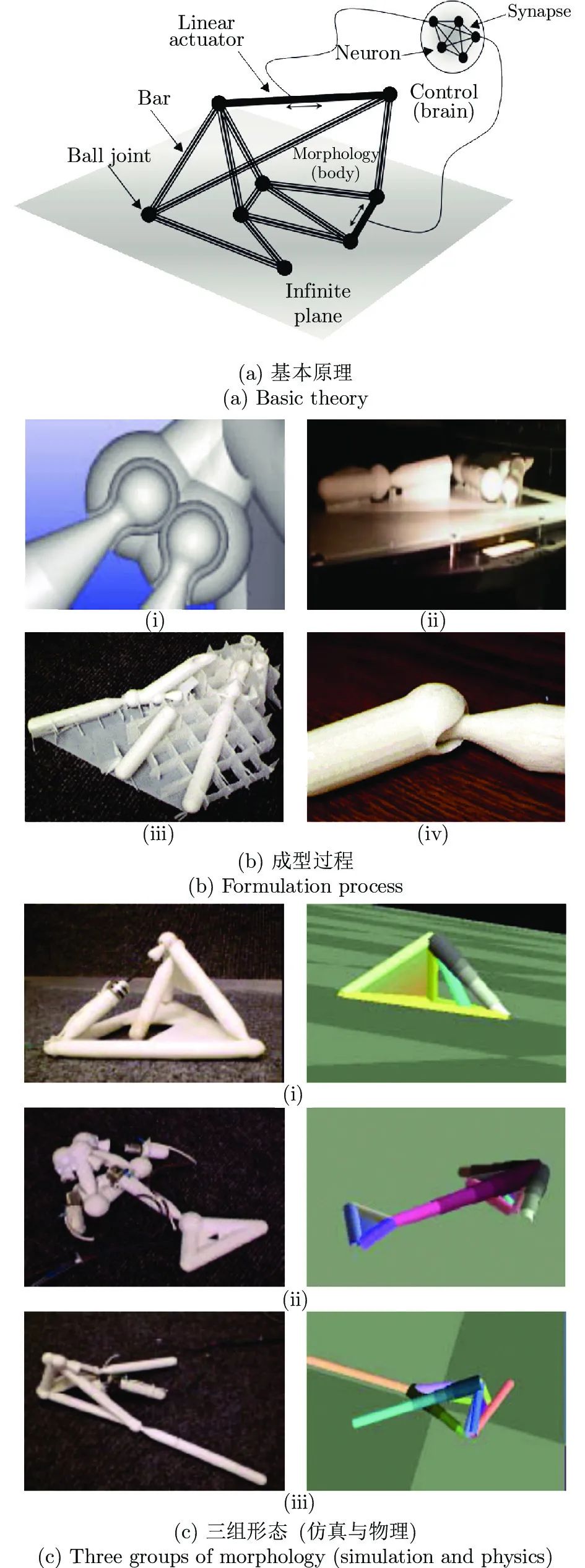
Literature [89] further developed this idea, and designed an indirect encoding strategy by using Compositional pattern producing networks (CPPN) for the spherical shape connected by revolving joints, which can effectively realize the generation of multi-resolution shapes. And realized the co-evolution of shape and control (see Figure 13). Literature [90] pointed out that the co-evolution of shape and control is similar to the simulation of brain-body coordination, but ignores the influence of the environment. The morphological evolution strategy of robots under changing conditions verifies the effect of environmental complexity on morphological complexity, and constructs more complex morphologies using the voxel method based on triangular meshes.
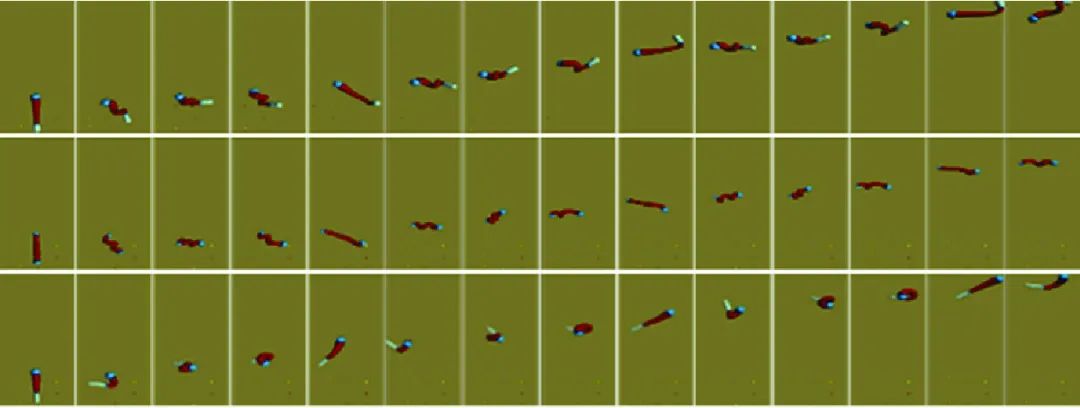
Although the collaborative optimization of shape and control has made some progress at the beginning of this century, the optimization process is limited by the limitations of software and hardware simulation conditions, so no major breakthroughs have been made. In the past ten years, with the development of 3D printing With the rapid development of advanced additive manufacturing technology, graphics simulation and rendering technology, and computing power technology represented by GPU, methods including evolutionary optimization and reinforcement learning have made great achievements in the collaborative optimization of shape and control, and have been It has been extended to different robot forms including operation and software [91]. This section will illustrate the application of several methods including "evolutionary reinforcement learning" in form-control collaborative optimization from the perspectives of simulation environment and physical environment.
4.1 Collaborative optimization in simulation environment
In the early stage of research, the collaborative optimization of shape and control was mainly based on evolutionary search methods, such as literature [81, 83, 89], etc.; in recent years, the work in this area has mainly focused on the study of different morphological coding methods for specific task requirements [92 -94], but the existing problem is still that the evolutionary search is difficult due to the large parameter space (including the search of morphological parameters and the search of controller parameters).
Although morphology and control should be jointly optimized, the two are actually optimized on different scales. Taking organisms as an example, the change of morphology (including structure and parameters) is more similar to an evolutionary process, that is, in the long-term environmental adaptation process Optimize its own structure and parameters through the evolutionary process; while the design process of the controller is more similar to the acquired learning process, that is, after the shape is determined, it will try hard to reach the limit of the exercise ability in its own life. Therefore, it is not difficult to see that, A very natural idea is to use the evolutionary optimization method to optimize the morphological structure and parameters, and use the reinforcement learning strategy to realize the optimization of the control structure and parameters. The two are nested in two loops, where the evolutionary optimization method is the outer loop, Reinforcement learning is an internal cycle. On the basis of the graph neural network controller proposed in [72], literature [95] describes the shape design of the robot as a graph search problem, introduces the concept of population, and designs a controller that can add and delete nodes. Mutation operator, realize evolutionary search on graph. Using graph neural network as controller, parameters can be shared between controllers, thus greatly reducing the time of controller learning, which is conducive to efficient evaluation of morphological performance. Literature [96 ] developed an environment called "evolutionary playground" and developed a computational framework called "deep evolutionary reinforcement learning" to explore the relationship between embodied intelligence and the environment. This paper also uses this morphological evolutionary learning mechanism Verified the "Baldwin effect" in evolutionary biology.
Since the evolutionary algorithm is essentially equivalent to zero-order optimization, the efficiency is low. Relying on the rapid progress of reinforcement learning in recent years, some scholars also try to use the reinforcement learning method to unify the optimization form and control. For example, literature [97] combines design parameters with The control parameters are jointly calculated by the proximal strategy optimization method. Since the shape search space is too large, and the search of shape and control is difficult to decouple, learning convergence is very difficult. Therefore, the author restricts the space of shape search, and can only target the specified The shape optimizes the parameters of the robot components, but does not optimize the structure of the robot (see Figure 14). On this basis, the literature [98] uses reinforcement learning to realize the shape search and policy control learning for legged soft robots, and further realizes the Migration from simulation to physics.
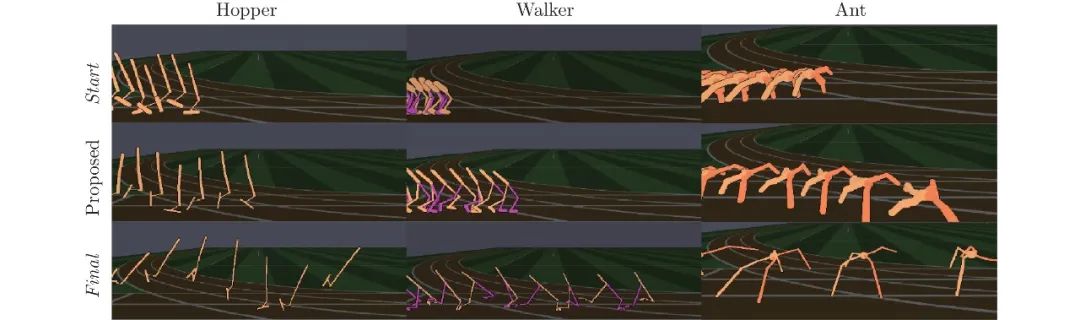
Literature [99] aimed at the self-assembled morphological agent, unified the form and control in the action space, described the morphological search and control design as a reinforcement learning problem, and designed the corresponding dynamic graph network controller to make its form and agent Morphology matching. Literature [100] uses reinforcement learning to realize the joint learning of shape and control strategy for the obstacle-crossing problem. Literature [101] separates shape transformation and control optimization into two stages in the learning process and uses the policy gradient method to jointly optimize shape and control strategy. Control action. Recently, literature [102-103] introduced the graph neural network into the reinforcement learning framework of the collaborative optimization of shape and control, which provides a feasible way for the physical transfer of shape learning.
At present, some researchers have tried to apply the joint optimization method of shape and control to the design optimization of the shape of the manipulator. The main problem in this regard is to make the optimized shape adaptable to the objects to be manipulated and grasped. Literature [104] put the evolutionary strategy It is applied to solve the shape optimization of the manipulator, and the search efficiency is improved by introducing the Graph element network, and the fine-tuning of the shape of the two fingers for the customized two-finger gripper is realized (see Figure 15(a)). Literature [105] Aiming at three types of grasping tasks such as Power grasp, Pinch grasp and Lateral grasp, projecting the shape parameters and control parameters of the manipulator into the public latent space (Latent space), proposed to use the Bayesian optimization algorithm to search for the best The method of manipulating the shape of the hand (see Figure 15(b)).
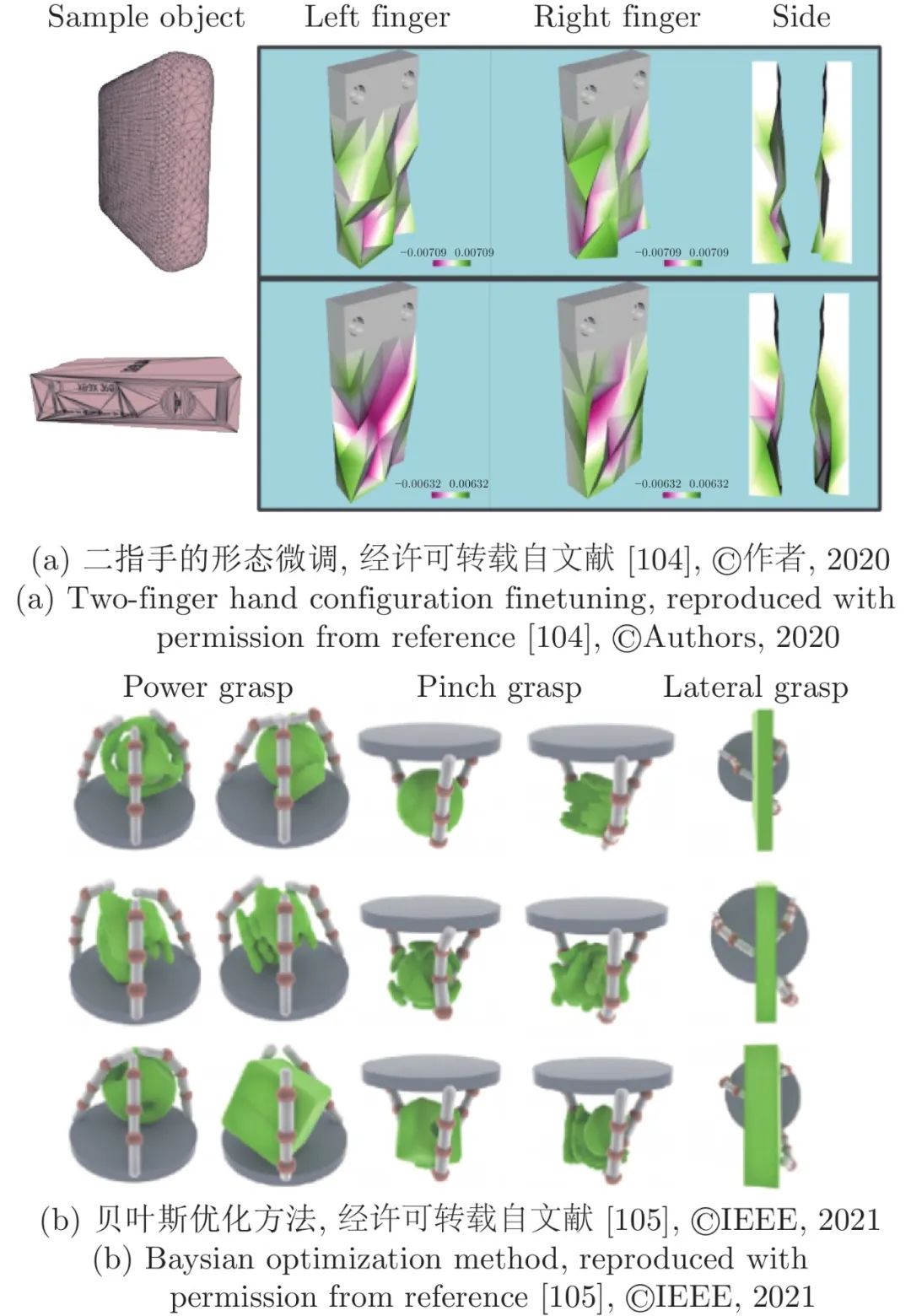
Recently, from the perspective of computer graphics, literature [106] designed a method for general shape representation using Cage-based deformation model (CBD). The main advantage of this method is that it can describe rich shapes with fewer parameters. By combining this model with a differentiable simulator, an end-to-end learning method is formed.
4.2 Collaborative optimization in physical environment
Although the evolutionary optimization, reinforcement learning and other strategies used in the joint optimization process of shape and control make the whole learning process need to be carried out in a simulation environment, people have not given up the effort to realize the learned shape in the physical world. In fact, there is also Only the performance verified in the physical environment can better reflect the effect of learning. As early as 2000, literature [83] studied the use of 3D printing technology to make and realize the evolved form, but also found that in the simulation and physical world There is a big difference between them, so that it is difficult to achieve the expected effect after the shape with satisfactory performance is obtained in the simulation environment[107]. To solve this problem, the literature [108] proposed a continuous shape modeling method. Literature[108] 109] studied the method of integrating the migration effect from the simulation to the physical environment into the optimization process. Literature [110] further studied the relationship between the simulation physical difference and the morphological complexity for the flapping wing aircraft, and pointed out that this relationship is not monotonous .
How to physically realize the shape obtained through morphological learning, there are currently several different solutions, including using 3D printing technology to make some parts, and then installing them on the existing robot platform[111]; or making parts directly through 3D printing , and then assembled. Of course, the most direct way is to implement morphological learning directly on the hardware platform.
Most of the current work stays in simulation, and some work is aimed at different types of robots, first performing morphological evolution in the simulation environment, and then using migration technology to migrate to the physical system, such as legged robots [112-113], soft robots [114] , Modular Robots[115] (see Figure 16). Most of these works follow the “simulation-to-physics” migration model. Literature [115] developed a software simulation system called RoboGen, which can be used for the morphological evolution of robots, and is compatible with Combined with 3D printing, the homework for graduate course training is designed.
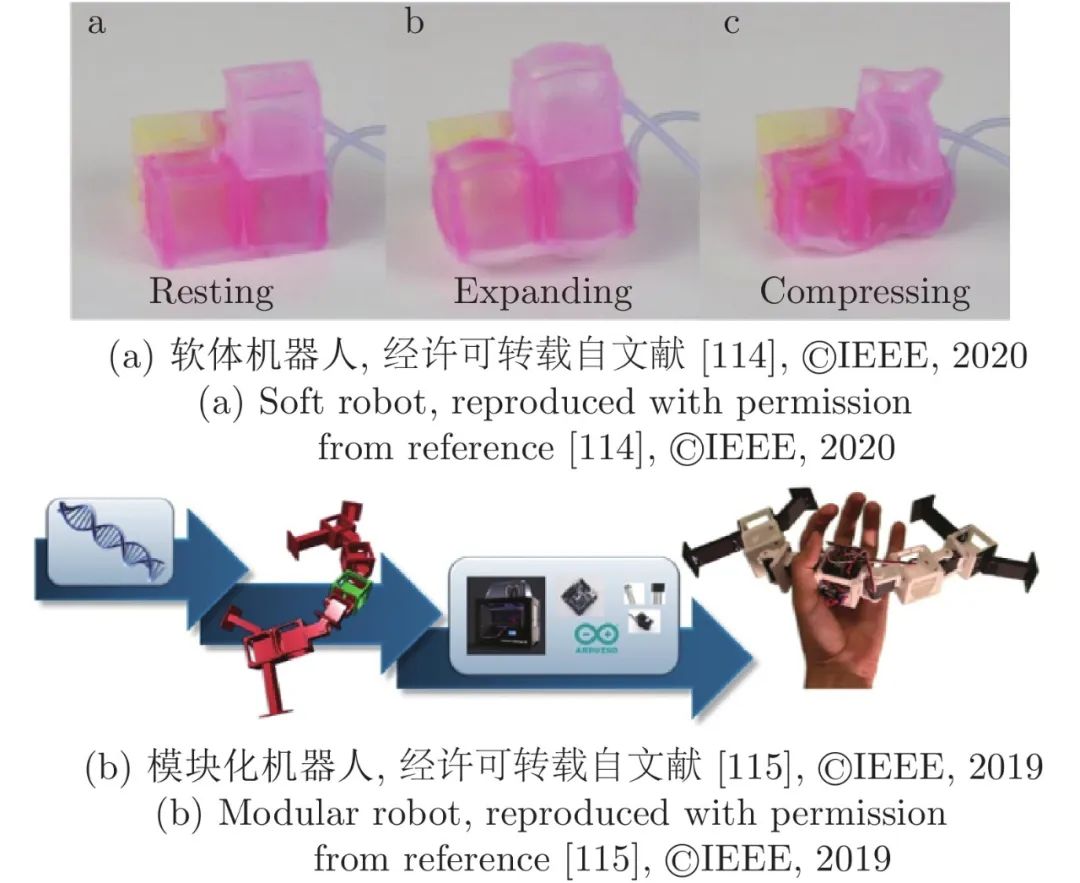
With the increasing variety of robot components and the continuous reduction in cost, the morphological adaptation and evolution of direct physical systems become possible. Literature [116] uses a robotic arm to manipulate different modules (including active and passive modules). Gene coding, as well as the actual ability evaluation of the assembled form (such as walking ability) to achieve evolutionary optimization selection, thus realizing the direct morphological evolution of the physical robot system (see Figure 17). Of course, limited by the scenarios set in the paper , the research in this paper is actually the corresponding physical realization of the simulation system, which is still far away from the actual robot morphological learning. Recently, in the four-legged robot designed in literature [117-118], straight lines are installed on the femur and tibia The length of the motor can be extended by 50 mm and 100 mm respectively[119] (see Fig. 18). Based on these optimization degrees of freedom, the method of direct learning of physical morphology evolution is completed, and experiments are carried out on different terrains. Overall, the In other words, the study of morphological evolution directly on the physical system is still at a very early stage, and the parameters that can be optimized are also very limited.

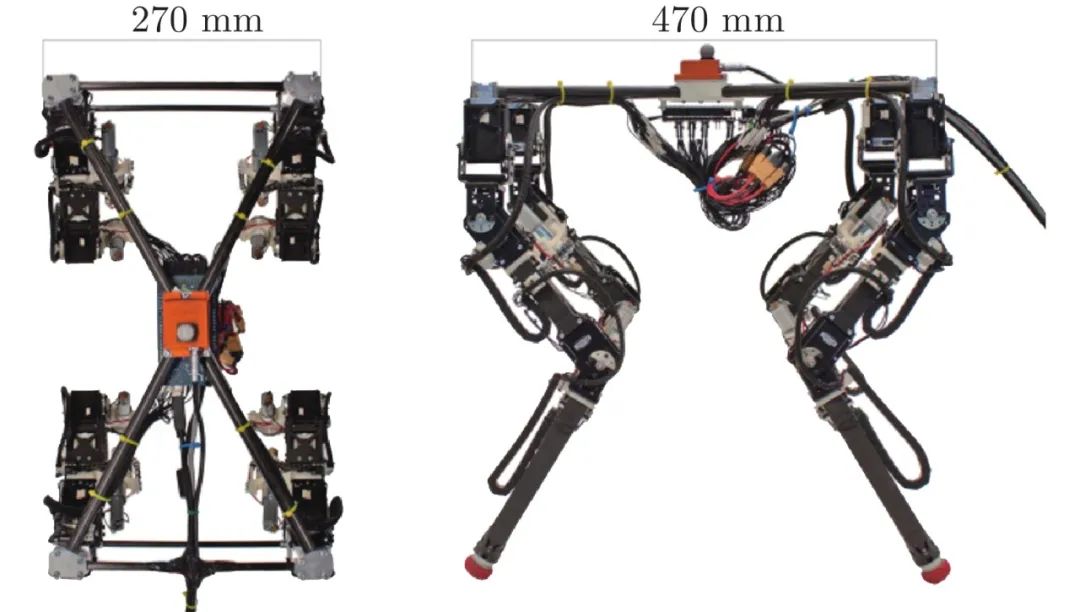
4.3 Summary
In general, the main scientific problem of using learning to optimize morphology is how to efficiently realize the coupling optimization of morphological structure and control. The related work listed above is mainly summarized into the following three types.
1) Evolutionary optimization is used for both morphology and control. This type of method is easy to implement, but the search efficiency is very low, and the coding of morphology and control needs to be optimized according to the characteristics of the problem. In addition, due to the limited optimization space of the controller, it is difficult to learn more precise The control action is suitable for scenes that do not require high control precision but require high morphological structure.
2) Evolutionary optimization is used for morphology, and reinforcement learning is used for control. This method comprehensively utilizes the advantages of evolutionary optimization and reinforcement learning. In particular, how to comprehensively coordinate the multi-time-scale coupling relationship of phylogenetic morphological evolution, ontogenetic control optimization, and ontogenetic intellectual learning , is also an issue that needs to be focused on in the future.
3) Both form and control use reinforcement learning. This method uses reinforcement learning to optimize the parameters of form and control. The method is relatively intuitive, but it is limited by the search ability of reinforcement learning. The parameters of the search form under constraints are suitable for fine-tuning scenarios where the morphological structure has already been relatively formed.
Most of the current research work is still verified by the simulation environment, and the optimization of fine materials has not yet started. How to transfer the results of morphological evolution to the physical system, or directly evolve based on the physical system, is a frontier issue worthy of further discussion in the future. In addition , it is worth noting that there are already many mature rules and experiences in the field of mechanism science for shape design, how to introduce these empirical information, as well as related physical constraints and external knowledge guidance to combine data-driven learning methods to optimize shape and The design of control will be a sharp tool to improve learning efficiency.
5. Typical case — soft robot
The above work focuses on method research, so it is mainly aimed at some typical embodied agents in the simulation scene. The research work related to morphological intelligence should be closely related to specific tasks. In recent years, soft robots have characteristics, and has achieved considerable development[120]. Compared with rigid robots, soft robots have great advantages in driving on complex roads and operating on unknown targets. Due to its complex body dynamics (high-dimensional , elasticity, etc.), the control of soft robots is very challenging, but from the perspective of embodied morphological computing, such complex dynamics are valuable computing resources. Soft robots themselves can be regarded as a morphological computing device, and have become It is an ideal platform for research on embodied intelligence, but because most of its important achievements have been made in recent years, and it has been influenced by the research on theoretical models of embodied morphological computing during the development process, so this article will describe it separately.
5.1 Morphological Computation Based on Soft Robots
Many studies have verified that soft bodies can achieve complex motions by changing their own geometry and mechanism characteristics[121-125], but most of the research is limited to the passive adaptation of soft bodies to the environment, and there are few studies on active environment detection. Literature [126 ] pointed out that the wrinkles produced by soft materials soaked in water for a long time can help realize some computing tasks, and apply it to active tactile perception. Literature [127] uses soft haptic morphological computing to realize active distance detection. In addition, literature [128] For the soft gripper, the method of adjusting the damping behavior of soft silicone was studied, and the dynamic shape calculation was realized. Literature [129] listed several shape design methods of soft robots inspired by the shape of animals and plants. Advanced Robotics and IEEE RAM in Both 2018 and 2020 held morphological computing specials, emphasizing the important position of soft robots [22-23].
The calculation of the physical storage pool can also be realized by using soft robots. Literature [130] uses the soft tentacles inspired by the shape of the octopus as the storage pool calculation device, and can simulate complex nonlinear behaviors by deriving linear and static outputs from its physical body. And without an external controller, closed-loop control can be realized by converging to the limit cycle. Based on this work, literature [131] demonstrates the approximation and control capabilities of this type of soft robot for nonlinear continuous functions. Literature [132 ] used this platform to study tasks including time series prediction and anomaly detection (see Figure 19(a)). Literature [133] further used this mechanism to realize the detection and positioning of underwater targets (see Figure 19(b)). In addition, literature [134] expanded the scope of morphological calculation of soft hands and developed a reserve pool model of pneumatic soft hands. It can be seen that soft robots, as a very promising robot form, have become an important development of morphological calculations In this direction, the development of soft robots will definitely drive a new wave of embodied form computing.
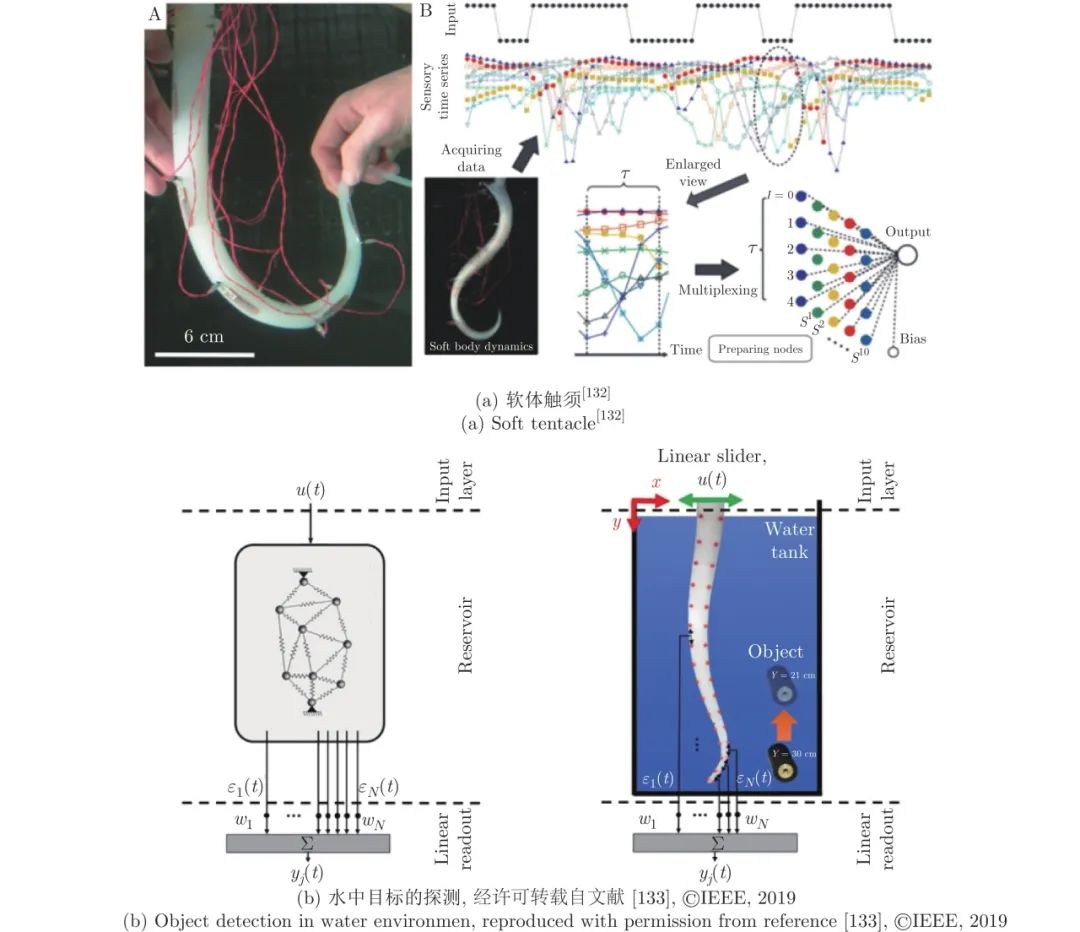
5.2 Morphological control of soft robots
Soft robots are typical underactuated systems (the degree of freedom of the controller is far less than the degree of freedom of the output), due to the influence of distribution parameters and continuum dynamics, it is not easy to control it with high precision and flexibility. There is no general control method for general soft robots. Integrating the morphological characteristics of soft robots in the controller design process is a relatively effective method. Literature [135] explores the role of morphology in the control of soft robots. But it is mainly limited to the mechanism modeling design method .
Due to the complexity of the shape and control of soft robots, it has become an important trend to design more general or transferable control strategies using reinforcement learning and other ideas. However, designing software robot control algorithms using reinforcement learning also faces many challenges. Under the framework of learning, how to effectively represent the state of the soft robot, how to describe the interaction between the soft robot and the environment, how to mine the special structure and material properties of the soft robot, and how to realize the migration between different forms and between virtual and real, etc. Literature [136] reviewed some applications of deep reinforcement learning in the navigation and operation of soft robots. Literature [137-138] also realized the control strategy of soft manipulators for some typical reinforcement learning algorithms. But these works did not repeat the mining of soft robots. morphological characteristics, resulting in low sample usage efficiency, and can only be applied to some simple morphological structures.
As mentioned in Section 3.2 and Section 3.3, if the morphological structure of the soft robot can be effectively expressed and integrated into the framework of reinforcement learning, the learning efficiency can be significantly improved and the transferability can be improved. Inspired by this idea, Some preliminary research work has emerged recently. For example, literature [139] initially tried to introduce graph theory methods to describe the interaction between soft robots and the environment. Literature [140] used graph neural networks to convert the non-rigid motion of soft manipulators to The learning of the learning chain is transformed into learning an explicit connection graph, and an unsupervised learning method for learning this model is proposed. Literature [141] also regards the inverse kinematics model learning of soft robots as a sequential prediction problem, and designs A Transformer structure is used to realize model estimation. Generally speaking, the current research on morphological learning control of soft robots is still very preliminary.
It is worth mentioning that, in order to strengthen the research on morphological learning control, many scholars have developed simulation environments suitable for reinforcement learning of soft robots based on the existing robot learning environment, such as Elastica[142], SofaGym[143], SoMoGym[144] etc. These environments provide convenience for further research on morphological learning control of soft robots.
5.3 Morphological optimization of soft robots
As mentioned above, soft robots have become an important tool for shape calculation and shape control, so the optimization of their shape is also a common concern. An important reason why the research on robot shape evolution has been slow in the past few years is that it mainly focuses on the consideration of rigidity. The finite combination of elements, which is quite different from the material-related evolution in organisms in nature. To this end, literature [145] uses genetic algorithms to realize the shape optimization of voxel-structured soft robots that include multiple material properties. Literature [92] The shape of the voxel model of different materials is studied (it can simply simulate bones, tissues, muscles, etc.), and the advantages and disadvantages of direct coding and indirect coding are analyzed. Literature [146] further embeds the control system into the physical simulation of the robot shape , proposed the so-called Evolved electrophysiological soft robots (Evolved electrophysiological soft robots). Recently, literature [94] realized the morphological evolution of 3D voxel soft robots by direct coding, and applied it to the realization of biological organisms (see Figure 20).
Figure 20 Morphological evolution of soft robots (different colors correspond to different material properties)
Due to the great difficulties in shape optimization, control, and production of three-dimensional soft robots, some related research work has begun to transfer to the evolutionary learning of two-dimensional voxel-based soft robots (Voxel-based soft robots, VSR)[147-148 ]. Reference [149] also used evolutionary algorithms to study the "transformation" phenomenon of soft robots. Most of the work mainly considered the morphological evolution itself, and less consideration was given to the control optimization in the process of environment interaction. In view of this problem, reference [150] proposed a composite structure of evolutionary optimization combined with reinforcement learning to realize the form-control collaborative design of soft robots, and developed the Evolution Gym environment for two-dimensional VSR, covering more than 30 different task environments, including running, climbing steps, etc. , Climbing, carrying objects, etc. The robot in Evolution Gym is composed of many "cells" as the basic unit, including soft cells that can deform freely, rigid cells that are hard, and actuator cells that can actively shrink or expand. This flexible shape allows the robot to freely "evolve" its shape, and finally complete a series of tasks such as movement and manipulation of objects on different terrains (see Figure 21).
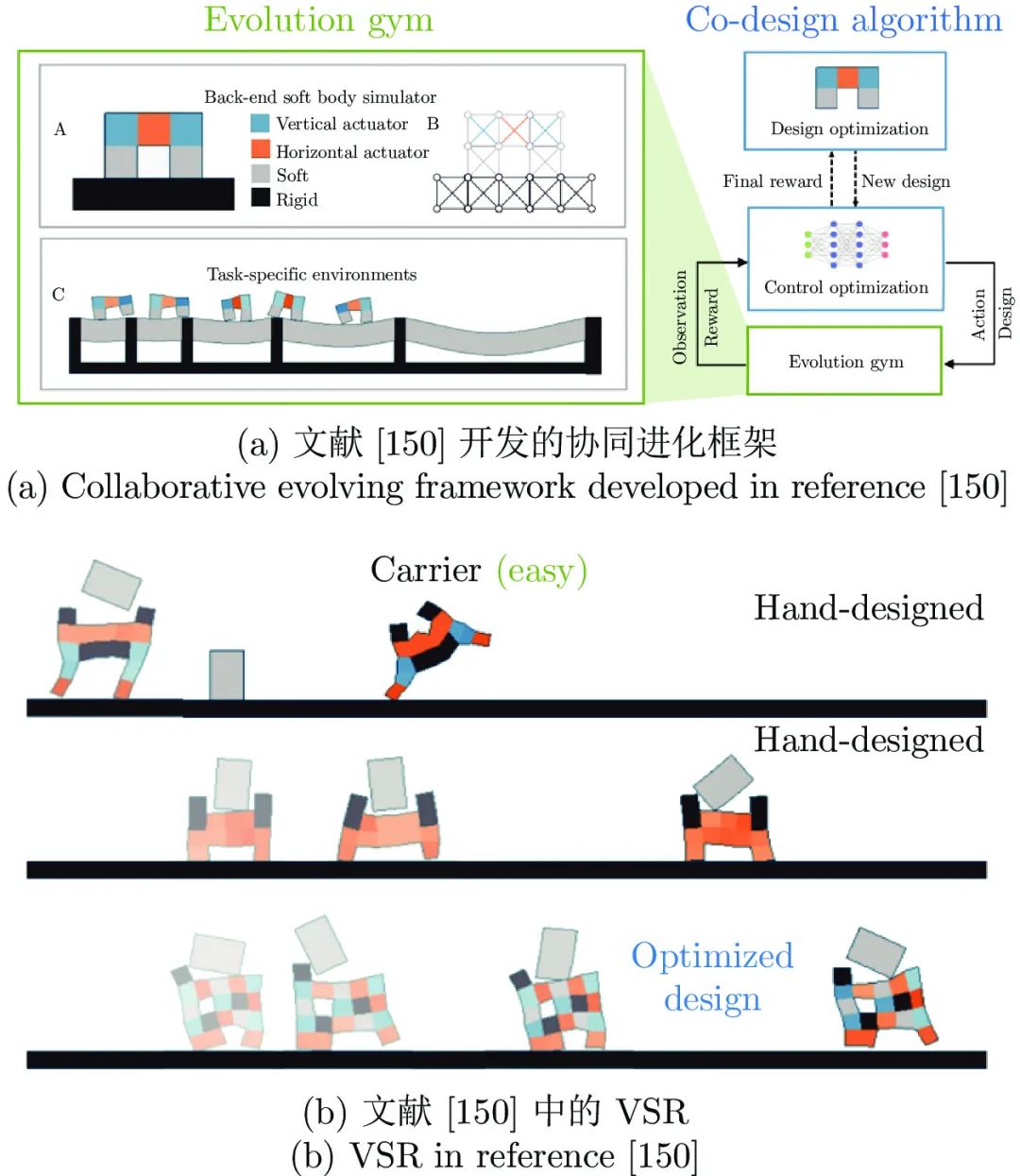
6. Summary and Outlook
This paper discusses the three important issues of morphological computing, morphological control, and collaborative optimization of morphological-controlling, and reviews the relevant important progress. Specifically, we analyze the behavior of morphological computing based on physical devices and theoretical models. In this paper, the learning-based morphological control is analyzed from different algorithm perspectives, the learning-based morphological optimization is analyzed from the perspectives of simulation environment and physical realization, and the related progress is analyzed in detail by taking soft robots as a typical case.
What needs to be emphasized is that in the architecture in Figure 1, the dotted line represents "using behavior to realize learning" is the most cutting-edge direction in embodied intelligence research. Although machine learning has been developed as an important branch of artificial intelligence for many years, However, its main learning paradigm is still learning based on collected data samples, and it does not pay enough attention to the sample collection process. Technologies such as active machine learning have considered the use of samples to a certain extent, but have not yet solved the problem in the process of interacting with the environment. However, the learning mechanism based on the agent’s embodied behavior can integrate data collection and model learning, and truly realize active interactive learning, which is also a more advanced simulation of the human learning process. But currently The research work has just started, and its main feature is to study how to efficiently obtain training samples through navigation for mainstream visual perception tasks. For example, for target detection tasks, literature [151] introduces a semantic curiosity mechanism as embodied intelligence The literature [152] further introduces the self-supervised learning mechanism to design a method for continuous improvement of the object detector. Literature [153-154] carried out related research on object segmentation and 3D object detection. Literature [155] also studied the direct use of the robot's physical characteristics to realize joint visual-tactile feature learning. The research work in this area is still developing rapidly.
As a summary of the full text, we regard the above-mentioned aspects as a whole, and re-examine this issue from the macroscopic perspective of embodied intelligence. Figure 22 is an overall summary of this issue. Many, relying on morphological computing to realize morphological control is the core of morphological intelligence, but the functions that can be realized at present are still relatively limited. For complex morphological and functional requirements, most of them still need methods based on reinforcement learning. Further, on the basis of morphological control combined with evolutionary Optimizing the search for a suitable shape is the future development direction of shape design and generation. At present, the work in this area has made great progress, but it is still difficult to support the generation of complex shapes in real physical environments.
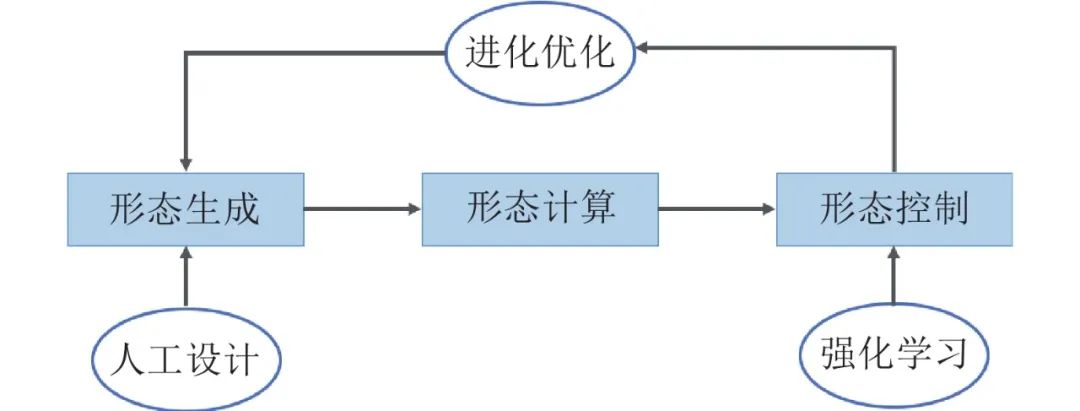
Furthermore, important development directions that are expected to achieve breakthroughs in the future include:
-
1) Morphological emergence: At present, the work on morphological computing and morphological generation is basically separated. In terms of embodied computing, most of them are artificially designed morphologies based on physical constraints. How to combine with morphological evolution and control to realize autonomous morphologies Emergence is the key issue for pushing form-based embodied intelligence to applications in the future.
-
2) Perceptual evolution: Most of the current work on morphological evolution is coupled with the morphological controller, and the goal of its morphological optimization is mainly to obtain better control effects. The actual embodied agent has rich perception and execution capabilities, while The evolution of these perception and execution capabilities are currently untouched issues. In future research, we expect embodied agents to have the evolution capabilities of perception and execution components similar to the laws of biological evolution, so as to achieve more efficient embodied intelligence tasks.
-
3) Physical implementation: Although there are some efforts in the aspects of shape optimization and control learning to transfer the results of simulation optimization to the actual physical system, there is still a long way to go, especially the work of directly optimizing iterations in physical form It is still at a very early stage. With the progress of virtual reality technology and materials science, the migration from simulation to physics is bound to lay the foundation for the physical realization of embodied agents.
-
4) Multi-agent collaboration: The current research work on form-based embodied intelligence is still mostly limited to a single body. Although in the process of evolutionary optimization we can see that a population constructed by multiple agents participates in optimization, they share the optimization goal, Its purpose is only to select the best individual through survival of the fittest. There are no related reports on multi-agent morphological development and control optimization for practical tasks. This is also an important direction for future development [156].
Finally, we emphasize once again that although embodied intelligence is very important, it also has its own limitations. Perhaps the current theory and algorithm tools cannot well support the description, understanding and generation of embodied forms. Embodied intelligence and out-of-body intelligence The close combination of INT is the only way to achieve general intelligence. From the relevant progress summarized in this paper, we can see that connectionist methods including deep learning have been widely used in the research of embodied intelligence [157]. In addition, knowledge graphs, Related technologies of scene graphs have also been introduced into the research of embodied perception [158-160], which can significantly improve the comprehensive performance of scene understanding and navigation control. In fact, symbolism emphasizing representation, connectionism emphasizing computation, and emphasizing Interactive behaviorism is an important approach to the realization of artificial intelligence technology. Research on embodied artificial intelligence should fully absorb nutrients from various fields and strive to solve the generation of intelligent behavior in actual scenarios. In this sense, we should not Too much attention should be paid to why artificial intelligence should be embodied, and more exploration should be made on how artificial intelligence should be embodied.
Disclaimer: The articles and pictures reproduced on the official account are for non-commercial educational and scientific research purposes for your reference and discussion, and do not mean to support their views or confirm the authenticity of their content. The copyright belongs to the original author. If the reprinted manuscript involves copyright and other issues, please contact us immediately to delete it.
"Artificial Intelligence Technology and Consulting" released