1 Overview
Taking into account the application of automobile autopilot, author defogging combined with target detection, the establishment of a network end to end.
FIG generating the mist is typically atmospheric scattering model based on:
where
represented by FIG fog,
represents the true picture,
and
are two key parameters, respectively represent light transmittance FIG atmosphere,
,
atmospheric scattering coefficient,
from the camera to the object is. Therefore, the true picture and can be expressed as
On the AOD network draws its application to video defogging, combined Faster-RCNN apply it to the video object detection, as shown shelter of the final model.
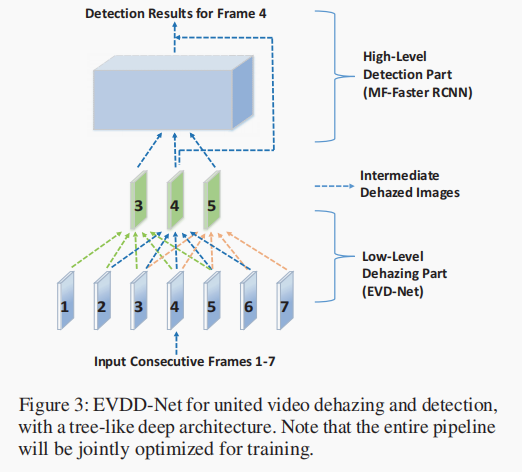
2. AOD
AOD network structure as shown below:
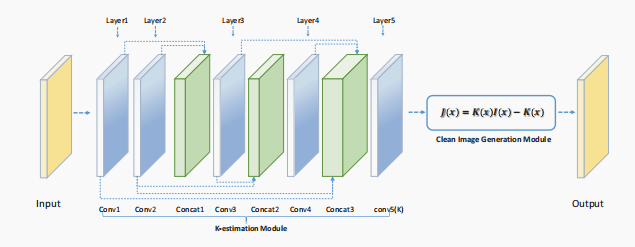
AOD formula (1) can be rewritten: 其中 将 和 合并到新变量 中。
2.1 Pipline
- 对输入 提取特征输出 ,
- 应用公式(3)输出清晰图像。
3 AOD应用到视频去雾
Since AOD for defogging a single image, which the authors has been improved to handle video defogging problems, the main problem is that the mixing (temporal fusion) consecutive frames . Because successive frames intrinsically linked, so the use of multi-frame coherence of video defogging have great prospects.
Three Strategies 3.1 Hybrid consecutive frames
The authors also 5 (later explain why 5) pictures input to the network, in three different phases which are fused, analyzed and compared their results
- I-Level Fusion: will cancatenate five branches in the input stage.
- K-Level Fusion: the five branches of the picture feature maps were concatenate the K estimation phase.
- J-Level Fusion: The fused five branches wherein the output stage.
The author of AOD as the initialization parameter values to facilitate the training model.
3.2 The choice of hyper-parameters
By contrast the final test, as the number of input select five consecutive frames (3 too, seven consecutive frames not so much caused by the bite Information) in 3,5,7; K-Level Fusion selected as a feature fusion strategy.
4 loss function
Select the MSE as a function of loss
5 Target Detection
slightly