Reprinted knew almost
Meow, recently did not brush Lock, sin sin, to no nutrients and cried Haw
Like most understandable to explain a thing.
First, the covariance:
It can be understood as popular: two variables change in the same direction in the process of change? Or changes in the opposite direction? In the same direction or reverse what extent?
You become big, I also increases, indicating that the two variables change in the same direction, then the covariance is positive.
You become larger, and I become smaller, indicating that the two variables is the reverse change, then the covariance is negative.
From the numerical perspective, the greater the value covariance, two variables larger extent also in the same direction. vice versa.
We start to understand what the formula:
Translate simple formula is: If you have X, Y two variables, "the difference between the mean of X-value" Every time multiplied by the "poor Y value of its mean" get a product, then this product every time sum and obtains the mean (in fact seeking "expectations", but the idea is not a new concept too much, simply that is averaging a).
Here's an example to illustrate it:
For example there are two variables X, Y, observed t1-t7 (7 time) their changes.
FIG made a simple: are represented by red dot green dot, and X, Y, and the horizontal axis is time. You can see the X, Y are around their mean movement, and obviously with the change.
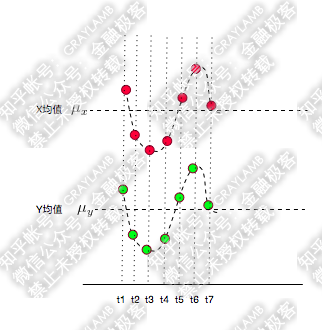
In this case, we found that each time the value of the
"sign" of the same constant value (as shown below: For example at time t1, with the same positive, t2 have the same time is negative):
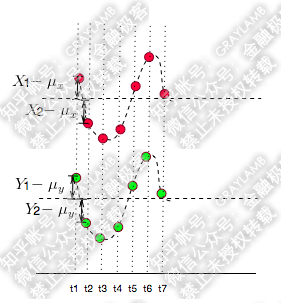
So, like on the map as when the same direction when they change, and
the product is positive. Thus, when you put the time t1-t7
with
the product are added together, i.e. the averaged number of n.
If the reverse movement of it?
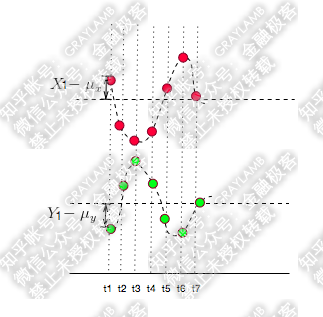
Obviously, the value of
the "sign" a certain value of the opposite, so
the
product is a negative value. So that when you put the time t1-t7
and
the product together, averaging time is a negative number.
Of course, the above said are two special cases, many times X, Y movements are irregular, such as:
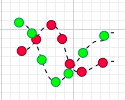
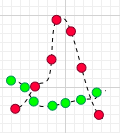
这时,很可能某一时刻的值与
的值乘积为正,另外一个时刻
的值与
的值乘积为负。
将每一时刻与
的乘积加在一起,其中的正负项就会抵消掉,最后求平均得出的值就是协方差,通过协方差的数值大小,就可以判断这两个变量同向或反向的程度了。
所以,t1-t7时刻中,与
的乘积为正的越多,说明同向变化的次数越多,也即同向程度越高。反之亦然。
总结一下,如果协方差为正,说明X,Y同向变化,协方差越大说明同向程度越高;如果协方差为负,说明X,Y反向运动,协方差越小说明反向程度越高。
--------LINE---------
一般的同学看到above the line的内容就ok了。但有一些爱钻研的同学,可能会进一步提问:
那如果X,Y同向变化,但X大于均值,Y小于均值,那与
的乘积为负值啊?这不是矛盾了吗?
那就继续往下看……
这种情况是有可能出现的,比如:
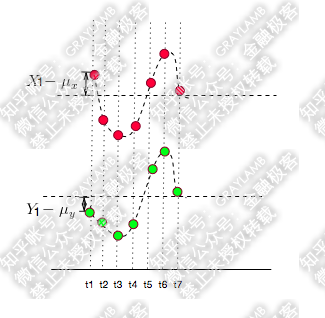
可以看到,t1时刻,与
的符号相反,他们的乘积为负值。
但是,总体看,这两个变量的协方差仍然是正的,因为你还要计算t2,t3……t7时刻与
的乘积,然后再把这7个时刻的乘积求和做均值,才是最后X,Y的协方差。1个负、6个正,显然最后协方差很大可能性是正的。
所以t1时刻与
的乘积为负值,并不能说明他们反向运动,要结合整体的情况来判断。
那么你可能又要问了,既然都是同向变化,那t1时刻与
的乘积为负值、其他时刻乘积为正的这种情况,与,t1-t7时刻
与
的乘积均为正值的情况,到底有什么差异呢?这点其实前面也解释过了,差异就是:第一种情况的同向程度不如第二种情况的同向程度大(第一种情况6正1负,第二种情况7正,所以第一种情况的协方差小于第二种情况的协方差,第一种情况X,Y变化的同向程度要小于第二种情况)。
另外,如果你还钻牛角尖,说如果t1,t2,t3……t7时刻X,Y都在增大,而且X都比均值大,Y都比均值小,这种情况协方差不就是负的了?7个负值求平均肯定是负值啊?但是X,Y都是增大的,都是同向变化的,这不就矛盾了?
这个更好解释了:这种情况不可能出现!
因为,你的均值算错了……
X,Y的值应该均匀的分布在均值两侧才对,不可能都比均值大,或都比均值小。
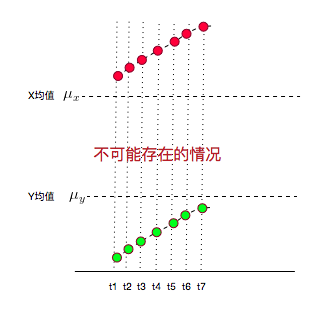
所以,实际它的图应该是下面这样的:
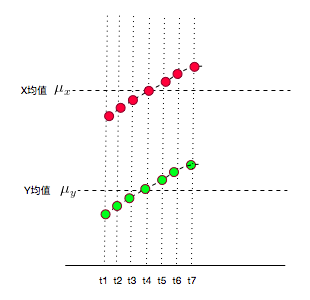
发现没有,又变成与
的符号相同的情况了~有没有种被大自然打败的感觉~
好了,现在,对于协方差应该有点感觉了吧?
二、相关系数:
对于相关系数,我们从它的公式入手。一般情况下,相关系数的公式为:
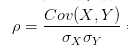
翻译一下:就是用X、Y的协方差除以X的标准差和Y的标准差。
所以,相关系数也可以看成协方差:一种剔除了两个变量量纲影响、标准化后的特殊协方差。
既然是一种特殊的协方差,那它:
1、也可以反映两个变量变化时是同向还是反向,如果同向变化就为正,反向变化就为负。
2、由于它是标准化后的协方差,因此更重要的特性来了:它消除了两个变量变化幅度的影响,而只是单纯反应两个变量每单位变化时的相似程度。
比较抽象,下面还是举个例子来说明:
首先,还是承接上文中的变量X、Y变化的示意图(X为红点,Y为绿点),来看两种情况:
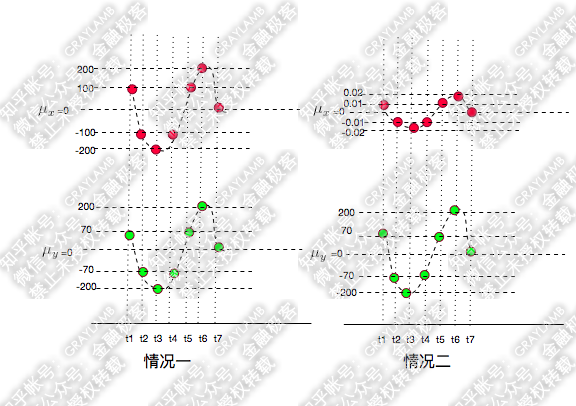
很容易就可以看出以上两种情况X,Y都是同向变化的,而这个“同向变化”,有个非常显着特征:X、Y同向变化的过程,具有极高的相似度!无论第一还是第二种情况下,都是:t1时刻X、Y都大于均值,t2时刻X、Y都变小且小于均值,t3时刻X、Y继续变小且小于均值,t4时刻X、Y变大但仍小于均值,t5时刻X、Y变大且大于均值……
可是,计算一下他们的协方差,
第一种情况下:
第二种情况下:
协方差差出了一万倍,只能从两个协方差都是正数判断出两种情况下X、Y都是同向变化,但是,一点也看不出两种情况下X、Y的变化都具有相似性这一特点。
这是为什么呢?
因为以上两种情况下,在X、Y两个变量同向变化时,X变化的幅度不同,这样,两种情况的协方差更多的被变量的变化幅度所影响了。
所以,为了能准确的研究两个变量在变化过程中的相似程度,我们就要把变化幅度对协方差的影响,从协方差中剔除掉。于是,相关系数就横空出世了,就有了最开始相关系数的公式:
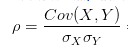
那么为什么要通过除以标准差的方式来剔除变化幅度的影响呢?咱们简单从标准差公式看一下:

从公式可以看出,标准差计算方法为,每一时刻变量值与变量均值之差再平方,求得一个数值,再将每一时刻这个数值相加后求平均,再开方。
“变量值与变量均值之差”是什么呢?就是偏离均值的幅度:
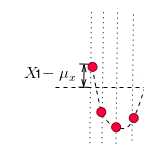
那为何要对它做平方呢?因为有时候变量值与均值是反向偏离的(见下图),是个负数,平方后,就可以把负号消除了。这样在后面求平均时,每一项数值才不会被正负抵消掉,最后求出的平均值才能更好的体现出每次变化偏离均值的情况。
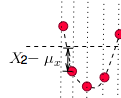
当然,最后求出平均值后并没有结束,因为刚才为了消除负号,把进行了平方,那最后肯定要把求出的均值开方,将这个偏离均值的幅度还原回原来的量级。于是就有了下面标准差的公式:

所以标准差描述了变量在整体变化过程中偏离均值的幅度。协方差除以标准差,也就是把协方差中变量变化幅度对协方差的影响剔除掉,这样协方差也就标准化了,它反应的就是两个变量每单位变化时的情况。这也就是相关系数的公式含义了。
同时,你可以反过来想象一下:既然相关系数是协方差除以标准差,那么,当X或Y的波动幅度变大的时候,它们的协方差会变大,标准差也会变大,这样相关系数的分子分母都变大,其实变大的趋势会被抵消掉,变小时也亦然。于是,很明显的,相关系数不像协方差一样可以在+到-
间变化,它只能在+1到-1之间变化(相关系数的取值范围在+1到-1之间变化可以通过施瓦茨不等式来证明,有些复杂,这里就不赘述了,有兴趣的可以google下)。
总结一下,对于两个变量X、Y,
当他们的相关系数为1时,说明两个变量变化时的正向相似度最大,即,你变大一倍,我也变大一倍;你变小一倍,我也变小一倍。也即是完全正相关(以X、Y为横纵坐标轴,可以画出一条斜率为正数的直线,所以X、Y是线性关系的)。
随着他们相关系数减小,两个变量变化时的相似度也变小,当相关系数为0时,两个变量的变化过程没有任何相似度,也即两个变量无关。
当相关系数继续变小,小于0时,两个变量开始出现反向的相似度,随着相关系数继续变小,反向相似度会逐渐变大。
当相关系数为-1时,说明两个变量变化的反向相似度最大,即,你变大一倍,我变小一倍;你变小一倍,我变大一倍。也即是完全负相关(以X、Y为横纵坐标轴,可以画出一条斜率为负数的直线,所以X、Y也是线性关系的)。
有了上面的背景,我们再回到最初的变量X、Y的例子中,可以先看一下第一种情况的相关系数:
X的标准差为
Y的标准差为
于是相关系数为
说明第一种情况下,X的变化与Y的变化具有很高的相似度,而且已经接近完全正相关了,X、Y几乎就是线性变化的。
那第二种情况呢?
X的标准差为
Y的标准差为
于是相关系数为
Description In the second case, although the magnitude of the change in X X than the first case of a small change in the magnitude of 10,000 times, but did not change the "change with changes in X-Y has a high degree of similarity" this conclusion. Meanwhile, since the first correlation coefficient, the second case are equal, so in both cases, the process of change X, Y has the same similarity.
Well, talk so much, I do not know whether you read the correlation coefficient has also been some feeling?
Third, written in the last
After this paper or would like to give non-science and engineering, entry-level look at my friends, and myself have been suffering in the vast sea of the formula, but later discovered the principle of a formula had a feeling that it will become easy to remember a lot but also like to delve into it. This article is to train you covariance, correlation coefficient of this feeling. However, for easy to understand, in some places not comprehensive, rigorous. Perhaps you read this article, learn through their own research, will have some of their own ideas, then you can continue to look at other answers A master under this title by introducing a vector, such as the definition of the inner product, will covariance, correlation a more rigorous explanation coefficient and thorough. In short learning is a gradual process, do not feel completely understand what it often is the first step you step into a field.
(Finish)