Random Variables
What is a random variable? I.e., a given sample space , the real-valued function which is
referred to as (real-valued) random variables.
Expect
The product of all possible values of the discrete random variable corresponding thereto is called a probability P and the mathematical expectation
variance
A random variable variance (Variance) is described in its degree of dispersion, i.e. the distance variable from its desired value
Covariance
In probability theory and statistics used to measure the overall error of two variables. The variance is the covariance a special case, i.e., when the two variables are the same situation.
The correlation coefficient
Measure of the random variable X and Y of relevance to a method, the correlation coefficient is in the range [-1,1]. The larger the absolute value of the correlation coefficient, the X and Y indicates a higher degree of correlation. When the X and Y linear, the correlation coefficient value of 1 (positive linear correlation) or negative (negative linear correlation).
Central Limit Theorem
Heart limit theorem, under appropriate conditions, a large number of independent random variables after suitably normalized mean converges in distribution to a normal distribution . This group theorem is the mathematical statistics theory foundation and error analysis, pointed out that the conditions for a large number of random variables and approximate normal distribution. And normally distributed.
Bayesian formula
P(h∣D)=P(h)P(D∣h)/P(D)
Bayes' theorem is a theorem of conditions for the random events A and B of probability. A where P is the possibility of occurring in the case of B occurs , the posterior probability x of the y, y converted prior probability and posterior probability with respect to x, and simply, the calculation of the conditional probability poor conversion good conditions for the calculation of probability
Full probability formula
The sample space is provided Experiment E S, A is the event E, B1, B2, ..., Bn is a partition of S, and P (Bi)> 0 (i = 1,2, ..., n) , then
P (A) = P (A | B1) * P (B1) + P (A | B2) * P (B2) + ... + P. (A | Bn) * P (Bn)
on the said formula for the whole probability formula
The effect of all probability formula that will solve the probability of complex events into a simple probability events that occur in different situations sum
Sample space
Definition: the set of all randomized trials of E E is referred to as sample space, denoted S = {e}, said element e S of the sample point, a single set of elements known as the base point event.
Law of large numbers
In the same test conditions, the test is repeated several times, the frequency of random events similar to its probability. Occasionally it contains some kind of inevitable.
Common sampling distribution
hypothetical test
In scene throughout the population distribution is unknown or known only in the form of, various parameters are unknown, only some tests of sample data, we put forward a hypothesis. Using a sample, a reasonable assumption is verified.
Priori probability
Things did not happen, but based on past statistics, analyze the possibility of what happened, that a priori probabilities.
Posterior probability
What has happened, there have been results, but for the possibility of causing factors of these things happen, there are fruit solicited by, that the posterior probability. The posterior probability, cause, is a measure of possible errors.
Calculate posterior probability, based on a priori probability preconditions. If you only knew what the results without knowing a priori probabilities (no previous statistics), is unable to calculate the posterior probability.
Calculate the posterior probability of the need to apply Bayes' formula
Confidence interval
Seeking to meet a range of probabilities. That can be understood as, in this context, to achieve a certain credibility, confidence intervals.
Principal component analysis
It is a statistical method. Orthogonal transform by the presence of a variable set of possible correlation variables into a set of linearly independent, the set of the converted variables called principal components. Widely used to go inside dimension reduction
Conditional probability, joint probability, marginal probability
Conditional probability is the probability of event A has occurred under the condition occurs in another event B. Expressed as a conditional probability P (A | B), is read as "the probability of B at condition A"
Joint probability represents the probability of two events occur together. The joint probability of A and B represents or
.
Marginal probability is the probability of an event occurring. Marginal probability is obtained in this way: In the joint probability, the end result of those events are not required to merge its total probability events disappear (discrete random variable with probability summation was full, continuous random variables have full integration probability). This is called marginalization (marginalization). A marginal probability is represented as P (A), B is represented by an edge probability P (B).
Maximum likelihood estimate
In the case of the known experimental results, to meet the parameters used to estimate the distribution of these samples, the most likely of the parameter [theta] [theta] as a real [theta] ^ parameter estimates θ ^ of. Said the popular point: maximum likelihood estimation is the use of a known sample results, thrust reversers are most likely (most probable) cause the value of this parameter results .
2> likelihood expression derivation pretreated if necessary, such as logarithmic (logistic regression needed), the derivative is allowed to 0, to obtain the likelihood function.
3> solution solving the likelihood function, parameter solution that is obtained by maximum likelihood estimation.
Discrete random variables


Continuous random variable
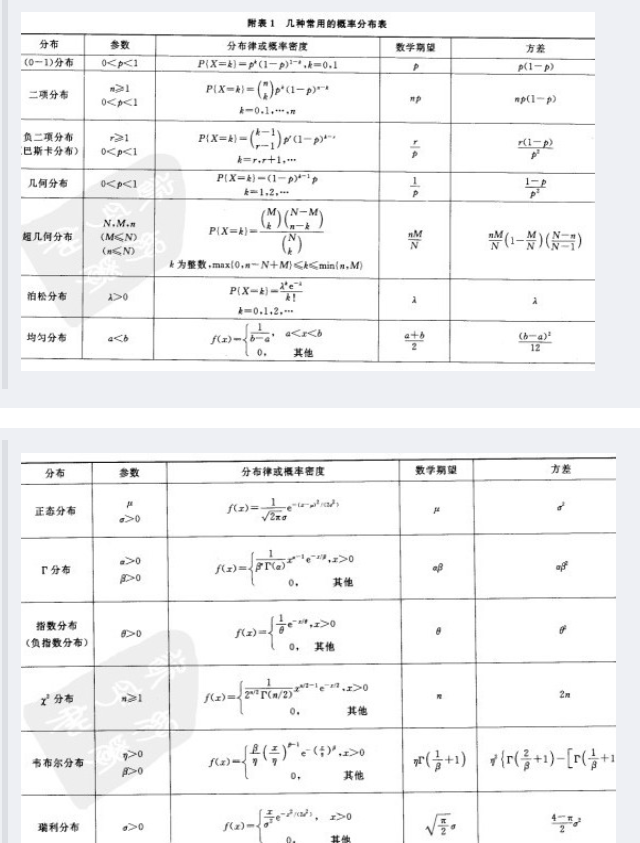