- GSVA Introduction of
Gene Set Variation Analysis, is called a gene variation analysis set, unsupervised analysis is a non-parametric method is mainly used to evaluate the results of gene sets enriched nuclear chip set. Mainly in the expression level between sample matrix by transforming into a gene set gene expression between different sample matrices, thereby assess whether the enriched different metabolic pathways between different samples. In fact, it is to study differential gene sets of interest between these different samples, or find the more important gene sets, as an analytical method, mainly in order from the perspective of bioinformatics to explain the cause phenotypic differences. Its main file input file and gene expression of matrix set, by the method can be the outcome of gsva; both chip processing result, the processing result may be transcriptome.
- GSVA installation
source("http://bioconductor.org/biocLite.R")
biocLite('GSVAdata')
biocLite('GSVA')
biocLite("limma")
biocLite("genefilter")
- GSVA operational considerations
- If the data chip is the need to filter the data, refer to the following test data codes, see the official documents or
- GSVA itself provides three algorithms, generally use the default algorithm on it
- For RNA-seq data if the read count is to be selected Possion distribution, if the expression values obtained after homogenization, can select the default parameters of the Gaussian distribution can be a
- Read the reference data format to the final format of the expression data, the need to produce their own information packet
- Using simulated data
#构造一个 30个样本,2万个基因的表达矩阵, 加上 100 个假定的基因集
library(GSVA)
p <- 20000 ## number of genes
n <- 30 ## number of samples
nGS <- 100 ## number of gene sets
min.sz <- 10 ## minimum gene set size
max.sz <- 100 ## maximum gene set size
X <- matrix(rnorm(p*n), nrow=p, dimnames=list(1:p, 1:n))
dim(X)
gs <- as.list(sample(min.sz:max.sz, size=nGS, replace=TRUE)) ## sample gene set sizes
gs <- lapply(gs, function(n, p) sample(1:p, size=n, replace=FALSE), p) ## sample gene sets
es.max <- gsva(X, gs, mx.diff=FALSE, verbose=FALSE, parallel.sz=1)
es.dif <- gsva(X, gs, mx.diff=TRUE, verbose=FALSE, parallel.sz=1)
pheatmap::pheatmap(es.max)
pheatmap::pheatmap(es.dif)
- GSVA use
requires the following package
library(GSEABase)
library(GSVAdata)
data(c2BroadSets)
c2BroadSets
library(Biobase)
library(genefilter)
library(limma)
library(RColorBrewer)
library(GSVA)
#官方文档有数据的预处理过程
cacheDir <- system.file("extdata", package="GSVA")
cachePrefix <- "cache4vignette_"
file.remove(paste(cacheDir, list.files(cacheDir, pattern=cachePrefix), sep="/"))
data(leukemia)
leukemia_eset
head(pData(leukemia_eset))
table(leukemia_eset$subtype)
data(leukemia)
leukemia_eset
filtered_eset <- nsFilter(leukemia_eset, require.entrez=TRUE, remove.dupEntrez=TRUE,var.func=IQR, var.filter=TRUE, var.cutoff=0.5, filterByQuantile=TRUE,feature.exclude="^AFFX")
leukemia_filtered_eset <- filtered_eset$eset
- Calculating the difference of gene sets GSVA
cache(leukemia_es <- gsva(leukemia_filtered_eset, c2BroadSets,min.sz=10, max.sz=500, verbose=TRUE),dir=cacheDir, prefix=cachePrefix)
adjPvalueCutoff <- 0.001
logFCcutoff <- log2(2)
design <- model.matrix(~ factor(leukemia_es$subtype))
colnames(design) <- c("ALL", "MLLvsALL")
fit <- lmFit(leukemia_es, design)
fit <- eBayes(fit)
allGeneSets <- topTable(fit, coef="MLLvsALL", number=Inf)
DEgeneSets <- topTable(fit, coef="MLLvsALL", number=Inf,
p.value=adjPvalueCutoff, adjust="BH")
res <- decideTests(fit, p.value=adjPvalueCutoff)
summary(res)
- Calculating the difference expressed genes limma
logFCcutoff <- log2(2)
design <- model.matrix(~ factor(leukemia_eset$subtype))
colnames(design) <- c("ALL", "MLLvsALL")
fit <- lmFit(leukemia_filtered_eset, design)
fit <- eBayes(fit)
allGenes <- topTable(fit, coef="MLLvsALL", number=Inf)
DEgenes <- topTable(fit, coef="MLLvsALL", number=Inf,
p.value=adjPvalueCutoff, adjust="BH", lfc=logFCcutoff)
res <- decideTests(fit, p.value=adjPvalueCutoff, lfc=logFCcutoff)
#summary(res)
- RNAseq data
of the original integer if the read count is RNAseq gsva in use need to set kcdf = "Possion", if RPKM log is taken over, the TPM and the like can use the default value of the result, so if we use for fpkm when analyzed using the default parameters can Bureau
data(commonPickrellHuang)
stopifnot(identical(featureNames(huangArrayRMAnoBatchCommon_eset),featureNames(pickrellCountsArgonneCQNcommon_eset)))
stopifnot(identical(sampleNames(huangArrayRMAnoBatchCommon_eset),sampleNames(pickrellCountsArgonneCQNcommon_eset)))
canonicalC2BroadSets <- c2BroadSets[c(grep("^KEGG", names(c2BroadSets)),grep("^REACTOME", names(c2BroadSets)),grep("^BIOCARTA", names(c2BroadSets)))]
data(genderGenesEntrez)
MSY <- GeneSet(msYgenesEntrez, geneIdType=EntrezIdentifier(),collectionType=BroadCollection(category="c2"), setName="MSY")
XiE <- GeneSet(XiEgenesEntrez, geneIdType=EntrezIdentifier(),collectionType=BroadCollection(category="c2"), setName="XiE")
canonicalC2BroadSets <- GeneSetCollection(c(canonicalC2BroadSets, MSY, XiE))
#使用GSVA方法进行计算
esmicro <- gsva(huangArrayRMAnoBatchCommon_eset, canonicalC2BroadSets, min.sz=5, max.sz=500,mx.diff=TRUE, verbose=FALSE, parallel.sz=1)
esrnaseq <- gsva(pickrellCountsArgonneCQNcommon_eset, canonicalC2BroadSets, min.sz=5, max.sz=500,kcdf="Poisson", mx.diff=TRUE, verbose=FALSE, parallel.sz=1)
- In fact, the document also compared GSVA and various other methods, here a little over
The actual expression profiling data test project
The expression of matrix format
gene AdA3_0h-1 AdA3_0h-2 AdA3_0h-3 AdA3_0h-4 AdA3_0h-5 AdA3_0h-6 AdA3_16h-3 AdA3_16h-4 AdA3_16h-5 AdA3_16h-6 AdGFP_0h-1 AdGFP_0h-2 AdGFP_0h-3 AdGFP_0h-4 AdGFP_0h-5 AdGFP_0h-6 AdGFP_16h-1 AdGFP_16h-2 AdGFP_16h-3 AdGFP_16h-4 AdGFP_16h-5 AdGFP_16h-6
Gnai3 73.842026 52.385326 73.034203 70.679092 67.094292 74.611313 74.853683 72.340401 73.230095 77.39888 70.019714 69.995277 72.172127 72.398514 74.176201 72.700516 60.29203 60.199333 58.043304 58.425671 61.812901 60.219734
Pbsn 0 0 0 0 0 0 0 0 0 0 0 0 0 0 0 0 0 0 0 0
Cdc45 3.653137 5.67695 3.560387 3.287101 4.034892 2.92406 11.427282 14.665288 11.368333 11.96515 4.289696 3.869604 4.055799 3.617629 4.800964 4.790279 20.065876 25.753405 19.732252 26.296944 21.906717 20.378906
Scml2 0.15765 0.022811 0.301977 0.056921 0.022823 0.104651 1.542295 0.370989 0.226674 0.426919 0.132548 0.028549 0.066578 0 0.069393 0.10476 0.981483 1.607109 0.84421 0.912878 1.281105 2.309552
Gene set format
G1/S-Specific Transcription NA Rrm2 Ccne1 E2f1 Fbxo5 Cdc6 Dhfr Cdc45 Pola1 Cdt1 Cdk1 Tyms
E2F mediated regulation of DNA replication NA Rrm2 Ccne1 E2f1 Fbxo5 Cdc6 Pola2 Dhfr Cdc45 Pola1 Cdt1 Cdk1 Ccnb1 Tyms
Formation of Fibrin Clot (Clotting Cascade) NA Fga F11 Fgb Proc Serping1 Thbd Tfpi F7 Serpine2 Fgg Serpind1 Serpina5 F8 Pf4
Resolution of D-loop Structures through Holliday Junction Intermediates NA Dna2 Bard1 Brca1 Rad51 Rad51c Brip1 Rad51b Exo1 Gen1 Wrn Blm Brca2 Eme1
Hemostasis NA Fga Serpinf2 Atp1b1 Sparc Slc16a3 Plaur Plat Gpc1 Tgfb1 F11 Fam49b Arrb2 Fgb Rapgef4 Proc Ahsg Wee1 Vav2 Serping1 Esam Kif2c Syk Thbd Kif11 Igf1 Tfpi Rad51c Serpinb2 Lgals3bp Dock11 Prkcg Timp3 Racgap1 Col1a2 Rad51b Pde5a Nos3 Dock8 F7 Serpine2 Kif4 Tek Ehd3 Col1a1 Cav1 Prkch Fgg Serpind1 Serpina5 Igf2 Cd74 Ctsw Irf1 Fcer1g Pde9a Plek Rapgef3 Lcp2 P2rx7 Pde10a Kif15 Kif23 Csf2rb2 Gnai1 Zfpm1 Slc7a8 Spp2 Kif5a Il2rg Lck Rac2 Hist2h3c1 Gng2 Pla2g4a Cyb5r1 F8 Gata3 Cd63 Pf4 Serpina3c Kif3c P2ry12 Slc16a8 F2rl3 Ppia Vav3 Ttn Kif18a Pde3a Csf2 Prkcb
Resolution of D-Loop Structures NA Dna2 Bard1 Brca1 Rad51 Rad51c Brip1 Rad51b Exo1 Gen1 Wrn Blm Brca2 Eme1
Common Pathway of Fibrin Clot Formation NA Fga Fgb Proc Thbd Serpine2 Fgg Serpind1 Serpina5 F8 Pf4
Kinesins NA Kif2c Kif11 Racgap1 Kif4 Kif15 Kif23 Kif5a Kif3c Kif18a
Xenobiotics NA Cyp2c50 Cyp2c37 Cyp2c54 Cyp2e1 Cyp2c29 Cyp2f2 Cyp2c38 Cyp2b9 Cyp2c55 Arnt2 Cyp2a4
Resolution of D-loop Structures through Synthesis-Dependent Strand Annealing (SDSA) NA Dna2 Bard1 Brca1 Rad51 Rad51c Brip1 Rad51b Exo1 Wrn Blm Brca2
Initial triggering of complement NA Crp C2 C4b C3 C1qc C1qa C1qb Cfp
Integrin cell surface interactions NA Fga Icam1 Itgb6 Spp1 Fgb Col5a2 Itga2 Tnc Col18a1 Col1a2 Itga9 Col1a1 Fgg Col7a1 Icam2 Fbn1 Itga8 Col8a1 Col4a4
CRMPs in Sema3A signaling NA Dpysl3 Dpysl2 Cdk5r1 Dpysl5 Plxna4 Sema3a Crmp1 Plxna3
Phase 1 - Functionalization of compounds NA Adh1 Cyp4a14 Cyp2c50 Cyp2c37 Cyp4a10 Maob Cyp2c54 Cyp2e1 Cyp4b1 Cyp2c29 Cyp39a1 Cyp2f2 Cyp3a25 Nr1h4 Marc1 Fmo2 Aoc2 Aldh1b1 Cyp2c38 Cmbl Cyp27b1 Adh7 Aoc3 Cyp2b9 Cyp2c55 Fdx1l Arnt2 Cyp2a4
Meiotic Recombination NA Brca1 Rad51 Hist1h2bc Hist1h2bg Blm Brca2 Hist2h2aa2 Hist2h2aa1 Hist1h2bh Hist1h2bj Hist1h2bl Hist1h2bp Hist1h2bk Hist1h2ba H2afv Hist3h2a Hist1h2bn Hist1h2bf Hist1h2bm Hist2h3c1 Hist1h2bb Prdm9 Msh4 Hist2h2ac Hist1h4h
Specific command is as follows
#读取基因集文件
geneSets <- getGmt("test.geneset")
#读取表达量文件并去除重复
mydata <- read.table(file = "all.genes.fpkm.xls",header=T)
name=mydata[,1]
index <- duplicated(mydata[,1])
fildup=mydata[!index,]
exp=fildup[,-1]
row.names(exp)=name
#将数据框转换成矩阵
mydata= as.matrix(exp)
#使用gsva方法进行分析,默认mx.diff=TRUE,min.sz=1,max.zs=Inf,这里是设置最小值和最大值
res_es <- gsva(mydata, geneSets, min.sz=10, max.sz=500, verbose=FALSE, parallel.sz=1)
pheatmap(res_es)
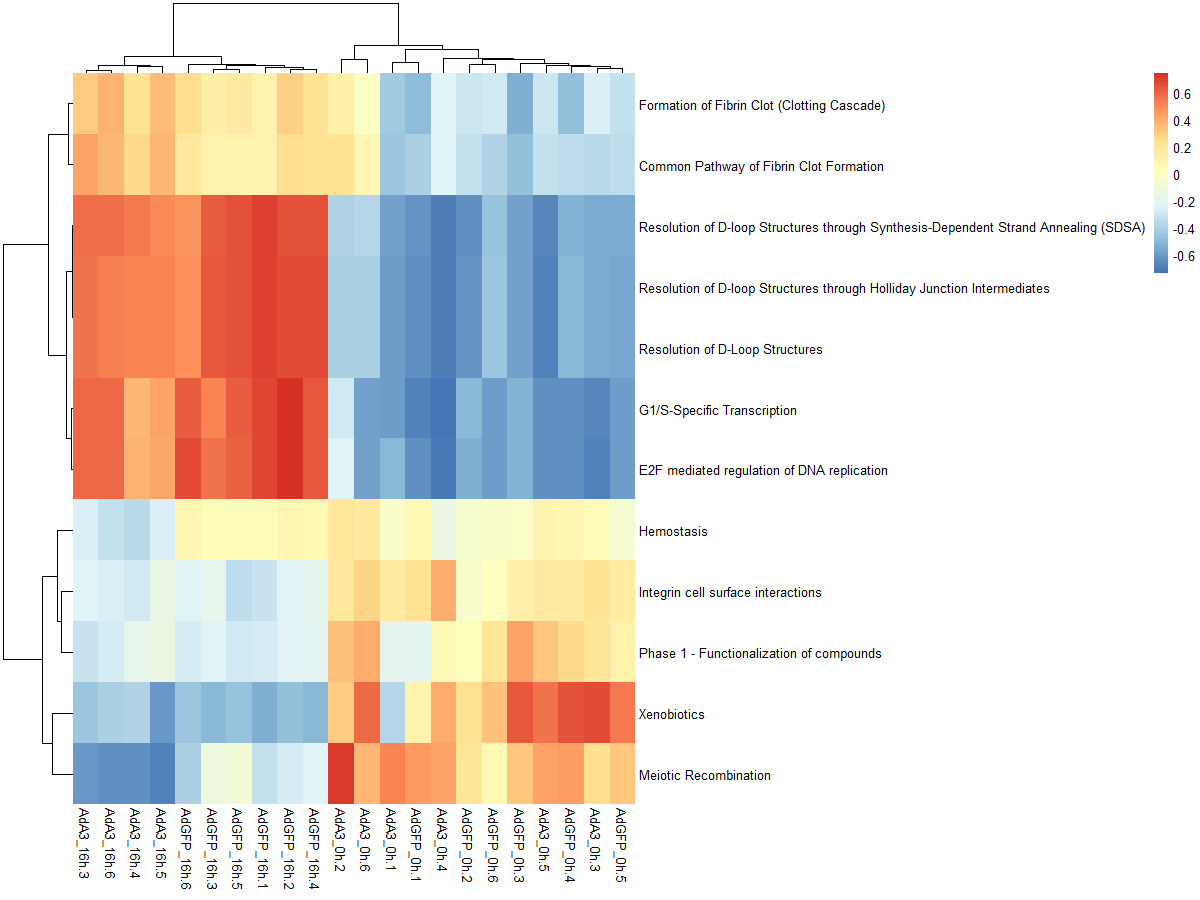
#mx.diff=FALSE es值是一个双峰的分布
es.max <- gsva(mydata, geneSets, mx.diff=FALSE, verbose=FALSE, parallel.sz=1)
pheatmap(es.max)
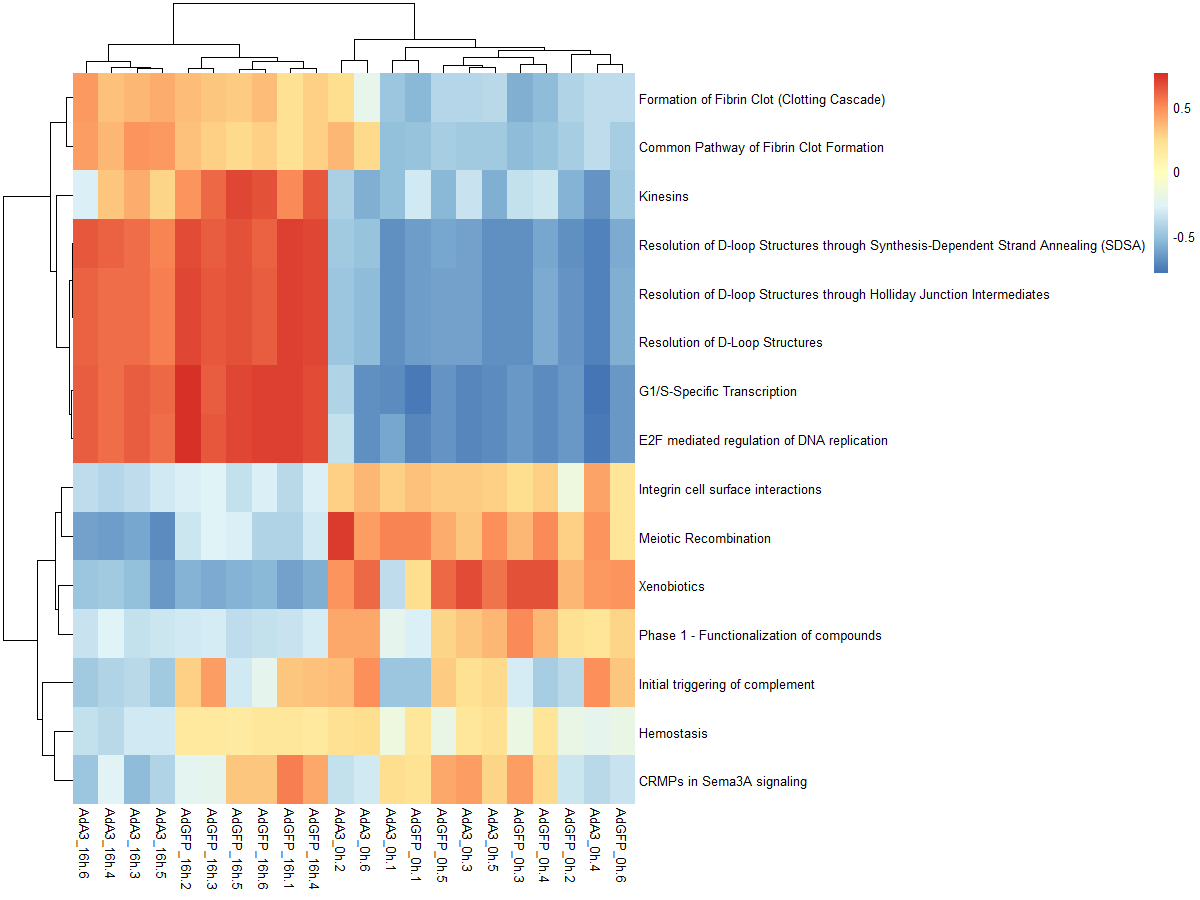
#mx.diff=TURE es值是一个近似正态分布
es.dif <- gsva(mydata, geneSets, mx.diff=TRUE, verbose=FALSE, parallel.sz=1)
pheatmap(es.dif)
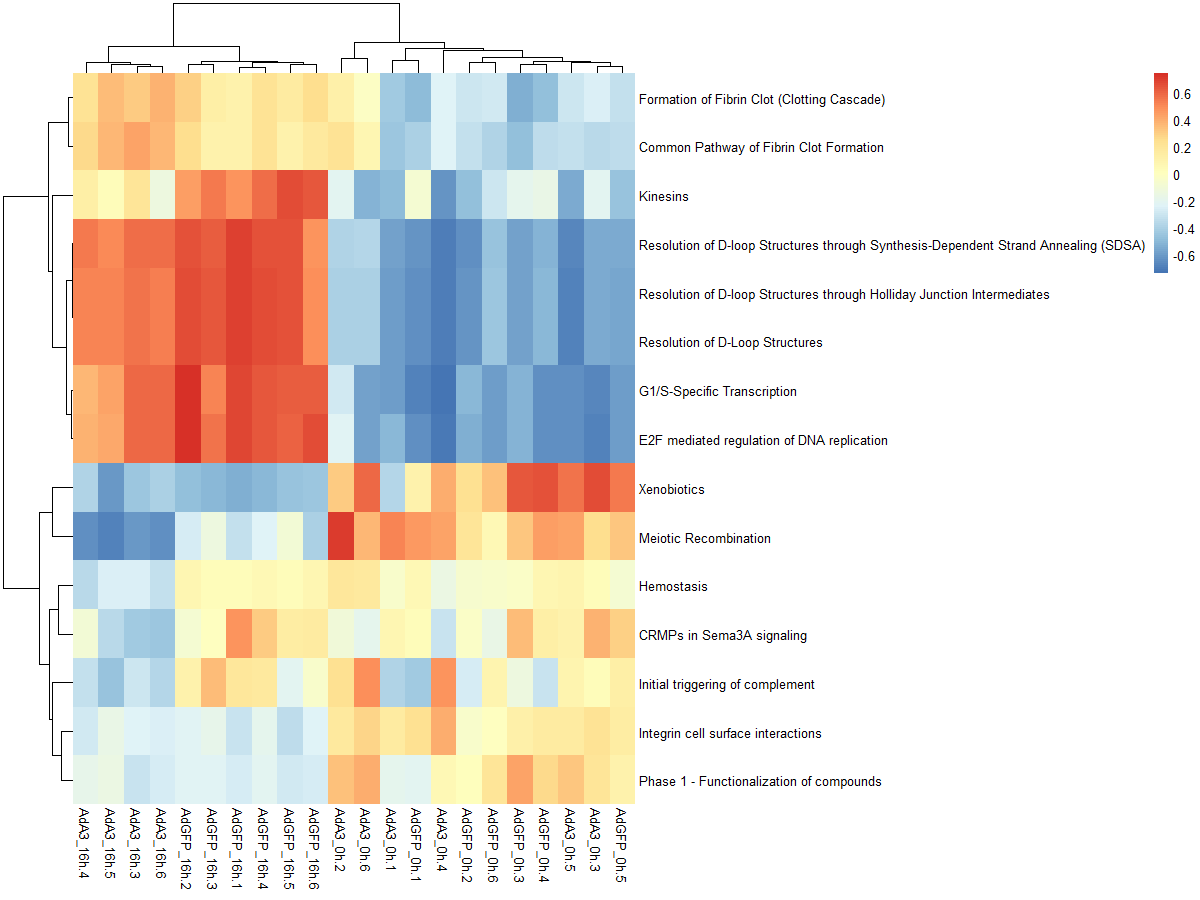
#可以看一下两种不同分布的效果,前者是高斯分布,后者是二项分布
par(mfrow=c(1,2), mar=c(4, 4, 4, 1))
plot(density(as.vector(es.max)), main="Maximum deviation from zero",xlab="GSVA score", lwd=2, las=1, xaxt="n", xlim=c(-0.75, 0.75), cex.axis=0.8)
axis(1, at=seq(-0.75, 0.75, by=0.25), labels=seq(-0.75, 0.75, by=0.25), cex.axis=0.8)
plot(density(as.vector(es.dif)), main="Difference between largest\npositive and negative deviations",xlab="GSVA score", lwd=2, las=1, xaxt="n", xlim=c(-0.75, 0.75), cex.axis=0.8)
axis(1, at=seq(-0.75, 0.75, by=0.25), labels=seq(-0.75, 0.75, by=0.25), cex.axis=0.8)
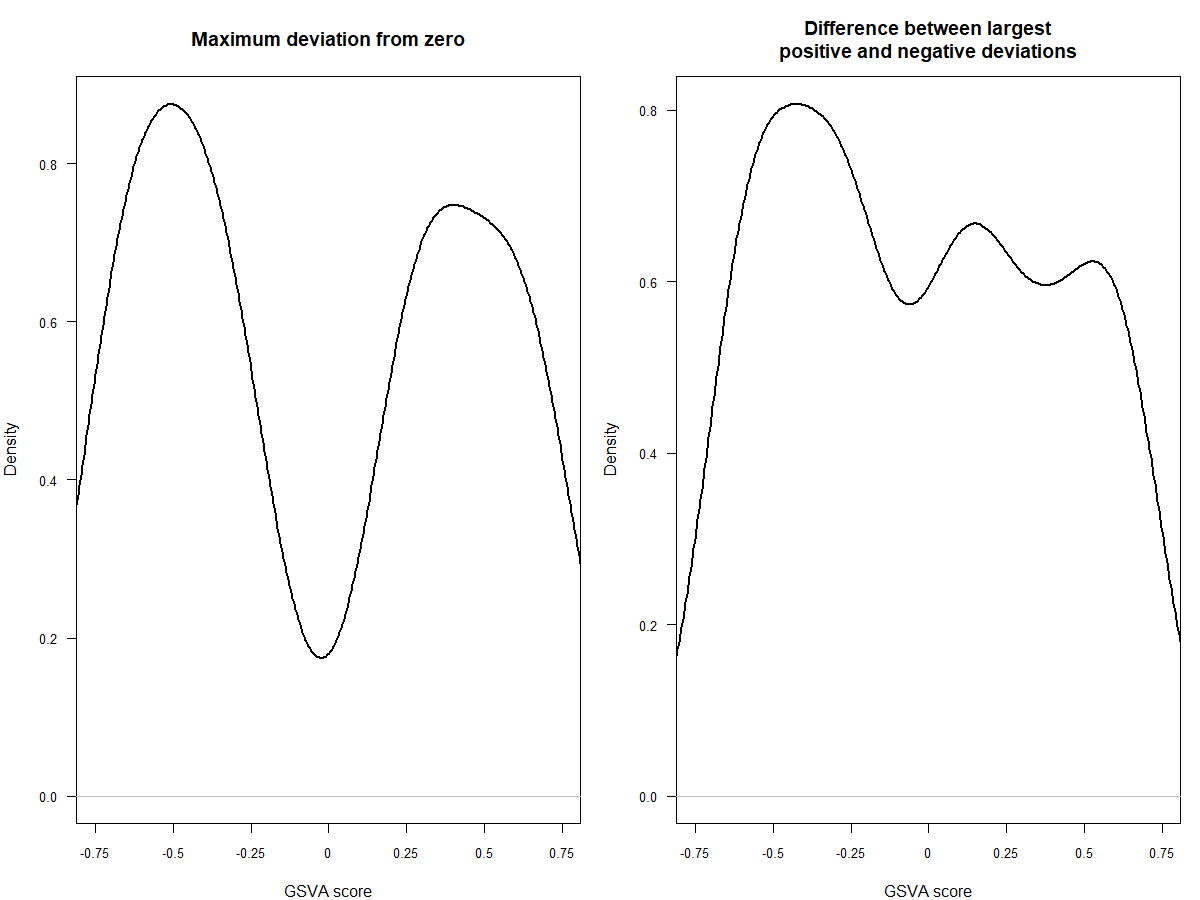
- The method of setting the packet limma
group_list <- factor(c(rep("control",2), rep("siSUZ12",2)))
design <- model.matrix(~group_list)
colnames(design) <- levels(group_list)
rownames(design) <- colnames(counts)
#设置分组
col = names(exp)
sample=col[1:10]
group=c(rep('control',6),rep('treat',4))
phno=data.frame(sample,group)
Group<-factor(phno$group,levels=levels(phno$group))
design<-model.matrix(~0+Group)
colnames(design) <- c("control", "treat")
#获取需要进行差异分析的分组
res=es.max[,1:10]
#定义阈值
logFCcutoff <- log2(1.5)
adjPvalueCutoff <- 0.001
#进行差异分析
fit <- lmFit(res, design)
fit <- eBayes(fit)
allGeneSets <- topTable(fit, coef="treat", number=Inf)
DEgeneSets <- topTable(fit, coef="treat", number=Inf,p.value=adjPvalueCutoff, adjust="BH")
res <- decideTests(fit, p.value=adjPvalueCutoff)
#summary(res)
#画火山图
DEgeneSets$significant="no"
DEgeneSets$significant=ifelse(DEgeneSets$logFC>0|DEgeneSets$logFC<0,"up","down")
ggplot(DEgeneSets,aes(logFC,-1*log10(adj.P.Val)))+geom_point(aes(color =significant)) + xlim(-4,4) + ylim(0,30)+labs(title="Volcanoplot",x="log[2](FC)", y="-log[10](FDR)")+scale_color_manual(values =c("#00ba38","#619cff","#f8766d"))+geom_hline(yintercept=1.3,linetype=4)+geom_vline(xintercept=c(-1,1),linetype=4)
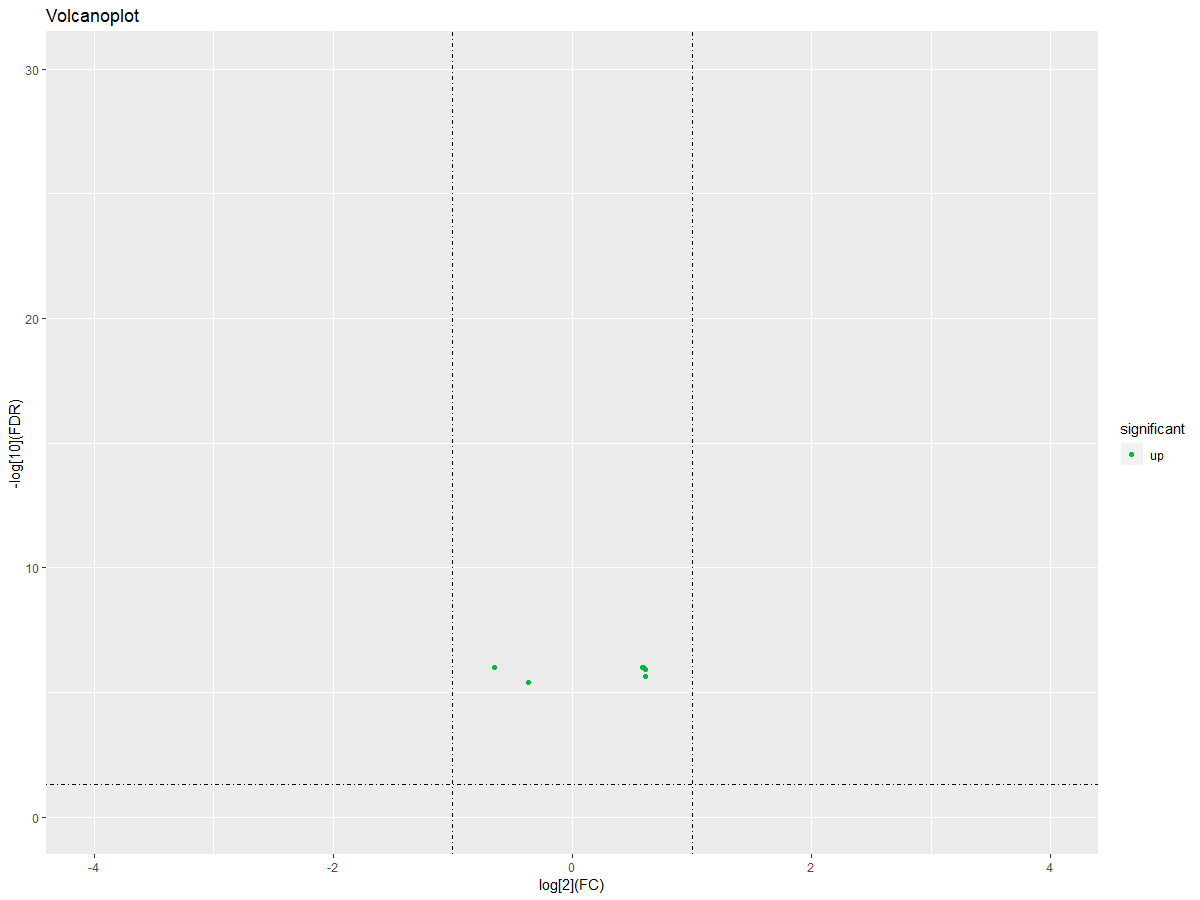
#获取差异基因集的表达量
DEgeneSetspkm = merge(DEgeneSets,es.max,by=0,all.x=TRUE)[,c(1,11:20)]
degsetsp=DEgeneSetspkm[,-1]
name=DEgeneSetspkm[,1]
row.names(degsetsp)=name
pheatmap(degsetsp)
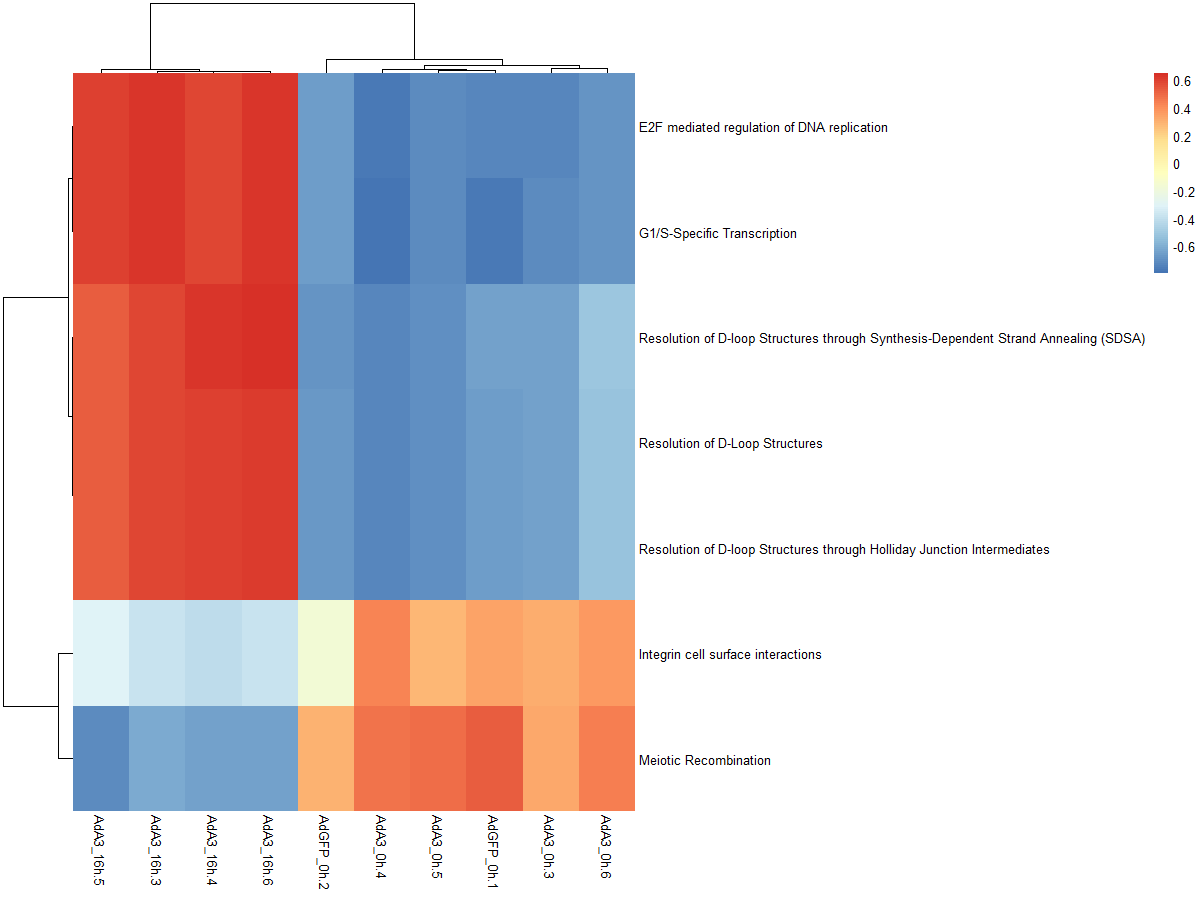