GAN was published in 2014, until recently all the AI generation of the fire has traces of GAN, come and implement it easily! ! !
Using GANs through an innovative combination of computational graphs and game theory, they show that, given sufficient modeling power, two competing models will be able to be jointly trained via plain old backpropagation.
These models play two different (literally adversarial ) roles. Given some real dataset R , G is the generator , trying to create fake data that looks like real data, and D is the discriminator , taking data from the real set or G and labeling the differences. G is like a counterfeit machine. After many times of drawing practice, the drawing is like a real picture. And D is the team of detectives trying to tell the difference. (Except in this case, forger G never sees the raw data - only D' s judgment. They're like blind men exploring forgers .
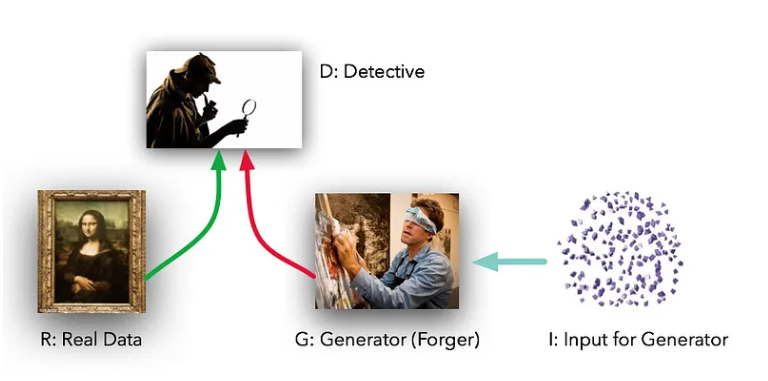
GAN implementation code
#!/usr/bin/env python
import numpy as np
import torch
import torch.nn as nn
import torch.optim as optim
from torch.autograd import Variable
matplotlib_is_available = True
try:
from matplotlib import pyplot as plt
except ImportError:
print("Will skip plotting; matplotlib is not available.")
matplotlib_is_available = False
# Data params
data_mean = 4
data_stddev = 1.25
# ### Uncomment only one of these to define what data is actually sent to the Discriminator
#(name, preprocess, d_input_func) = ("Raw data", lambda data: data, lambda x: x)
#(name, preprocess, d_input_func) = ("Data and variances", lambda data: decorate_with_diffs(data, 2.0), lambda x: x * 2)
#(name, preprocess, d_input_func) = ("Data and diffs", lambda data: decorate_with_diffs(data, 1.0), lambda x: x * 2)
(name, preprocess, d_input_func) = ("Only 4 moments", lambda data: get_moments(data), lambda x: 4)
print("Using data [%s]" % (name))
# ##### DATA: Target data and generator input data
def get_distribution_sampler(mu, sigma):
return lambda n: torch.Tensor(np.random.normal(mu, sigma, (1, n))) # Gaussian
def get_generator_input_sampler():
return lambda m, n: torch.rand(m, n) # Uniform-dist data into generator, _NOT_ Gaussian
# ##### MODELS: Generator model and discriminator model
class Generator(nn.Module):
def __init__(self, input_size, hidden_size, output_size, f):
super(Generator, self).__init__()
self.map1 = nn.Linear(input_size, hidden_size)
self.map2 = nn.Linear(hidden_size, hidden_size)
self.map3 = nn.Linear(hidden_size, output_size)
self.f = f
def forward(self, x):
x = self.map1(x)
x = self.f(x)
x = self.map2(x)
x = self.f(x)
x = self.map3(x)
return x
class Discriminator(nn.Module):
def __init__(self, input_size, hidden_size, output_size, f):
super(Discriminator, self).__init__()
self.map1 = nn.Linear(input_size, hidden_size)
self.map2 = nn.Linear(hidden_size, hidden_size)
self.map3 = nn.Linear(hidden_size, output_size)
self.f = f
def forward(self, x):
x = self.f(self.map1(x))
x = self.f(self.map2(x))
return self.f(self.map3(x))
def extract(v):
return v.data.storage().tolist()
def stats(d):
return [np.mean(d), np.std(d)]
def get_moments(d):
# Return the first 4 moments of the data provided
mean = torch.mean(d)
diffs = d - mean
var = torch.mean(torch.pow(diffs, 2.0))
std = torch.pow(var, 0.5)
zscores = diffs / std
skews = torch.mean(torch.pow(zscores, 3.0))
kurtoses = torch.mean(torch.pow(zscores, 4.0)) - 3.0 # excess kurtosis, should be 0 for Gaussian
final = torch.cat((mean.reshape(1,), std.reshape(1,), skews.reshape(1,), kurtoses.reshape(1,)))
return final
def decorate_with_diffs(data, exponent, remove_raw_data=False):
mean = torch.mean(data.data, 1, keepdim=True)
mean_broadcast = torch.mul(torch.ones(data.size()), mean.tolist()[0][0])
diffs = torch.pow(data - Variable(mean_broadcast), exponent)
if remove_raw_data:
return torch.cat([diffs], 1)
else:
return torch.cat([data, diffs], 1)
def train():
# Model parameters
g_input_size = 1 # Random noise dimension coming into generator, per output vector
g_hidden_size = 5 # Generator complexity
g_output_size = 1 # Size of generated output vector
d_input_size = 500 # Minibatch size - cardinality of distributions
d_hidden_size = 10 # Discriminator complexity
d_output_size = 1 # Single dimension for 'real' vs. 'fake' classification
minibatch_size = d_input_size
d_learning_rate = 1e-3
g_learning_rate = 1e-3
sgd_momentum = 0.9
num_epochs = 5000
print_interval = 100
d_steps = 20
g_steps = 20
dfe, dre, ge = 0, 0, 0
d_real_data, d_fake_data, g_fake_data = None, None, None
discriminator_activation_function = torch.sigmoid
generator_activation_function = torch.tanh
d_sampler = get_distribution_sampler(data_mean, data_stddev)
gi_sampler = get_generator_input_sampler()
G = Generator(input_size=g_input_size,
hidden_size=g_hidden_size,
output_size=g_output_size,
f=generator_activation_function)
D = Discriminator(input_size=d_input_func(d_input_size),
hidden_size=d_hidden_size,
output_size=d_output_size,
f=discriminator_activation_function)
criterion = nn.BCELoss() # Binary cross entropy: http://pytorch.org/docs/nn.html#bceloss
d_optimizer = optim.SGD(D.parameters(), lr=d_learning_rate, momentum=sgd_momentum)
g_optimizer = optim.SGD(G.parameters(), lr=g_learning_rate, momentum=sgd_momentum)
for epoch in range(num_epochs):
for d_index in range(d_steps):
# 1. Train D on real+fake
D.zero_grad()
# 1A: Train D on real
d_real_data = Variable(d_sampler(d_input_size))
d_real_decision = D(preprocess(d_real_data))
d_real_error = criterion(d_real_decision, Variable(torch.ones([1]))) # ones = true
d_real_error.backward() # compute/store gradients, but don't change params
# 1B: Train D on fake
d_gen_input = Variable(gi_sampler(minibatch_size, g_input_size))
d_fake_data = G(d_gen_input).detach() # detach to avoid training G on these labels
d_fake_decision = D(preprocess(d_fake_data.t()))
d_fake_error = criterion(d_fake_decision, Variable(torch.zeros([1]))) # zeros = fake
d_fake_error.backward()
d_optimizer.step() # Only optimizes D's parameters; changes based on stored gradients from backward()
dre, dfe = extract(d_real_error)[0], extract(d_fake_error)[0]
for g_index in range(g_steps):
# 2. Train G on D's response (but DO NOT train D on these labels)
G.zero_grad()
gen_input = Variable(gi_sampler(minibatch_size, g_input_size))
g_fake_data = G(gen_input)
dg_fake_decision = D(preprocess(g_fake_data.t()))
g_error = criterion(dg_fake_decision, Variable(torch.ones([1]))) # Train G to pretend it's genuine
g_error.backward()
g_optimizer.step() # Only optimizes G's parameters
ge = extract(g_error)[0]
if epoch % print_interval == 0:
print("Epoch %s: D (%s real_err, %s fake_err) G (%s err); Real Dist (%s), Fake Dist (%s) " %
(epoch, dre, dfe, ge, stats(extract(d_real_data)), stats(extract(d_fake_data))))
if matplotlib_is_available:
print("Plotting the generated distribution...")
values = extract(g_fake_data)
print(" Values: %s" % (str(values)))
plt.hist(values, bins=50)
plt.xlabel('Value')
plt.ylabel('Count')
plt.title('Histogram of Generated Distribution')
plt.grid(True)
plt.show()
train()
code output result
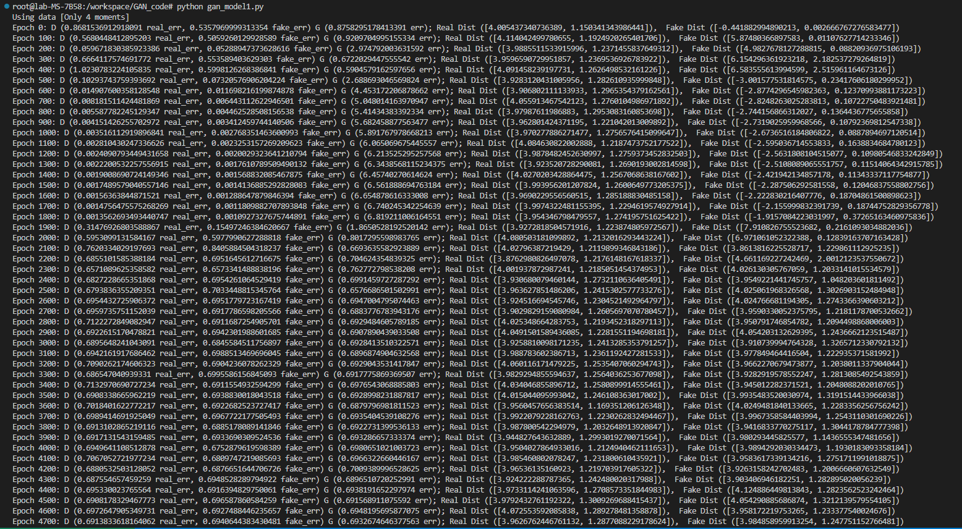
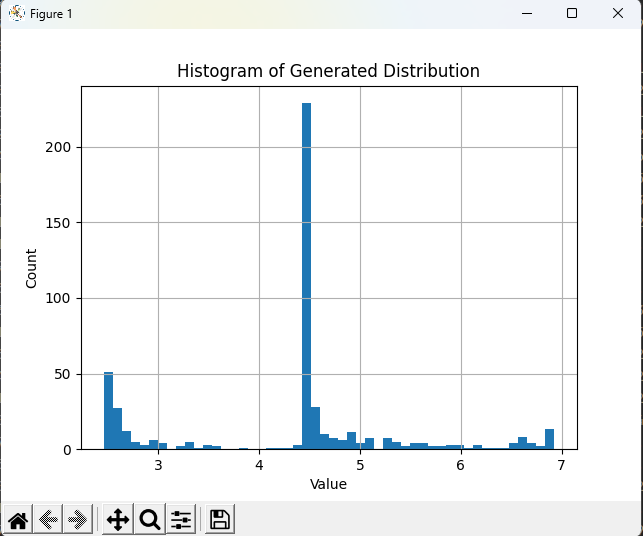
Personal summary
GAN from the perspective of programming (pure personal understanding, wrong can be corrected)
Use numpy's random method to randomly generate multidimensional noise vectors
Create a G network to generate
Create a D network to judge
The two networks are optimized separately during training
First train the D network to judge the true and false: if the training D is true, propagate; if the training D is false, propagate and put into the optimizer (1 is true, 0 is false)
Train G on top of D.
*Because it is randomly generated, the result of each generation is different