Let's first look at two customer cases where narrowband HD image quality enhancement technology improves the image quality of source video: the image quality enhancement of NBA live transcoding on BesTV APP and the image quality enhancement of Jiangsu Mobile's FIFA2022 World Cup live transcoding.
The right side is narrow-band high-definition image quality enhancement output
The video is limited to demonstrate the effect of the technical solution
https://v.youku.com/v_show/id_XNTk1MDY4NjUzMg==.html
The right side is narrow-band high-definition image quality enhancement output
The video is limited to demonstrate the effect of the technical solution
01 High-definition video has become a major trend
Video is the main carrier of information presentation and dissemination. From the early 625-line analog TV signal to the later VCD, DVD, Blu-ray, super-large TV, etc., users' endless pursuit of high-quality images has promoted the continuous progress of video technology and the vigorous development of the industry. It is predicted that in the future, more than 80% of individual consumer network traffic and more than 70% of industry application traffic will be video data.
At present, with the continuous upgrading of software/hardware configuration and performance of video shooting and video playback display devices, consumers have higher and higher requirements for video quality: from 360p to 720p to 1080p, and now it is fully jumping to 4K, and 8K is approaching. In video entertainment scenarios, video quality is a key factor affecting user interaction experience. High-definition videos often contain more details and information than low-definition videos, bringing users a better experience in video interaction, which also promotes user interaction. In video entertainment, the requirements for video quality are getting higher and higher. Once video consumers adapt to the different feelings and experiences brought by high-definition video, for example: high-definition video can restore details such as light, texture, character skin, and texture more realistically. will become lower and lower.
As the vanguard of innovation, Internet video sites are taking various countermeasures to meet the needs of consumers, and improving image quality has become a new battlefield for video sites besides fighting for IP. At present, 1080p has been fully popularized on domestic and foreign mainstream video websites/APPs, and 1080p has become a standard configuration; some video platforms, such as Ayouteng, Station B, and YouTube, also provide 4K versions of some program content.
02 Narrowband high-definition cloud transcoding helps optimize image quality in the "last mile"
Videos go through complex video processing and transmission links from collection to final distribution to end consumers for playback and viewing. A complete processing and transmission chain usually includes the following links:
l Acquisition/encoding : the video collected by the content provider will first be encoded into a specific format;
l Editing/editing/recoding : Perform various editing/editing operations on the original material, perform secondary creation, and then re-encode the output; some business scenarios may include multiple editing processes; the edited/encoded video will be Upload to the server;
l Cloud server transcoding : After the video is uploaded to the cloud server, it is usually transcoded on the cloud in order to adapt to different network environments and playback terminals (the narrowband high-definition transcoding discussed in this article occurs in this link, with a higher compression ratio to render higher quality video);
l Cloud publishing : CDN content distribution network
l Playback end : The video is accelerated to be distributed through the content distribution network (CDN), and finally played on the terminal device of the content consumer through decoding.
l Multi-platform playback : mobile phone, Pad, OTT, IPTV, Web
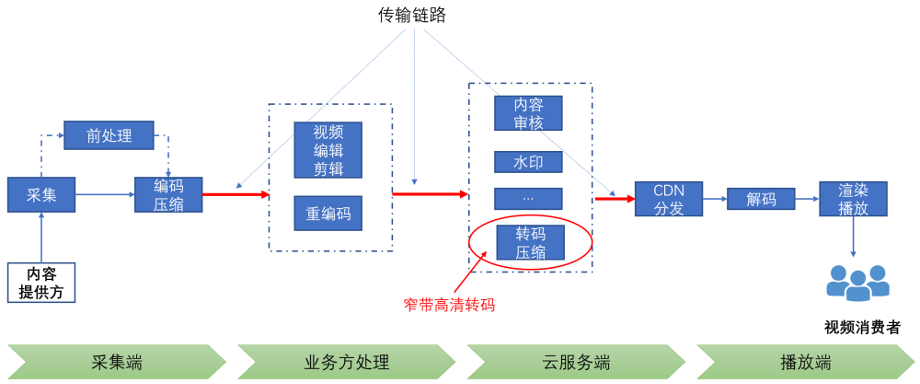
Figure 1 Video processing and transmission link
From the perspective of video processing, narrowband high-definition cloud transcoding is the last processing link for video content to reach end consumers; objectively speaking, it is the "last mile" of the entire link of video content production and consumption.
From the perspective of transmission, in the whole link of video production and consumption, there are many forms of data transfer between various links: SDI wired and cable methods, wireless cellular mobile communication, Internet and satellite communication, etc. Different data transmission schemes have huge differences in transmission environment stability and bandwidth. Therefore, in order to perform stable video transmission on bandwidth-limited links, it is necessary to perform deep coding and compression on video signals, and coding and compression will inevitably bring to varying degrees of image quality damage.
For example: Common video stream specifications: 1080p, 60 fps, YUV 4:2:0, 8-bit, raw data bit rate 1920*1080*1.5*8*60 = 1.49Gbps
Among the transmission methods mentioned above, only the 3G-SDI cable can support the real-time transmission of the stream. The way video content reaches end consumers is generally distributed via the Internet, and the bit rate needs to be controlled below 10Mbps, which means that the original video must be compressed hundreds of times.
To sum up, from the perspective of the entire video processing and transmission link, video content has to go through multiple video editing, processing, and re-encoding operations from acquisition to terminal playback. And every processing/encoding operation will more or less affect the image quality of the video, and usually damage the image quality. Therefore, even if the latest video capture equipment (which can output high-quality original video signals) is used at present, the end consumer side may not be guaranteed to experience high-quality images, because the image quality loss in the intermediate processing link.
Narrowband high-definition cloud transcoding is the last processing link of the entire video processing chain, and the image quality effect of the output code stream is the image quality effect that is finally distributed to end consumers. Therefore, if the appropriate image quality enhancement technology is used in this link, the image quality damage caused by the previous video processing link can be compensated to a certain extent, and the image quality can be optimized.
03 What problems should be prioritized for narrow-band HD image enhancement?
Video quality enhancement technologies can be roughly divided into three categories:
l Color/brightness/contrast dimension enhancement : color enhancement (color gamut, bit depth, HDR high dynamic range), defogging, low light/dark light enhancement, etc.;
l Time-domain frame rate enhancement : video frame rate conversion/intelligent frame insertion;
l Spatial dimension detail restoration/enhancement : anti-compression distortion, resolution doubling, noise reduction/scratch removal/bright spot removal, flicker removal, blur removal, shake removal, etc.
At the product implementation level, video enhancement technology is currently a popular choice to convert old video materials into high-definition, such as older movies, TV series, cartoons, and MV/concert videos. Old video footage is common: scratches, noise/scratches, flickering, blurred details, motion smearing, dim colors or only black and white, etc. De-flickering, resolution/frame rate doubling, and color enhancement (black and white coloring), etc., can comprehensively improve the overall look and feel of the material.
However, since the image quality problems faced by each old material are very different, and the current technical level is still difficult to provide satisfactory results for some image quality problems, the process of high-definition processing of old materials must introduce artificial intervene.
Manual intervention is reflected in two aspects: one is to diagnose the image quality problems of old materials, and configure appropriate processing models and processing procedures; the other is to manually review the model processing results, and make appropriate refinements and fine-tuning.
Narrowband high-definition image quality enhancement technology landing selection principles
Narrowband high-definition cloud transcoding is a fully automatic video transcoding operation without manual intervention, and the video quality enhancement and optimization technology used also needs to be fully automatic without manual intervention. We believe that when choosing a productization direction, the integrated video enhancement technology should meet the following conditions:
l Video enhancement technology can be fully automatic without manual intervention: the high-definition of old materials currently requires too much manual intervention, which does not meet this principle;
l Related technologies have a wide range of applications: low light/dark light enhancement and video deshaking are also required in some scenarios, but in video transcoding scenarios, the proportion of videos with such quality problems is very small;
l Sustained rigid demand: This technology can bring consumers a perceivable improvement in image quality, and the problems it solves will continue to exist in the next 5-10 years, so it can form a continuous rigid demand.
Narrowband HD image quality enhancement: solve the image quality loss introduced by the production link
According to the above principles, the image quality enhancement technology we finally chose to integrate in narrowband HD transcoding is: spatial dimension detail repair, which solves the image quality loss caused by the video production link, that is, the image quality loss caused by multiple encoding and compression.
From the perspective of the entire video processing and transmission link, let's analyze in detail the links that cause image quality loss:
1. The picture quality of the signal source itself
l Low bit rate caused by the transmission link: In the video production process, the bandwidth of the transmission link is usually limited to a certain extent. In order to give priority to ensuring smoothness, a low bit rate has to be adopted. Typical scenarios include: cross-border live streaming; long-distance transmission of on-site signals for large-scale events without dedicated line guarantee; and real-time signals from drone aerial photography. A typical bit rate setting is 1080p 50fps 4-6M. The live broadcast scene is usually hardware encoded, and the output bit stream has obvious encoding compression loss;
l Low bit rate caused by content copyright/business model: Due to video copyright or business model issues, video copyright owners only provide low bit rate signal sources to distribution channels;
l The original video material has been encoded and compressed many times, and there is already an obvious loss of image quality.
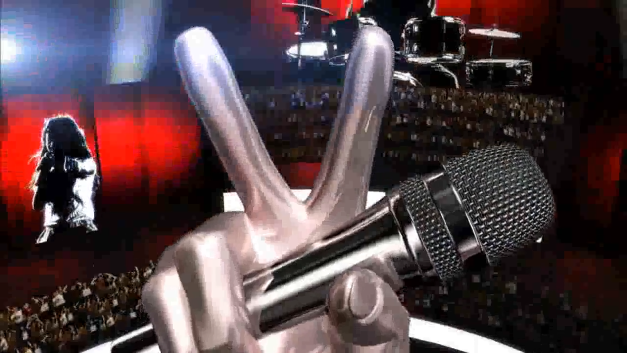
Figure 2 Image quality problem of low bit rate signal source: obvious coding block effect
2. Image quality problems introduced by editing/editing and secondary creation
l Image quality problems caused by encoding and compression of editing software.
In the field of UGC short videos, people are usually accustomed to using the mobile phone editing APP to edit videos. The editing APP will call the hardware encoding of the mobile phone to complete the encoded output of the rendered video; The compression performance varies greatly, so it is easy to cause poor picture quality after encoding and compression, even if the output bit rate is as high as 20M @1080p , as shown in the figure below;
l Image quality problems caused by re-encoding and compression of streaming tools.
In some business scenarios, such as an Internet celebrity blogger watching a game with you, the studio or commentary anchor will pull the original signal stream to the local through OBS, superimpose the commentary, and then push the stream to the cloud; OBS recoding will damage the original video again image quality.
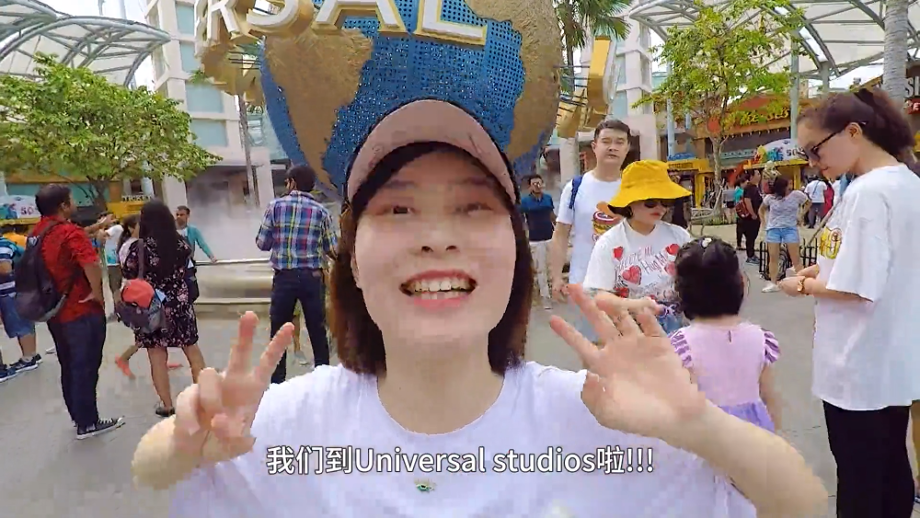
Figure 3 UGC short video, editing software output video:
The code rate is 20M, the resolution is 1920x1080, and the picture has obvious coding block effect and blur
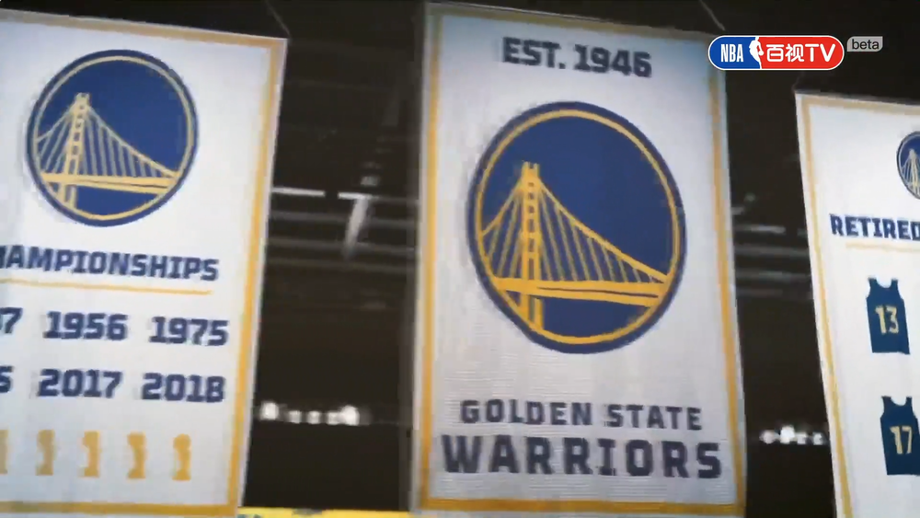
Figure 4 The anchor commentary, OBS streaming:
The code rate is 6M, the resolution is 1920x1080, and the picture has edge aliasing/burrs and blurring caused by a lot of encoding compression
From the perspective of demand duration, due to the limitation of transmission bandwidth, video coding and compression is an unavoidable processing operation in the entire video generation process, and compression will inevitably introduce image quality damage. Quality improvement will be a continuous demand.
04 Image quality enhancement technology for coding compression loss
From an academic point of view, to solve the image quality loss introduced by the production link, the main research technologies include: decompression distortion and super-resolution reconstruction. Decompression distortion mainly solves block effects caused by encoding compression, such as edge burrs and detail loss/blurring; super-resolution reconstruction can eliminate spatial resolution downsampling that may be introduced in the processing chain, and improve the overall sharpness and clarity of the picture .
The academic research on image super-resolution reconstruction technology has been going on for decades. Most of the early methods were based on spatial/temporal reconstruction techniques, and later developed into example-based learning methods. The more representative solutions are: (1) methods based on image self-similarity; (2) methods based on domain embedding ; (3) methods based on dictionary learning/sparse representation; (4) based on random forests, etc. However, it was not until the rise of super-resolution technology based on convolutional neural network (CNN) that the technology reached a commercially available level in terms of processing effects and performance, thus gaining widespread attention and application in the industry.
The first attempt to commercialize CNN-based image/video super-resolution technology was a start-up company called Magic Pony. The company made a very cool demo at CVPR 2016 - Real-Time Image and Video Super-Resolution on Mobile, Desktop and in the Browser[1, 3]. For the first time, CNN-based video super-resolution technology has been transplanted to mobile platforms (Samsung mobile phones and iPads), which can perform real-time super-resolution enhancement processing on live game screens, significantly improving the quality of source streams. The technology quickly caught the attention of Twitter and the company was acquired within a short period of time [2].
Later, with the holding of the first NTIRE super-resolution competition - NTIRE 2017 Challenge on Single Image Super-Resolution [4], more and more companies began to pay attention to CNN-based image super-resolution technology. Since then, this Landing applications in various aspects have also sprung up like mushrooms after rain.
l Conventional CNN decompression and distortion processing: this face is a bit fake
Although CNN-based image super-resolution technology can achieve far better processing results than previous technologies, there are still many problems in its productization process. A typical problem is: based on the MSE/SSIM loss function training to obtain the CNN super-resolution model (that is, the conventional CNN super-resolution model), the reconstructed image often lacks high-frequency detail information, so it appears too smooth and the subjective experience is not good. good.
The following three examples are the processing effect achieved by a typical conventional CNN super-resolution model:
The conventional CNN super-resolution model has a better smoothing effect on artifacts such as block effects, edge aliasing, and burrs caused by encoding compression, so that the entire picture looks cleaner, but the picture lacks details and texture, mainly reflected in the face area. There is a more obvious microdermabrasion effect. Therefore, in business scenarios that require details of the picture, such as PGC content production, users usually complain that the face dermabrasion is too obvious and a bit fake.
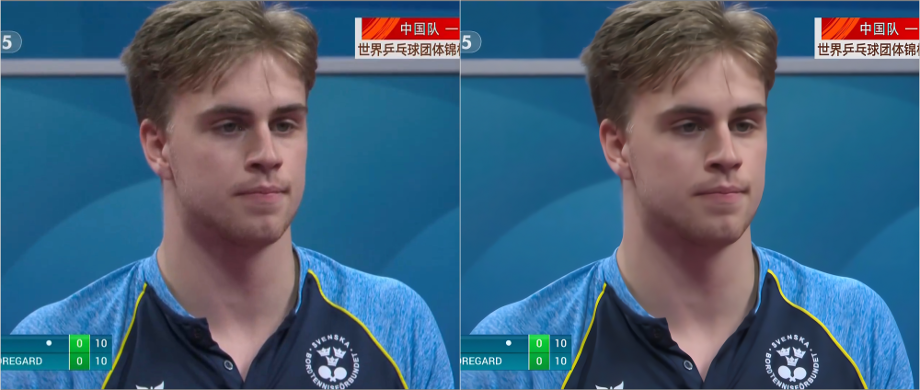
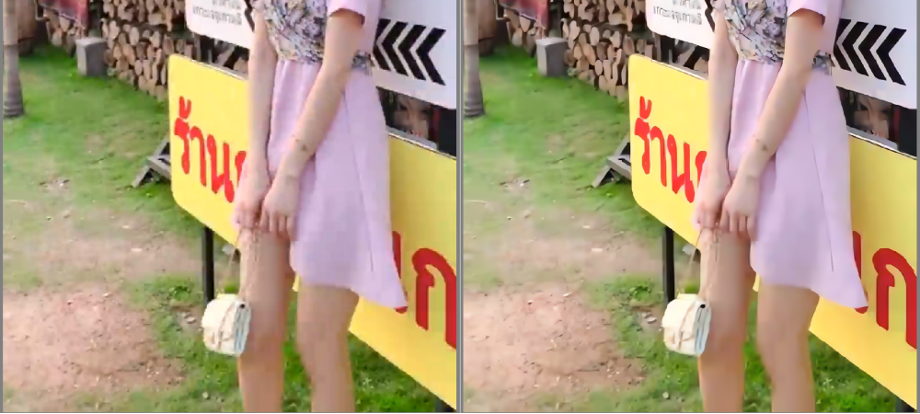
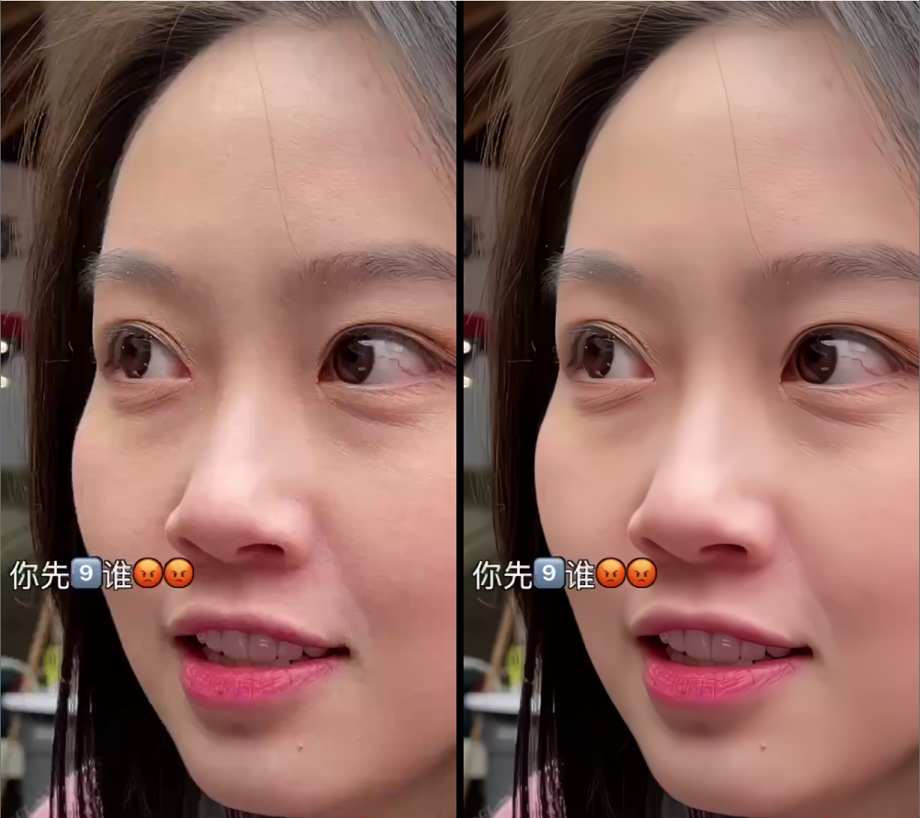
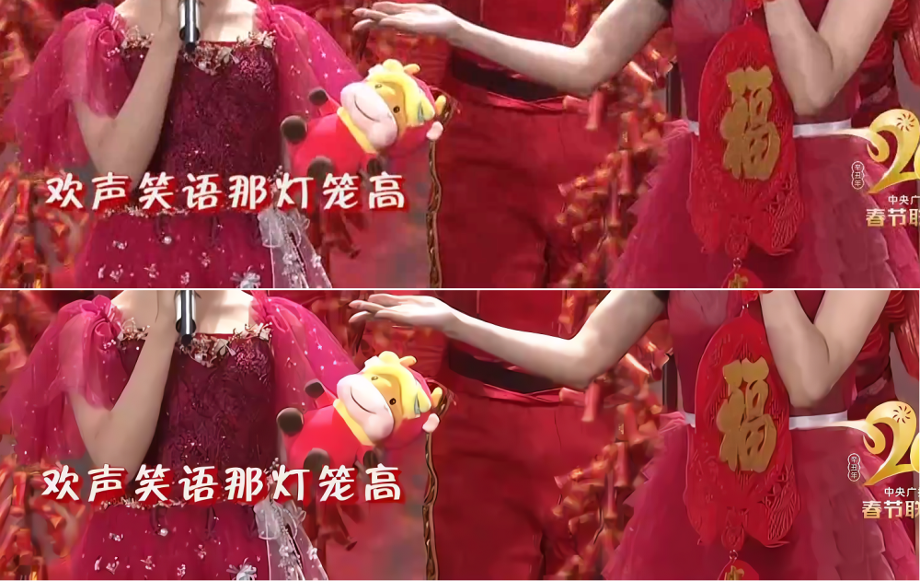
Figure 5 Demonstration of conventional CNN model processing effect:
After processing, encoding artifacts are effectively removed, and the picture is relatively clean and smooth.
But it lacks details and textures, such as details such as hair/eyebrows/beard/skin graininess/lip texture in the portrait area;
The texture details of the grass on the ground and the details of the actor's costumes and props in the video of the party show are lost
l GAN-based processing scheme
In order to solve the problem of lack of details and over-smoothing in the conventional CNN super-resolution model, the academic community proposed a super-resolution scheme based on the generation confrontation network (GAN) in 2017: super-resolution generation confrontation network (SRGAN) [5]. In the process of model training, SRGAN additionally uses a discriminator to identify the texture authenticity of the model output results, so that the model tends to output results with certain detailed textures.
As shown in the figure below, the MSE-based model tends to output smooth results, while the GAN-based model tends to output results with certain texture details.
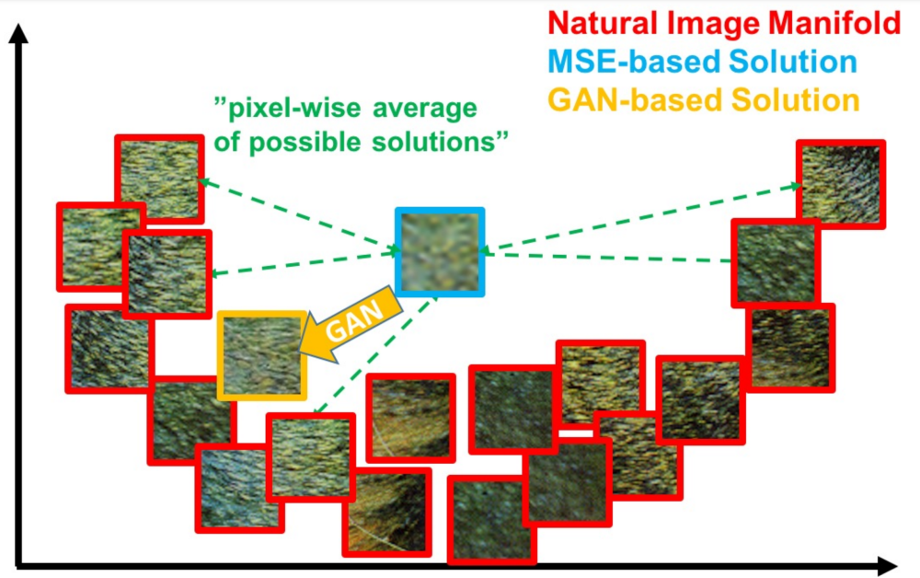
Figure 6 GAN-based SR scheme
Figure source: Paper Photo-Realistic Single Image Super-Resolution Using a Generative Adversarial Network
The GAN-based super-resolution model has the ability to generate details "out of nothing", so it can supplement the missing texture details of the original picture, which is of great help to solve the over-smoothing problem of the conventional CNN model. After the SRGAN model, there has been a lot of work in the academic community to continuously improve this technical direction [6, 7].
05 Narrowband high-definition GAN detail generation technology: time-domain stable detail generation capability
However, there are still many difficulties in technical implementation to make good use of GAN generation technology in actual business scenarios, especially in narrowband high-definition automatic transcoding operations. Since the texture details of GAN are "brain supplemented" after a large amount of data training, whether the detailed texture generated by "brain supplement" is natural, whether it is inconsistent with the original picture, and whether the generated results of adjacent frames are consistent etc., is very important to whether this technology can be successfully applied in actual video services.
Specifically, in order to use the GAN generation capability in narrowband high-definition automatic transcoding operations, the following problems need to be solved to meet commercial requirements:
l The texture generated by the "brain supplement" of the model is natural, and there is no sense of dissonance with the original picture;
l The generation effect of adjacent video frames is highly consistent, and there is no time-domain flicker phenomenon in continuous playback;
l Can be applied to automatic processing flow: the model has a good adaptive ability to the quality of the film source, and can benefit from film sources with different degrees of loss in image quality;
l The model can be applied to different types of video scenes, such as film and television dramas, variety shows, competitions, cartoons, etc.;
l The model processing process is simple, and the processing time is predictable and controllable (live broadcast scenarios have relatively high requirements for processing efficiency).
After continuous research on GAN generation technology, the Alibaba Cloud video cloud audio and video algorithm team has accumulated a number of GAN model optimization technologies, solved the above-mentioned difficulties in the commercial implementation of GAN detail generation capabilities, and created a system that can be applied to fully automatic transcoding operations. GAN detail generation scheme for . The core advantage of this solution is: time-domain stable detail generation capability.
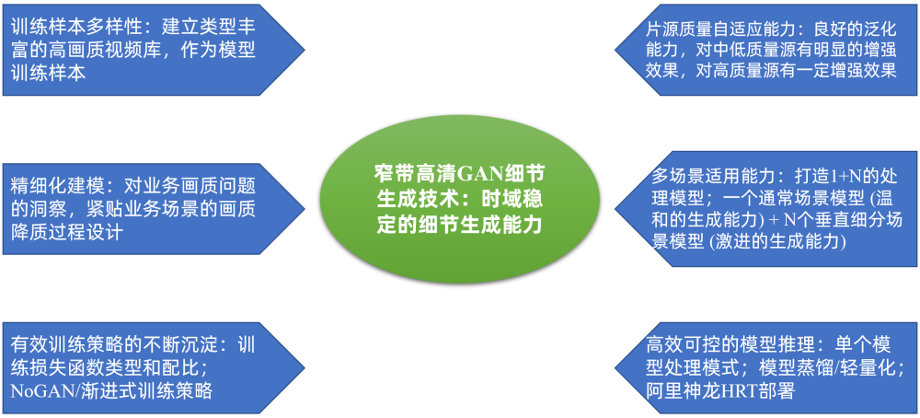
Figure 7 Alibaba Cloud narrowband HD GAN detail generation technology
Specifically, during the training process of the narrowband HD GAN detail generation model, we used the following optimization techniques:
1. Establish a high-quality video library with rich types, high definition, and rich details as high-definition samples for model training. The training samples contain a variety of texture features, which is of great help to the realism of GAN-generated textures;
2. Continuously optimize the training data preparation process through refined modeling: Based on the in-depth insight into the image quality problems faced by business scenarios, the training sample modeling method is continuously optimized to fit the business scenarios, and continuous exploration is used to achieve refined modeling;
3. Explore and accumulate effective model training strategies:
l Loss function: Training loss function configuration tuning, for example, perceptual loss uses different layer features, which will affect the granularity of generated textures, and the weight ratio of different losses will also affect the effect of texture generation;
l Training method: We used a training strategy called NoGAN in the model training process [8]. In image/video coloring GAN model training, the NoGAN training strategy has been proven to be a very effective training technique: on the one hand, it can improve the processing effect of the model, and on the other hand, it is also helpful for the stability of the model generation effect.
4. The adaptability of the model to the quality of the film source determines whether it can be applied to automatic processing operations. In order to improve the adaptability of the model to the source quality, we have done a lot of work on the diversity of training input sample quality and the training process. In the end, the GAN model we trained has a good ability to adapt to the quality of video sources: it has obvious detail generation enhancement capabilities for medium and low-quality video sources, and has a moderate enhancement effect for high-quality video sources;
5. Create multi-scenario processing capabilities: According to the experience of the academic community, the clearer the prior information of the processing target, the stronger the generation ability of GAN. For example, using GAN technology for face or text restoration, because of its single processing object (a low-dimensional manifold in a high-dimensional space), can get very amazing repair effects;
Therefore, in order to improve the processing effect of GAN on different scenarios, we adopted a "1+N" processing mode: "1" is to create a GAN generation model suitable for general scenarios, which has a relatively moderate generation ability; "N "is multiple vertical subdivision scenes. For vertical subdivision scenes, on the basis of the general scene model, the specific texture details of the scene are generated more aggressively. Strong generation effect; for cartoon scenes, the model has stronger generation ability for lines; for variety shows and stage performance scenes, the model has stronger generation ability for portrait close-up details. Special Note: As mentioned below, for the improvement of the generation effect of specific targets, we have not adopted a plan to deal with specific targets alone;
6. Controllable and predictable processing mode with computational complexity: The live broadcast scene has high requirements on the operating efficiency of the processing model. In order to meet the needs of live broadcast image quality enhancement, we currently adopt a single model processing mode, that is, a single model is used to process the full-frame images uniformly. Even if it is necessary to improve the generation effect of some specific targets, such as the portrait area and the grass texture of the football field, we did not adopt the scheme of extracting the targets and processing them separately. Therefore, our model inference time is predictable independent of image content. After model distillation and lightweight, based on the Alibaba Cloud Shenlong HRT GPU inference framework, our GAN detail generation model can be processed on a single NVIDIA Tesla V100 with a processing efficiency of 60fps@1920x1080.
GAN generates temporal stability guarantee technology
In order to ensure the inter-frame consistency of the GAN model generation effect and avoid visual flicker caused by inter-frame discontinuity, we have proposed a plug-and-play inter-frame consistency enhancement model-Temporal Consistency by cooperating with universities. Refinement Network (TCRNet). The workflow of TCRNet mainly includes the following three steps:
l Perform post-processing on the single-frame GAN processing results to enhance the inter-frame consistency of the GAN processing results and at the same time enhance some details and improve the visual effect;
l Use Iterative Residual Refinement of Offset Module (IRRO) combined with deformable convolution to improve the accuracy of inter-frame motion compensation;
l Use the ConvLSTM module to enable the model to fuse longer-distance timing information. And the spatial motion compensation is performed on the transmitted time series information through deformable convolution to prevent the information fusion error caused by the offset.
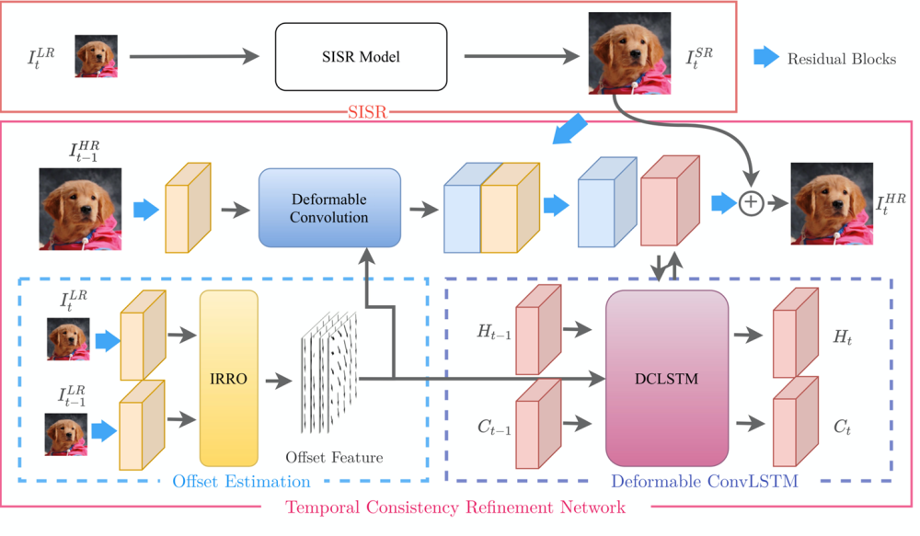
Figure 8 TCRNet algorithm flow, source: Paper Deep Plug-and-Play Video Super-Resolution
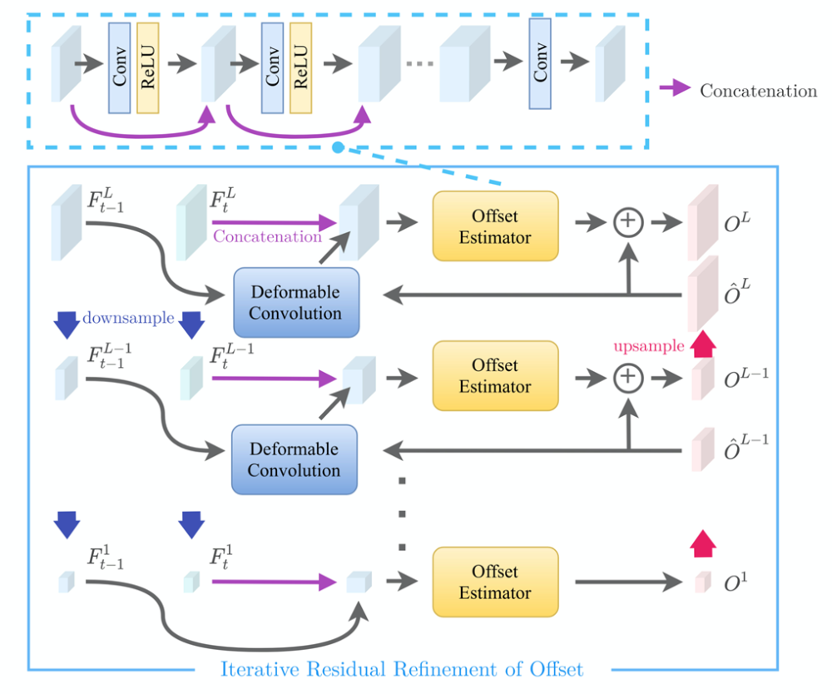
Figure 9 Algorithm flow of iterative offset correction module (IRRO)
Source: Paper Deep Plug-and-Play Video Super-Resolution
Narrowband HD GAN detail generation: Is this face effect fake?
Going back to the examples of conventional CNN processing effects mentioned above, let's take a look at the different results of using narrow-band high-definition GAN detail generation processing. For these examples, we use a generic scene model for processing.
Legend: from left to right: Narrowband HD GAN processing, input original frame, conventional CNN processing effect
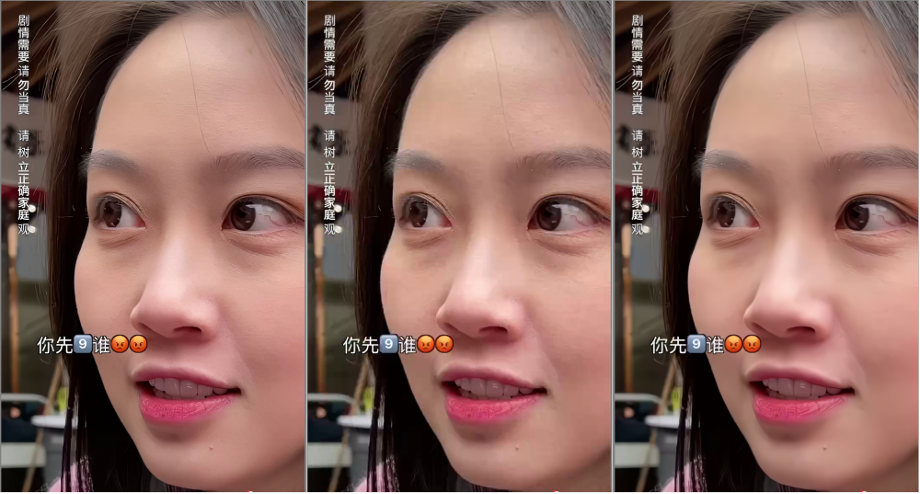
Figure 10 The skin of the human face has a grainy feel, which has a skin texture; the hair and eyebrows have a silky feeling; the texture of the lips is richer
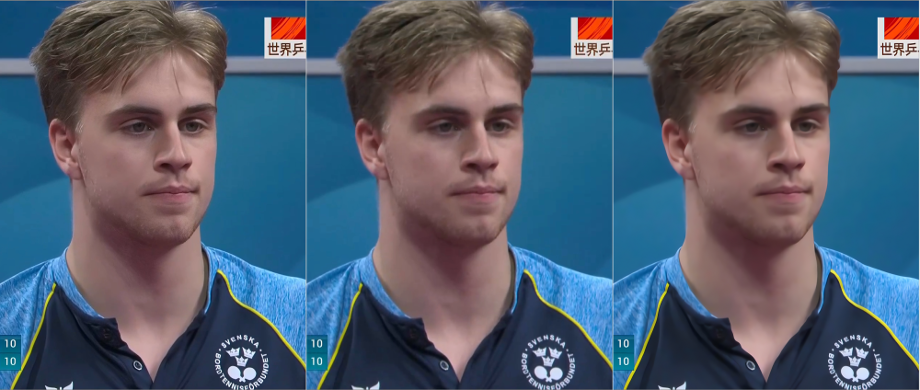
Figure 11 The details of the hair and beard are richer, and the face will not feel smooth
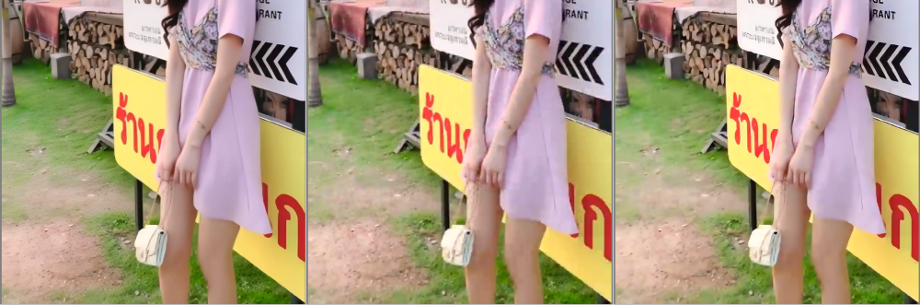
Figure 12 The ground/grass texture is richer and the details are clearer
Legend: from top to bottom: Narrowband HD GAN processing, input original frame, conventional CNN processing effect
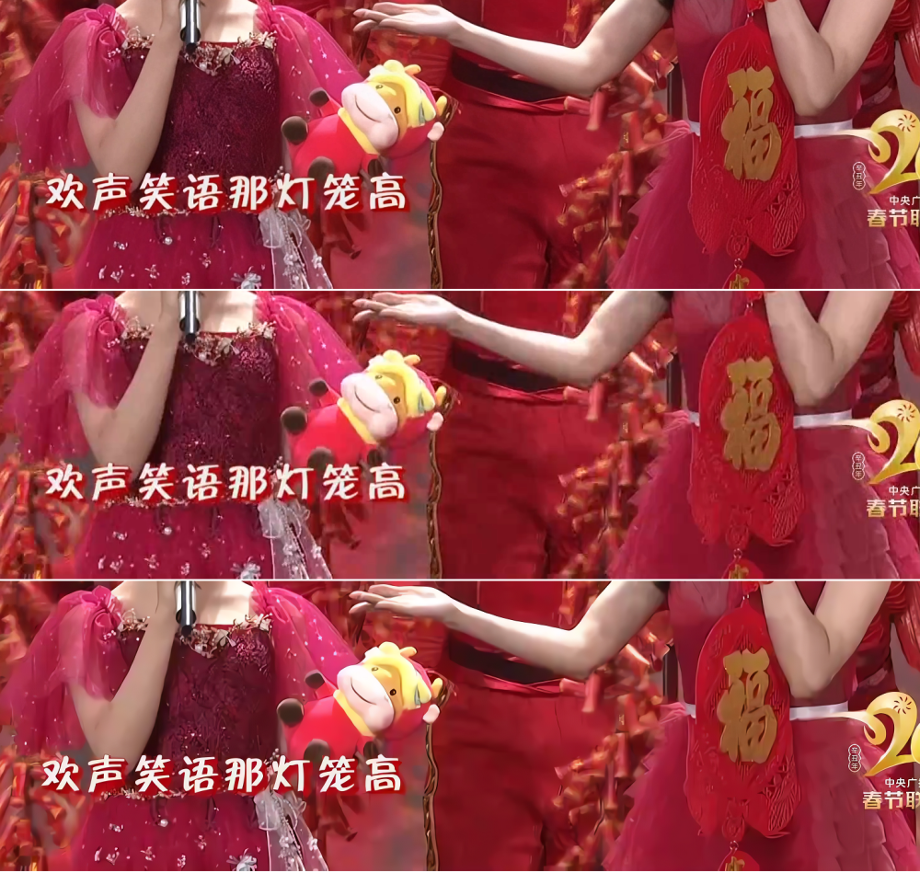
Figure 13 The actor's skirt on the left has richer texture; the actor's props on the right have richer texture and clearer details
The legend is limited to demonstrate the effect of the technical solution, from left to right are: narrow-band high-definition GAN processing, input original frame
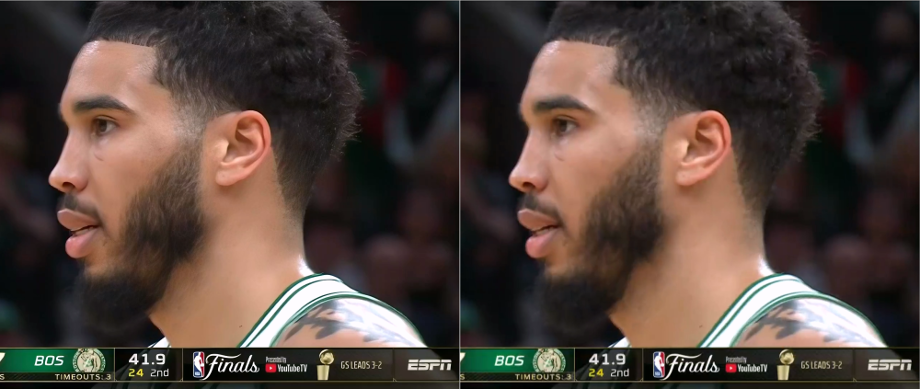
Figure 14 The hair and beard area has obvious details generated, and the texture is richer
As we mentioned earlier, for vertical subdivision scenes, the model will perform more aggressive texture generation for the specific targets of the scene. For example, for a football game scene, the model has a stronger ability to generate the grass texture of the field. Below are two examples:
Legend: from left to right: Narrowband HD GAN processing, input original frame
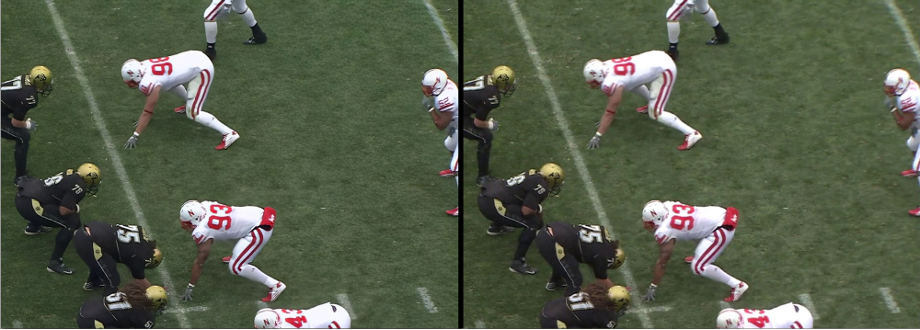
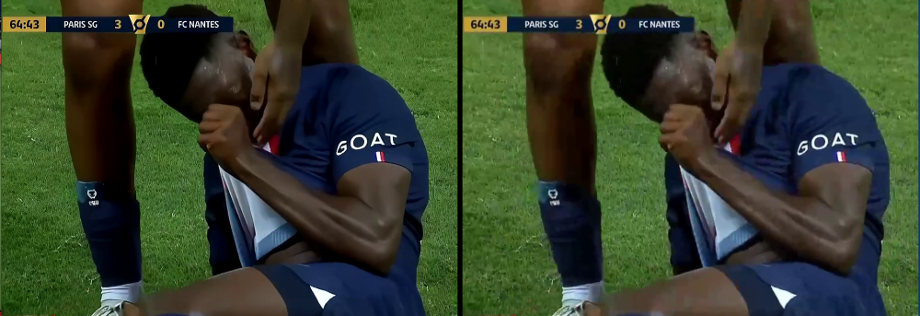
Figure 15 Football game scene, grass texture generation effect
In addition, for cartoon scenes, we also train a targeted GAN model, focusing on line generation capabilities. The following are the processing effects of the three cartoons.
Legend: from left to right: Narrowband HD GAN processing, input original frame
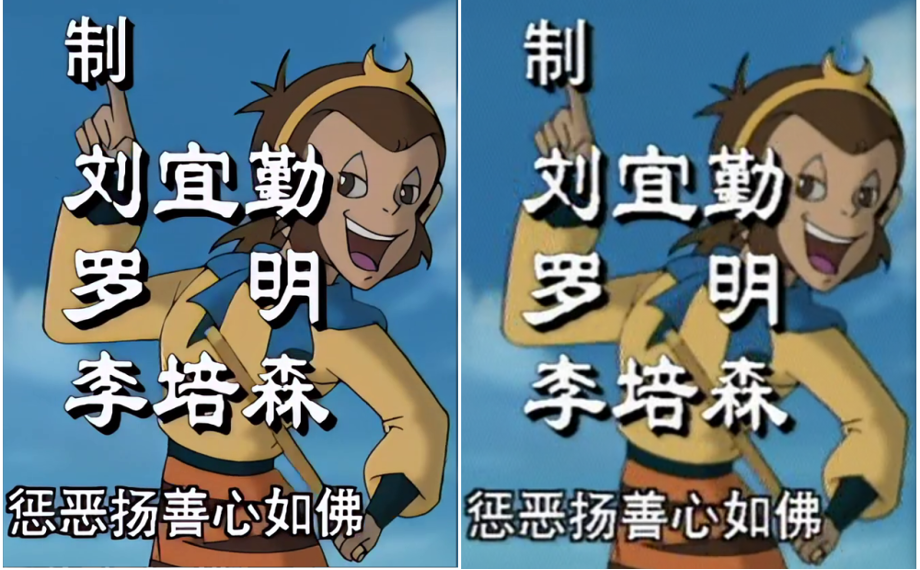
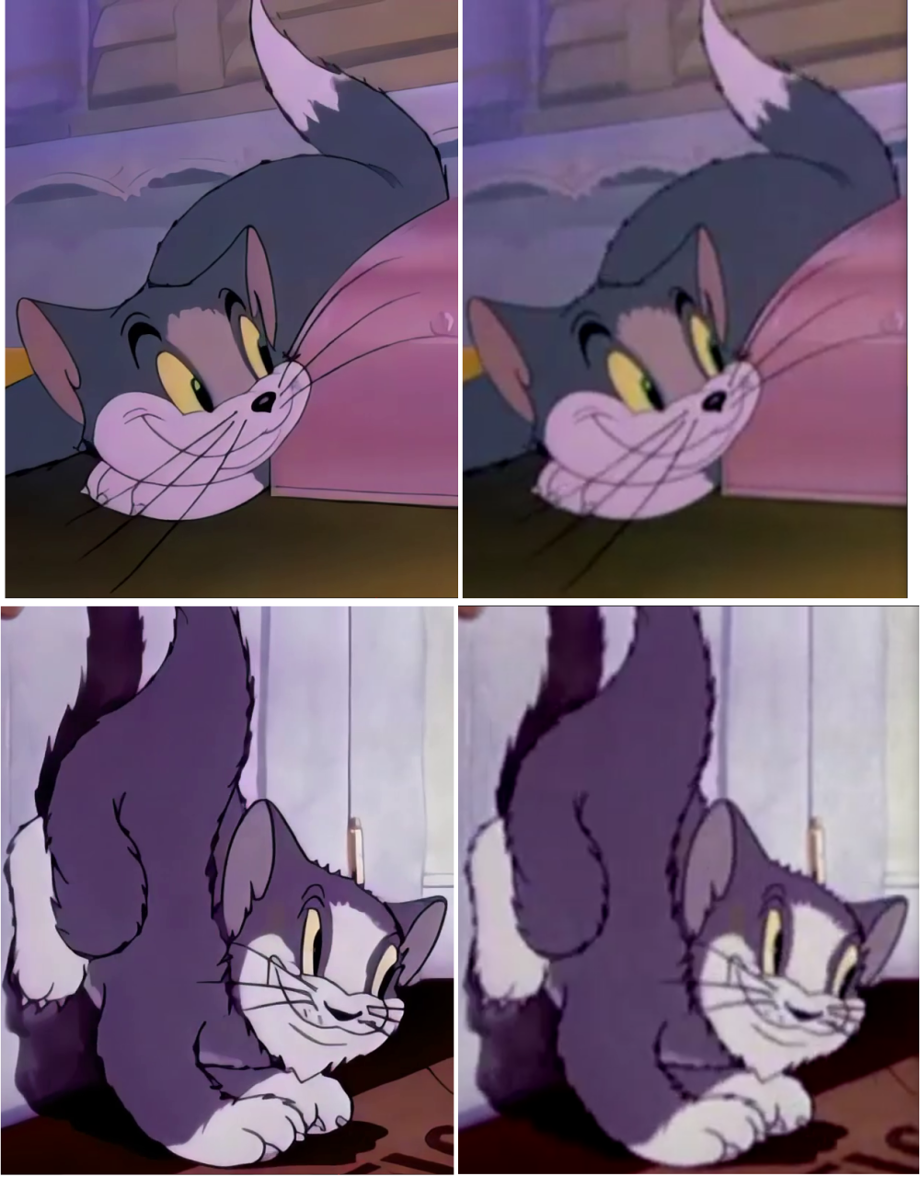
Figure 16 Animation processing effect
Narrowband high-definition GAN detail generation technology is commercially available
Currently, narrowband high-definition GAN detail generation capability has been fully enabled in Blockbuster TV NBA live transcoding. When you watch NBA games on BesTV APP, select the "Blu-ray 265" gear, and you can experience the image quality based on the transcoding output of narrow-band high-definition GAN detail generation capabilities. At the same time, Block TV has also used this function in the live broadcast of some variety shows and large-scale events.
In addition, in the FIFA2022 World Cup broadcast, Jiangsu Mobile used narrow-band high-definition GAN detail generation technology to improve the quality of Migu Video's original set-top box distribution stream. During the one-month event broadcast period, Narrowband HD provided picture quality enhancement capabilities for Jiangsu Mobile's 24-hour uninterrupted live broadcast.
In addition to Blockbuster TV and Jiangsu Mobile, several customers are currently testing the narrowband HD GAN detail generation capability, and the results of the POC test have been highly recognized by customers.
Demonstration of customer scene image quality enhancement effect:
On the left, the live streaming signal source of Baishi TV APP; on the right: narrow-band high-definition image enhancement output
On the left, Jiangsu Mobile’s live streaming signal source (Migu 8M); on the right: Narrow-band HD image enhancement output
Videos with high resolution, rich textures, and clear details can provide clearer images and a higher-level sensory experience, which is of great help in improving video quality and user visual experience. Narrowband high-definition GAN detail generation and restoration technology will continue to explore and improve in this field to create the ultimate detail restoration and enhancement effect, and provide video consumers with a high-quality video viewing experience.
In the future, the narrow-band high-definition GAN detail generation capability will continue to optimize algorithm performance, improve detail generation and repair effects, and continuously reduce processing costs.
better! Improve the effect of detail generation and restoration; in addition to the current GAN scheme, the detail generation technology based on the diffusion model will also be the key direction of our follow-up research;
Wider! Create more vertical subdivision scenes, and adopt aggressive generation strategies to improve the detail recovery effect of corresponding scenes;
More inclusive! Through the lightweight model and optimized deployment plan, the processing cost is continuously reduced, and more customers are served at an inclusive price.
[The videos and pictures involved in this article are actual cases and are only used for technology sharing and effect display]
Attachment: References
[1] https://cvpr2016.thecvf.com/program/demos
[2] https://www.gov.uk/government/news/magic-pony-technology-twitter-buys-start-up-for-150-million
[3] Wenzhe Shi et al., Real-Time Single Image and Video Super-Resolution Using an Efficient Sub-Pixel Convolutional Neural Network, CVPR 2016
[4] NTIRE 2017 Challenge on Single Image Super-Resolution: Dataset and Study, CVPRW 2017
[5] Christian Ledig et al., Photo-Realistic Single Image Super-Resolution Using a Generative Adversarial Network, CVPR 2017
[6] Kai Zhang et al., Designing a Practical Degradation Model for Deep Blind Image Super-Resolution, ICCV 2021
[7] Xintao Wang et al., Real-ESRGAN: Training Real-World Blind Super-Resolution with Pure Synthetic Data, ICCVW 2021
[8] https://www.fast.ai/posts/2019-05-03-decrappify.html#nogan-training
[9] Hannan Lu et al., Deep Plug-and-Play Video Super-Resolution, ECCVW 2020
thank you
Special thanks to the following students for their contributions to the algorithms involved in this paper. @刘佳辉(佳诺) @吕热瑶(相泉) @李岁丹(Suixi) @王伟(静瑶) @邵威航(生辉) @周明才(Mingshuo)