Reprinted from: https://zhuanlan.zhihu.com/p/27312651
In the contingency table, the two-dimensional table is the most basic type of table, and in the two-dimensional table, the four-table table is the most basic type of table.
The basic form of the four-table table is in " Classic Comparison Chapter 11: How to do the ratio comparison of small samples? " has been introduced, here is the table posted.
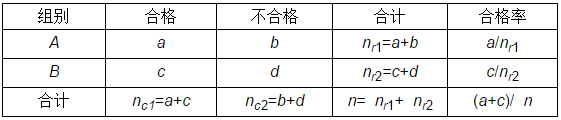
The corresponding analysis methods are described below for various forms of tabular data.
The analysis method of the basic four-table table
1. Normal approximation
The basic four-table table is actually a comparison of two ratios (that is, the last column of the above table). When the ratios satisfy the conditions np and n (1- p ) are greater than 5, normal approximation can be used for analysis. This is familiar to everyone and will not be covered in this article.
2. Chi-square test
The principle of chi-square test has been introduced in the previous article, see "Contingency Table One: Analysis of Two-way Unordered Table". For the four-table table, there is a dedicated formula for calculating the chi-square value:

This formula no longer needs to calculate the expected frequency, and it is not difficult to remember. It is here for everyone's reference.
3. Correction formula for chi-square test
The data in the four-table table is not continuous, so the calculated chi-square values are not continuous, but the distribution is continuous. When the degree of freedom is very small, especially when there is only 1 in the four-table table, the calculated chi-square value is small, and the probability of false positives increases. To this end, American statistician F. Yates proposed a continuity correction formula for calculating the chi-square value in 1934:
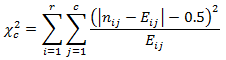
In particular, for the four-table table
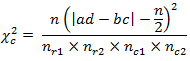
4. Fisher's exact test
This is a test method in the case of small samples. For the four-table table, it uses hypergeometric distribution for testing. For specific methods and steps, see "Classic Comparison Chapter 11: How to do the ratio comparison of small samples? ". For contingency tables larger than four grids, when the sample size is relatively small, especially when the expected frequency of less than 5 grids exceeds 20%, Fisher's exact test needs to be used, but the method is more complicated and requires software to calculate.
5. Choice of method
In Professor Sun Zhenqiu's "Medical Statistics" p.114, he introduced three selection principles for the chi-square test of the four-table table:
(1) When n ≥ 40 and all E ≥ 5 (that is , the theoretical frequency of the chi-square distribution corresponding to a , b , c , d ), the basic formula of the chi-square test can be used, but when p ≈ α , change Fisher's exact test was used.
(2) When n ≥ 40 but 1 ≤ E < 5, use the correction formula of chi-square test, or use Fisher's exact test instead.
(3) When n < 40, or E < 1, use Fisher's exact test of the four-table table.
Of course, now that the statistical software is very functional, I think that no matter what the data in the table belongs to, it will always be relatively accurate to use Fisher's exact test directly. Personal opinion, for reference only.
Chi-square test for paired four-table
Those who are familiar with classical comparisons know that there is a paired t -test, and there is also a paired contingency table in the contingency table. Similar to the paired t -test, the paired contingency table also requires the sample to remain unchanged, such as a comparison of parts before and after processing, or a comparison of two different evaluation methods. The table can be further written like this:
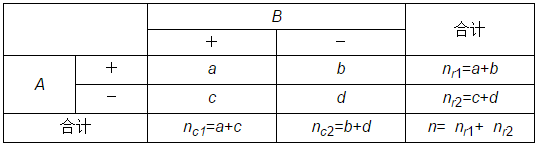
For the paired four-bar table, there are two analytical methods to choose from, namely Mcnemar's test and Kappa's test. The former focuses on differences, while the latter focuses on consistency.
1. Mcnemar test
a and d represent consistency of results, b and c represent variation in results. In the Mcnemar test, the null hypothesis is that the treatment applied to the sample has no significant effect, that is, the possibility of changes in different directions is the same, and there should be as many "+-" as there are "-+", that is, b = c , If the two are very different, it means that there is a significant difference between two different treatments, or there is a significant difference between the before and after states of one treatment.
From another perspective, Mcnemar's null hypothesis is that the marginal probabilities are equal, i.e.
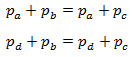
So the hypothesis of the Mcnemar test can be written as

The test statistic thus established is:
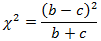
The slave statistic follows a distribution with 1 degree of freedom.
Example 1: A company plans to introduce Six Sigma management. For this purpose, 100 employees are selected. Before and after the implementation of the Six Sigma strategy, a survey is conducted on the necessity of introducing Six Sigma. The results of the survey are as follows. Q: Did the attitude of the staff change before and after the presentation?
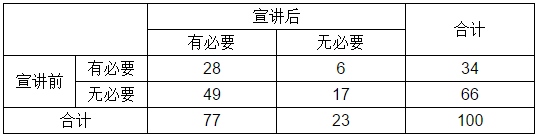
Suppose you don't write it, everyone knows how to write it. The calculated chi-square value is:
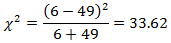
When α = 0.05, the critical value of the chi-square test with 1 degree of freedom is 3.84, so we reject the null hypothesis and believe that there is a significant change in the attitude of employees before and after the presentation.
According to Professor Sun Zhenqiu, when b + c < 40, the test statistic needs to be corrected, i.e.
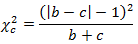
Some data point out that when b + c <25, the chi-square test will have a large deviation, and the exact test of the binomial distribution should be used at this time. The Mcnemar test becomes a one-proportion test, and the hypothesis becomes

where n = b + c . Single ratio test in "Classic Comparison Chapter Eleven: How to do the ratio comparison of small samples? " has been introduced, and will not be repeated here.
The Mcnemar test has nothing to do with the values of the two grids a and d . When these two values are large, even if the test result is significant, its practical significance is not very large. Therefore, we need to consider the problem of consistency, which requires Kappa test.
2. Kappa test
Seeing the Kappa test, people who are familiar with measurement system analysis will immediately remember that in attribute data measurement system analysis, Kappa value is widely used to measure the consistency of measurement results. This is described in detail in Professor Ma Fengshi's "Six Sigma Management Statistics Guide" p.399-402 .
The Kappa test was proposed by Cohen in 1960, so it is also called Cohen's Kappa. It considers whether the actual result is a random guess. For example, a new employee does not understand the test standard, but can also be partially wrong. The Kappa value is a measure of this, and its formula is:

in

is the actual consistent ratio, and

is the expected coincidence rate, that is, the ratio of matching.
The value of Kappa ranges from -1 to +1. -1 means complete disagreement ( a = d = 0 and b = c ); +1 means complete agreement ( b = c = 0 ); 0 means the result is purely blind; a negative value means the result is worse than blind (of course It also has little practical significance, and actually occurs very rarely); the closer the positive value is to 1, the better the consistency. Usually above 0.75 means satisfactory consistency, below 0.4 the consistency is not good. But for the measurement system, it needs to be above 0.9 to be a good measurement system.
Example 2: A factory generally uses two methods of manual inspection and equipment inspection for the surface quality of injection molded products. In order to understand the consistency of the two inspection methods, 35 samples were randomly selected and inspected by two methods respectively. The results are shown in the following table.
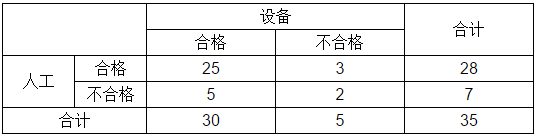
According to the above formula, the Kappa value is 0.2, indicating that the consistency of the results of the two tests is very poor.
One might ask that this analysis does not tell us which is better. In order to confirm which method is better, the standard factor can be added, that is, the samples are carefully identified by experts, the results of the standard are determined, and then the results of the two inspection methods are compared with this. One of the tables looks like this:
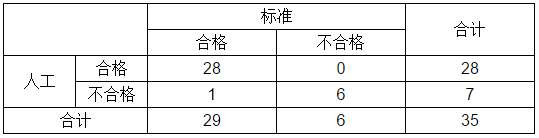
The Kappa value calculated according to this table is 0.906, indicating that the accuracy of manual inspection is very high.
The Kappa value is rarely tested for significance, so this article does not consider the issue of its distribution and test.
The Kappa value can also analyze contingency tables with more than four grids . The row sums of are multiplied by the column sums, then added and divided by the square of the total sample size. This is a bit difficult to understand, I don't want to list too many formulas, and use an example to illustrate.
Example 3: There are 80 multiple-choice questions in an exam, and each question has four answers: A, B, C, and D. In order to check whether a candidate's score is a random guess, you can use Kappa analysis to make a more accurate answer. judge. The data table is as follows:
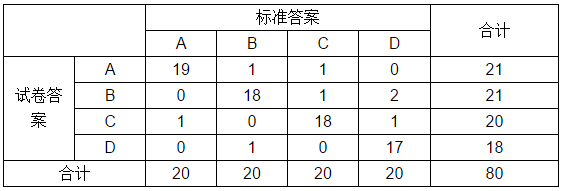
The exact agreement is 19+18+18+17=72, and P 0=72/80=0.9 is calculated.
Pe = (21 × 20 + 21 × 20 + 20 × 20 + 18 × 20) /80^2=0.25。
From this, Kappa=(0.9-0.25)/(1-0.25)=0.867 is calculated. This value is relatively large, indicating that the student's answer is not blind, but really learned.