原文:https://mp.weixin.qq.com/s/BDHXQHXSzDk-RTi-VNMNEw
1 scipy.optimize の概要
scipy.optimize パッケージは、一般的に使用されるいくつかの最適化アルゴリズムを提供します。
このモジュールには以下が含まれます。
1. さまざまなアルゴリズム (BFGS、ネルダーミード単体、ニュートンの共役勾配、COBYLA、SLSQP など) を使用した多変量スカラー関数の制約なしおよび制約なしの最小化 (最小化)
2. グローバル (総当たり) 最適化ルーチン (例: 盆地ジャンプ、difference_evolution)
3. 最小二乗最小化 (least_squares) および曲線近似 (curve_fit) アルゴリズム
4. スカラー単変数関数ミニマイザー (minimum_scalar) とルート ファインダー (Newton)
5. 複数のアルゴリズムを使用する多変量システム ソルバー (ルート) (ハイブリッド パウエル、レーベンバーグ-マルカート、またはニュートン-クリロフなどの大規模な方法など)。
詳細については、以下を参照してください。
https://docs.scipy.org/doc/scipy/reference/optimize.html
# https://docs.scipy.org/doc/scipy/reference/generated/scipy.optimize.minimize.html
from scipy.optimize import minimize
scipy.optimize.minimize(
fun, #可调用的目标函数。
x0, #ndarray,初值。(n,)
args=(), #额外的参数传递给目标函数及其导数
method=None, #类型的解算器。应该是其中之一:
#‘Nelder-Mead’、‘Powell’
#‘CG’、‘BFGS’
#‘Newton-CG’、‘L-BFGS-B’
#‘TNC’、‘COBYLA’
#‘SLSQP’、‘dogleg’
#‘trust-ncg’
jac=None, #目标函数的雅可比矩阵(梯度向量)。
#仅适用于CG, BFGS, Newton-CG,
#L-BFGS-B, TNC, SLSQP, dogleg,
#trust-ncg。如果jac是一个布尔值,
#且为True,则假定fun将随目标函数返回
#梯度。如果为False,则用数值方法估计梯
#度。Jac也可以是返回目标梯度的可调用对
#象。在这种情况下,它必须接受与乐趣相同
#的论点。
hess=None,
hessp=None,#目标函数的Hessian(二阶导数矩阵)或
#目标函数的Hessian乘以任意向量p。
#仅适用于Newton-CG, dogleg,
#trust-ncg。只需要给出一个hessp或
#hess。如果提供了hess,则将忽略
#hessp。如果不提供hess和hessp,则用
#jac上的有限差分来近似Hessian积。
#hessp必须计算Hessian乘以任意向量。
bounds=None, #变量的边界(仅适用于L-BFGS-B,
#TNC和SLSQP)。(min, max)
#对x中的每个元素,定义该参数的
#边界。当在min或max方向上没有边界
#时,使用None表示其中之一。
constraints=(), #约束定义
#(仅适用于COBYLA和SLSQP)
# 类型有: ‘eq’ for equality, ‘ineq’ for inequality
tol=None, #终止的边界。
callback=None,
options=None)
返回值: res : OptimizeResult
#以OptimizeResult对象表示的优化结果。重要的属性有:x是解决方案数组,
#success是一个布尔标志,指示优化器是否成功退出,以及描述终止原因的消息。
2 多変量スカラー関数の制約なしの最小化
2.1 シンプレックス法: ネルダー・ミード
ローゼンブロック関数:
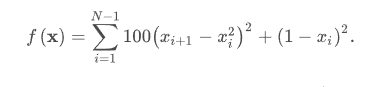
x=1の場合は最小値をとる。
def rosen(x):
"""The Rosenbrock function"""
return sum(100.0 * (x[1:] - x[:-1] ** 2.0) ** 2.0 + (1 - x[:-1]) ** 2.0)
解決:
import numpy as np
from scipy.optimize import minimize
# 初始迭代点
x0 = np.array([1.3, 0.7, 0.8, 1.9, 1.2])
# 最小化优化器,方法:Nelder-Mead(单纯形法)
res = minimize(rosen, x0, method='nelder-mead',
options={
'xatol': 1e-8, 'disp': True})
print(res.x)
#
Optimization terminated successfully.
Current function value: 0.000000
Iterations: 339
Function evaluations: 571
[1. 1. 1. 1. 1.]
パラメーターを使用して Rosenbrock 関数を検索します。

def rosen_with_args(x, a, b):
"""The Rosenbrock function with additional arguments"""
return sum(a*(x[1:]-x[:-1]**2.0)**2.0 + (1-x[:-1])**2.0) + b
res = minimize(rosen_with_args, x0, method='nelder-mead',
args=(0.5, 1.), options={
'xatol': 1e-8, 'disp': True})
2.2 準ニュートン法:BFGSアルゴリズム
導入:
https://blog.csdn.net/jiang425776024/article/details/87602847
ローゼンブロック導関数:
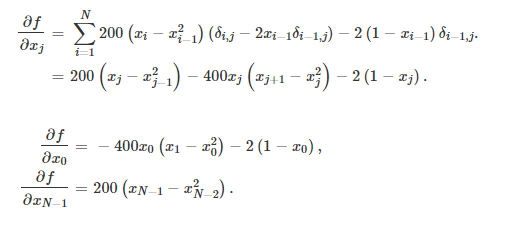
def rosen_der(x):
# rosen函数的雅可比矩阵
xm = x[1:-1]
xm_m1 = x[:-2]
xm_p1 = x[2:]
der = np.zeros_like(x)
der[1:-1] = 200 * (xm - xm_m1 ** 2) - 400 * (xm_p1 - xm ** 2) * xm - 2 * (1 - xm)
der[0] = -400 * x[0] * (x[1] - x[0] ** 2) - 2 * (1 - x[0])
der[-1] = 200 * (x[-1] - x[-2] ** 2)
return der
解決:
# 初始迭代点
res = minimize(rosen, x0, method='BFGS', jac=rosen_der,
options={
'disp': True})
print(res.x)
勾配情報を提供するもう 1 つの方法は、ターゲットと勾配を返す関数を作成することです。これは、jac=True を設定することで表現できます。この場合、最適化される Python 関数は、最初の値がターゲットで 2 番目の値が勾配を表すタプルを返す必要があります。
def rosen_and_der(x):
objective = sum(100.0*(x[1:]-x[:-1]**2.0)**2.0 + (1-x[:-1])**2.0)
xm = x[1:-1]
xm_m1 = x[:-2]
xm_p1 = x[2:]
der = np.zeros_like(x)
der[1:-1] = 200*(xm-xm_m1**2) - 400*(xm_p1 - xm**2)*xm - 2*(1-xm)
der[0] = -400*x[0]*(x[1]-x[0]**2) - 2*(1-x[0])
der[-1] = 200*(x[-1]-x[-2]**2)
return objective, der
res = minimize(rosen_and_der, x0, method='BFGS', jac=True,
options={
'disp': True})
2.3 ニュートン法:Newton-CG
ヘッセ行列と勾配を使用して最適化します。
導入:
https://blog.csdn.net/jiang425776024/article/details/87602847
目的関数の近似二次形式を構築します (テイラー展開)。

ヘッセ行列 H と勾配を使用して反復します。
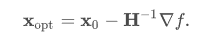
ヘッセ行列:
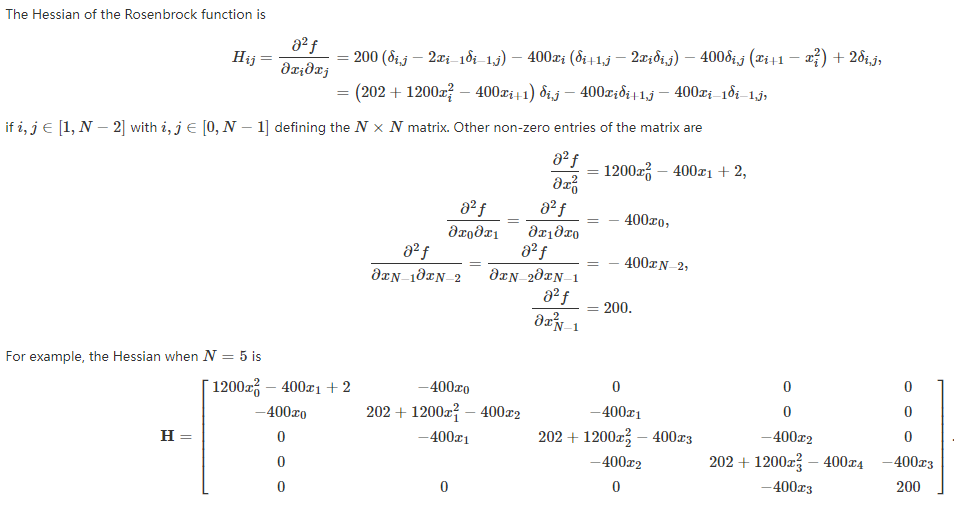
def rosen_hess(x):
x = np.asarray(x)
H = np.diag(-400*x[:-1],1) - np.diag(400*x[:-1],-1)
diagonal = np.zeros_like(x)
diagonal[0] = 1200*x[0]**2-400*x[1]+2
diagonal[-1] = 200
diagonal[1:-1] = 202 + 1200*x[1:-1]**2 - 400*x[2:]
H = H + np.diag(diagonal)
return H
res = minimize(rosen, x0, method='Newton-CG',
jac=rosen_der, hess=rosen_hess,
options={
'xtol': 1e-8, 'disp': True})
res.x
# array([1., 1., 1., 1., 1.])
より大きな最小化問題の場合、ヘッセ行列全体を保存すると、多くの時間とメモリが消費されます。行列は目的関数の形式で記述できます。
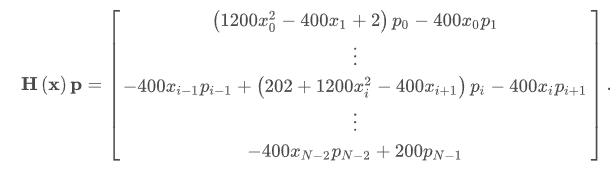
def rosen_hess_p(x, p):
x = np.asarray(x)
Hp = np.zeros_like(x)
Hp[0] = (1200*x[0]**2 - 400*x[1] + 2)*p[0] - 400*x[0]*p[1]
Hp[1:-1] = -400*x[:-2]*p[:-2]+(202+1200*x[1:-1]**2-400*x[2:])*p[1:-1] \
-400*x[1:-1]*p[2:]
Hp[-1] = -400*x[-2]*p[-2] + 200*p[-1]
return Hp
res = minimize(rosen, x0, method='Newton-CG',
jac=rosen_der, hessp=rosen_hess_p,
options={
'xtol': 1e-8, 'disp': True})
res.x
# array([1., 1., 1., 1., 1.])
Newton-CG アルゴリズムは、ヘッセ行列の条件が劣悪な場合には非効率になる可能性があります。これは、このような場合、メソッドによって提供される探索方向の品質が低いためです。trust-ncg アプローチは、この問題のある状況をより効率的に処理します。これについては以下で説明します。
2.4 共役勾配アルゴリズム: trust-krylov
trust-ncg 法と同様に、trust-krylov 法は行列ベクトル積による線形演算子としてヘシアンのみを使用するため、大規模な問題に適した手法です。trust-ncg 法よりも正確に二次部分問題を解きます。
Newton-CG 法は直線探索法です。関数の 2 次近似を最小化する探索方向を見つけ、直線探索アルゴリズムを使用してその方向 (近似) の最適なステップ サイズを見つけます。もう 1 つのアプローチは、最初にステップ サイズの制限を修正し、次に次の 2 次問題を解くことによって、指定された信頼半径内で最適なステップ サイズを見つけることです。
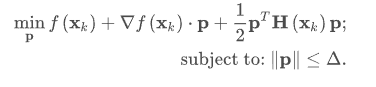
二次モデルと実関数の間の一貫性に従って、解xk + 1 = xk + p x_{k+1}=x_k+p を更新します。バツk + 1=バツk+p、信頼半径Δ \DeltaΔ。このようなメソッドは信頼領域メソッドと呼ばれます。trust-ncg アルゴリズムは、共役勾配アルゴリズムを使用して信頼ドメインの副次問題を解決する信頼ドメインの方法です。
# Full Hessian example
res = minimize(rosen, x0, method='trust-ncg',
jac=rosen_der, hess=rosen_hess,
options={
'gtol': 1e-8, 'disp': True})
# Hessian product example
res = minimize(rosen, x0, method='trust-ncg',
jac=rosen_der, hessp=rosen_hess_p,
options={
'gtol': 1e-8, 'disp': True})
3 多変量スカラー関数の制約付き最小化
最小化関数は、制約付き最小化アルゴリズム、つまり「trust-constr」、「SLSQP」、および「COBYLA」を提供します。制約を定義するには、少し異なる構造が必要です。
メソッド「trust-constr」では、制約を一連の線形および非線形制約オブジェクトとして定義する必要があります。
一方、メソッド「SLSQP」および「COBYLA」では、キー type、fun、jac を含む辞書のシーケンスとして制約を定義する必要があります。
3.1 信頼ドメイン: trust-constr
トラストドメイン制約メソッドで処理される制約付き最小化問題の形式は次のとおりです。
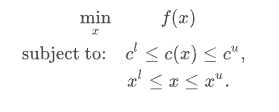
- 制約の境界を定義します。

from scipy.optimize import Bounds
bounds = Bounds([0, -0.5], [1.0, 2.0])
- 線形制約を定義する

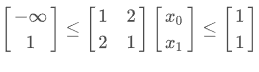
from scipy.optimize import LinearConstraint
linear_constraint = LinearConstraint([[1, 2], [2, 1]], [-np.inf, 1], [1, 1])
- 非線形制約を定義する
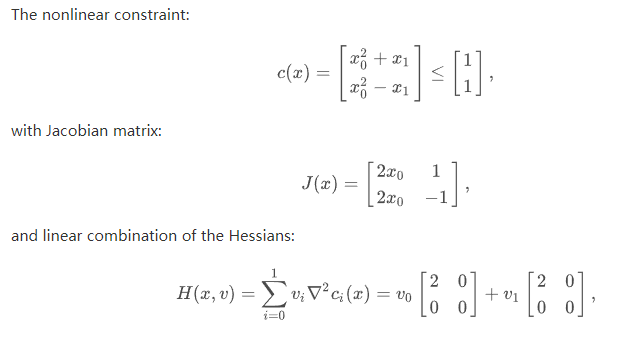
def cons_f(x):
return [x[0]**2 + x[1], x[0]**2 - x[1]]
def cons_J(x):
return [[2*x[0], 1], [2*x[0], -1]]
def cons_H(x, v):
return v[0]*np.array([[2, 0], [0, 0]]) + v[1]*np.array([[2, 0], [0, 0]])
from scipy.optimize import NonlinearConstraint
nonlinear_constraint = NonlinearConstraint(cons_f, -np.inf, 1, jac=cons_J, hess=cons_H)
x0 = np.array([0.5, 0])
res = minimize(rosen, x0, method='trust-constr', jac=rosen_der, hess=rosen_hess,
constraints=[linear_constraint, nonlinear_constraint],
options={
'verbose': 1}, bounds=bounds)
# may vary
# `gtol` termination condition is satisfied.
# Number of iterations: 12, function evaluations: 8, CG iterations: 7,
# optimality: 2.99e-09, constraint violation: 1.11e-16, execution time:
# 0.016 s.
print(res.x)
# [0.41494531 0.17010937]
3.2 逐次最小二乗プログラミング アルゴリズム: SLSQP
制約形式:
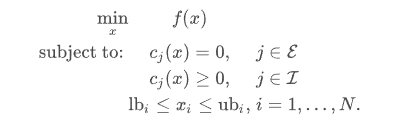
ineq_cons = {
'type': 'ineq',
'fun' : lambda x: np.array([1 - x[0] - 2*x[1],
1 - x[0]**2 - x[1],
1 - x[0]**2 + x[1]]),
'jac' : lambda x: np.array([[-1.0, -2.0],
[-2*x[0], -1.0],
[-2*x[0], 1.0]])}
eq_cons = {
'type': 'eq',
'fun' : lambda x: np.array([2*x[0] + x[1] - 1]),
'jac' : lambda x: np.array([2.0, 1.0])}
ここでの不等式はpymoo≥0
(≤0)の逆であることに注意してください。
x0 = np.array([0.5, 0])
res = minimize(rosen, x0, method='SLSQP', jac=rosen_der,
constraints=[eq_cons, ineq_cons], options={
'ftol': 1e-9, 'disp': True},
bounds=bounds)
# may vary
Optimization terminated successfully. (Exit mode 0)
Current function value: 0.342717574857755
Iterations: 5
Function evaluations: 6
Gradient evaluations: 5
print(res.x)
# [0.41494475 0.1701105 ]
3.3 最小二乗法:least_squares
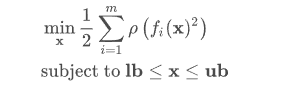
ここでf
損失関数を表します。
次のように仮定します。

その派生語:
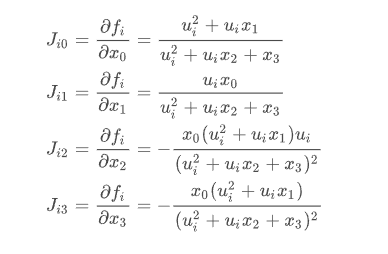
制約:

from scipy.optimize import least_squares
def model(x, u):
return x[0] * (u ** 2 + x[1] * u) / (u ** 2 + x[2] * u + x[3])
def fun(x, u, y):
return model(x, u) - y
def jac(x, u, y):
J = np.empty((u.size, x.size))
den = u ** 2 + x[2] * u + x[3]
num = u ** 2 + x[1] * u
J[:, 0] = num / den
J[:, 1] = x[0] * u / den
J[:, 2] = -x[0] * num * u / den ** 2
J[:, 3] = -x[0] * num / den ** 2
return J
u = np.array([4.0, 2.0, 1.0, 5.0e-1, 2.5e-1, 1.67e-1, 1.25e-1, 1.0e-1,
8.33e-2, 7.14e-2, 6.25e-2])
y = np.array([1.957e-1, 1.947e-1, 1.735e-1, 1.6e-1, 8.44e-2, 6.27e-2,
4.56e-2, 3.42e-2, 3.23e-2, 2.35e-2, 2.46e-2])
x0 = np.array([2.5, 3.9, 4.15, 3.9])
res = least_squares(fun, x0, jac=jac, bounds=(0, 100), args=(u, y), verbose=1)
# may vary
`ftol` termination condition is satisfied.
Function evaluations 130, initial cost 4.4383e+00, final cost 1.5375e-04, first-order optimality 4.92e-08.
res.x
# array([ 0.19280596, 0.19130423, 0.12306063, 0.13607247])
import matplotlib.pyplot as plt
u_test = np.linspace(0, 5)
y_test = model(res.x, u_test)
plt.plot(u, y, 'o', markersize=4, label='data')
plt.plot(u_test, y_test, label='fitted model')
plt.xlabel("u")
plt.ylabel("y")
plt.legend(loc='lower right')
plt.show()
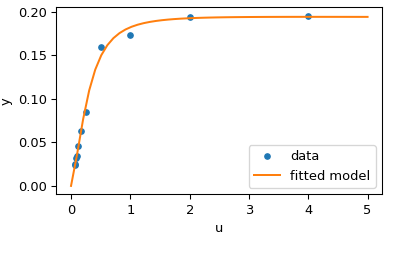
4 一変数関数ミニマイザー
from scipy.optimize import minimize_scalar
f = lambda x: (x - 2) * (x + 1)**2
res = minimize_scalar(f, method='brent')
print(res.x)
# 1.0
5 有界最小化
from scipy.special import j1
res = minimize_scalar(j1, bounds=(4, 7), method='bounded')
res.x
# 5.33144184241
6 カスタムミニマイザー
from scipy.optimize import OptimizeResult
def custmin(fun, x0, args=(), maxfev=None, stepsize=0.1,
maxiter=100, callback=None, **options):
bestx = x0
besty = fun(x0)
funcalls = 1
niter = 0
improved = True
stop = False
while improved and not stop and niter < maxiter:
improved = False
niter += 1
for dim in range(np.size(x0)):
for s in [bestx[dim] - stepsize, bestx[dim] + stepsize]:
testx = np.copy(bestx)
testx[dim] = s
testy = fun(testx, *args)
funcalls += 1
if testy < besty:
besty = testy
bestx = testx
improved = True
if callback is not None:
callback(bestx)
if maxfev is not None and funcalls >= maxfev:
stop = True
break
return OptimizeResult(fun=besty, x=bestx, nit=niter,
nfev=funcalls, success=(niter > 1))
x0 = [1.35, 0.9, 0.8, 1.1, 1.2]
res = minimize(rosen, x0, method=custmin, options=dict(stepsize=0.05))
res.x
# array([1., 1., 1., 1., 1.])
7 ルートを見つける
- 単変量
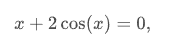
from scipy.optimize import root
def func(x):
return x + 2 * np.cos(x)
sol = root(func, 0.3)
sol.x
# array([-1.02986653])
sol.fun
# array([ -6.66133815e-16])
- 多変量
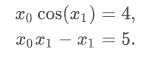
def func2(x):
f = [x[0] * np.cos(x[1]) - 4,
x[1]*x[0] - x[1] - 5]
df = np.array([[np.cos(x[1]), -x[0] * np.sin(x[1])],
[x[1], x[0] - 1]])
return f, df
sol = root(func2, [1, 1], jac=True, method='lm')
sol.x
# array([ 6.50409711, 0.90841421])
8 線形計画法
質問:
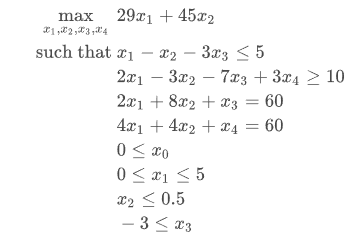
上記は最大サイズであり、ソルバーを使用する前に最小サイズに変換する必要がありますlinprog
。

定義 x:
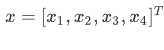
この場合、目的関数の係数は次のようになります。
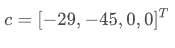
2 つの不等式制約を考えてみましょう。
1 つ目は「未満」不等式なので、すでに linprog が受け入れる形式になっています。2 つ目は「より大きい」不等式なので、「より小さい」不等式に変換するには、両辺に -1 を掛ける必要があります。
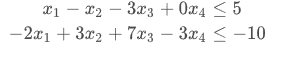
行列形式に擬似変換:
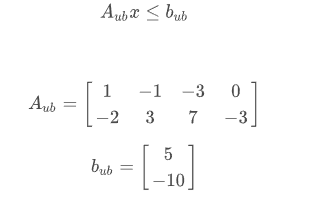
2 つの方程式を考えてみましょう。
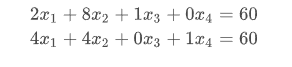
マトリックス形式:
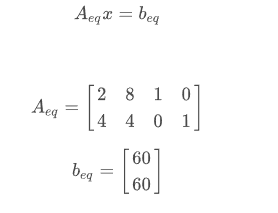
from scipy.optimize import linprog
c = np.array([-29.0, -45.0, 0.0, 0.0])
A_ub = np.array([[1.0, -1.0, -3.0, 0.0],
[-2.0, 3.0, 7.0, -3.0]])
b_ub = np.array([5.0, -10.0])
A_eq = np.array([[2.0, 8.0, 1.0, 0.0],
[4.0, 4.0, 0.0, 1.0]])
b_eq = np.array([60.0, 60.0])
x0_bounds = (0, None)
x1_bounds = (0, 5.0)
x2_bounds = (-np.inf, 0.5) # +/- np.inf can be used instead of None
x3_bounds = (-3.0, None)
bounds = [x0_bounds, x1_bounds, x2_bounds, x3_bounds]
result = linprog(c, A_ub=A_ub, b_ub=b_ub, A_eq=A_eq, b_eq=b_eq, bounds=bounds)
print(result)
con: array([15.5361242 , 16.61288005]) # may vary
fun: -370.2321976308326 # may vary
message: 'The algorithm terminated successfully and determined that the problem is infeasible.'
nit: 6 # may vary
slack: array([ 0.79314989, -1.76308532]) # may vary
status: 2
success: False
x: array([ 6.60059391, 3.97366609, -0.52664076, 1.09007993]) # may vary
この問題は実行不可能であることが判明しました。これは、すべての制約を満たす解ベクトルが存在しないことを意味します。
これは必ずしも何かが間違って行われたことを意味するわけではありません。いくつかの問題は実際には機能しません。
制約が厳しすぎるので緩和できるとします。コード x1_bounds =(0,6) を調整します。
x1_bounds = (0, 6)
bounds = [x0_bounds, x1_bounds, x2_bounds, x3_bounds]
result = linprog(c, A_ub=A_ub, b_ub=b_ub, A_eq=A_eq, b_eq=b_eq, bounds=bounds)
print(result)
con: array([9.78840831e-09, 1.04662945e-08]) # may vary
fun: -505.97435889013434 # may vary
message: 'Optimization terminated successfully.'
nit: 4 # may vary
slack: array([ 6.52747190e-10, -2.26730279e-09]) # may vary
status: 0
success: True
x: array([ 9.41025641, 5.17948718, -0.25641026, 1.64102564]) # may vary
x = np.array(result.x)
print(c @ x)
# -505.97435889013434 # may vary
9 課題の問題
水泳メドレーリレーチームの生徒選抜を考えてみましょう。5 人の生徒の各ストロークのタイムをリストした表があります。
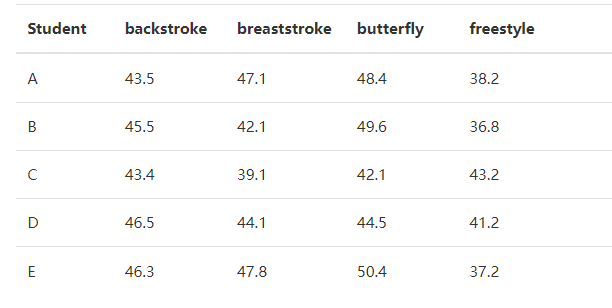
解決策: 各行の 1 つの列のみが値を持つコスト行列 C を定義します。行列の合計が最終コストになります。
目的関数を定義します。
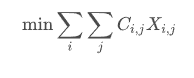
X は 2 値行列で、行 i を列 j に割り当てると、X ij = 1 X_{ij}=1バツ私は=1。
cost = np.array([[43.5, 45.5, 43.4, 46.5, 46.3],
[47.1, 42.1, 39.1, 44.1, 47.8],
[48.4, 49.6, 42.1, 44.5, 50.4],
[38.2, 36.8, 43.2, 41.2, 37.2]])
from scipy.optimize import linear_sum_assignment
row_ind, col_ind = linear_sum_assignment(cost) # 行索引,列索引
row_ind
# array([0, 1, 2, 3])
col_ind
# array([0, 2, 3, 1])
# 最优分配
styles = np.array(["backstroke", "breaststroke", "butterfly", "freestyle"])[row_ind]
students = np.array(["A", "B", "C", "D", "E"])[col_ind]
dict(zip(styles, students))
{
'backstroke': 'A', 'breaststroke': 'C', 'butterfly': 'D', 'freestyle': 'B'}
# 最少时间
cost[row_ind, col_ind].sum()
# 163.89999999999998
参考:
https://blog.csdn.net/xu624735206/article/details/117320847
https://blog.csdn.net/jiang425776024/article/details/87885969
https://docs.scipy.org/doc/scipy/tutorial/optimize.html