Google, Alibaba, Microsoft and other large deep learning CTR 10 model the evolution of the most complete map [recommended, advertising, search Field
Here is the "Wang Zhe machine learning notes" thirteenth article, and today we look back together at all major deep learning CTR model the past three years, but also summarize the knowledge of my spare time, I hope to help you sort out the recommendation system, progress in the field of cutting-edge advertising depth learning calculations.
With Microsoft's Deep Crossing, Google's Wide & Deep, and FNN, PNN large number of outstanding depth learning CTR forecast models have been proposed in 2016, the field of computing advertising and recommendation system fully into the era of deep learning, to date, depth CTR learning model has become the mainstream advertising and recommend areas beyond doubt. In the previous column, " before the era of deep learning CTR estimate road evolution models ," we discuss with you the relationship between the structural characteristics and evolution of the traditional model of CTR together. After entering the era of deep learning, CTR model not only in the ability to express, a qualitative improvement on the model results, and draws heavily on deep learning and integration of image, voice, natural language processing and the outcome of direction, were quickly on the model structure evolution.
This article summarizes the advertising, the most popular areas of the recommended 10 depth study of the structural characteristics of CTR model was constructed evolutionary patterns between them. Select the standard model try to follow the following three principles:
- In the industry influence of large models;
- It has been Google, Microsoft, Alibaba and other well-known Internet companies successful application;
- Project-oriented, rather than only experimental data validation or use of academic innovation.
Below are listed first map of this evolution CTR depth learning model, and then be introduced one by one:
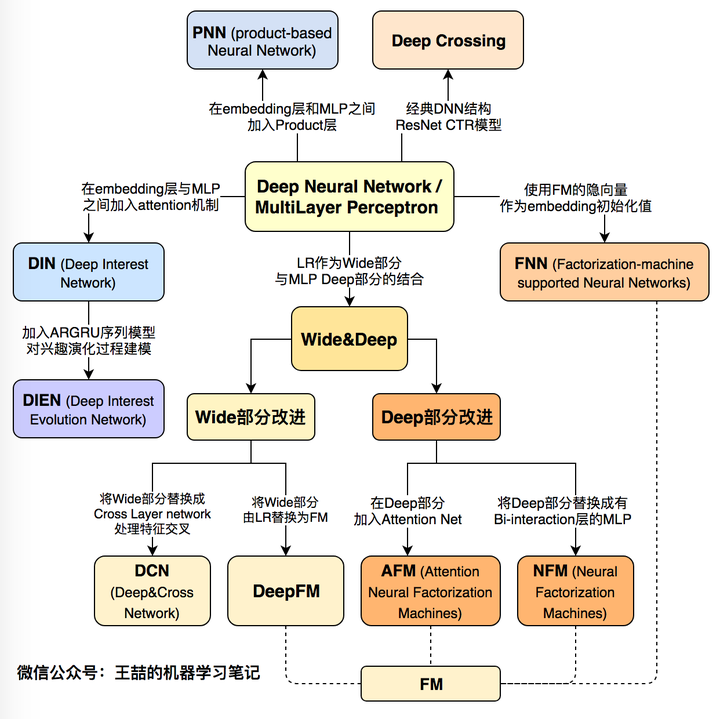
First, Microsoft Deep Crossing (2016) - the depth of learning CTR model base model
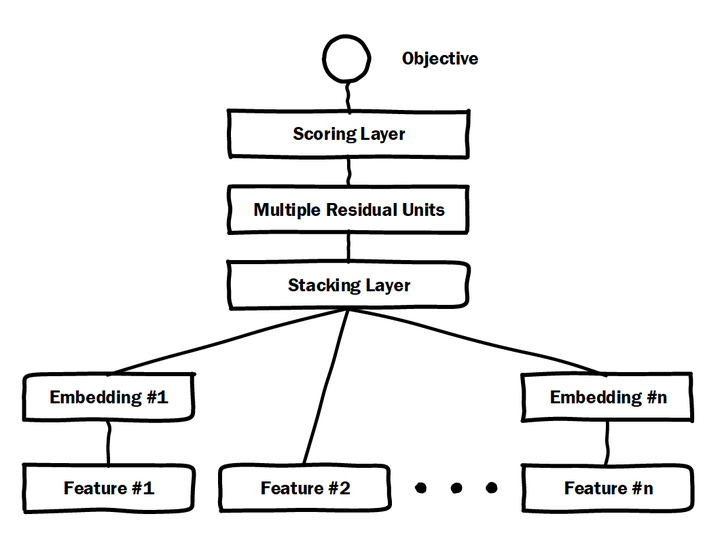
Microsoft made in 2016 Deep Crossing arguably the most typical and basic model of deep learning CTR model. FIG model structure shown in FIG. 2, it covers most typical depth CTR feature model, i.e. by the addition of embedding layer into low-dimensional feature sparse dense features, by stacking layer, called a feature vector concat layer or the segmented connected, and then complete the multilayer neural network characterized by the combination of the conversion, the final completion CTR calculated by scoring layer. With classic DNN different is, multilayer perceptron Deep crossing used by the network of residuals, which will undoubtedly benefit from the famous 152 layer ResNet MSRA famous researcher Ho Kai Ming raised.
Two, FNN (2016) - the complete Embedding implicit initialization vector of FM
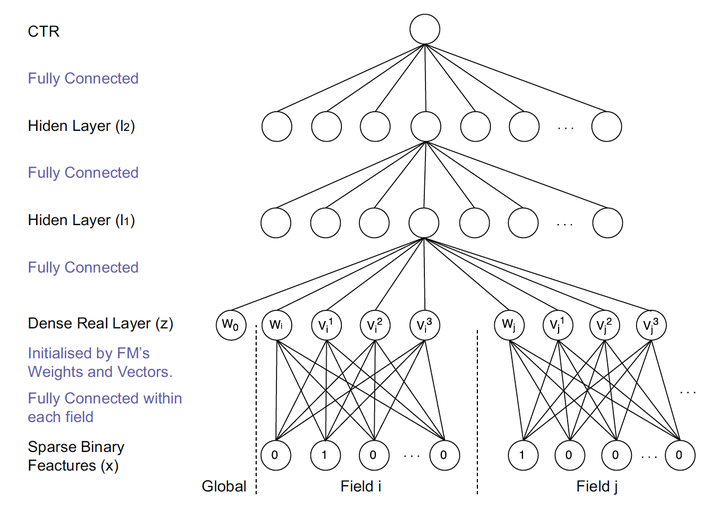
FNN compared Deep Crossing innovation is the use of hidden layer as a vector FM and Embedding User item, thus completely avoiding the state from a random training Embedding. Since the class id feature extensive use of one-hot encoding, lead to great dimensions, very sparse vector, the connection layer and the input layer Embedding very much, gradient descent low efficiency, which greatly increases the training time and the model Embedding instability, the use of pre train way to complete training Embedding layer, reducing the depth of the learning model is undoubtedly the complexity and instability of the effective training of engineering experience.
论文:[FNN] Deep Learning over Multi-field Categorical Data (UCL 2016)
Three, PNN (2016) - the feature-rich cross the
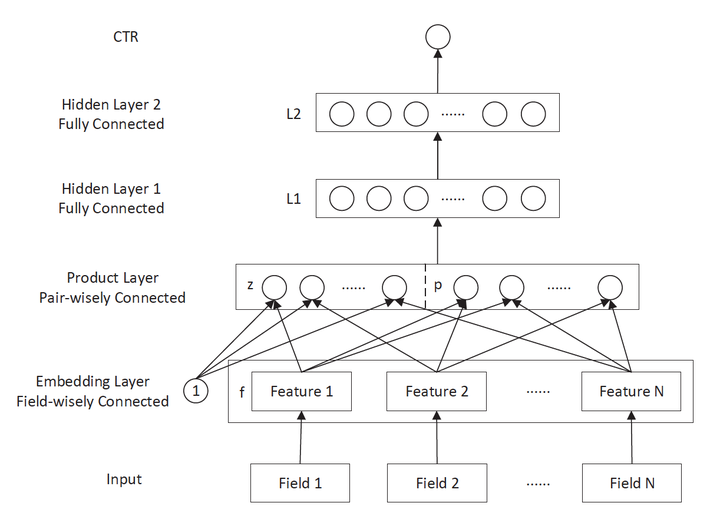
PNN stands Product-based Neural Network, PNN key embedding layer lies between the connection layer and the whole joined Product layer. Traditional DNN direct connection layer to complete the full features and combinations of multilayer cross, but a certain lack of "targeted" in such a manner. First connection layer and not the whole cross domains for different characteristics; secondly, the whole operation of the connection layer is also not designed for direct wherein the cross. But in practical problems, the importance of cross features self-evident, such as age and gender of the cross is a very important grouping features, including a large number of high-value information, we are in urgent need of deep learning network to be able to characterize the targeted structure these messages. Product layer PNN thus completed by the addition of a cross-targeted features, combinations of features which product operations between different features domains. And defines a plurality of different product of inner product, outer product operation capturing other cross information, enhance the ability of the model to characterize different data patterns.
论文:[PNN] Product-based Neural Networks for User Response Prediction (SJTU 2016)
Four, Google Wide & Deep (2016) - the memory capacity and generalization ability tradeoff
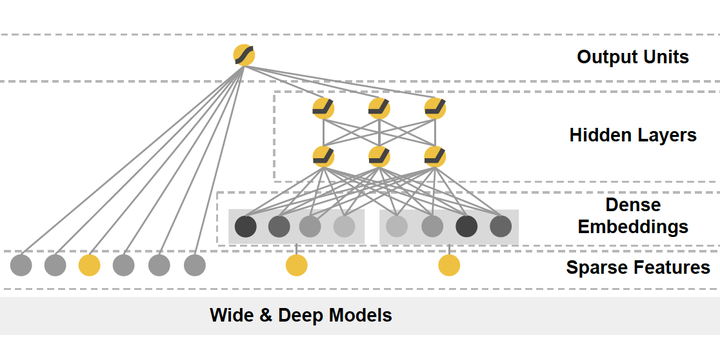
The main idea of Google Wide & Deep model as its name, the Wide partially single input layer and partially through the multilayer perceptron Deep connected, the input layer together with the final output. Wide portions of which the main role is to model memory (Memorization), Wide partial monolayer adept at handling a large number of sparse id class features, easy to make direct model "remember" a lot of historical information of users; main role is part of the Deep let model "generalized" (the generalization), using a strong DNN expression characteristics, features hidden behind the mining data pattern. LR using the final output layer portion and be combined Wide Deep portion to form a unified model. After Wide & Deep affecting model that - the depth of a large number of learning models even using a two-part multi-part composition, the use of different network structures different mining combining information, and combines the full advantage of the characteristics of different network structures.
论文:[Wide&Deep] Wide & Deep Learning for Recommender Systems (Google 2016)
Fifth, Huawei DeepFM (2017 years) - Wide section instead of using FM
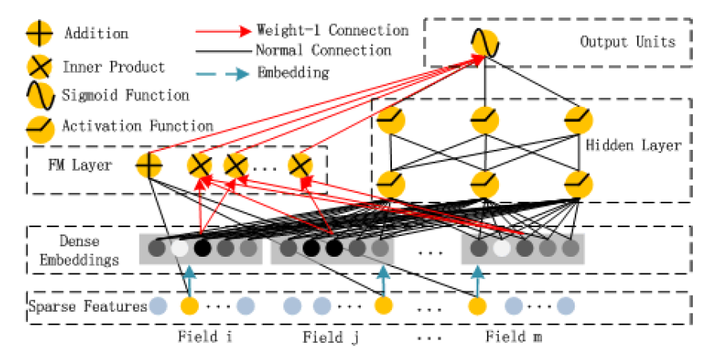
After Wide & Deep, many dual network model of continuation of the combined structure, DeepFM is one of them. DeepFM Wide & Deep of improvement that is, it was replaced with the original FM off Wide section enhance the ability of the network portion of the shallow combinations of features. In fact, since the FM is itself part of the first-order and second-order part of, DeepFM equivalent composition while part of the original Wide + + Deep crossing portion of second order wherein three structural part, will undoubtedly further enhance the expressive power of the model.
论文:[DeepFM] A Factorization-Machine based Neural Network for CTR Prediction (HIT-Huawei 2017)
Six, Google Deep & Cross (2017) - the network instead of using the Wide Cross section
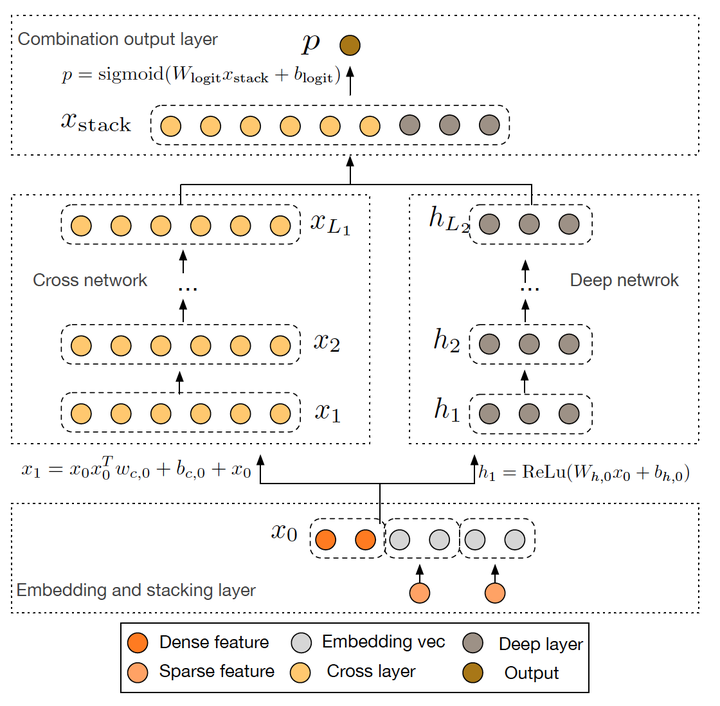
Google 2017 published the Deep & Cross Network (DCN) is also a further improvement Wide & Deep, the main idea of using Cross network to replace the original Wide part. Wherein the basic motivation Cross network design is to increase the strength of the interaction between features, multilayer cross layer on an input vector wherein crossover. The basic operation is the single cross layer input vectors xl cross layer cross with the original input vector x0, and the added bias vector and the original input vectors xl. DCN on the nature of the problem or part of the shortage of skills Wide & Deep Wide improved, and ideas DeepFM very similar.
论文:[DCN] Deep & Cross Network for Ad Click Predictions (Stanford 2017)
Seven, NFM (2017) - the part of the improvement of the Deep
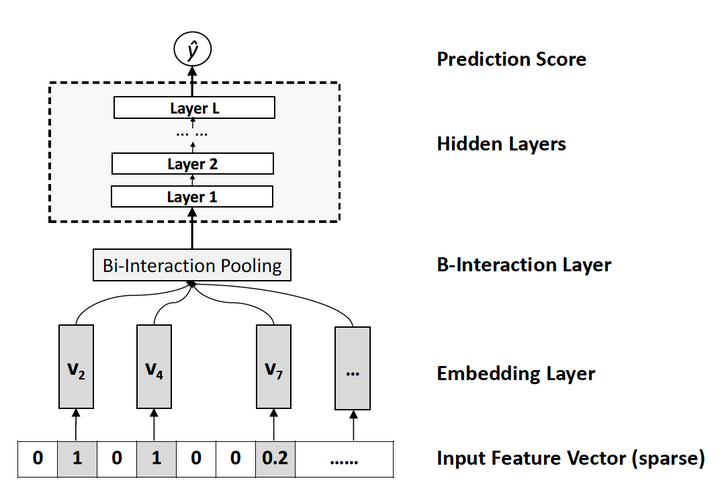
And with respect to DeepFM DCN for improving Wide & Deep Wide portion, NFM can be seen as an improvement over the Deep portion. NFM stands for Neural Factorization Machines, if we look at FM in terms of depth learning network architecture, FM can also be seen as crossing a single layer LR and second-order features consisting Wide & Deep architecture and classic W & differs from D only in Deep hidden part becomes a second order vector multiplied form. Still further, the NFM FM from the modified second order partial perspective, with a band Bi-interaction DNN Pooling layer replaces the characteristic FM intersection, forming a unique Wide & Deep architecture. Wherein Bi-interaction Pooling can be seen in the form of different features of embedding the element-wise product. This is also the NFM compared to Google Wide & Deep innovations.
论文:[NFM] Neural Factorization Machines for Sparse Predictive Analytics (NUS 2017)
Eight, AFM (2017) - the introduction of FM Attention Mechanism
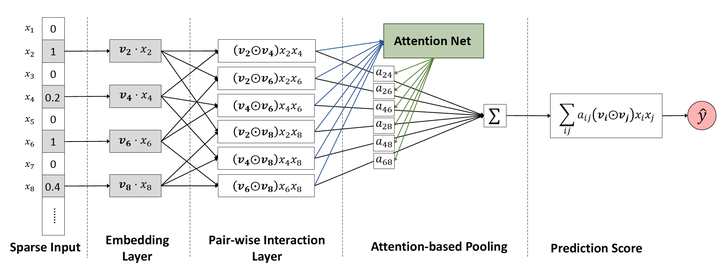
AFM stands Attentional Factorization Machines, by previous presentation we clearly know, FM is actually a classic Wide & Deep structure in which Wide part is a first-order part of the FM, Deep part of the second-order part of the FM, while the AFM by definition, the introduction of FM Attention mechanisms, specific to the model of the structure, AFM is actually a feature of each cross section of the second-order FM is given a weight, the weight control the impact of cross features on the final outcome, it is very similar to the field of NLP attentional mechanisms (attention mechanism). Attention to weight training, the AFM Attention Net added, using the well trained Attention Attention Net weights, then reverse acting cross-over feature FM second order, characterized in that the FM gain the ability to adjust the weight according to the characteristics of the sample.
Nine, Ali DIN (2018 years) - Attention mechanism Ali added depth learning network
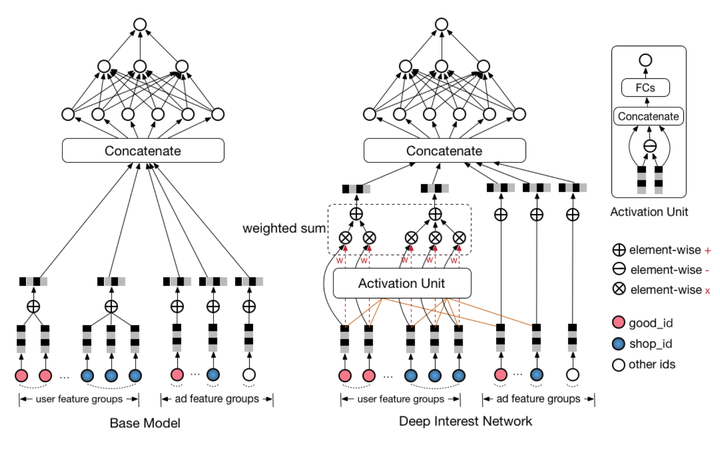
AFM joined the Attention mechanism FM in 2018, Alibaba formally proposed mechanism combines Attention depth learning model --Deep Interest Network. With AFM The Attention and FM binding is different, DIN the Attention mechanism of action than the depth neural network, between embedding layer and concatenate layer model joined attention unit, so that the model can be based on different candidate commodity, the right to adjust different characteristics of the weight .
论文:[DIN] Deep Interest Network for Click-Through Rate Prediction (Alibaba 2018)
Ten, Ali DIEN (2018 Nian) - DIN "evolution"
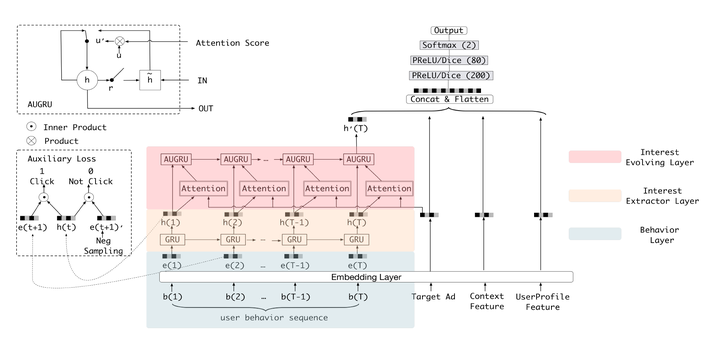
DIEN is called the Deep Interest Evolution Network, it is not only a DIN further "evolution" is more important is DIEN by introducing a series model AUGRU simulate the process of evolution of user interest. Specifically, the main features of the model is between Embedding layer and Concatenate layer joined Interest Evolving layer Interest Extractor Layer and simulate the evolution of the interest generated interest. Wherein Interest Extractor Layer structure using DIN extracted in each time slice of the user's interest, Interest Evolving layer structure using the series model will AUGRU concatenated user interest at different times, forming a chain of interest evolution. And then finally "interest vector" current time input upper multilayer fully connected network, the CTR final estimate together with other features.
论文:[DIEN] Deep Interest Evolution Network for Click-Through Rate Prediction (Alibaba 2019)
Summary - depth learning era CTR model
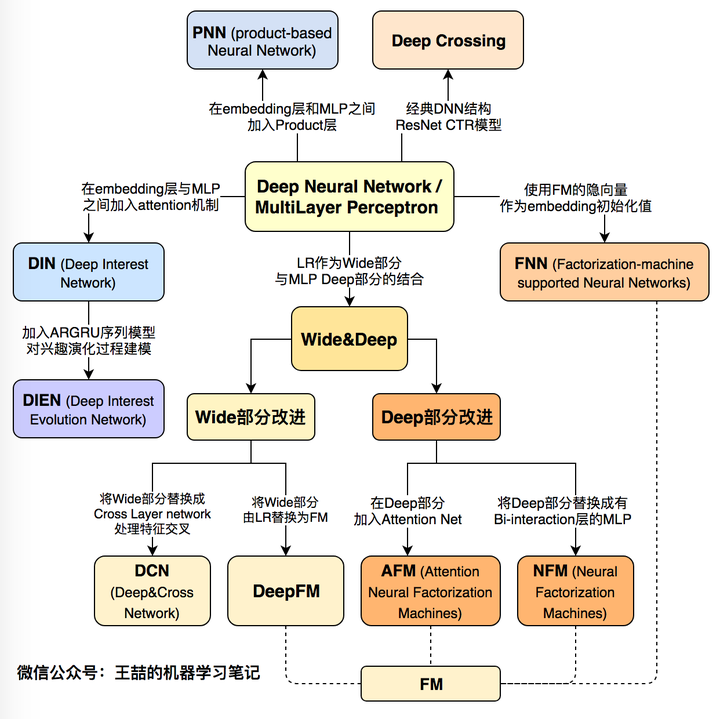
Finally, I once again emphasize the depth of this article to learn CTR model evolution chart, it is no exaggeration to say that this figure includes the evolution of the structural characteristics of all major deep learning CTR models in recent years, and the evolution of the relationship between them. We are hoping to help the recommendation algorithm advertising, search field engineers to establish a complete system of knowledge, very much at home can apply for business characteristics and compare the effects of different models, which use the most current data for model-driven business model.
Combined with his experience, learning about the depth model I would like to share two elements:
- 没有银弹。从来没有一个深度学习模型能够在所有数据集上都表现最优,特别是推荐、广告领域,各家的数据集,数据pattern、业务领域差异巨大,不存在能够解决一切问题的“银弹”模型。比如,阿里的DIEN对于数据质量、用户整个life cycle行为完整性的要求很高,如果在某些DSP场景下运用这个模型,预计不会收到良好的效果。再比如Google 的Deep&Cross,我们也要考虑自己的数据集需不需要如此复杂的特征交叉方式,在一些百万量级的数据集上,也许浅层神经网络的表现更好。
- 算法工程师永远要在理想和现实间做trade off。有一种思想要避免,就是我为了要上某个模型就要强转团队的技术栈,强买某些硬件设备。模型的更新过程一定是迭代的,一定是从简单到复杂的,一定是你证明了某个模型是work的,然后在此基础上做改进的。这也是我们要熟悉所有模型演化关系的原因。
就在我们熟悉这些已有模型的时候,深度学习CTR模型的发展从没有停下它的脚步。从阿里的多模态、多目标的深度学习模型,到YouTube基于RNN等序列模型的推荐系统,再到Airbnb使用Embedding技术构建的搜索推荐模型,深度学习的应用不仅越来越广泛,而且得到了越来越快的进化。在今后的专栏文章中,我们不仅会更深入的介绍深度学习CTR模型的知识,而且会更加关注深度学习CTR模型的应用与落地,期待与大家一同学习。
这里是「王喆的机器学习笔记」,因为文章的内容较多,水平有限,希望大家能够踊跃指出文章中的错误,先多谢大家!
最后欢迎大家关注我的 微信公众号:王喆的机器学习笔记(wangzhenotes),跟踪计算广告、推荐系统等机器学习领域前沿。想进一步交流的同学也可以通过公众号加我的微信一同探讨技术问题,谢谢!