Image segmentation is a significant area of the image into the process. Foreground image region may be a single object or the background. These regions can be constructed using the feature, such as color, edges, or the like neighbor similarity.
Code a
The following presents a smaller one with a calculation of the python-graph kit maximum flow / minimum cut a simple example:
from pygraph.classes.digraph import digraph
from pygraph.algorithms.minmax import maximum_flow
gr = digraph()
gr.add_nodes([0,1,2,3])
gr.add_edge((0,1), wt=4)
gr.add_edge((1,2), wt=3)
gr.add_edge((2,3), wt=5)
gr.add_edge((0,2), wt=3)
gr.add_edge((1,3), wt=4)
flows,cuts = maximum_flow(gr, 0, 3)
print ('flow is:' , flows)
print ('cut is:' , cuts)
Print and cut out the flow results:
The above two flows through the python dictionary contains a flag for each node and each edge: comprising a part of FIG 0 are the source point and the sink node 1 is connected to
Code two
The following example reads an image from the two estimated probability matrix type region of the image, and then create a graph:
# -*- coding: utf-8 -*-
from scipy.misc import imresize
from PCV.tools import graphcut
from PIL import Image
from numpy import *
from pylab import *
im = array(Image.open("empire.jpg"))
im = imresize(im, 0.07)
size = im.shape[:2]
print ("OK!!")
# add two rectangular training regions
labels = zeros(size)
labels[3:18, 3:18] = -1
labels[-18:-3, -18:-3] = 1
print ("OK!!")
# create graph
g = graphcut.build_bayes_graph(im, labels, kappa=1)
# cut the graph
res = graphcut.cut_graph(g, size)
print ("OK!!")
figure()
graphcut.show_labeling(im, labels)
figure()
imshow(res)
gray()
axis('off')
show()
The following results for the training of the image area and the coverage area and displays a result of image segmentation
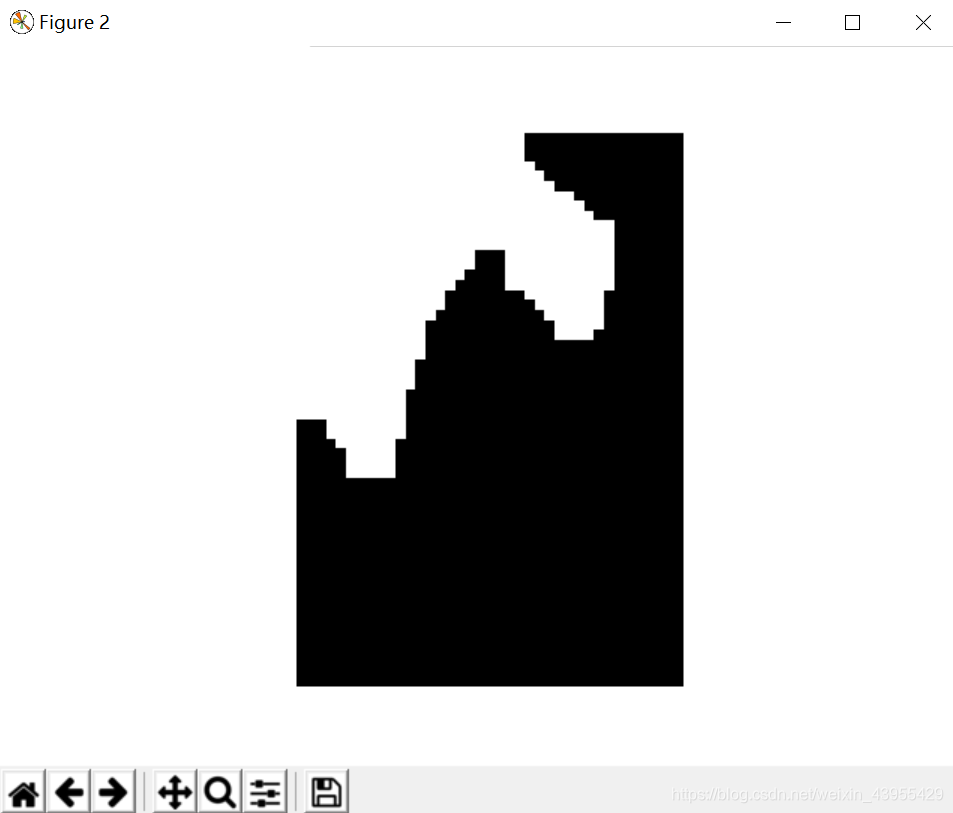
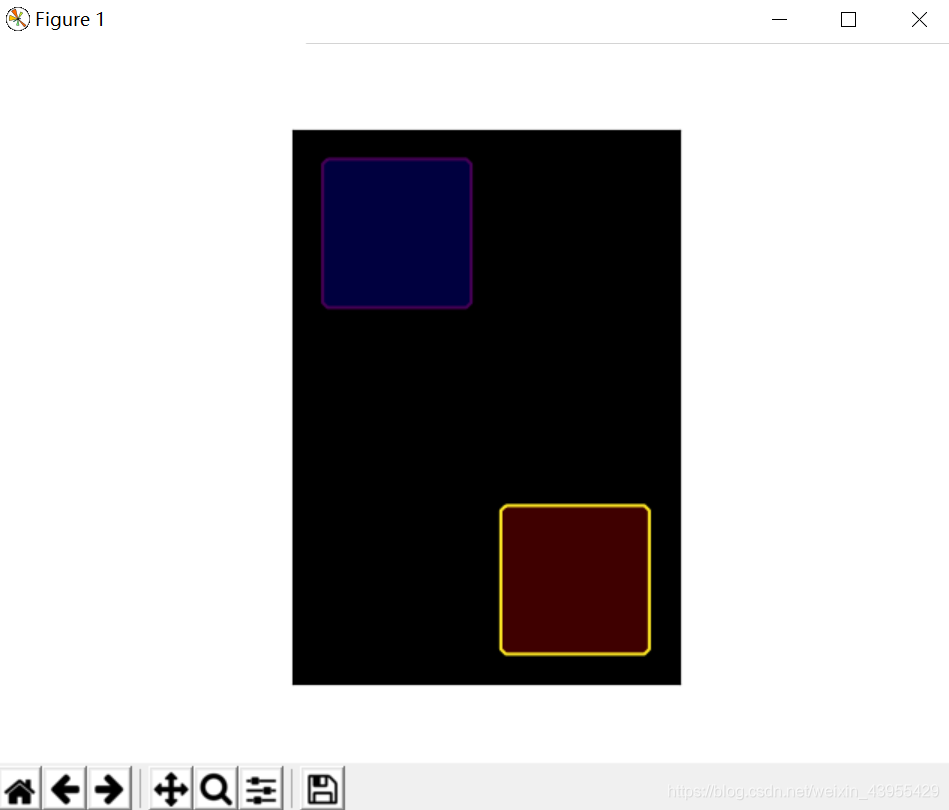