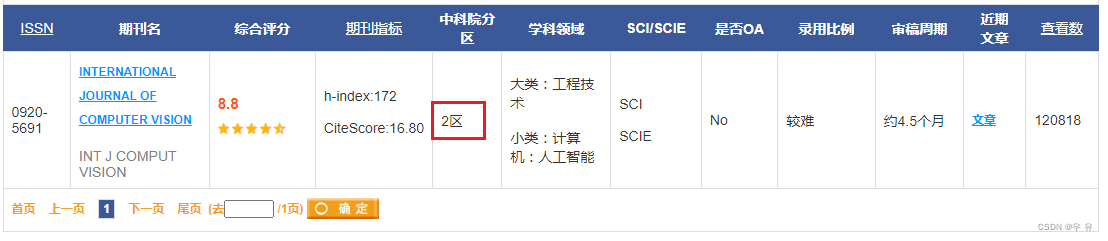
Abstract
Related Work
For deep learning based facial landmark detection, there are two widely used detection heads, namely heatmap regression and coordinate regression. Heatmap regression can achieve good results, but it has two drawbacks: (1) it is computationally expensive; (2) it is sensitive to outliers (see Figure 5(b)). In contrast, coordinate regression is fast and robust, but not accurate enough (see Figure 5(a)). Although coordinate regression can be used in a multi-stage manner to yield better performance, its inference speed becomes slow as a result.
Based on DL facial point detection method×2: heat map regression, coordinate regression
Heatmap: √ good results × large amount of calculation, sensitive to outliers
coordinate: √ Fast and robust × Insufficient accuracy (although multi-stage improves performance, it will reduce speed)
⇒The purpose is to combine the advantages of both (the first study in this area that discusses the connection between heatmap and coordinate regression.)
Coordinate Regression Models
Heatmap Regression Models
Cross-Domain Generalization
Semi-Supervised Facial Landmark Detection
Method
PIP regression、
neighbor regression module、
self-training with curriculum framework、
implicit prior we observe from CNN-based facial landmark detectors
Knowledge points + vocabulary
Generalization capability across domains Generalization capability across domains
domain gaps
Stacked hourglass networksStacked hourglass networks
hybrids of classification and regression
leverage
Related Links
- Pixel-in-Pixel Net: Towards Efficient Facial Landmark Detection in the Wild - 知乎 (zhihu.com)
- PIPNet: Face key point detection for natural scenes "Pixel-in-Pixel Net: Towards Efficient Facial Landmark Detection in the Wild"_Shaojiwan's Blog-CSDN Blog
- Understand the Heatmap and Regression methods of key point positioning from another angle - Zhihu (zhihu.com)