The most basic and initial step of NLP analysis is keyword extraction, in NLP we have many algorithms that help us extract keywords from text data. In this article, Mr. Yunduo will learn four simple and effective methods with you, which are Rake, Yake, Keybert and Textrank. It will briefly outline the usage scenarios of each method, and then apply it to extracting keywords with additional examples.
Keywords in this article : keyword extraction, key phrase extraction, Python, NLP, TextRank, Rake, BERT
In my previous article, I introduced the use of Python and TFIDF to extract keywords from text . The TFIDF method relies on corpus statistics to weight the extracted keywords, so one of its disadvantages is that it cannot be applied to a single text.
In order to illustrate the implementation principle of each keyword extraction method (Rake, Yake, Keybert, and Textrank) , the abstract of the published article [1] and the keywords specified by the topic will be used , and by examining which methods' extracted keywords are consistent with The keywords set by the authors are closer to examine each method. In the keyword extraction task, there are explicit keywords, which appear explicitly in the text; there are also implicit keywords, that is, the keywords mentioned by the author do not appear explicitly in the text, but are related to the field of the article relevant.
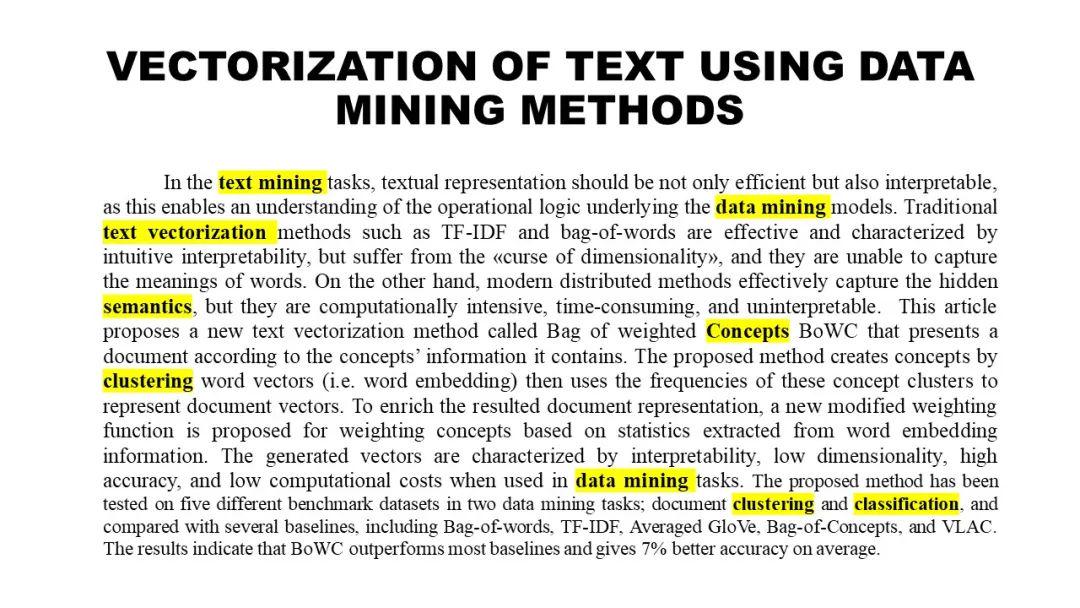
In the example shown above, with the text title and article abstract, the standard keywords (defined by the author in the original article) are marked in yellow. Note that the term machine learning is ambiguous and not found in the abstract. Although it can be extracted from the full text of the article, here for simplicity, the corpus data is limited to the abstract.
text preparation
Headings are often combined with the provided text because headings contain valuable information and provide a high-level overview of what the article is about. Therefore, we simply concatenate the two variables text and title by adding a plus sign.
title = "VECTORIZATION OF TEXT USING DATA MINING METHODS"
text = "In the text mining tasks, textual representation should be not only efficient but also interpretable, as this enables an understanding of the operational logic underlying the data mining models. Traditional text vectorization methods such as TF-IDF and bag-of-words are effective and characterized by intuitive interpretability, but suffer from the «curse of dimensionality», and they are unable to capture the meanings of words. On the other hand, modern distributed methods effectively capture the hidden semantics, but they are computationally intensive, time-consuming, and uninterpretable. This article proposes a new text vectorization method called Bag of weighted Concepts BoWC that presents a document according to the concepts’ information it contains. The proposed method creates concepts by clustering word vectors (i.e. word embedding) then uses the frequencies of these concept clusters to represent document vectors. To enrich the resulted document representation, a new modified weighting function is proposed for weighting concepts based on statistics extracted from word embedding information. The generated vectors are characterized by interpretability, low dimensionality, high accuracy, and low computational costs when used in data mining tasks. The proposed method has been tested on five different benchmark datasets in two data mining tasks; document clustering and classification, and compared with several baselines, including Bag-of-words, TF-IDF, Averaged GloVe, Bag-of-Concepts, and VLAC. The results indicate that BoWC outperforms most baselines and gives 7% better accuracy on average"
full_text = title +", "+ text
print("The whole text to be usedn", full_text)
Now start using today's four protagonists to extract keywords!
His
It is a lightweight, unsupervised automatic keyword extraction method that relies on statistical text features extracted from individual documents to identify the most relevant keywords in the text. The method does not need to be trained on a specific set of documents, nor does it depend on dictionaries, text sizes, domains, or languages. Yake defines a set of five features to capture keyword characteristics, which are combined heuristically to assign a score to each keyword. The lower the score, the more important the keyword. You can read the original paper [2] , and yake's Python package [3] about it.
Feature extraction mainly considers five factors (after removing stop words)
uppercase term
(Casing)
Capitalized terms (except the beginning word of each sentence) are more important than those in lowercase.
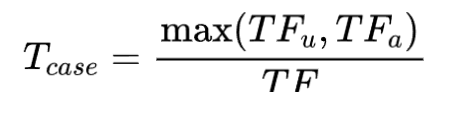
Among them, represents the capitalization times of the word, and represents the abbreviation times of the word.
word position
(Word Position)
The importance of the sentence at the beginning of the text is greater than that of the sentence at the back.

where is the median position in the document of all sentences containing the word.
word frequency
(Term Frequency)
The more frequently a word appears in the text, the more important it is relatively speaking. At the same time, in order to avoid the problem of higher frequency of long text words, a normalization operation will be performed.
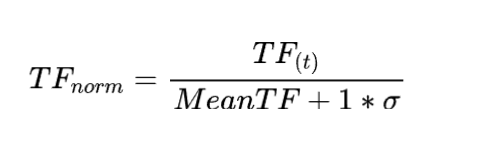
Among them, MeanTF is the average word frequency of the whole word, and is the standard deviation.
context
(Term Related to Context)
The more different words a word co-occurs with, the less important that word is.
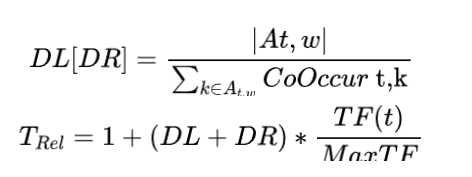
Among them, it means that the window size is sliding from the left, and means sliding from the right. Indicates the number of different words appearing under the fixed window size. Indicates the maximum value of all word frequencies.
How often the word appears in the sentence
(Term Different Sentence)
The more a word appears in a sentence, the more important it is
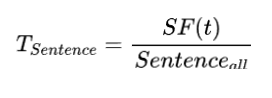
Where SF(t) is the frequency of sentences containing the word t tt, and represents the number of all sentences.
最后计算每个term的分值公式如下:
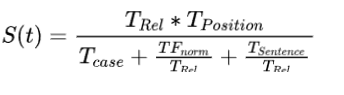
S(t)表示的是单词t 的分值情况,其中 s(t)分值越小,表示的单词 t越重要。
安装和使用
pip install git+https://github.com/LIAAD/yake
import yake
首先从 Yake 实例中调用 KeywordExtractor 构造函数,它接受多个参数,其中重要的是:要检索的单词数top,此处设置为 10。参数 lan:此处使用默认值en。可以传递停用词列表给参数 stopwords。然后将文本传递给 extract_keywords 函数,该函数将返回一个元组列表 (keyword: score)。关键字的长度范围为 1 到 3。
kw_extractor = yake.KeywordExtractor(top=10, stopwords=None)
keywords = kw_extractor.extract_keywords(full_text)
for kw, v in keywords:
print("Keyphrase: ",kw, ": score", v)
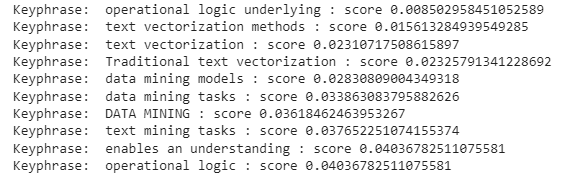
从结果看有三个关键词与作者提供的词相同,分别是text mining, data mining 和 text vectorization methods。注意到Yake会区分大写字母,并对以大写字母开头的单词赋予更大的权重。
Rake
Rake 是 Rapid Automatic Keyword Extraction 的缩写,它是一种从单个文档中提取关键字的方法。实际上提取的是关键的短语(phrase),并且倾向于较长的短语,在英文中,关键词通常包括多个单词,但很少包含标点符号和停用词,例如and,the,of等,以及其他不包含语义信息的单词。
Rake算法首先使用标点符号(如半角的句号、问号、感叹号、逗号等)将一篇文档分成若干分句,然后对于每一个分句,使用停用词作为分隔符将分句分为若干短语,这些短语作为最终提取出的关键词的候选词。
每个短语可以再通过空格分为若干个单词,可以通过给每个单词赋予一个得分,通过累加得到每个短语的得分。Rake 通过分析单词的出现及其与文本中其他单词的兼容性(共现)来识别文本中的关键短语。最终定义的公式是:

即单词 的得分是该单词的度(是一个网络中的概念,每与一个单词共现在一个短语中,度就加1,考虑该单词本身)除以该单词的词频(该单词在该文档中出现的总次数)。
然后对于每个候选的关键短语,将其中每个单词的得分累加,并进行排序,RAKE将候选短语总数的前三分之一的认为是抽取出的关键词。
安装和使用
# $ git clone https://github.com/zelandiya/RAKE-tutorial
# 要在python代码中导入rake:
import rake
import operator
# 加载文本并对其应用rake:
filepath = "keyword_extraction.txt"
rake_object = rake.Rake(filepath)
text = "Compatibility of systems of linear constraints over the set of natural numbers. Criteria of compatibility of a system of linear Diophantine equations, strict inequations, and nonstrict inequations are considered.Upper bounds for components of a minimal set of solutions and algorithms of construction of minimal generatingsets of solutions for all types of systems are given. These criteria and the corresponding algorithms for constructing a minimal supporting set of solutions can be used in solving all the considered types of systems and systems of mixed types."
sample_file = open(“data/docs/fao_test/w2167e.txt”, ‘r’)
text = sample_file.read()
keywords = rake_object.run(text) print “Keywords:”, keywords
候选关键字
如上所述,我们知道RAKE通过使用停用词和短语分隔符解析文档,将包含主要内容的单词分类为候选关键字。这基本上是通过以下一些步骤来完成的,首先,文档文本被特定的单词分隔符分割成一个单词数组,其次,该数组再次被分割成一个在短语分隔符和停用单词位置的连续单词序列。最后,位于相同序列中的单词被分配到文本中的相同位置,并一起被视为候选关键字。
stopwordpattern = rake.build_stop_word_regex(filepath)
phraseList = rake.generate_candidate_keywords(sentenceList, stopwordpattern)
关键词得分
从文本数据中识别出所有候选关键字后,将生成单词共现图,该图计算每个候选关键字的分数,并定义为成员单词分数。借助该图,我们根据图中顶点的程度和频率评估了计算单词分数的几个指标。
keywordcandidates = rake.generate_candidate_keyword_scores(phraseList, wordscores)
提取关键词
计算候选关键字得分后,将从文档中选择前T个候选关键字。T值是图中字数的三分之一。
totalKeywords = len(sortedKeywords)
for keyword in sortedKeywords[0:(totalKeywords / 3)]:
print “Keyword: “, keyword[0], “, score: “, keyword[1]
另一个库
# pip install multi_rake
from multi_rake import Rake
rake = Rake()
keywords = rake.apply(full_text)
print(keywords[:10])
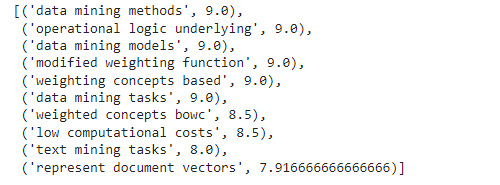
TextRank
TextRank 是一种用于提取关键字和句子的无监督方法。它一个基于图的排序算法。其中每个节点都是一个单词,边表示单词之间的关系,这些关系是通过定义单词在预定大小的移动窗口内的共现而形成的。
该算法的灵感来自于 Google 用来对网站进行排名的 PageRank。它首先使用词性 (PoS) 对文本进行标记和注释。它只考虑单个单词。没有使用 n-gram,多词是后期重构的。
TextRank算法是利用局部词汇之间关系(共现窗口)对后续关键词进行排序,直接从文本本身抽取。其主要步骤如下:
把给定的文本T按照完整句子进行分割,即

对于每个句子,进行分词和词性标注处理,并过滤掉停用词,只保留指定词性的单词,如名词、动词、形容词,即
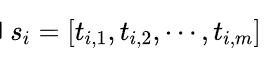
其中是保留后的候选关键词。
构建候选关键词图G=(V, E) ,其中V为节点集,由(2)生成的候选关键词组成,然后采用共现关系co-occurrence构造任两点之间的边,两个节点之间存在边仅当它们对应的词汇在长度为K的窗口中共现,K表示窗口大小,即最多共现K个单词。
根据上面公式,迭代传播各节点的权重,直至收敛。
对节点权重进行倒序排序,从而得到最重要的T个单词,作为候选关键词。
由(5)得到最重要的T个单词,在原始文本中进行标记,若形成相邻词组,则组合成多词关键词。例如,文本中有句子“Matlab code for plotting ambiguity function”,如果“Matlab”和“code”均属于候选关键词,则组合成“Matlab code”加入关键词序列。
安装及使用
要使用Textrank生成关键字,必须首先安装 summa 包,然后必须导入模块 keywords。
pip install summa
from summa import keywords
之后,只需调用 keywords 函数并将要处理的文本传递给它。我们还将 scores 设置为 True 以打印出每个结果关键字的相关性。
TR_keywords = keywords.keywords(full_text, scores=True)
print(TR_keywords[0:10])
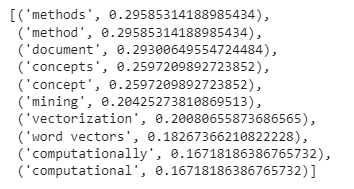
KeyBERT
KeyBERT[4]是一种简单易用的关键字提取算法,它利用 SBERT 嵌入从文档中生成与文档更相似的关键字和关键短语。首先,使用 sentences-BERT 模型生成文档embedding。然后为 N-gram 短语提取词的embedding。然后使用余弦相似度测量每个关键短语与文档的相似度。最后将最相似的词识别为最能描述整个文档并被视为关键字的词。
安装和使用
要使用 keybert 生成关键字,必须先安装 keybert 包,然后才能导入模块 keyBERT。
pip install keybert
from keybert import KeyBERT
然后创建一个接受一个参数的 keyBERT 实例,即 Sentences-Bert 模型。可以从以下来源[5]中选择想要的任何embedding模型。根据作者的说法,all-mpnet-base-v2模型是最好的。
kw_model = KeyBERT(model='all-mpnet-base-v2')
它将像这样开始下载:
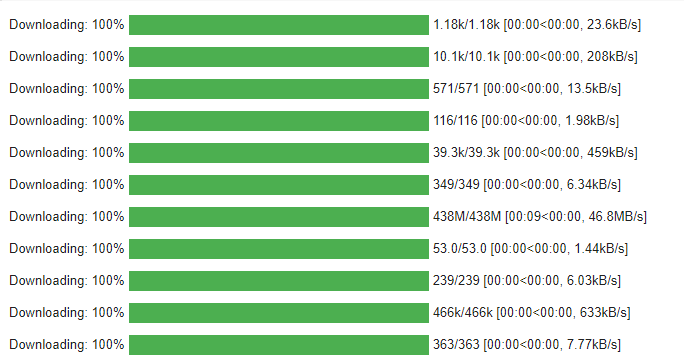
下载 BERT 预训练模型
keywords = kw_model.extract_keywords(full_text,
keyphrase_ngram_range=(1, 3),
stop_words='english',
highlight=False,
top_n=10)
keywords_list= list(dict(keywords).keys())
print(keywords_list)
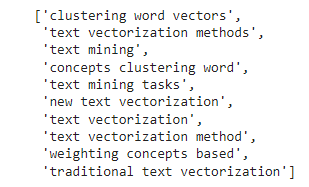
考虑到大多数关键短语的长度在 1 到 2 之间,可以将 keyphrase_ngram_range 更改为 (1,2)。这次我们将 highlight 设置为 true。
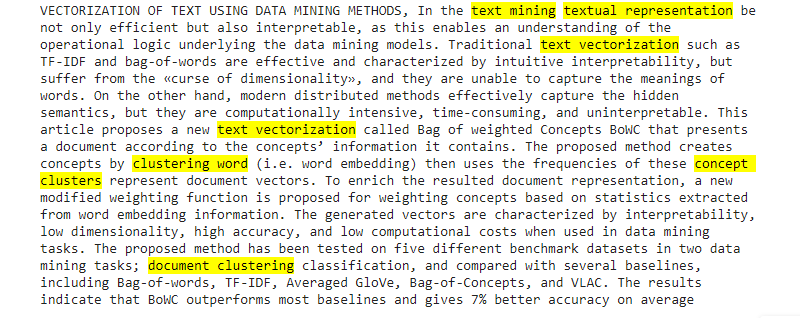
参考资料
[1]
文章: https://www.researchgate.net/publication/353592446_TEXT_VECTORIZATION_USING_DATA_MINING_METHODS
[2]
论文: https://www.sciencedirect.com/science/article/abs/pii/S0020025519308588
[3]
yake包: https://github.com/LIAAD/yake
[4]
KeyBERT: https://github.com/MaartenGr/KeyBERT
[5]
pretrained_models: https://www.sbert.net/docs/pretrained_models.html
[6]
[7]
https://blog.csdn.net/chinwuforwork/article/details/77993277