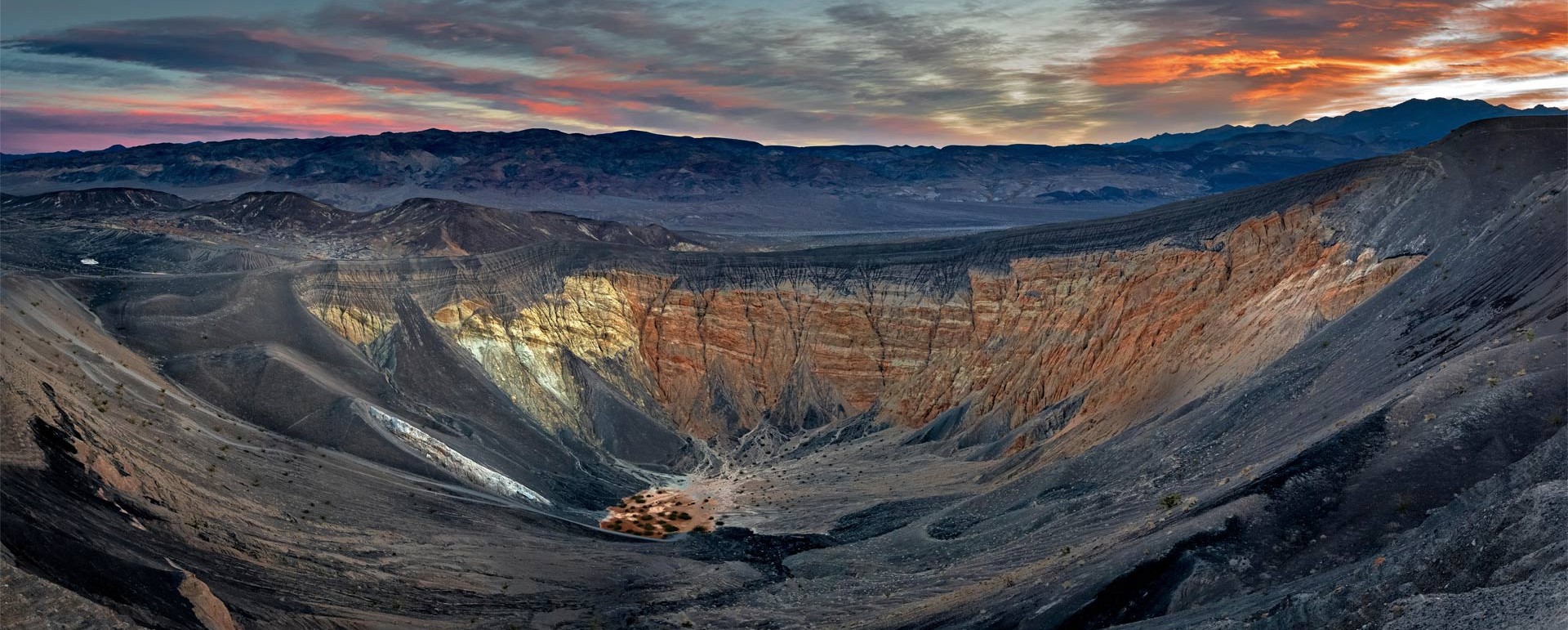
Python: usage and code examples of scipy.signal.coherence
1. scipy.signal.coherence Usage
scipy.signal.coherence
What it does in Python : Evaluate a discrete-time signal XX using Welch's methodX与YYMagnitude Squared Coherence Estimation of Y (MSC, Magnitude Squared Coherence Estimation)C xy C_{xy}Cxy。 C x y = ∣ P x y ∣ 2 / ( P x x × P y y ) C_{xy} = |P_{xy}|^{2/(P_{xx} \times P_{yy})} Cxy=∣Pxy∣2/(Pxx×Pyy) , whereP xx P_{xx}Pxx与P yy P_{yy}Pyyfor XXX与YYPower Spectral Density Estimation (PSD, Power Spectral Density Esitmation) of Y , P xy P_{xy}Pxyfor XXX与YYCross Spectral Density Estimation (CSD, Cross Spectral Density Estimation) of Y.
usage API
scipy.signal.coherence(
x, # (array_like) 测量值的时间序列
y, # (array_like) 测量值的时间序列
fs=1.0, # (float) x 与 y 时间序列的采样率
windows='hann', # (str, tuple 或 数组) 窗口
nperseg=None, # (int) 每个段的长度,如果为 str 或 tuple,则设置为256
noverlap=None, # (int) 段之间重叠的点数
nfft=None, # (int) 如果需要用 0 填充 FFT,则使用 FFT 的长度
detrend='constant', # (str) 制定如何去除每段的趋势
axis=-1 # (int) 计算相关性的轴
)
return value
- (1)
f: ndarray
: Number of sampling frequency groups; - (2)
Cxy: ndarray
: x x x与yyMagnitude-squared dependence of y .
2. Examples
from scipy import signal
import numpy as np
import matplotlib.pyplot as plt
# 下面代码生成两个具有一共同特征的测试信号
fs = 16000 # 采样频率
N = 1e5 # 采样点数
amp = 50 # 信号幅值
freq = 1000 # 信号的特征频率
noise_power = 0.001 * fs / 2 # 信号的噪声功率
time = np.arange(N) / fs # 信号的时间轴
b, a = signal.butter(2, 0.25, 'low') # 构造 butter 滤波器
x = np.random.normal(scale=np.sqrt(noise_power), size=time.shape)
y = signal.lfilter(b, a, x)
x += amp*np.sin(2*np.pi*freq*time)
y += np.random.normal(scale=0.1*np.sqrt(noise_power), size=time.shape)
# 计算 x 与 y 的幅度平方相关估计
f, Cxy = signal.coherence(x, y, fs, nperseg=1024)
# 使用 welch 方法分别计算 x 与 y 各自的功率谱密度估计
f, Pxx = signal.welch(x, fs, nperseg=1024)
f, Pyy = signal.welch(y, fs, nperseg=1024)
# 下面绘制结果
lnxy = plt.semilogy(f, Cxy, color='r', label='$C_{xy}$')
plt.ylabel('Coherence')
# 绘制第二个 y 轴,用于标注 x 与 y 的功率谱密度估计
plt.twinx()
lnx = plt.semilogy(f, Pxx, color='g', label="$P_{xx}$")
lny = plt.semilogy(f, Pyy, color='b', label="$P_{yy}$")
plt.ylabel('PSD [$V^2/Hz$]')
plt.xlabel('frequency [Hz]')
# 绘制图例
lns = lnxy + lnx + lny
labs = [l.get_label() for l in lns]
plt.legend(lns, labs)
plt.show()
The result of code execution is shown in the figure below:
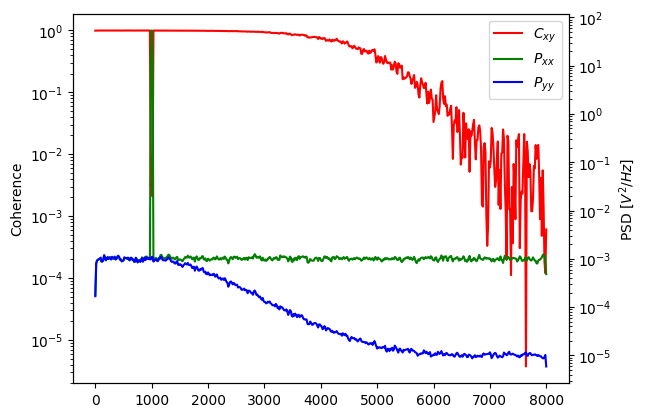