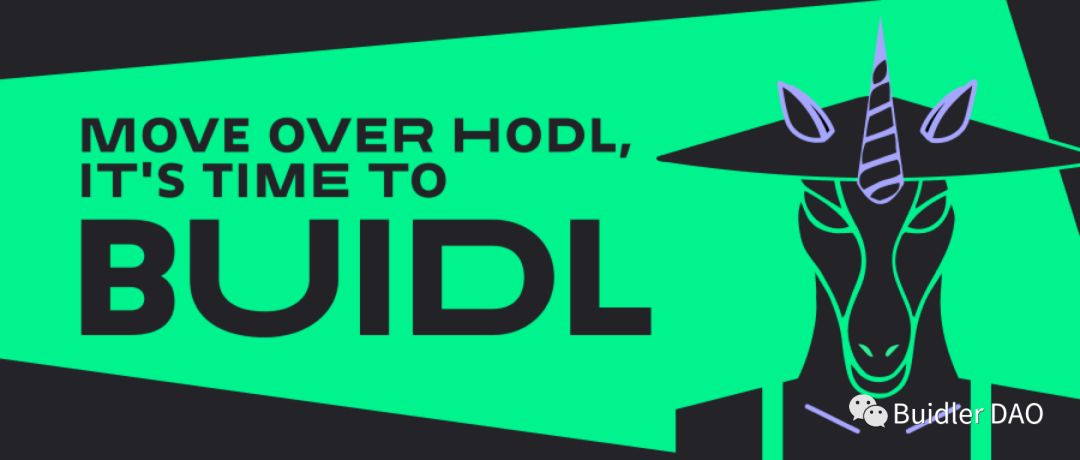
Buidler DAO: Web3 talent and project incubator
https://linktr.ee/buidlerdao
Host: @suki Co-host: @Julie
Documentation: @Axer
Article formatting: @Coucou
When we were founded and ideally, the blockchain was anonymous. The user is identified by a string of alphanumeric characters for the public key. But in reality, as applications become more widespread and standardized, people’s demand for security is far greater than their demand for privacy. Various on-chain analysis tools are also emerging in endlessly, and the privacy of cryptocurrency is gradually disintegrating. But privacy has always been a basic requirement to protect users and expand the use of cryptocurrency, and is considered to be one of the most important directions of web 3.0. This is also the topic we gathered tonight - the Privacy Track.
Note: This represents only my personal views and does not constitute any investment advice.
Guests and project introduction
Victor
@victorJi15|Manta network co-founder
Manta Network has been building infrastructure related to privacy technology. Users will have two sets of addresses on it, one is a public address and the other is a private address, called zkAddress. The assets above are also private assets and can be transferred freely. It mainly uses the technical principles of zero-knowledge proof.
William
@liukaikobe24|OASIS network Ecological Product Manager
OASIS network is a layer 1 public chain focusing on privacy. It has just launched a development environment that is EVM compatible and has privacy protection functions. It mainly uses the privacy computing technology of Trusted Execution Environment TEE.
w3tester
@xiao_zcloak|Founder of zClock network
zCloak Network is a set of privacy computing infrastructure based on W3C, DID standards and zero-knowledge proofs. We hope to help users solve the problem of using web2 data on the chain in a privacy-protecting manner, and return the data to users through "data available but not visible".
James
@have2changeA|PlatON network CTO
PlatON has a relatively lofty goal, which is to make private AI. By studying various algorithms and frameworks to build an infrastructure for private computing, attract experts and users from all fields to join the ecosystem, and finally achieve the vision of a private AI network. This allows users to contribute more data under the premise of data protection and collaborate together to generate greater value.
The full text is 8800 words, and the estimated reading time is 22 minutes.
TL:DR
▪ The boom in the entire market in 2021, the explosion of the Web3 concept, the rapid development of zk and other related technologies, and the increasing awareness of privacy among users are the reasons why this track has become one of the most recognized tracks in the capital market.
▪ Zero-knowledge proof solves the problems of blockchain’s scalability and lack of privacy by using only mathematical cryptography.
▪ The current mainstream privacy technologies can be simply classified into hardware implementation and software implementation according to the implementation logic:
Implemented by hardware : represented by the Trusted Execution Environment TEE, which inputs data into the hardware of the trusted execution environment for encrypted calculation and processing, and finally outputs an encrypted execution result that protects privacy.
Implementation by software : including privacy protection through algorithms, mathematics, cryptography and other knowledge. Representatives include:
Zero-knowledge proof (ZK): Mainly through the interactive proof of the prover and the verifier, the data is provable but not visible.
Multi-party secure computation (MPC): refers to the collaborative calculation of an agreed function by multiple participants in the absence of a trusted third party. Except for the calculation results, each participant cannot infer other participants through the interaction data during the calculation process. of original data.
Homomorphic encryption (HE): roughly divided into partial homomorphism, approximate homomorphism, finite series full homomorphism and complete homomorphism. It can be simply understood that with the help of homomorphic encryption, the effect of directly operating data on ciphertext is the same as operating data on plaintext and then encrypting it.